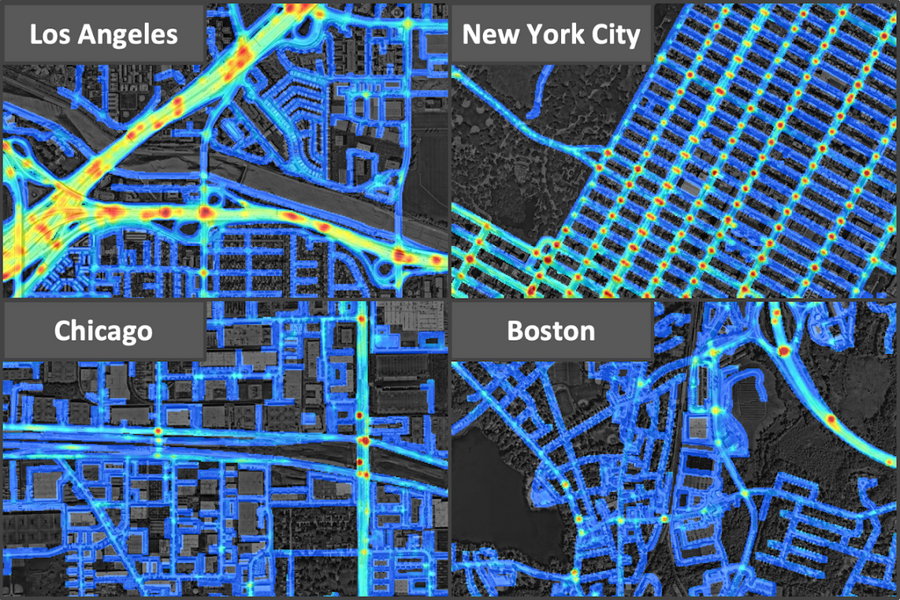
A neural network trained on historical data and satellite images predicts the probability of an accident on certain sections of the road with ultra-high resolution. The model is planned to be implemented in navigators to build safer routes.
Scientists from the Laboratory of Computer Science and Artificial Intelligence of the Massachusetts Institute of Technology and the Qatar Center for Artificial Intelligence have developed a deep learning model that plots the probability of an accident on road maps with a resolution of 5×5 meters. The model was trained on GPS tracking data, which provides information about the density, speed and direction of traffic, historical data on accidents, as well as satellite images that allow you to determine the number of lanes, the presence of curbs or large pedestrian flows.
The dataset covers 7,500 square kilometers in Los Angeles, New York, Chicago and Boston. Among the four cities, Los Angeles was the most unsafe, as it had the highest accident density, followed by New York, Chicago and Boston.
To evaluate the model, scientists trained the model on data for 2017 and 2018, and then tested its effectiveness in predicting accidents in 2019 and 2020. It is curious that many sections of roads were marked by the neural network as places of increased risk, despite the fact that no accidents were registered in them, but accidents occurred on them in subsequent years. Also, scientists have found that highways have a higher probability of an accident compared to nearby roads, and the maximum risk of accidents are exits from the highway.
The model is planned to be used to find safer routes, provide insurance companies with individual insurance plans based on customer trajectories and help architects design safer roads.