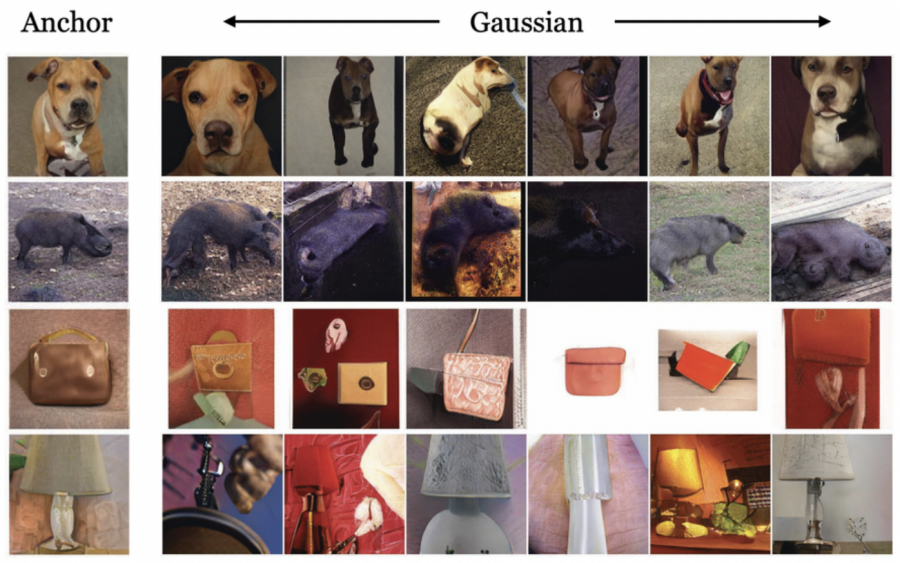
MIT researchers have developed a method in which a controlled model of synthetic image generation is integrated into a classification model. The method allows you to reduce the cost of collecting large-scale datasets.
Creating datasets for classification can cost millions of dollars. However, for some tasks, suitable data does not exist in principle, and even the best datasets are often subject to the problem of bias.
To circumvent these difficulties, MIT scientists proposed instead of using datasets to generate realistic synthetic data that can train a classification model. Their results show that the contrast learning model, trained using only synthetic data, is able to study visual representations that compete or even surpass those obtained on real data.
One of the key advantages of such a model is a multiple reduction in the amount of memory required to store the dataset. The use of synthetic data can also circumvent some privacy and copyright issues that limit the ability to distribute some real data.
The generative model can also be edited to remove certain attributes, such as race or gender, which will help eliminate some of the biases that exist in traditional datasets.