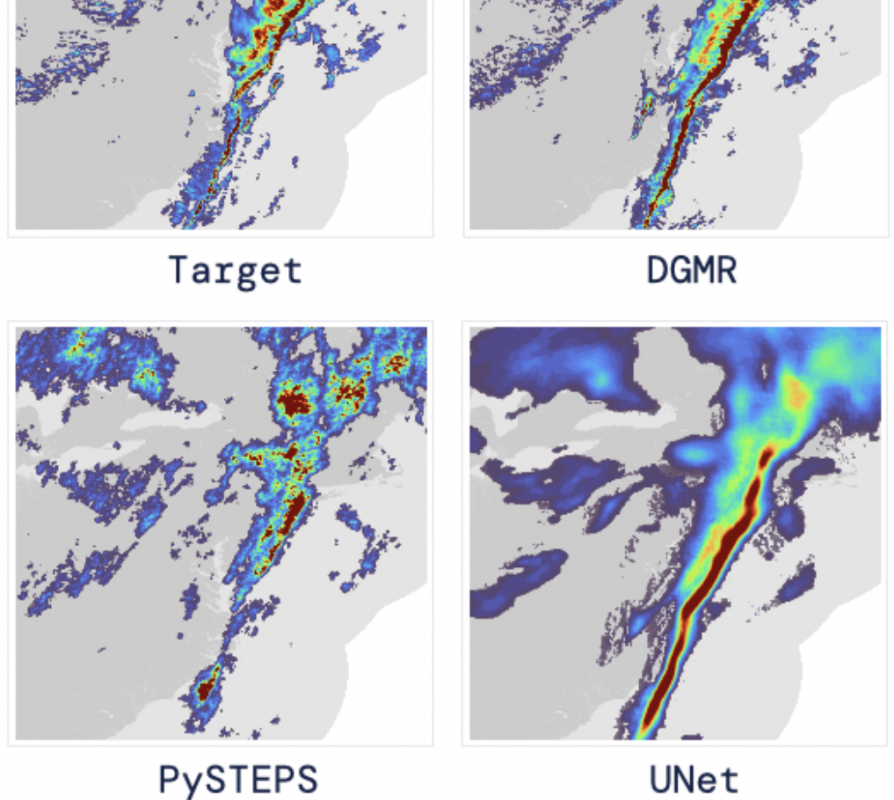
DeepMind has introduced a generative-adversarial DGMR network for weather prediction. DGMR has the highest accuracy of short-term forecasts among similar models.
Rain forecasting is crucial for many industries, including aviation and emergency services. The best existing methods of weather forecasting are based on large-scale computer simulation of atmospheric physics. They work well for long-term forecasting, but they have a large margin of error when making short-term forecasts.
The DeepMind team trained the model on radar data. Many countries publish images of radar measurements that track the formation and movement of clouds. For example, in the UK, new data is published every five minutes. The researchers transferred this data to a generative-adversarial network trained to generate new data samples similar to the real data on which it was trained. After training, DGMR learned to generate synthetic radar images that continued the sequence of real measurements.
To test this approach, the team asked 56 forecasters from the British Weather Bureau to evaluate DGMR in a blind comparison with forecasts made using physical modeling and a competing deep learning tool. 89% of experts decided that DGMR forecasts have the highest accuracy.