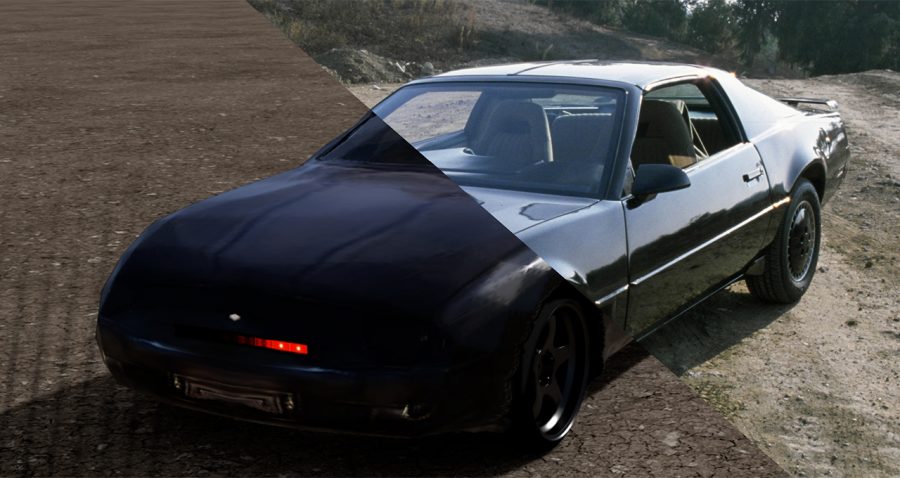
The neural network from NVIDIA allows one to transform a flat image into a realistic three-dimensional model that can be manipulated in virtual space. The new approach to generation of training data has significantly increased the accuracy of reconstruction while reducing the requirements for data labeling.
The new GANverse3D architecture consists of two neural networks that render images. The generative adversarial network (GAN) constructs a training dataset based on photos of vehicles published in the Internet, generating images of cars from different angles. For this purpose, the first four layers of the neural network were used, while the remaining 12 layers were frozen. By manually setting the positions of the viewpoints of vehicles photographed at a certain height and a certain distance from the camera, the researchers were able to quickly create a dataset with images of cars at different viewing angles from two-dimensional images. The output of the network consisted of 55,000 images for training. In addition to photos of vehicles, the researchers also developed datasets based on photos of animals (Fig. 1). The developed model allows one to reconstruct objects with different textures and shapes.
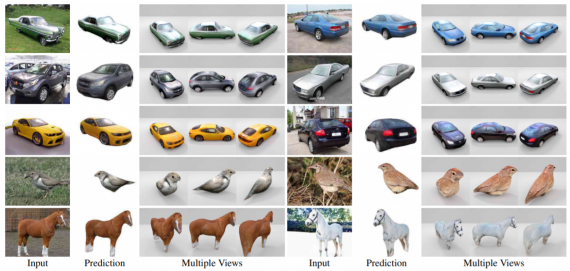
Datasets with real photos that show the same object from different angles are quite rare. Therefore, previously, datasets with artificially generated three-dimensional models of objects (for example, ShapeNet) were used to train inverse graphics neural networks. The new approach has reduced the number of data labels in the dataset by more than 10,000 times. The resulting sets of photos of vehicles at different angles were fed to the input of a inverse graphics neural network that extracts a three-dimensional model of an object from two-dimensional images. After GANverse3D training, only one image is required to build a three-dimensional model. Using real images for training instead of artificially generated data allows one to use GANverse3D in existing 3D rendering applications. A model trained on 55,000 images of cars created by a GAN outperformed inverse graphics neural network trained using the popular Pascal3D dataset.
The use of a combination of two neural networks that perform rendering – GAN and inverse graphics network – leads to an improvement in the quality of reconstruction of three-dimensional models from the image from one angle. GANverse3D will allow architects, designers, and game developers to add new objects to three-dimensional layouts without the cost of rendering.