Data Science and Machine Learning Courses Collection
Welcome to our comprehensive collection of Data Science and Machine Learning courses! Whether you’re a beginner or an experienced professional, our platform offers top-notch educational resources to meet the demands of the data-driven landscape. Our carefully curated courses cover statistics, programming, machine learning algorithms, and more.
Taught by industry experts, our flexible formats cater to all learning styles. With clear descriptions, learning objectives, and prerequisites, you can easily choose the best fit for your skill level and goals. Embark on this transformative learning experience and position yourself at the forefront of the data revolution. Enroll now and unlock a world of endless possibilities in data science.
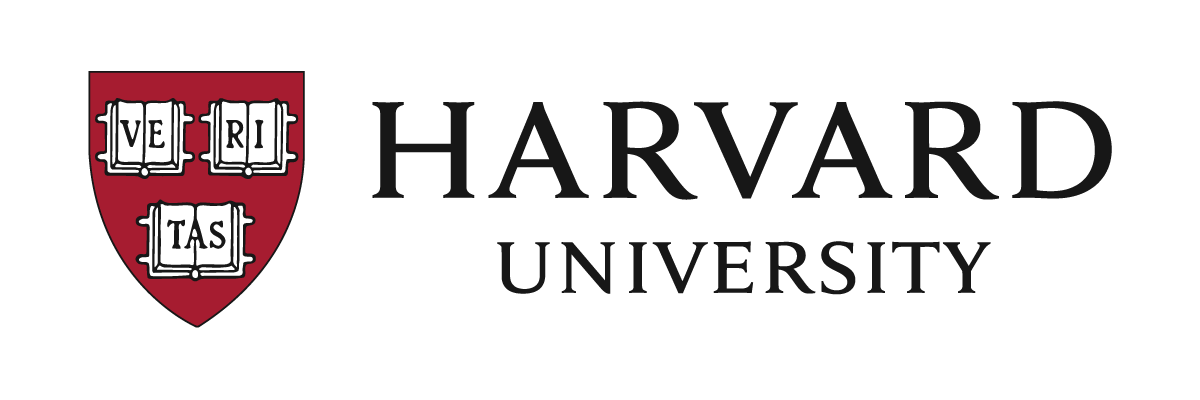
Data Science: Visualization
Description
In this course, students will learn basic data visualization principles and how to apply them using ggplot2. The course follows an incremental approach where students learn how to apply the learned techniques to small datasets initially, and then gradually transition to more complex real-world use-cases. The course is part of a Professional Certification.
Teachers:
- Harvard: Rafael Irizarry, Professor of Biostatistics
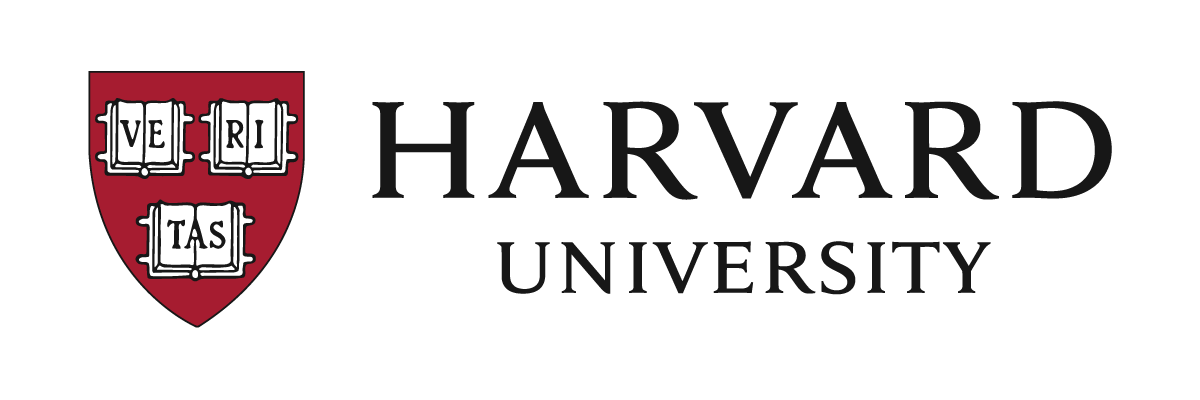
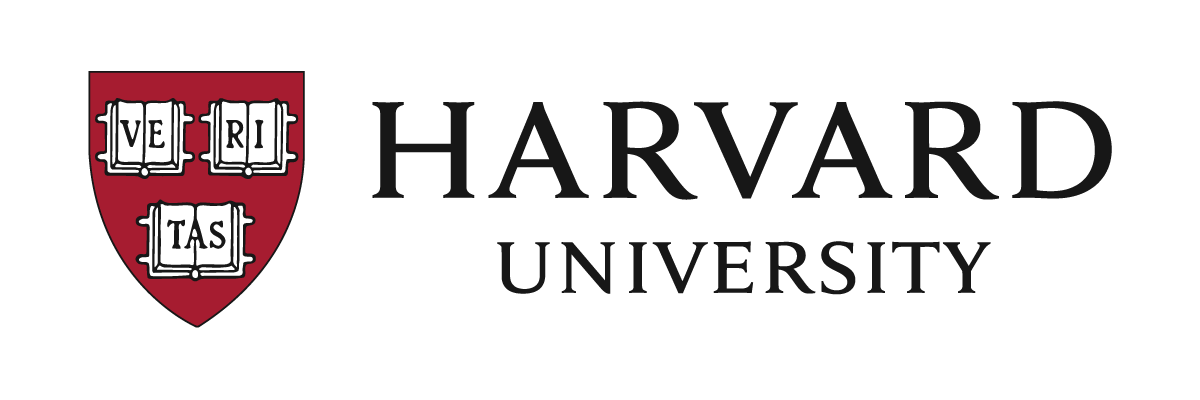
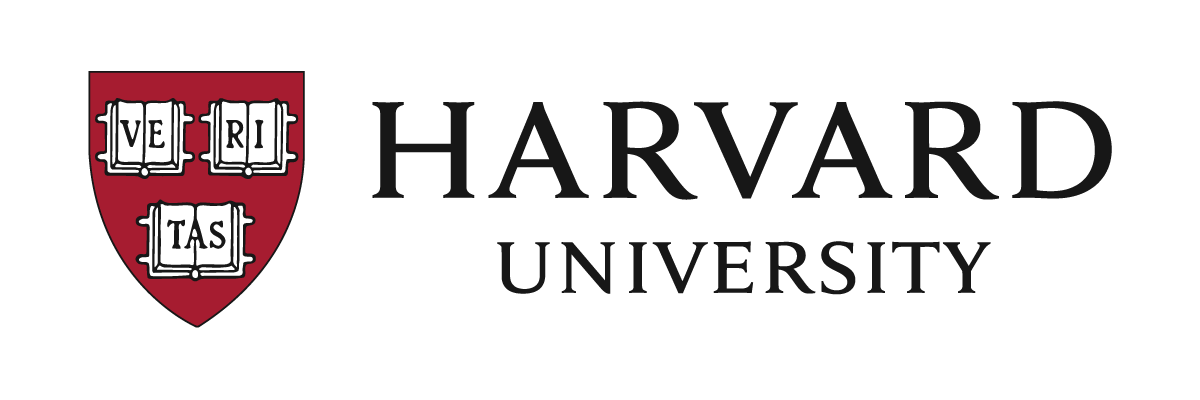
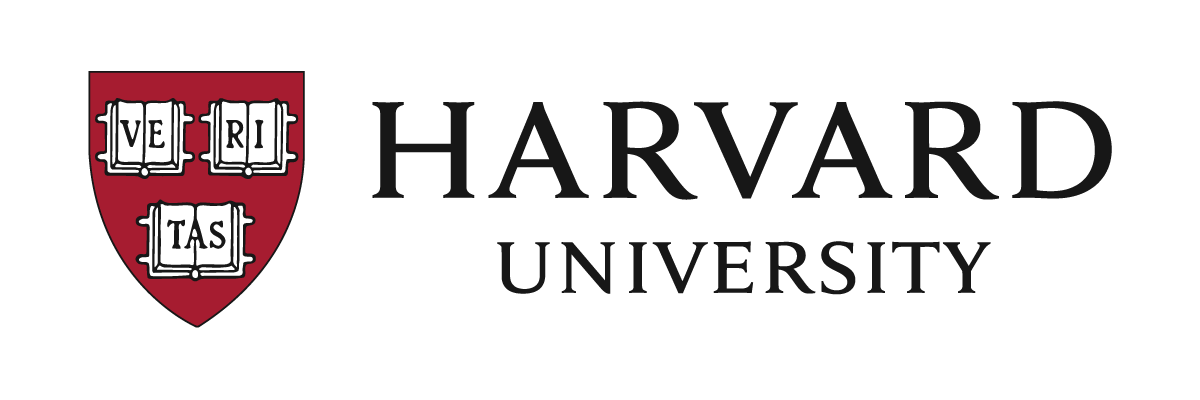
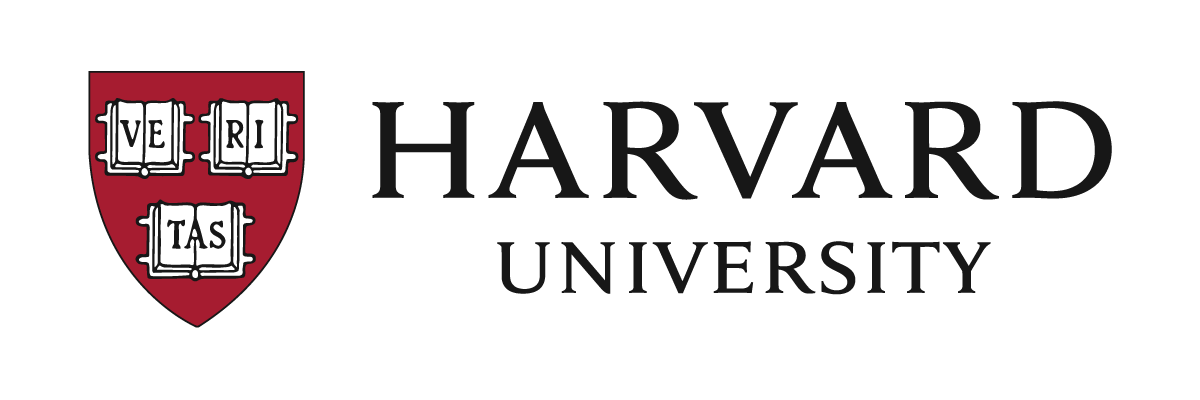
Data Science: R Basics
Description
This course will allow students to learn the basic programming with R and how to clean, analyze and visualize data using R and R packages. Students will work with a real-world dataset of crimes in the United States. The course is the first course in a Professional Certification.
Teachers:
- Harvard: Rafael Irizarry, Professor of Biostatistics
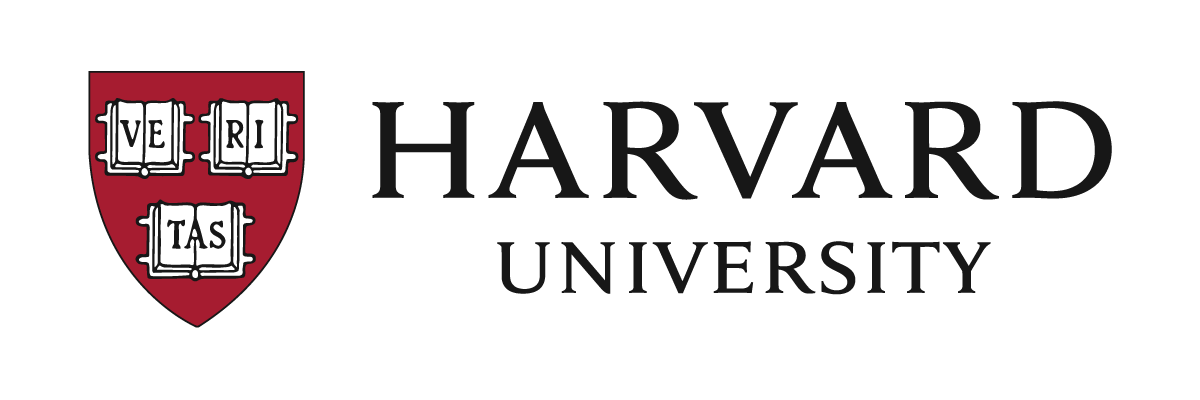
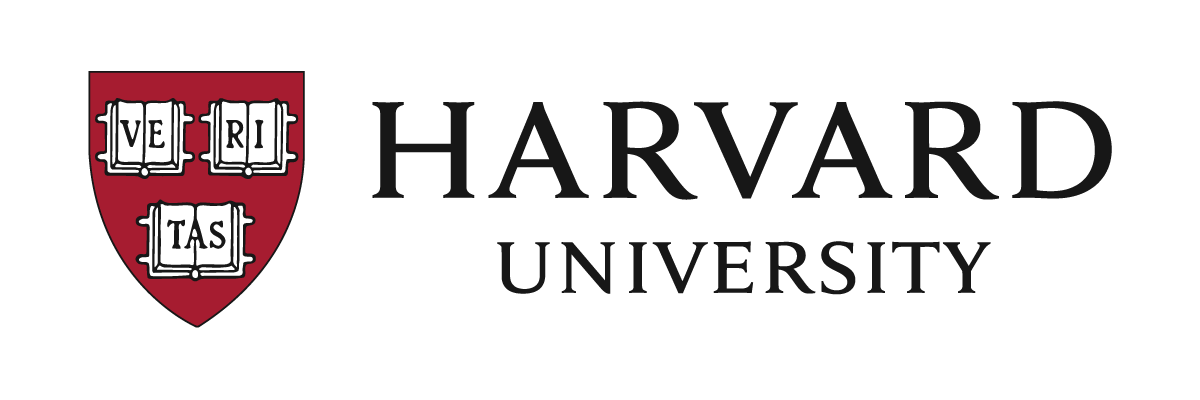
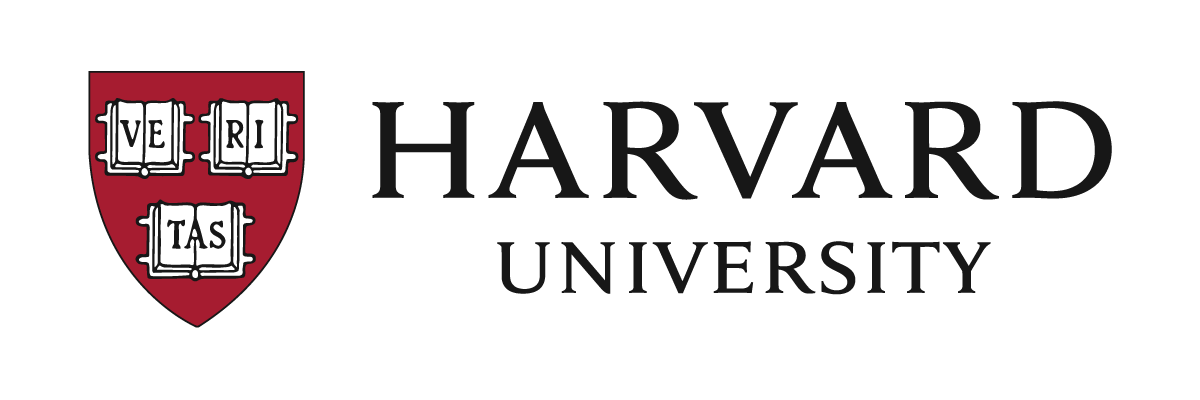
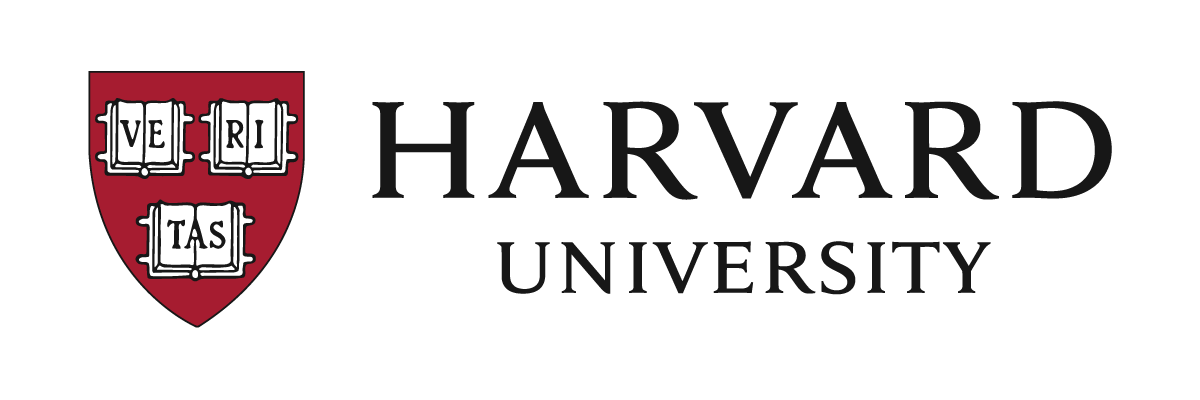
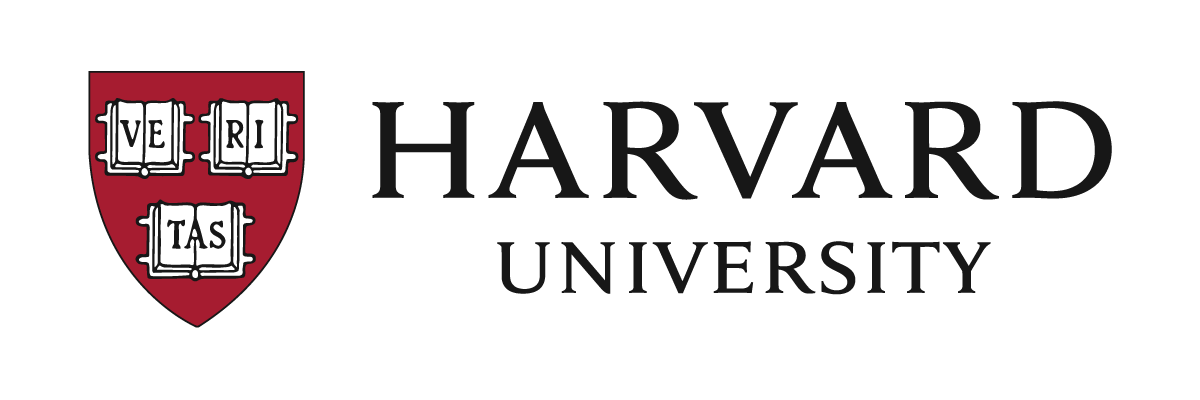
Data Science: Machine Learning
Description
In this Machine Learning course, students will learn the most popular and successful data science and machine learning techniques and will have the change to apply them to build a movie recommendation system. The course is part of a Professional Certification in Data Science.
Teachers:
- Harvard: Rafael Irizarry, Professor of Biostatistics
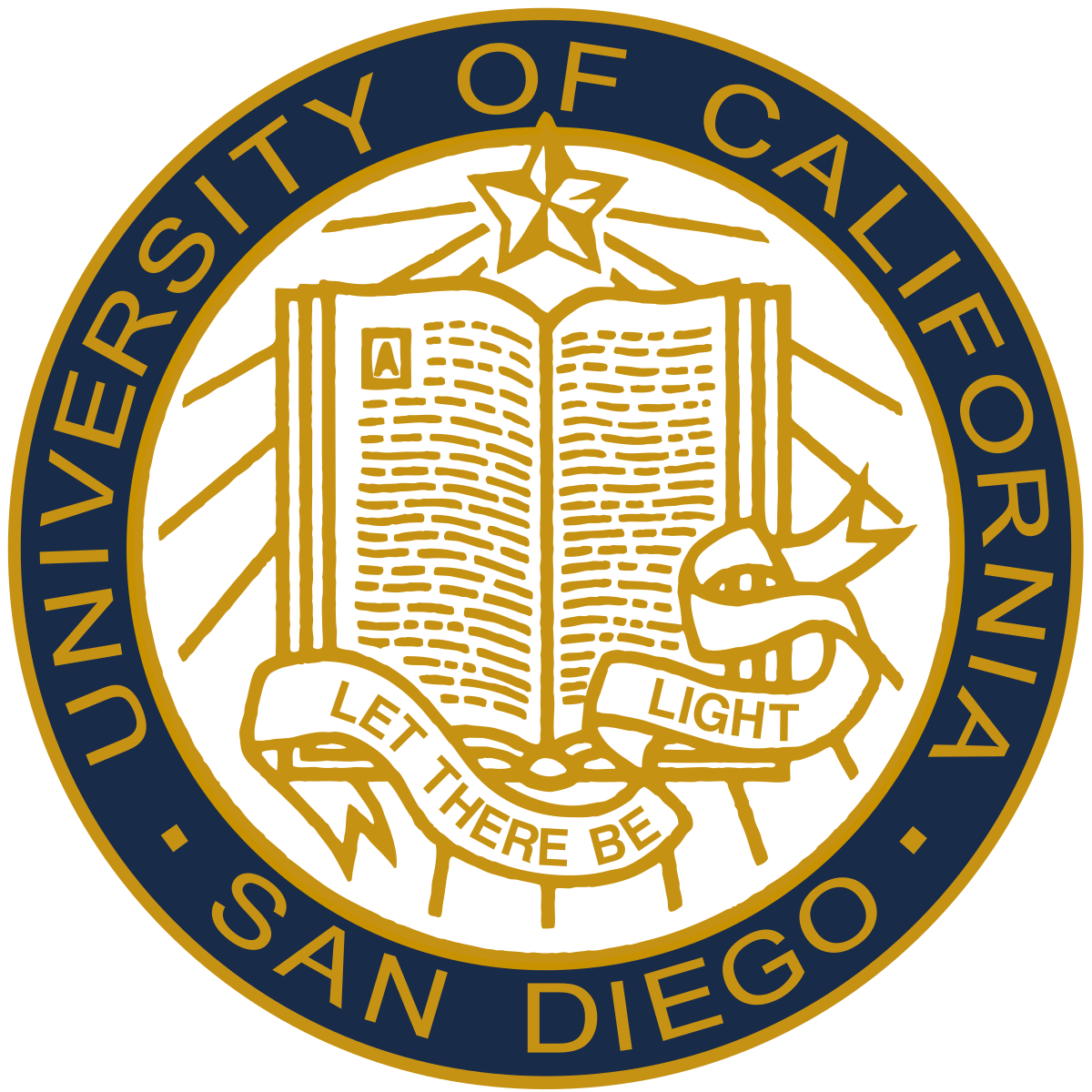
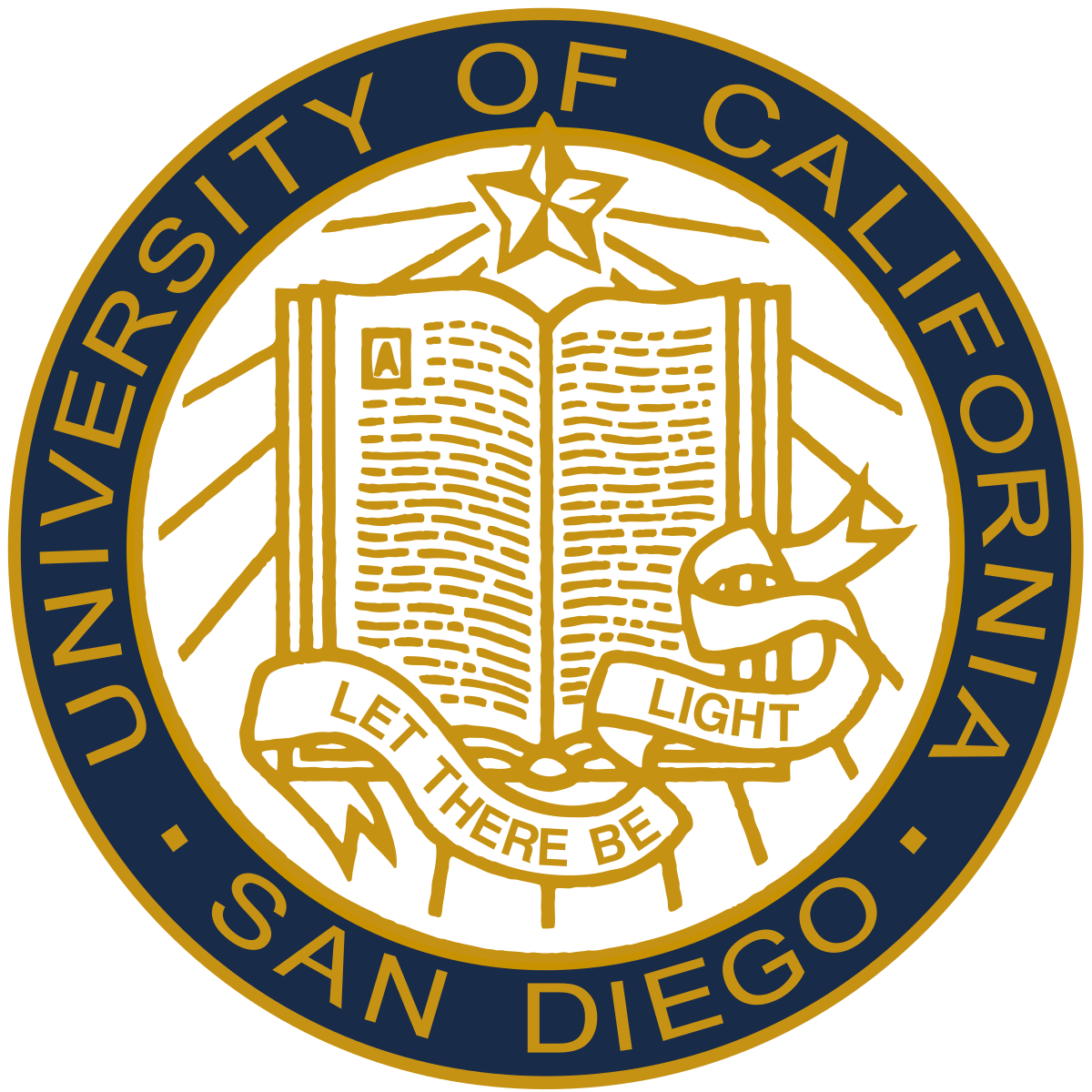
Master’s Degree in Data Science
Description
MicroMasters programme in Data Science offered by the University of UC San Diego. The program covers the following topics: Python programming and data analysis, probability and statistics, machine learning and big data analytics. Students will gain practical knowledge on how to clean and pre-process data, make reliable inferences from noisy data, apply machine learning techniques.
Teachers:
- UC San Diego: Leo Porter
- UC San Diego: Alon Orlitsky
- UC San Diego: Yoav Freund
- UC San Diego: Sanjoy Dasgupta
- UC San Diego: Ilkay Altinas
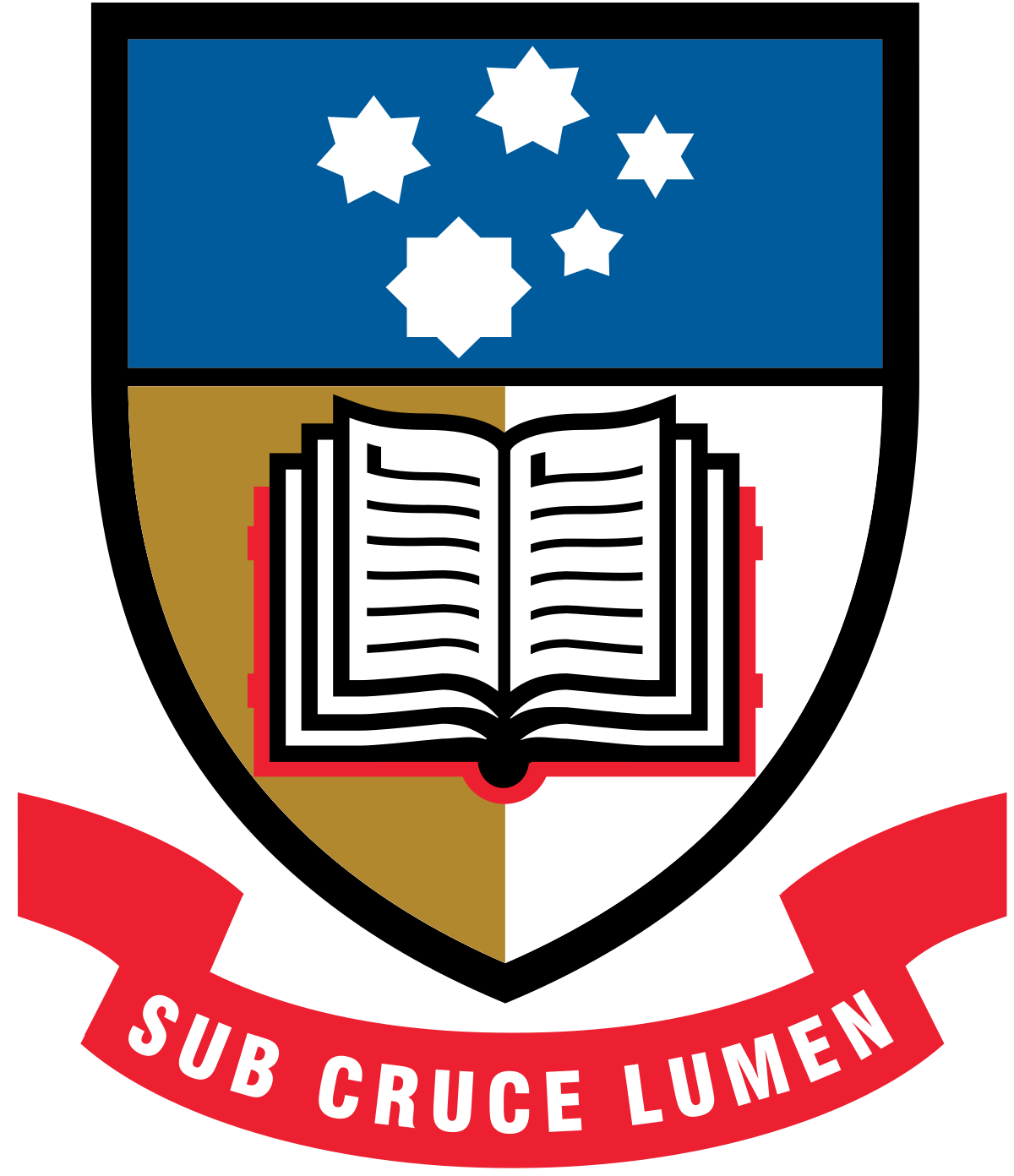
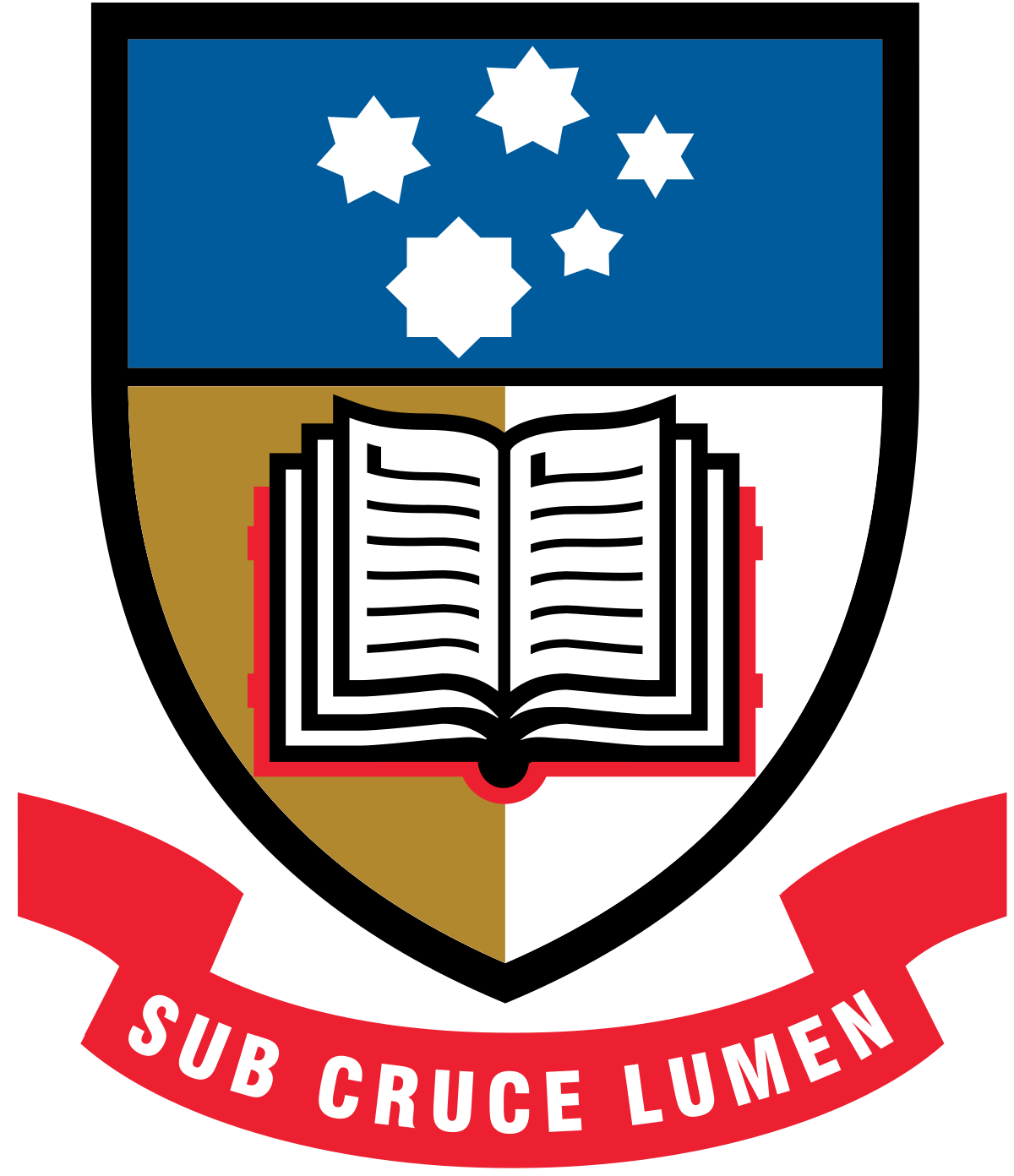
Big data
Description
A MicroMasters programme offered by the University of Adelaida. It consists of 5 credit-eligible courses that were designed to offer students the possibility to learn tools and analytical methods used for informed, data-driven decision making. Students will learn how to organize, clean and process data at scale.
Teachers:
- University of Adelaida: Katrina Falkner Head - School of Computer Science
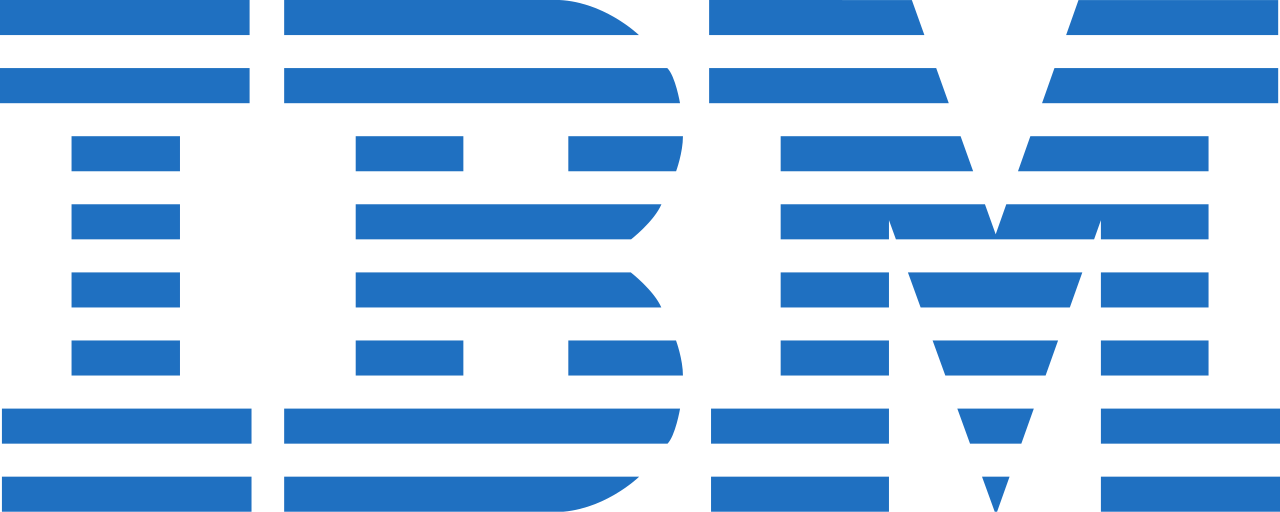
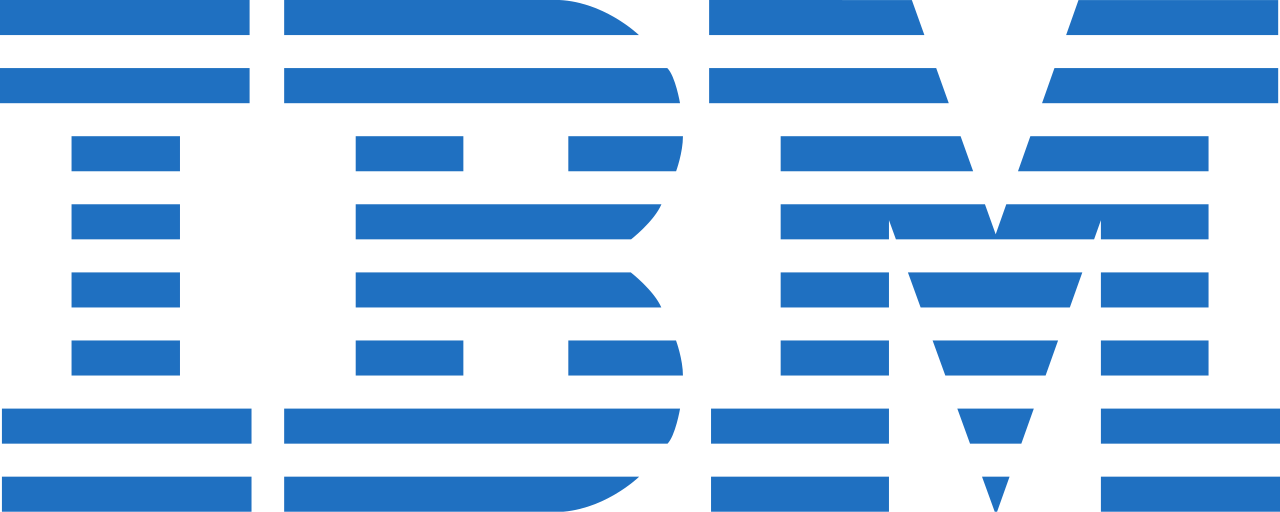
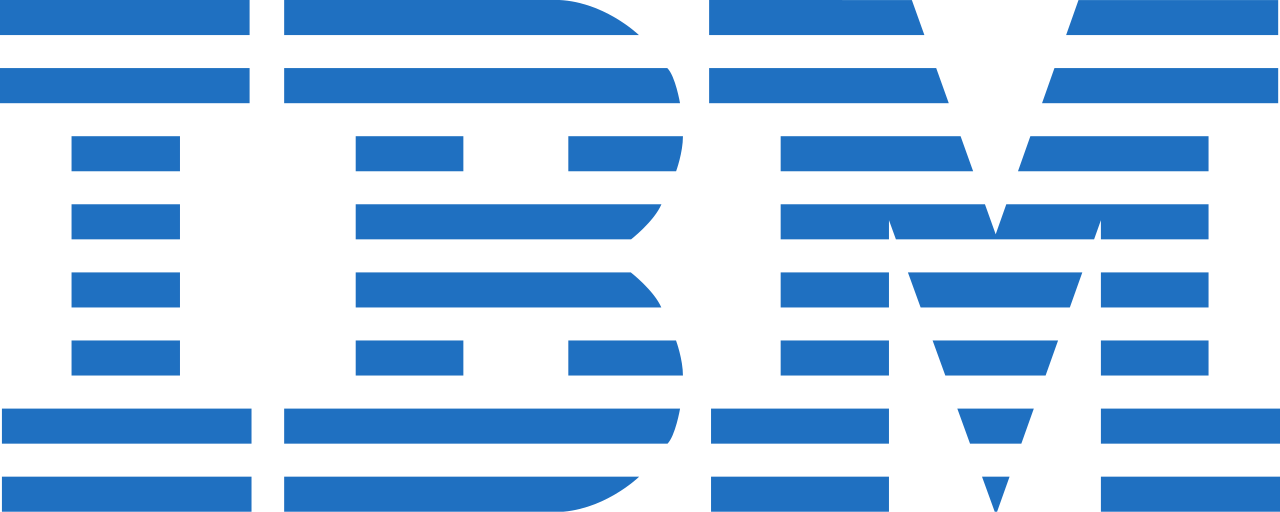
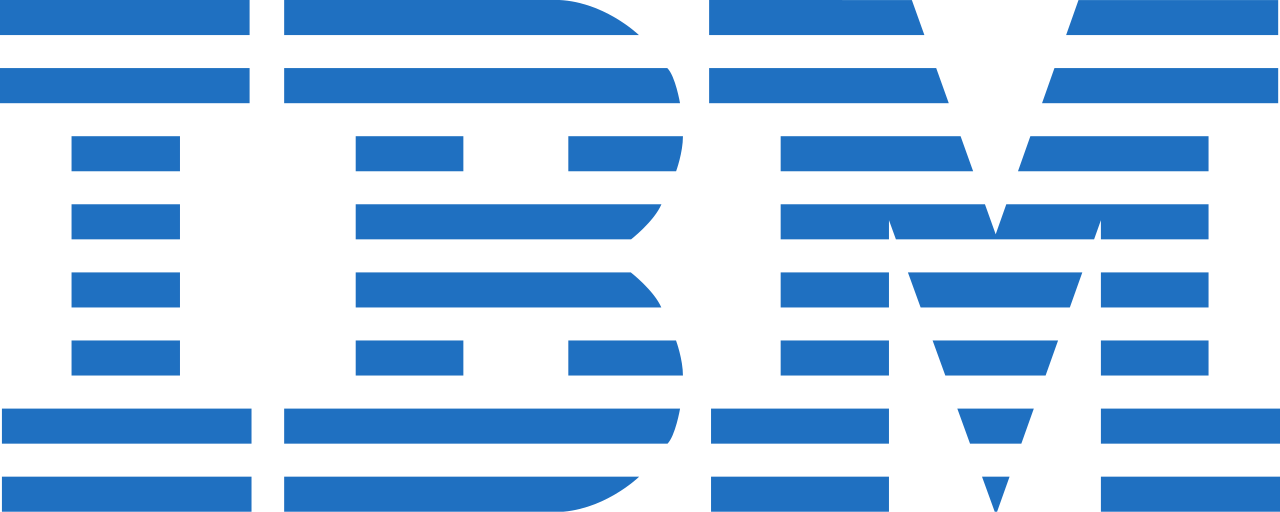
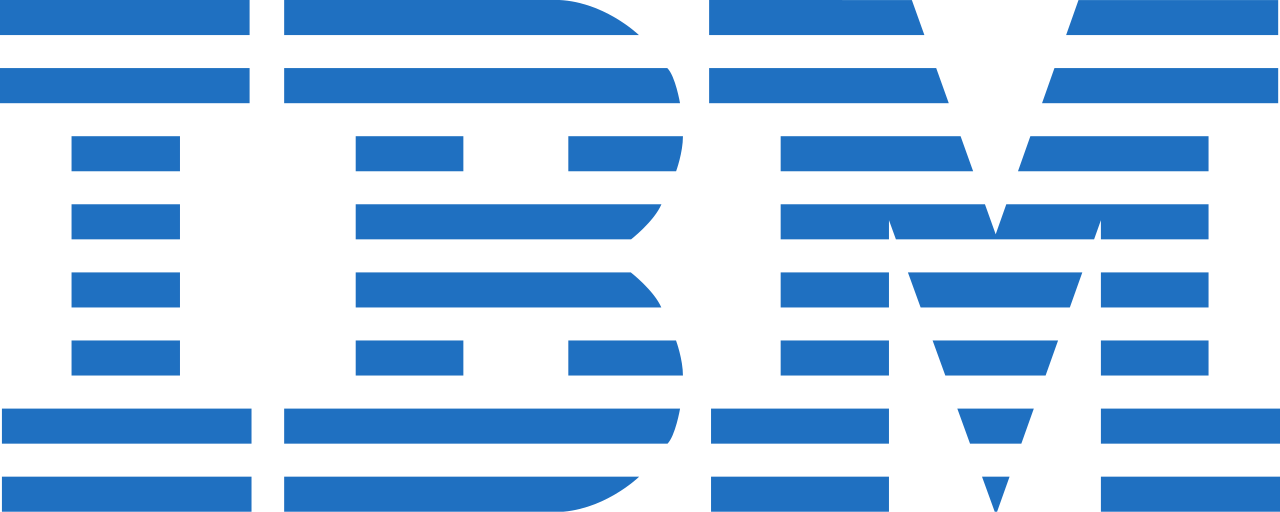
Deep Learning
Description
A professional certification provided by IBM, consisting of five Deep Learning courses. Students will have the opportunity to learn the core concepts of deep learning from both theoretical and practical experience. The courses cover building, training and deploying different types of deep neural networks using the popular Deep Learning libraries: Keras, Pytorch and Tensorflow.
Teachers:
- IBM: Joseph Santarcangelo PhD., Data Scientist
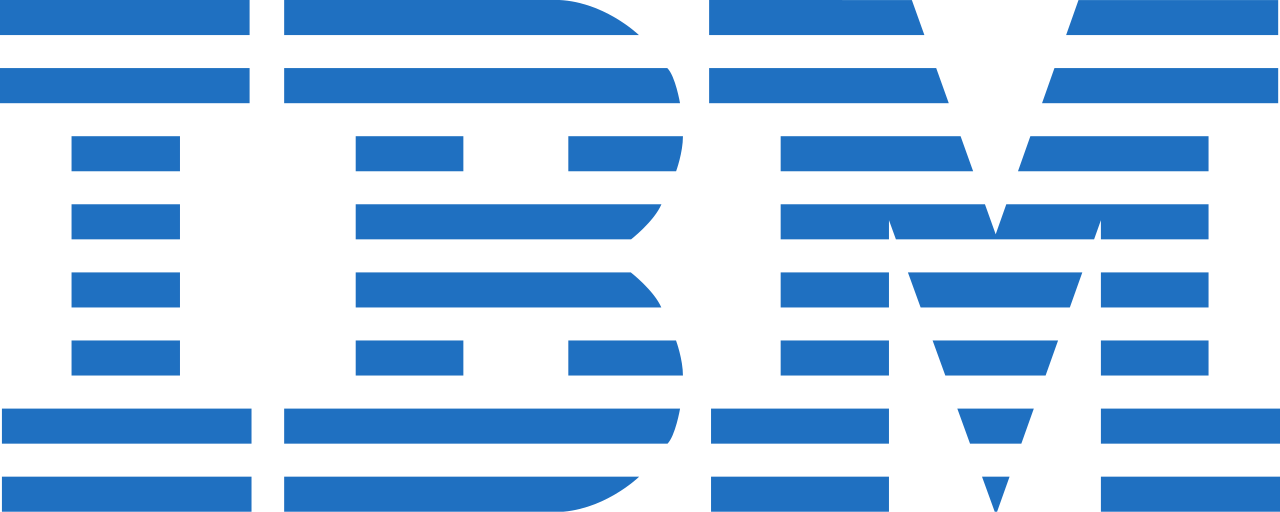
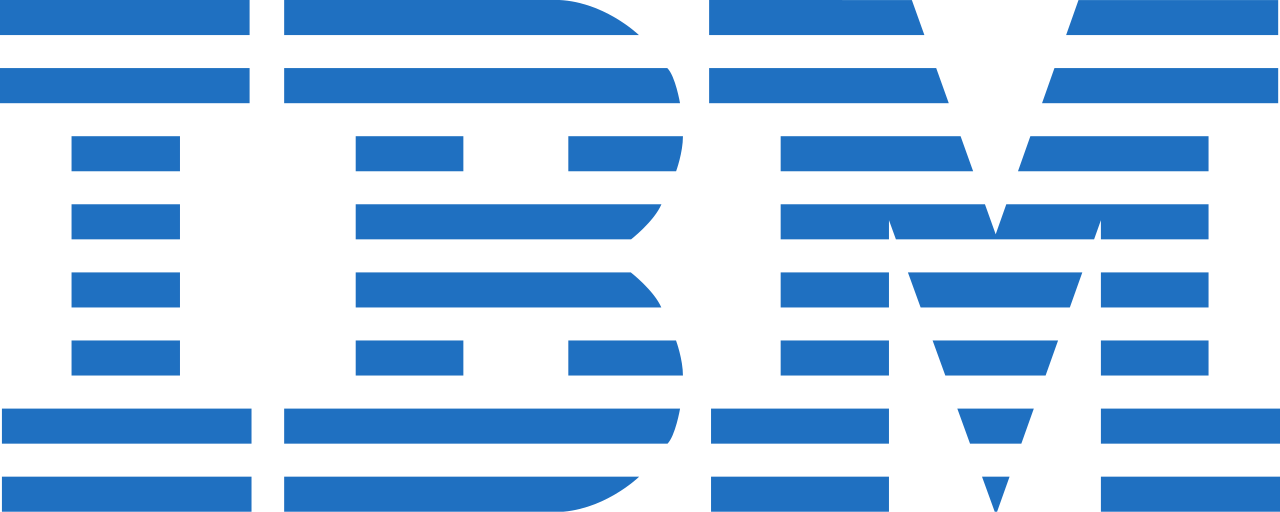
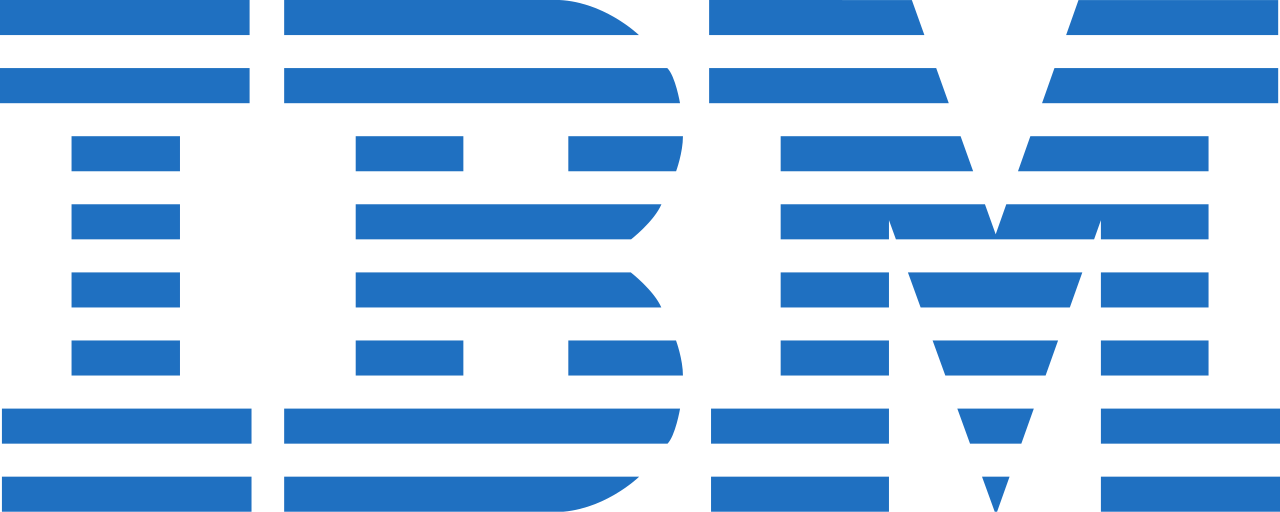
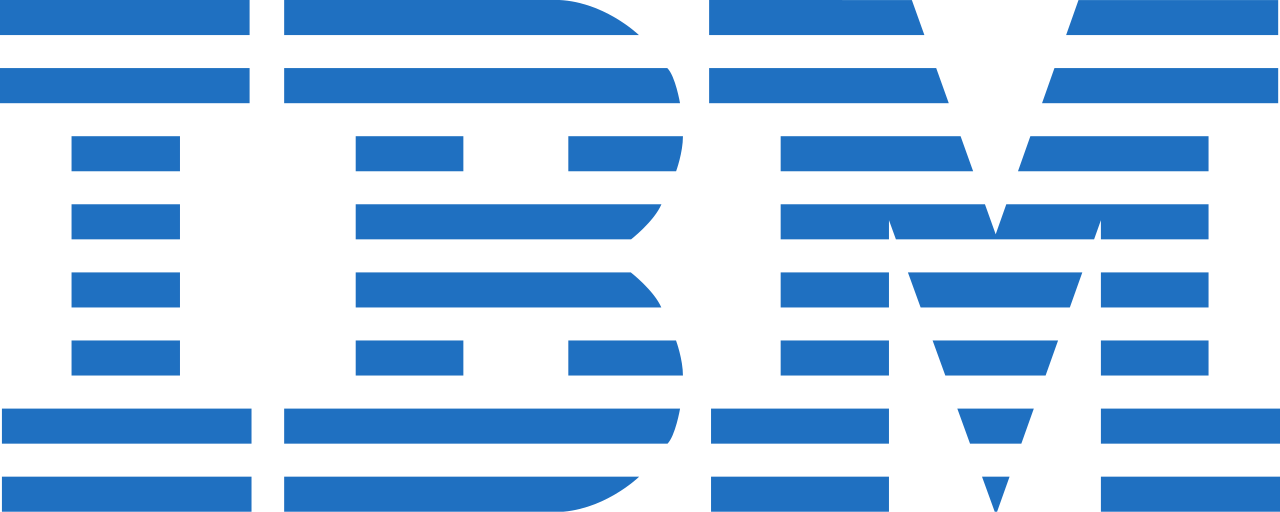
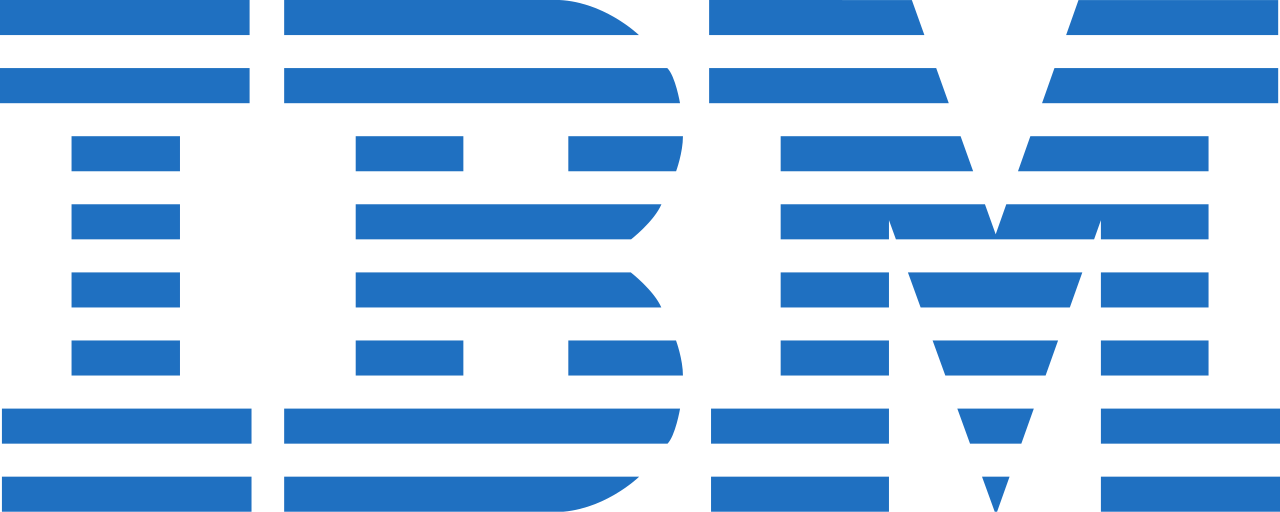
Data Science with Python
Description
This specialization consists of 5 high quality skill-building courses that cover the following topics: Python programming, Jupyter notebooks and interactive Data Science, Python libraries for machine learning and visualization and applied Data Science. The certification is provided by IBM and courses are thought by IBM experts.
Teachers:
- IBM: Joseph Santarcangelo PhD., Data Scientist
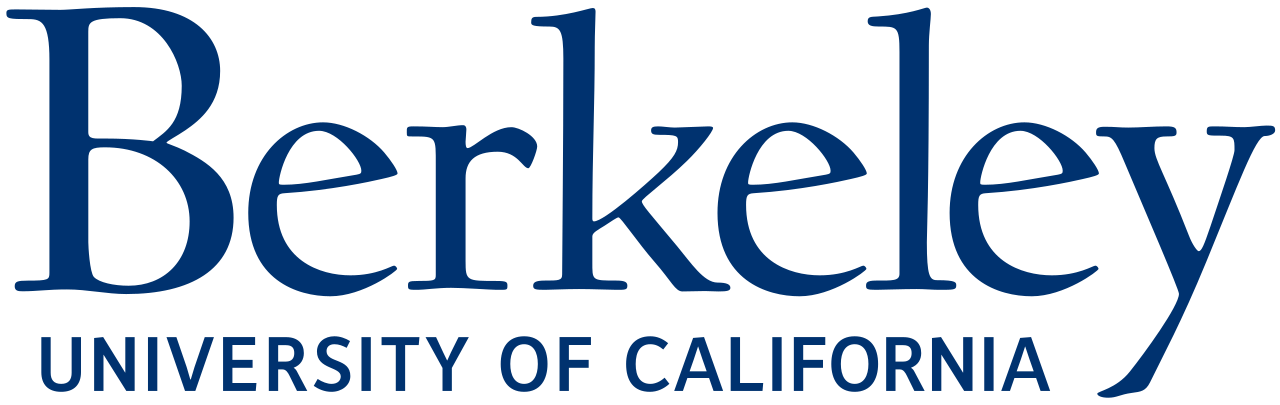
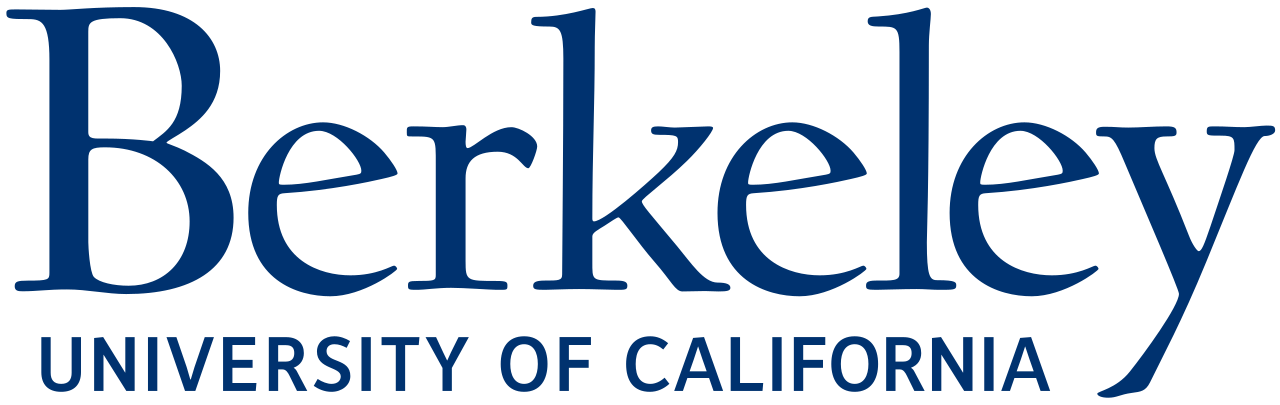
Foundations of Data Science
Description
Students will learn how to apply inferential statistics and machine learning techniques to real-world data from a wide range of different domains. The specialization consists of 3 courses: Computational thinking with Python, Inferential thinking through simulations, Machine learning, and predictions. The courses are offered by the University of California Berkeley.
Teachers:
- Berkeley University: David Wagner, Professor of Computer Science
- :
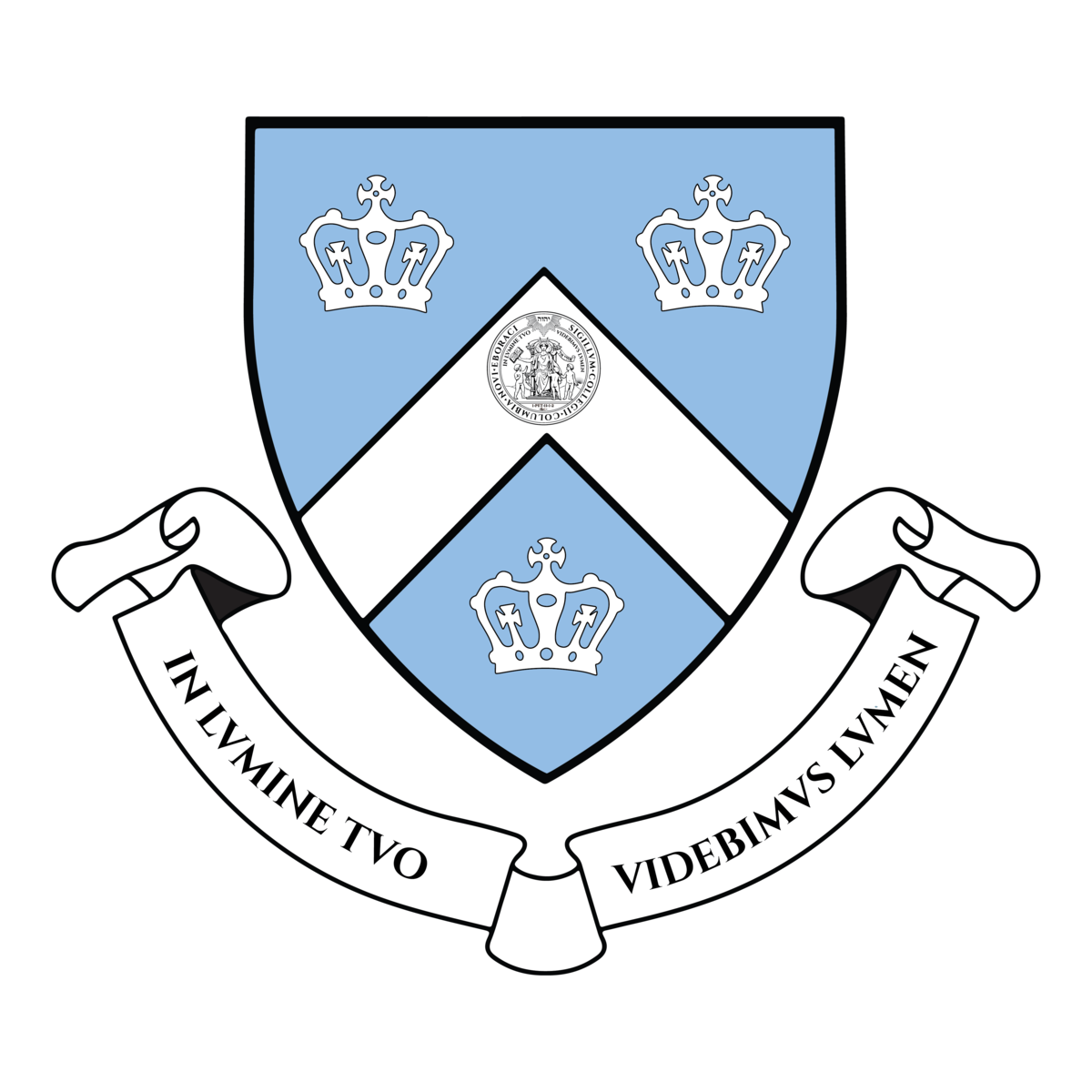
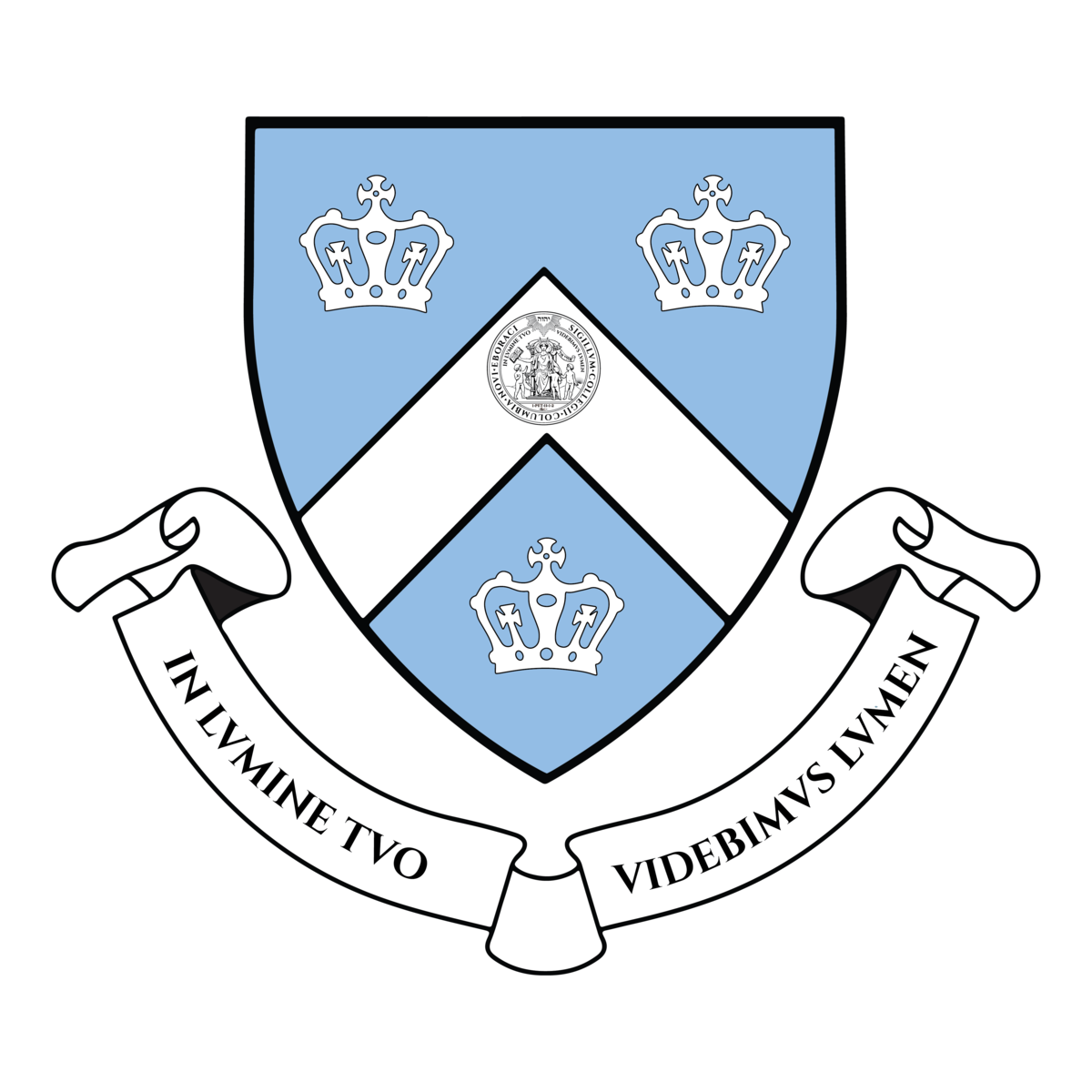
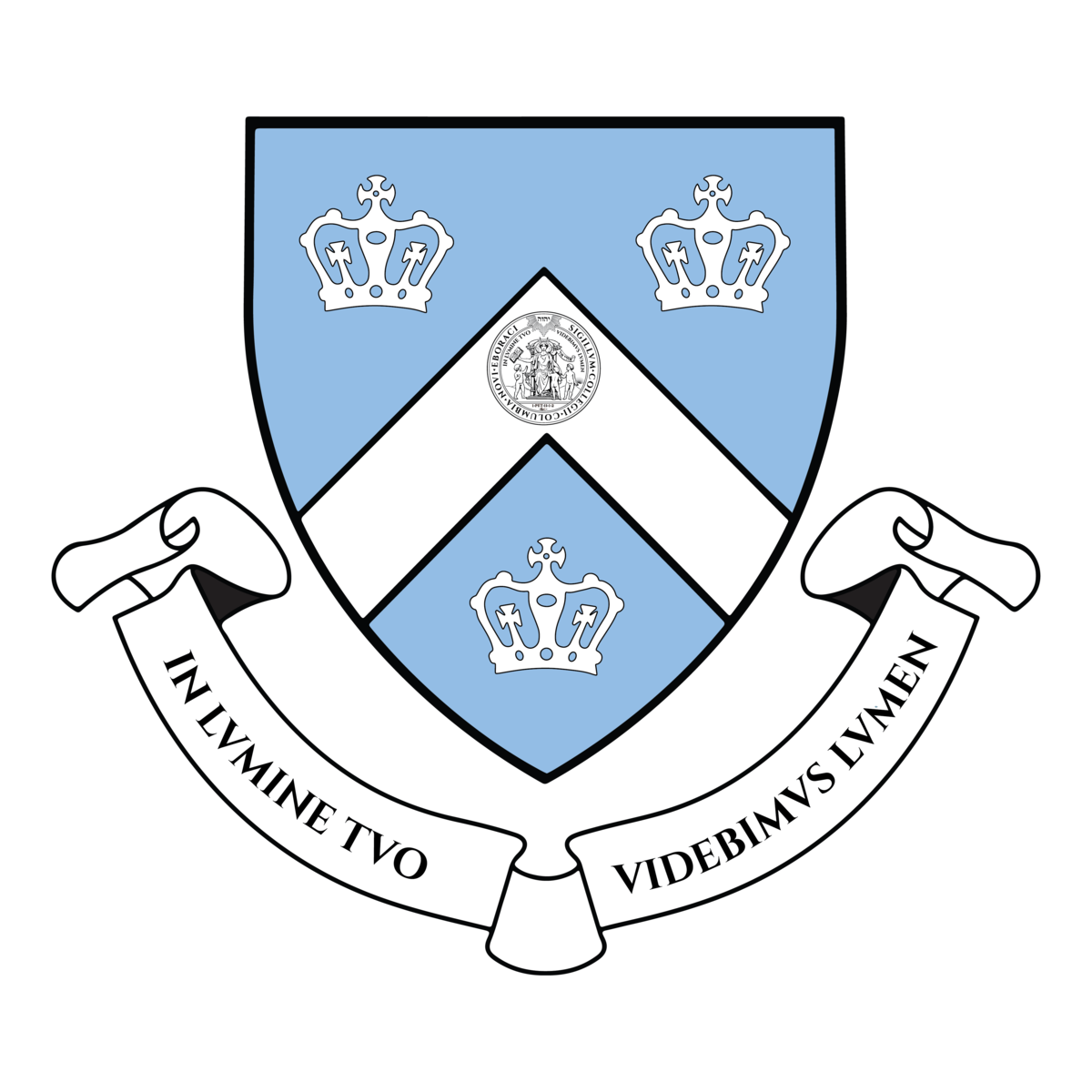
Artificial Intelligence
Description
This MicroMasters programme consists of 4 graduate-level courses that offer students to gain an in-depth understanding of the fundamentals of AI. Within the courses, students will learn how to apply machine learning techniques to real-world problems. They will gain knowledge on how to build and train deep neural networks and harness their power,
Teachers:
- Columbia University: Ansaf Salleb-Aouissi, Department of Computer Science, CU
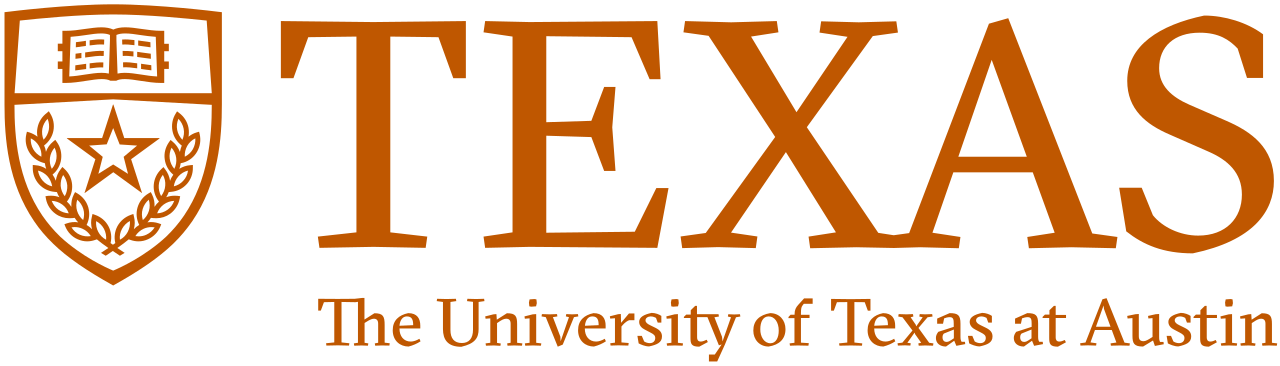
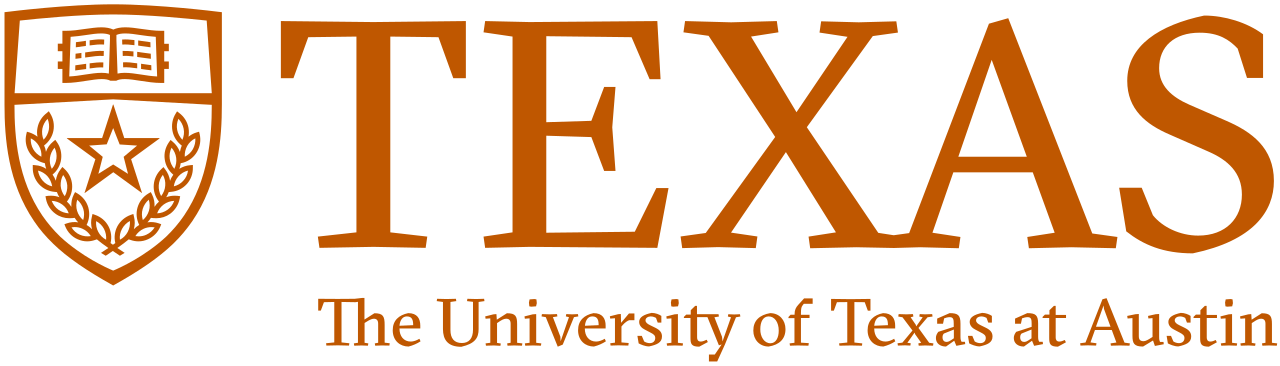
Master’s Degree in Computer Science
Description
This Master’s programme is offered by the University of Texas at Austin as a fully online masters course, which is expected to be completed in 1.5 to 3 years. According to the description, the online master programme will be indistinguishable from its on-campus counterpart and students can enjoy a flexible learning scheme. A Bachelor’s degree is needed as a requirement for enrolling in this Master programme.
Teachers:
- UC San Diego: Leo Porter Assistant Teaching Professor, Computer Science and Engineering
- :
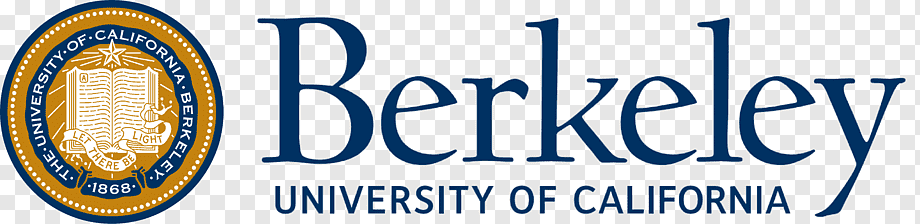
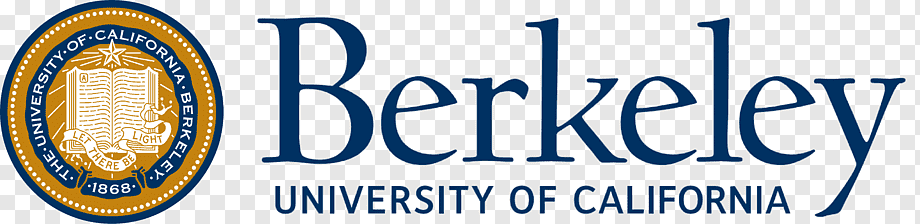
Data Science and Engineering with Spark
Description
3 courses in which students will gain practical experience with Spark and its libraries. The courses will teach students how to approach large scale data science, how to use the Spark APIs and finally some use-cases for Spark will be described.
Teachers:
- Berkeley: Jon Bates, Spark Instructor
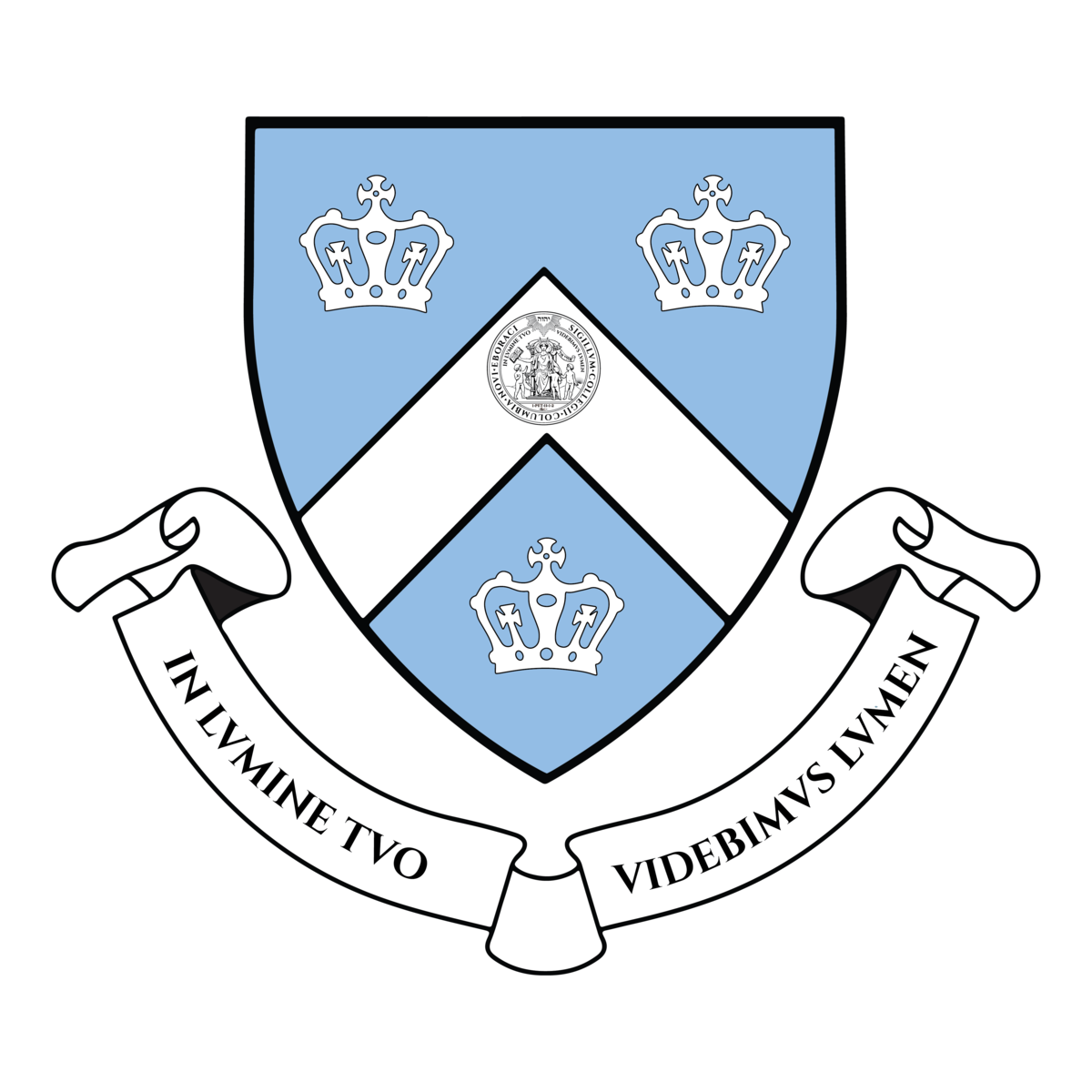
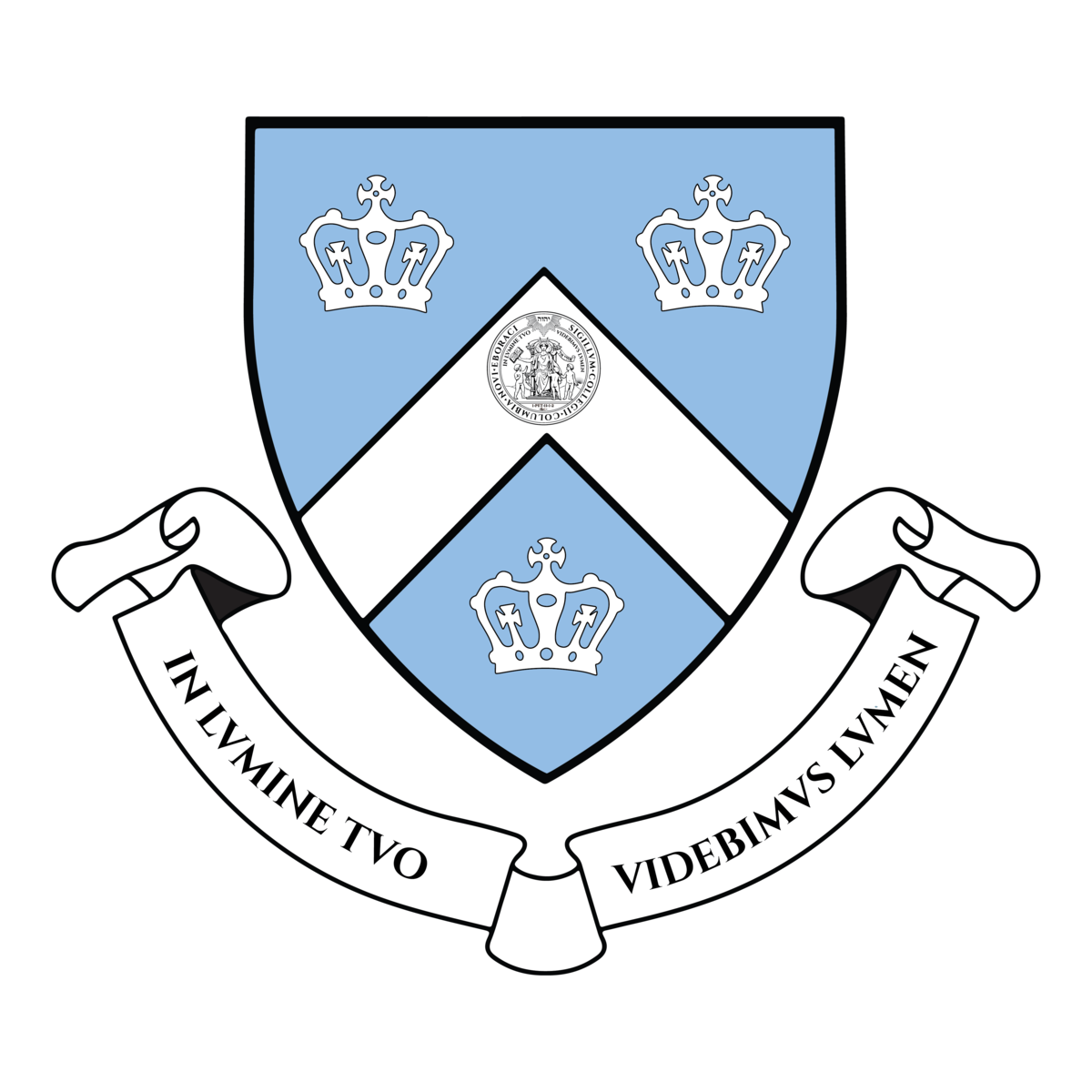
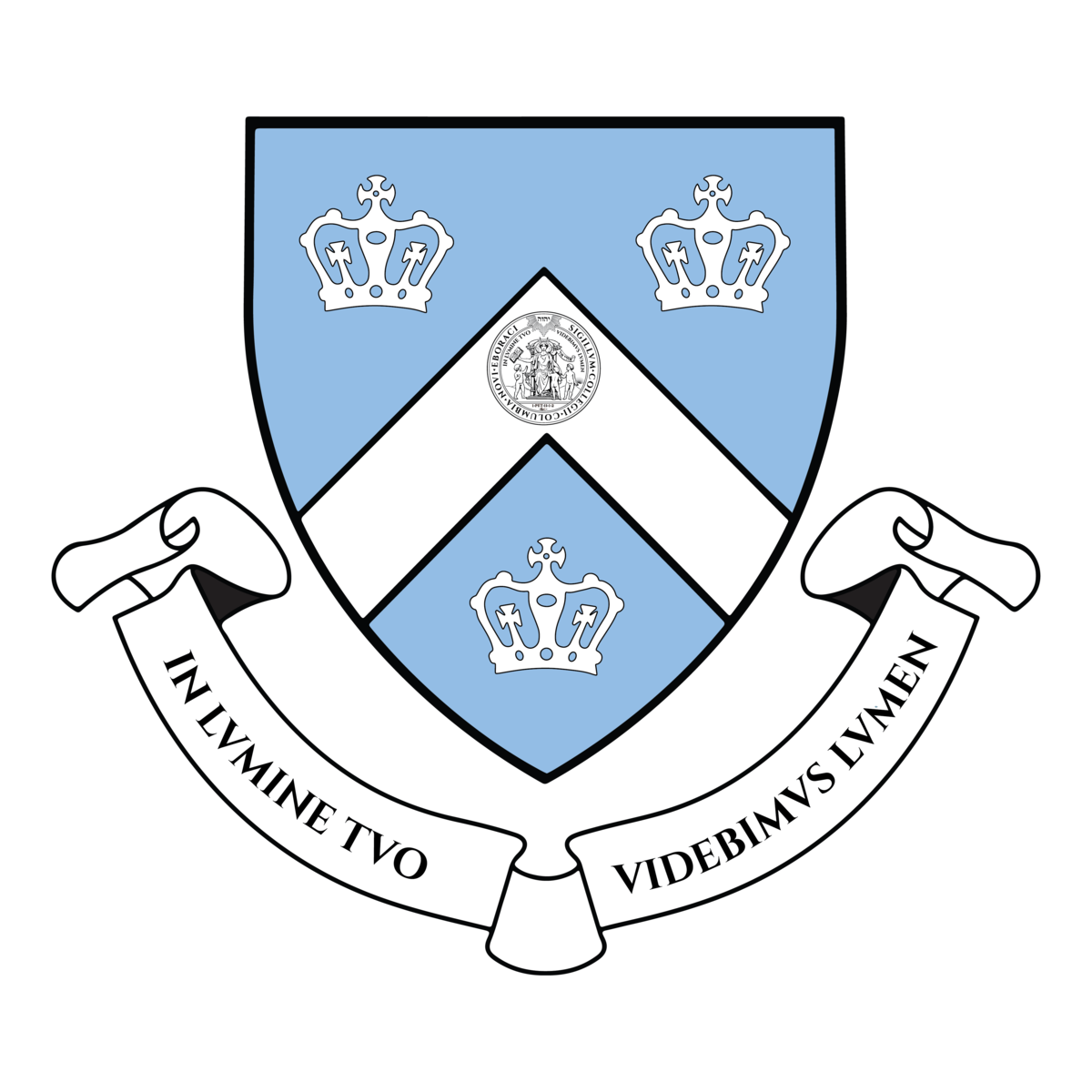
Professional Certificate in Data Science for Executives
Description
This certification is tailored for executives who want to gain insights into how data science and analytics are used in decision making across multiple sectors today. The 3 courses that are part of this specialization cover topics such as NLP, Internet of Things (IoT), statistical thinking etc.
Teachers:
- Colombia University: Cliff Stein, Professor of IEOR and of Computer Science
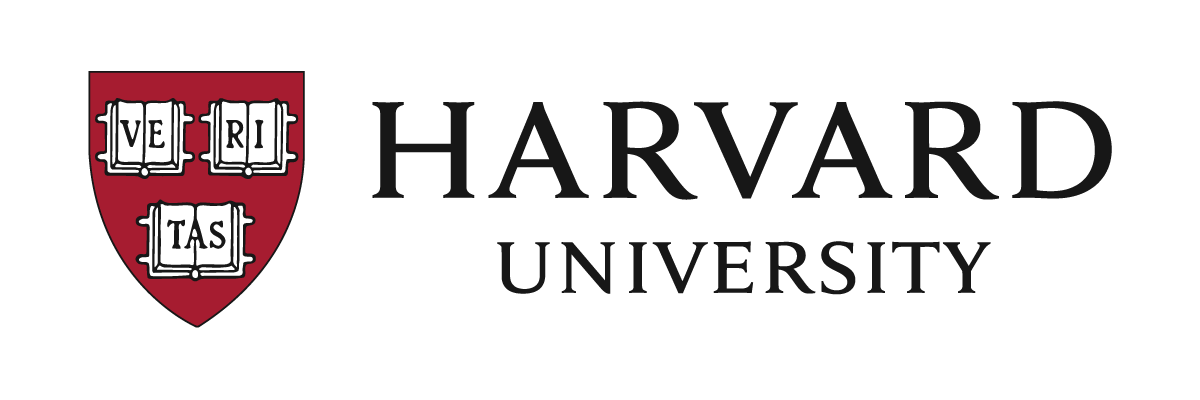
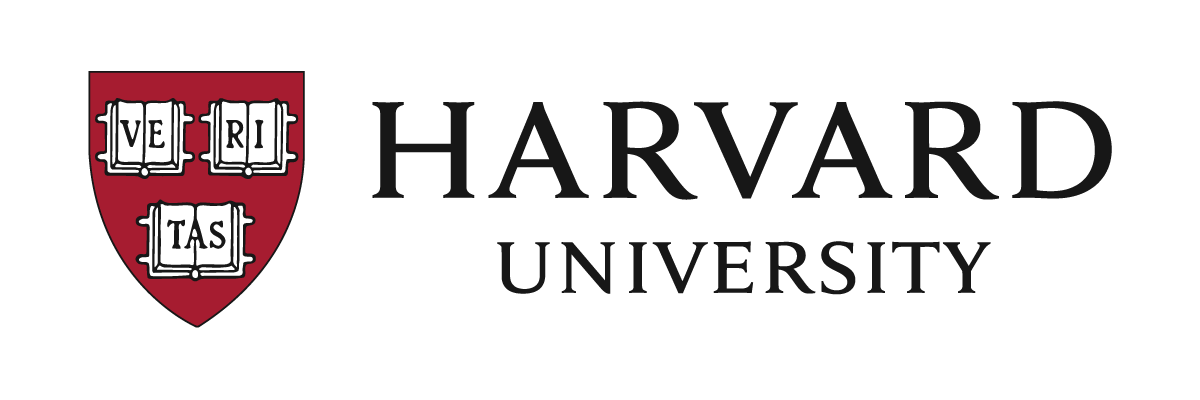
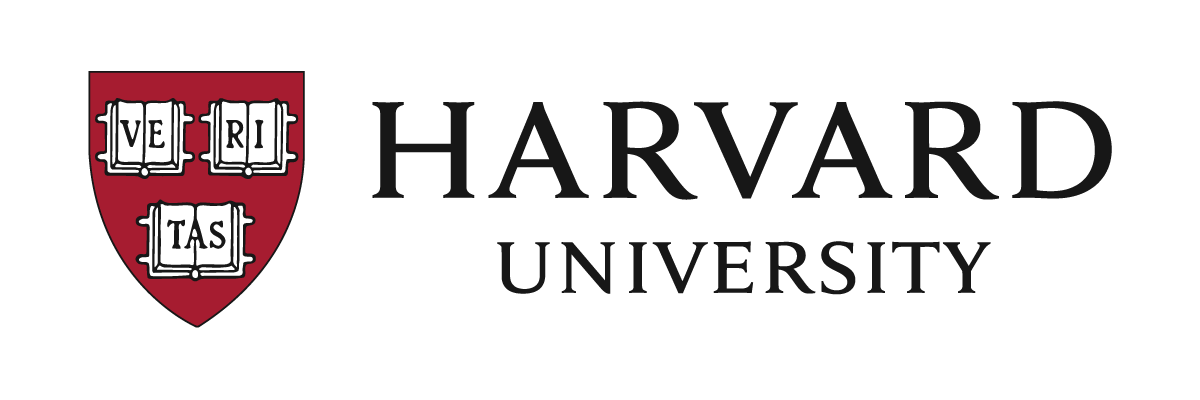
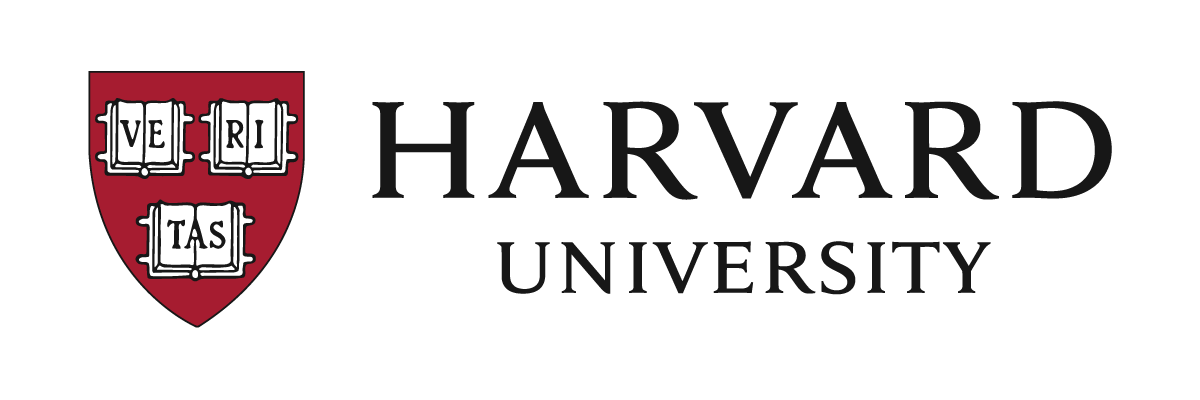
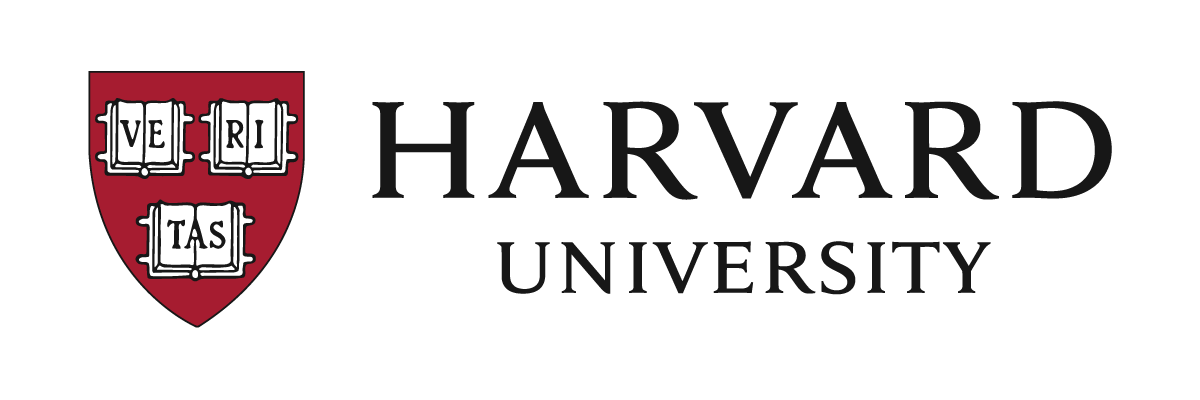
Professional Certificate in Data Science
Description
The 9 skill-building courses cover topics such as statistical analysis, inference, modeling, machine learning etc. Students will learn fundamentals of programming in the R programming language and get practical experience with visualization tools. It is expected that the students who finish the courses possess in-depth knowledge of fundamental data science.
Teachers:
- Harvard: Rafael Irizarry, Professor of Biostatistics
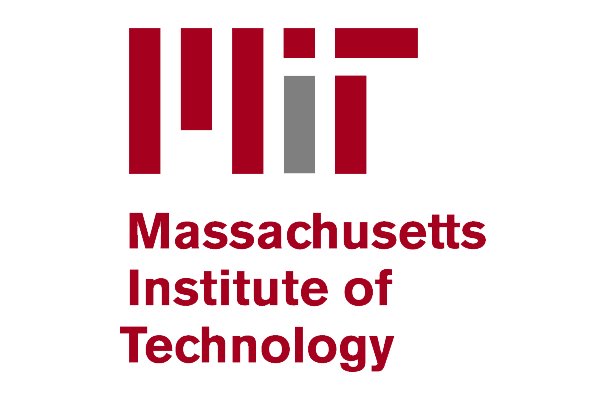
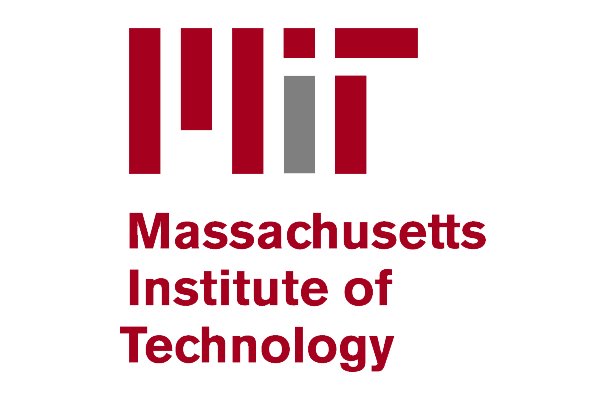
MicroMasters® Program in Statistics and Data Science
Description
5 graduate-level courses thought by MITx instructors. The students will be prepared for job titles such as: Data Scientist, Data Analyst, Business Intelligence Analyst, Systems Analyst, Data Engineer. The courses cover data science topics and teach students how to perform data analysis and do data-driven decision making.
Teachers:
- MIT: Regina Barzilay, Delta Electronics Professor
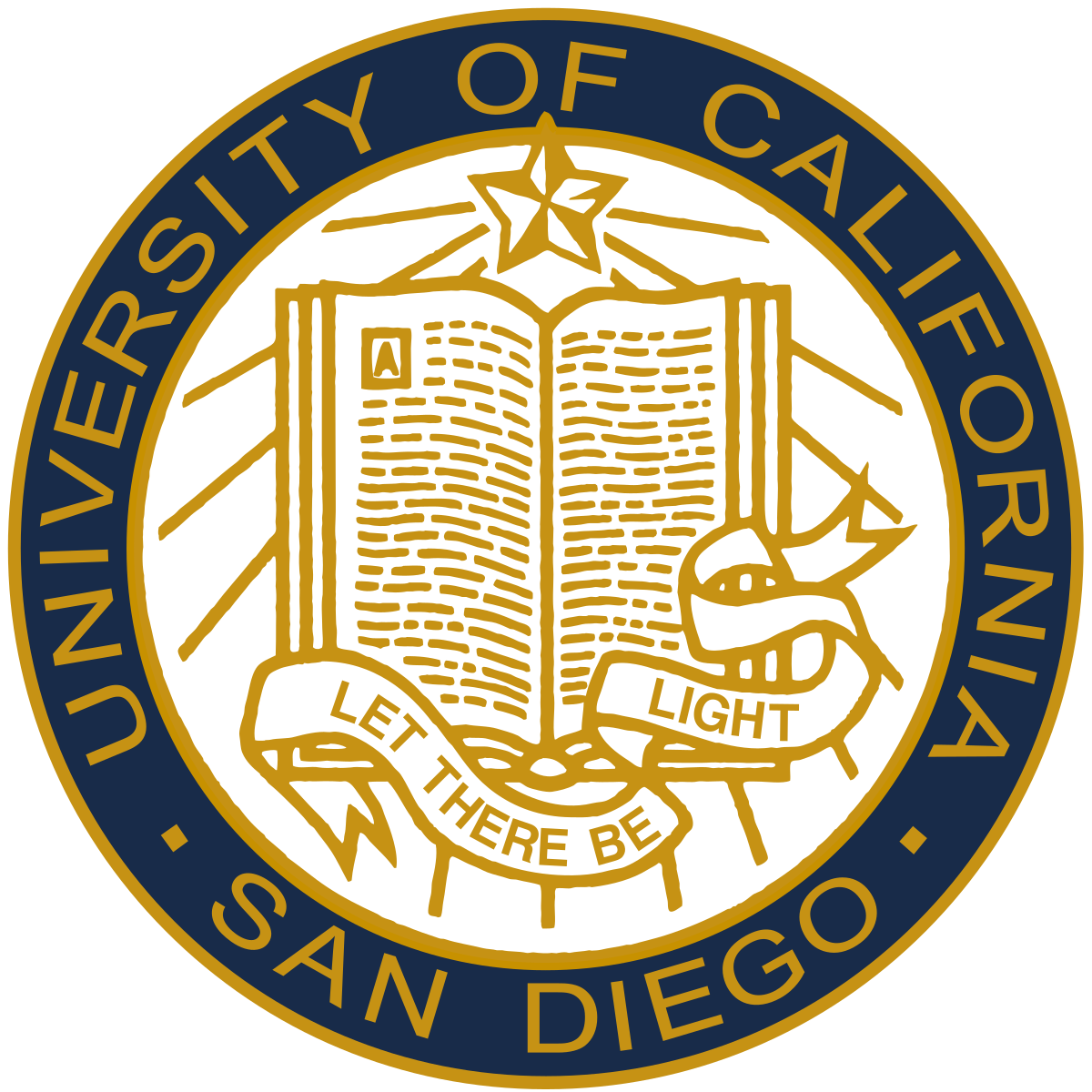
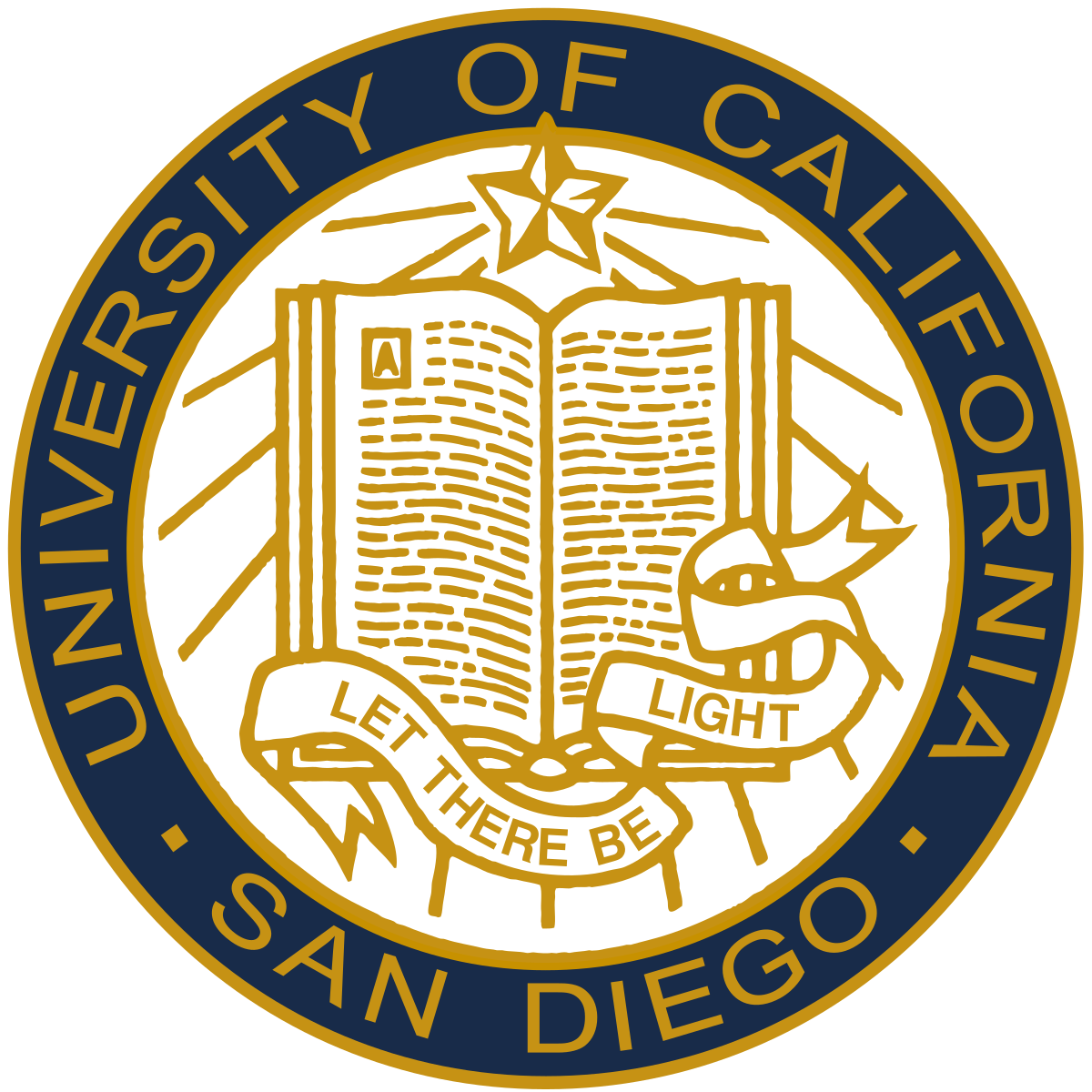
MicroMasters Program in Data Science
Description
This course is a 70-week long micro masters program offered by the University of California San Diego. It covers 4 main courses: Machine Learning Fundamentals, Python for Data Science, Probability and Statistics in Data Science, Big Data Analysis using Spark. Students will learn how to load and clean real-world data, make reliable statistical inferences from noisy data, use machine learning to learn models, visualize complex data, use Apache Spark.
Teachers:
- UC San Diego: Leo Porter, Assistant Teaching Professor
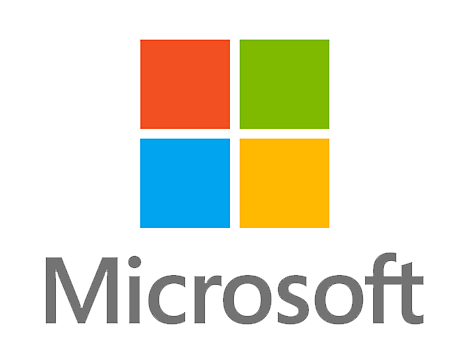
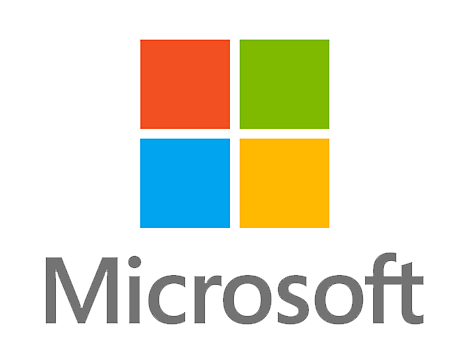
Microsoft Professional Program in Data Science
Description
This program offered by Microsoft in collaboration with edX covers: data science fundamentals, key data science tools, and widely-used programming languages from industry and academic experts
Teachers:
- Microsoft: Graeme Malcolm, Senior Content Developer
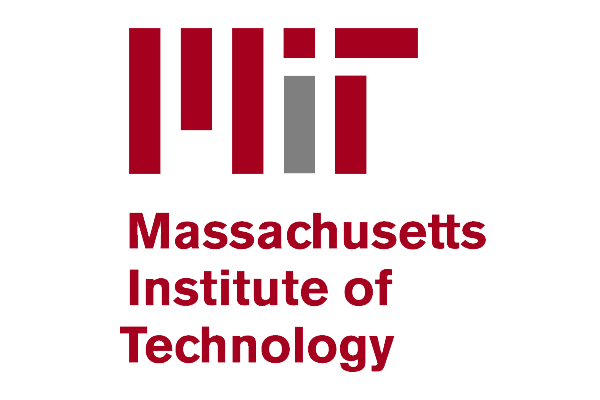
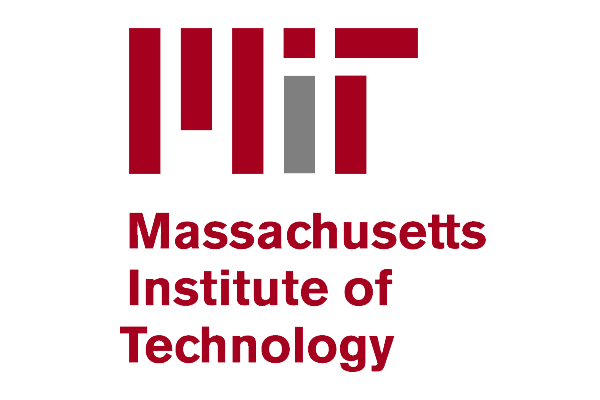
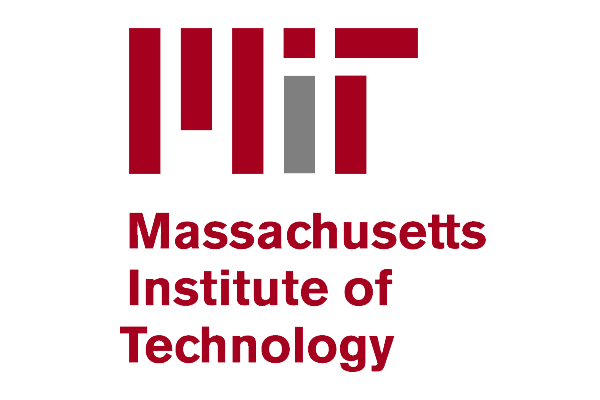
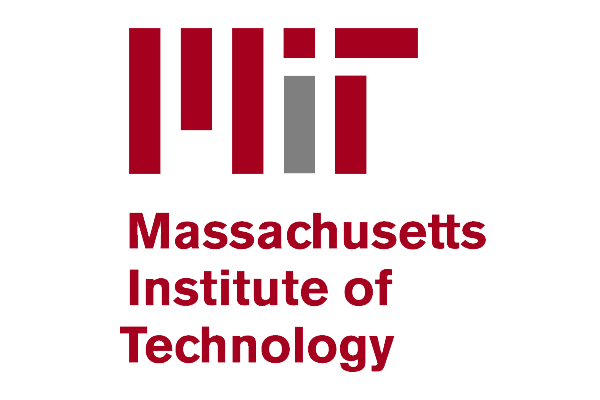
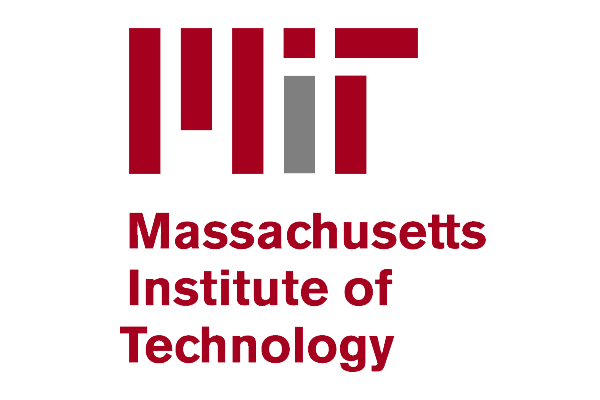
Machine Learning with Python: from Linear Models to Deep Learning
Description
As part of MIT’s MicroMasters in Statistics and Data Science, this course covers an in-depth introduction to the field of machine learning, from linear models to deep learning and reinforcement learning, through hands-on Python projects. Students will learn different models including: linear models, kernel machines, neural networks, and graphical models and their applications.
Teachers:
- MIT: Regina Barzilay, Professor
- MIT: Tommi Jaakkola, Professor
- MIT: Karene Chu, Research Scientist
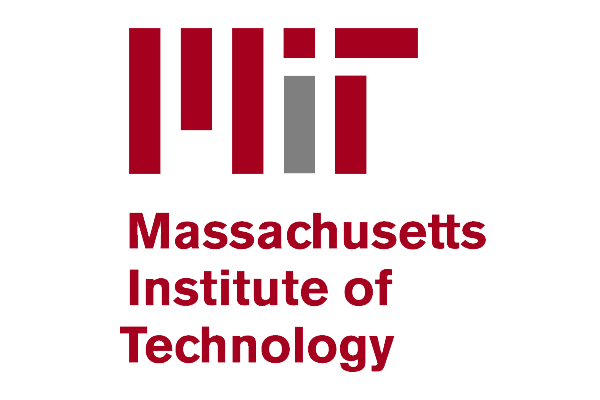
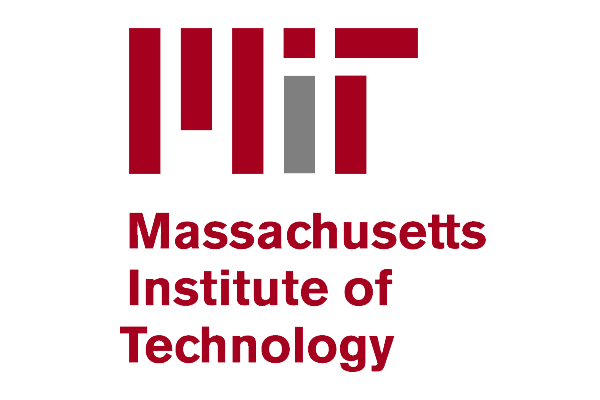
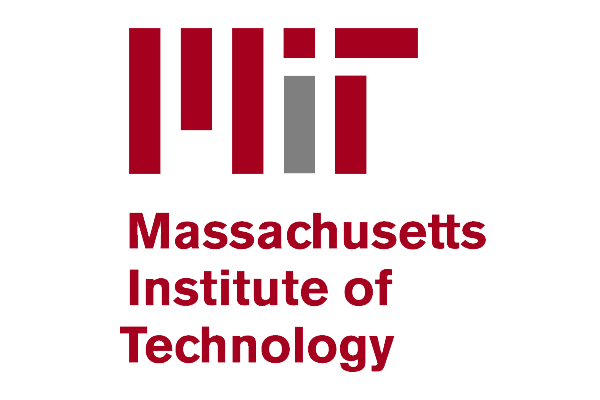
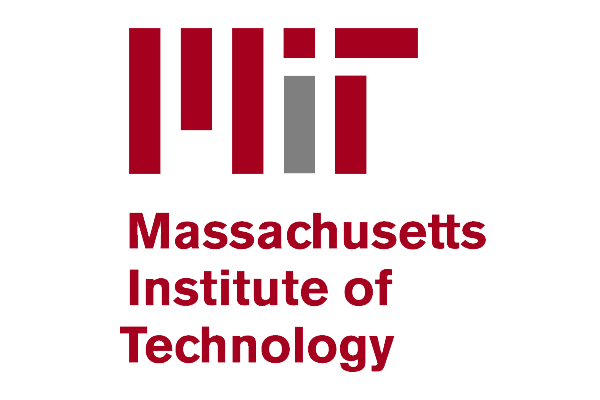
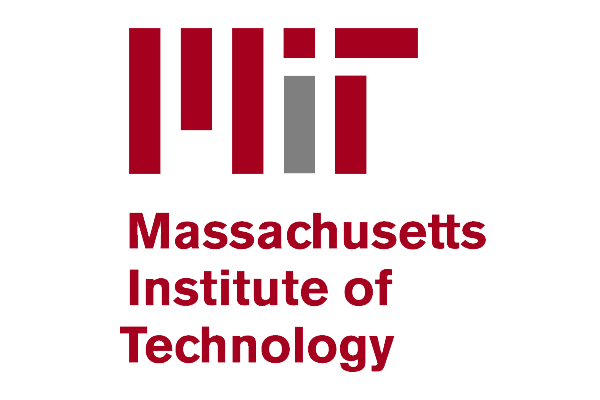
Fundamentals of Statistics
Description
The course covers core topics from statistics that are the underlying machinery of machine learning and Artificial Intelligence. It offers students to gain deep understanding of the principles that underpin statistical inference: estimation, hypothesis testing and prediction.
Teachers:
- MIT: Philippe Rigollet Associate Professor
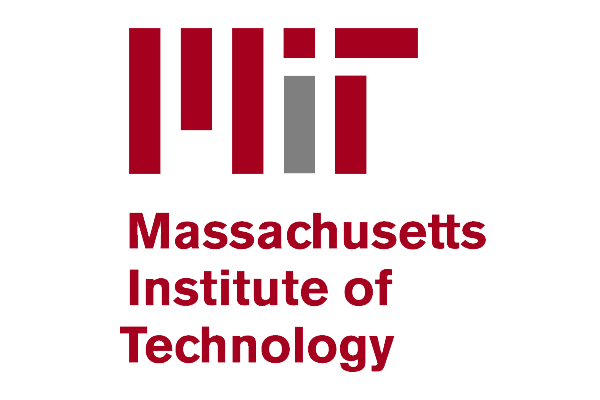
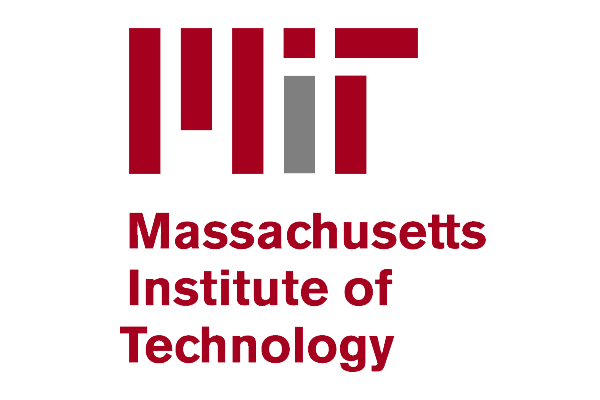
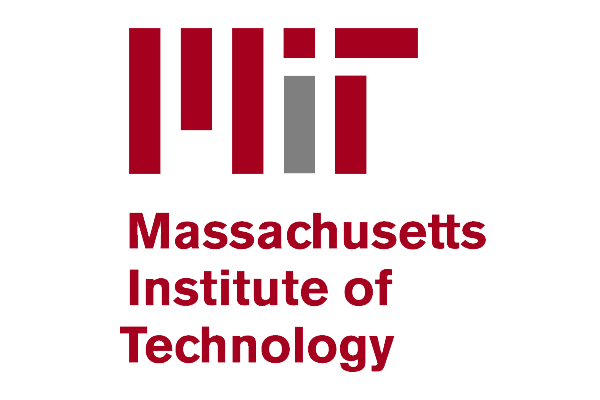
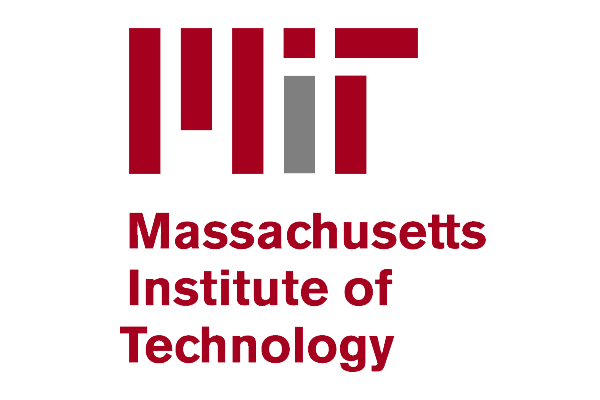
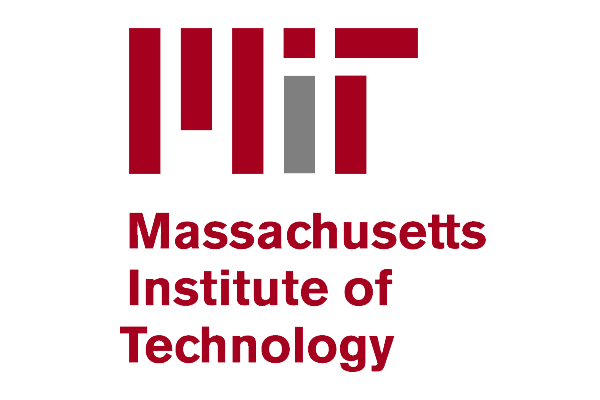
Data Analysis in Social Science—Assessing Your Knowledge
Description
Another course from the MIT MicroMasters in Statistics and Data Science. Students will learn how to process and analyze data and give answers to many questions in social sciences. Topics covered: data summarization, intuition behind statistical methods, methods for presenting results and analyzing data in R.
Teachers:
- MIT: Esther Duflo, Professor
- MIT: Sara Fisher Elisson
- MIT: Karene Chu
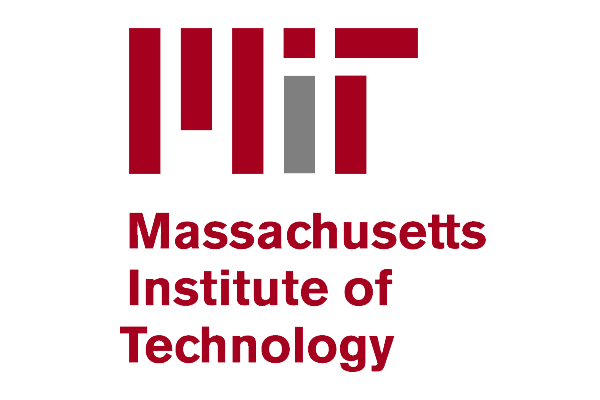
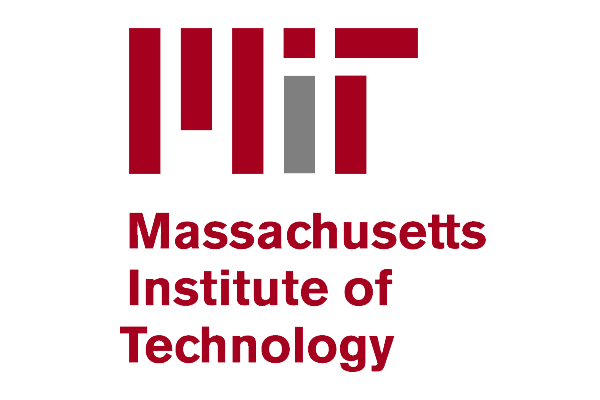
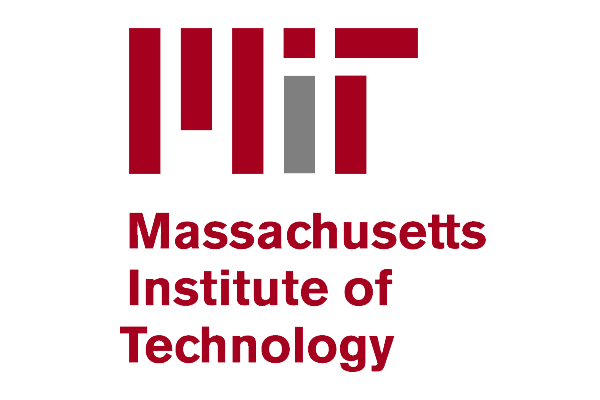
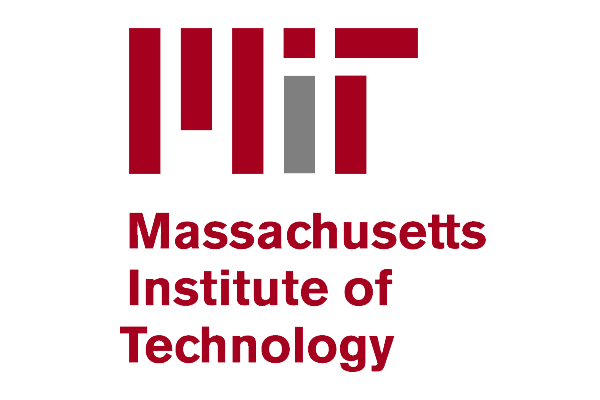
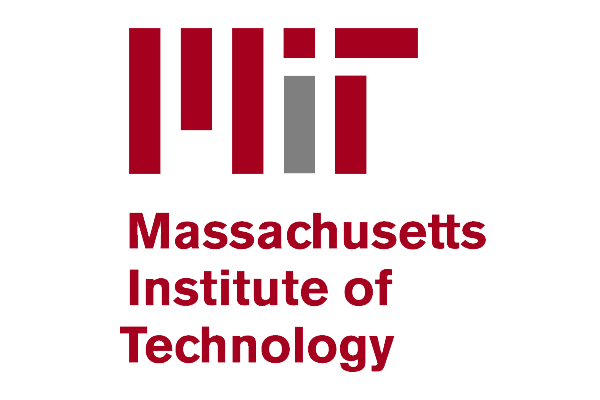
Probability - The Science of Uncertainty and Data
Description
The course covers all of the basic probability concepts. Probabilistic models, random variables, distributions, inference methods etc. The course is the course 1 of the MIT’s MicroMasters in Statistics and Data Science. The lecturers are professors and scientists from MIT.
Teachers:
- MIT: John Tsitsiklis, Professor
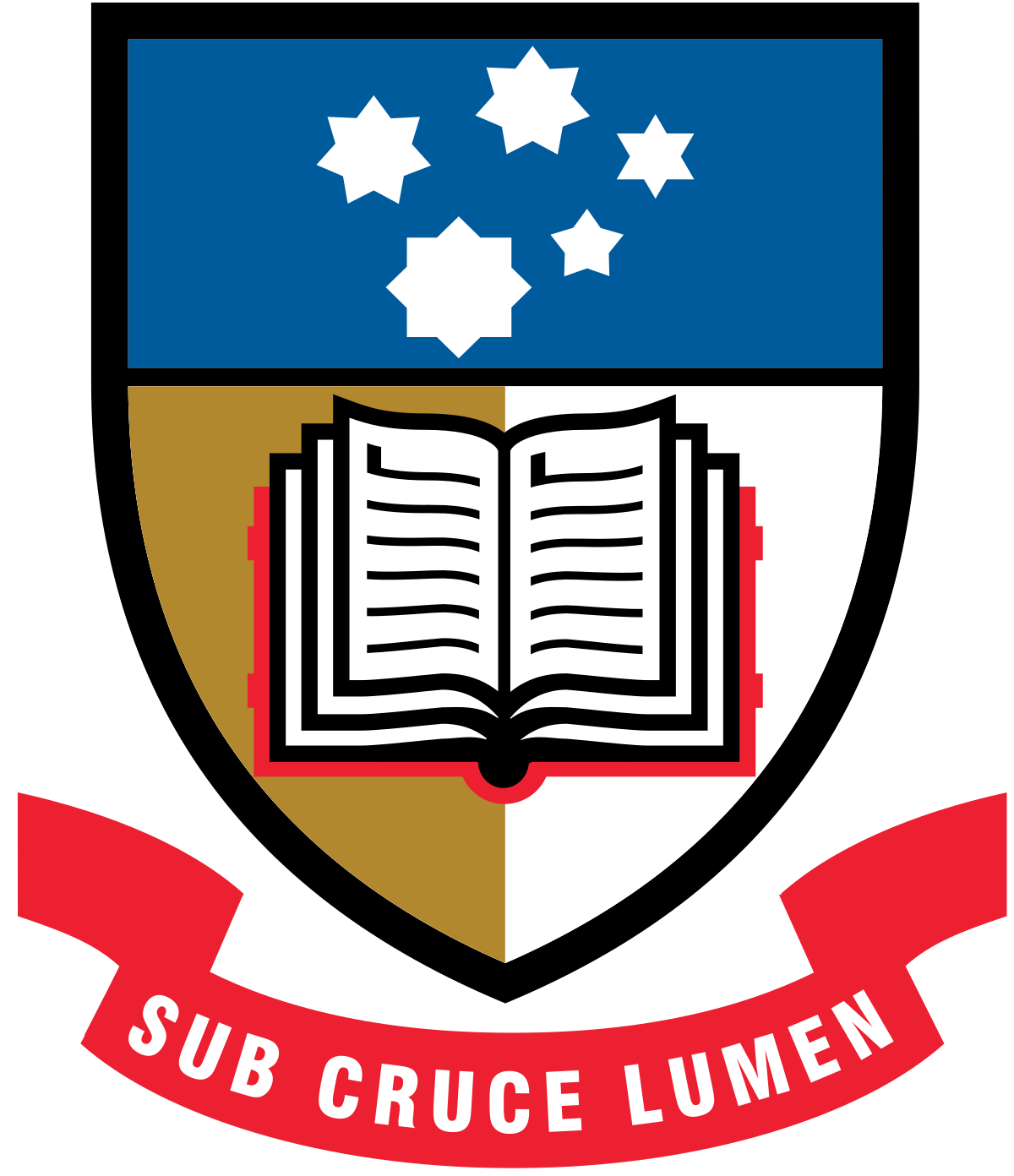
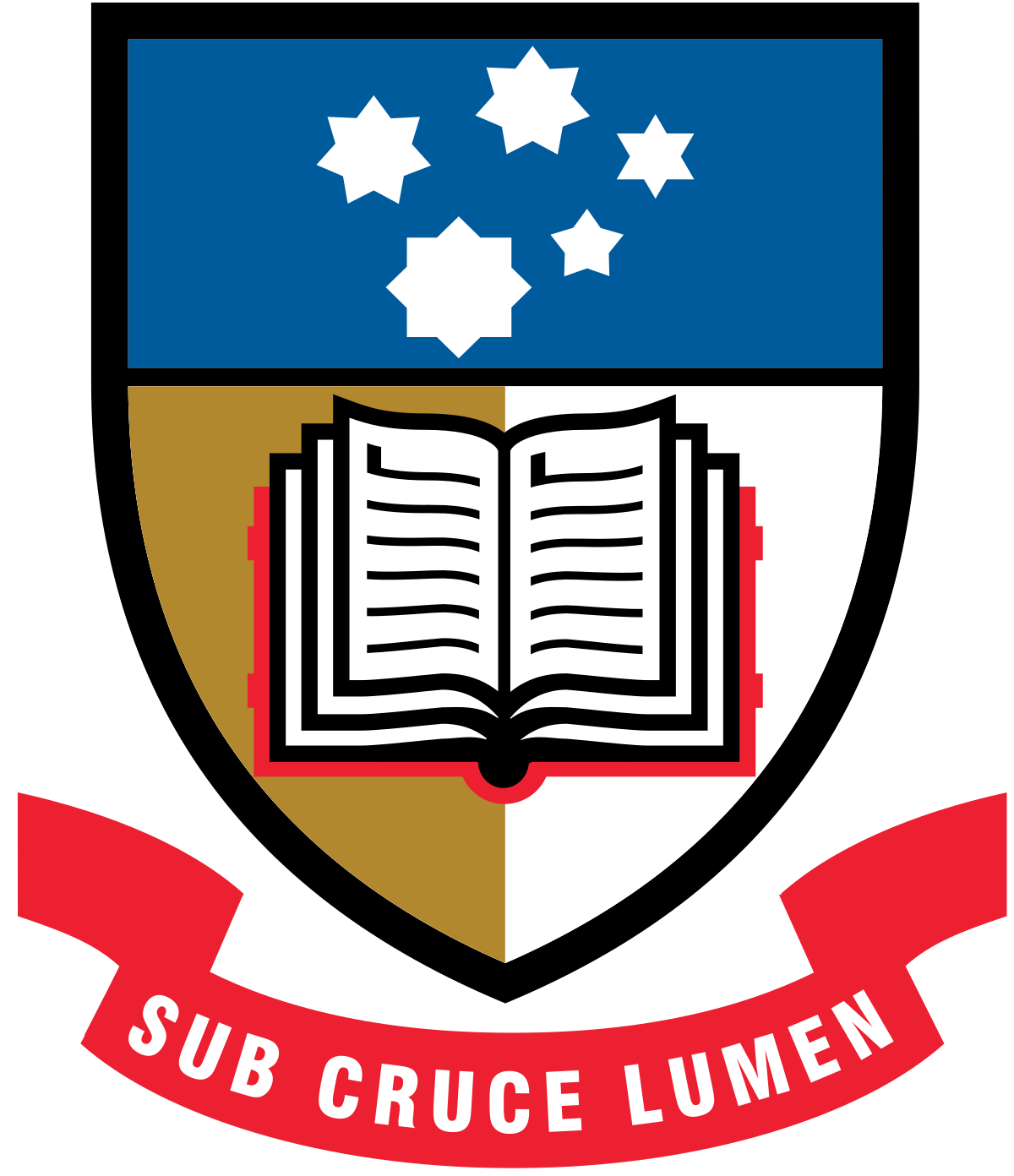
Programming for Data Science
Description
Data science fundamentals, key data science tools, and widely-used programming languages from industry and academic experts. Free online course offered by Microsoft and edX. The certificate is optional and costs 150$ after completion of the course.
Teachers:
- Adelaida: Katrina Falkner, Head - School of Computer Science
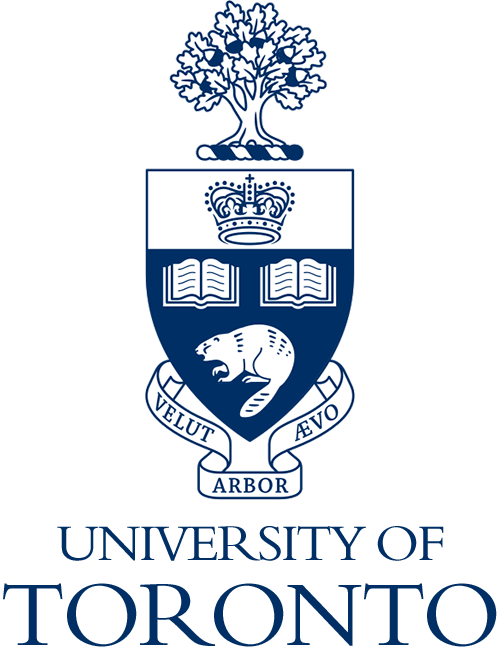
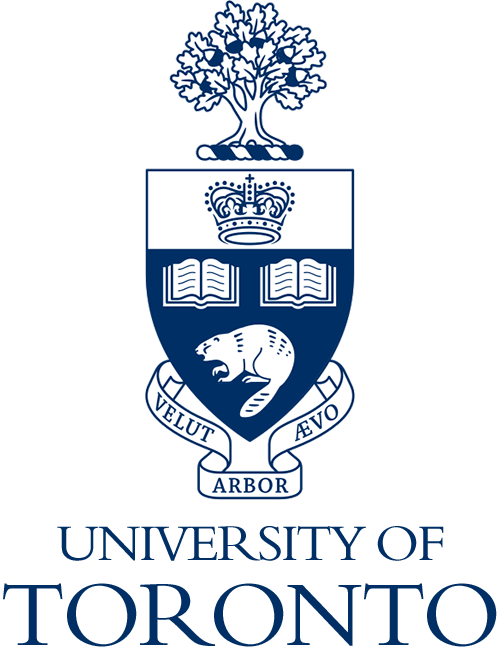
Learn to Program: The Fundamentals
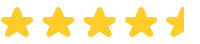
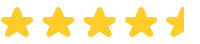
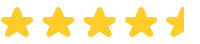
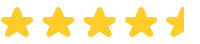
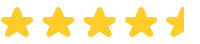
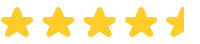
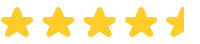
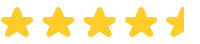
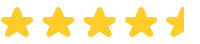
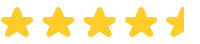
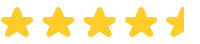
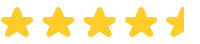
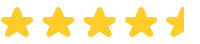
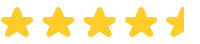
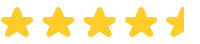
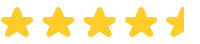
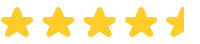
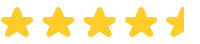
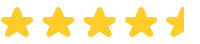
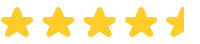
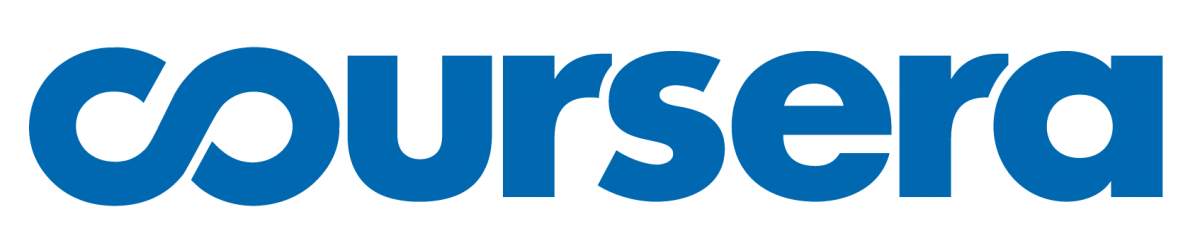
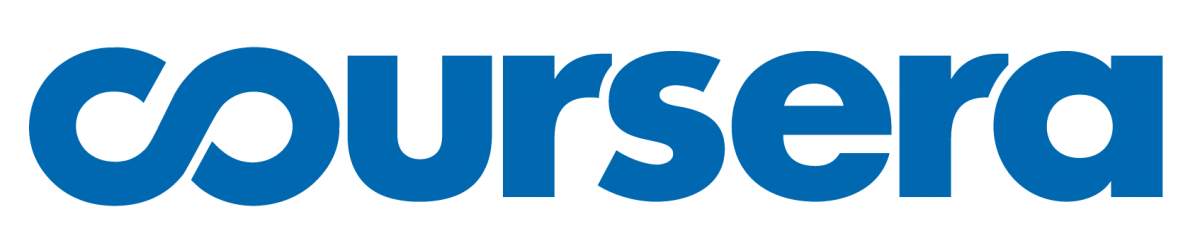
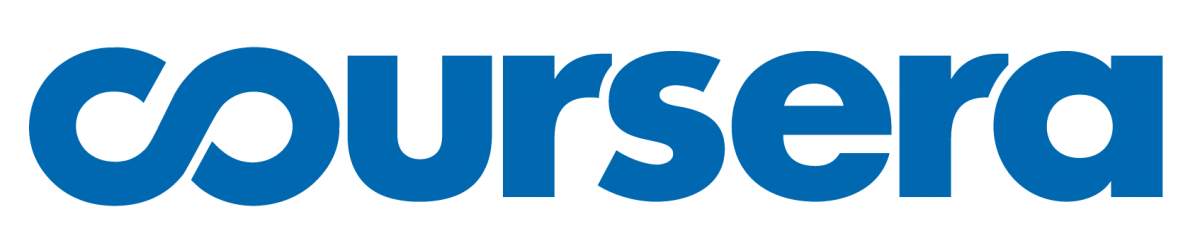
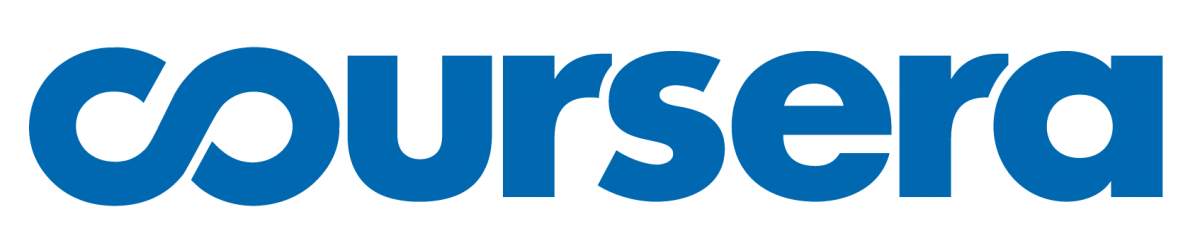
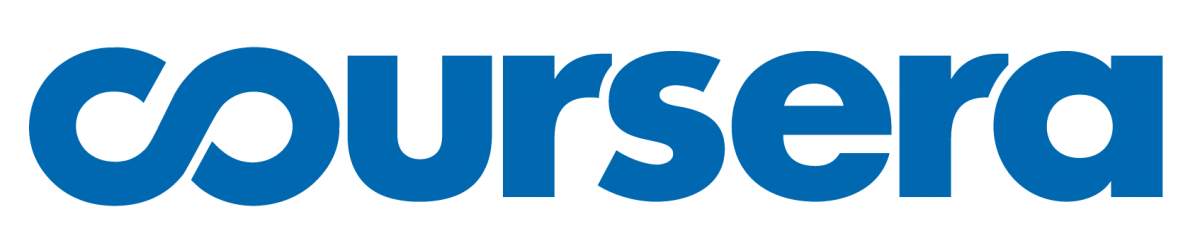
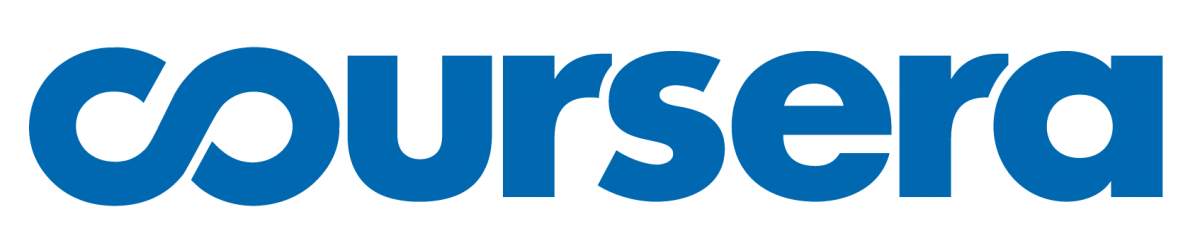
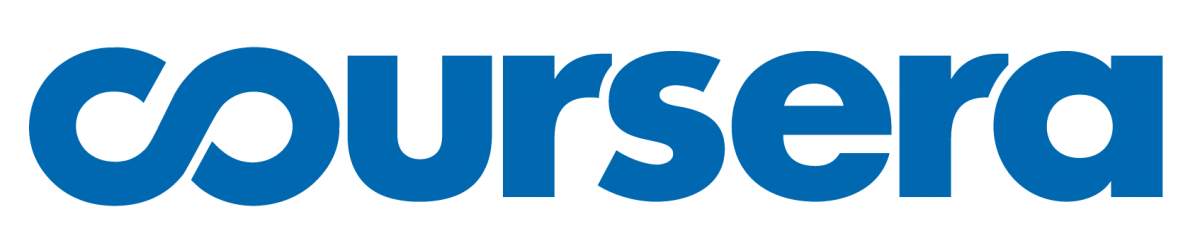
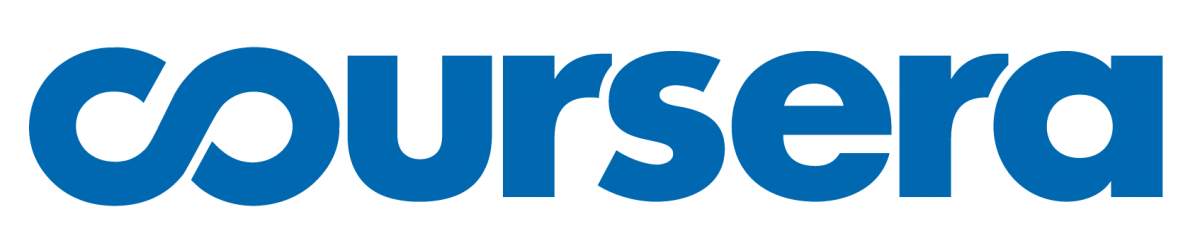
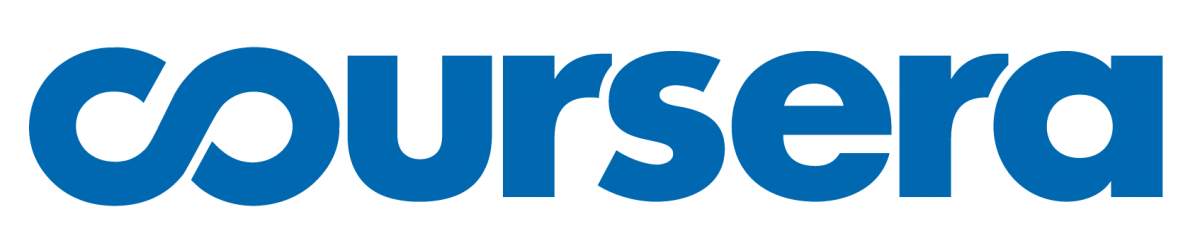
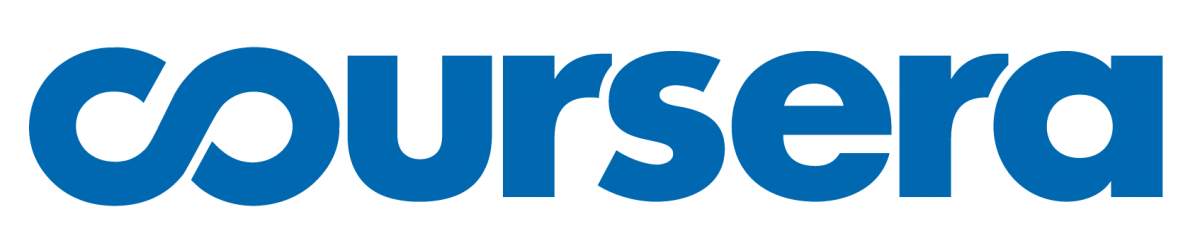
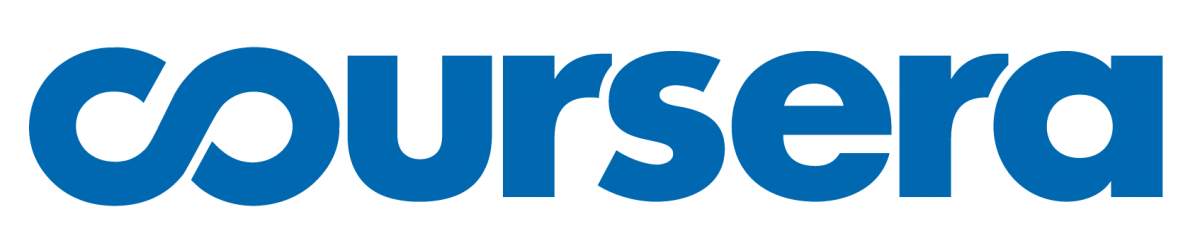
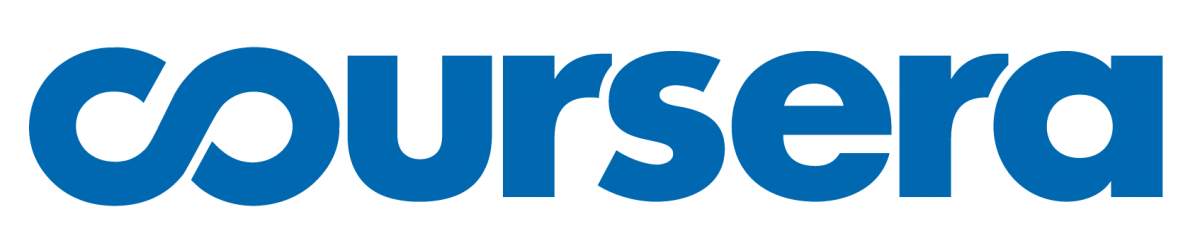
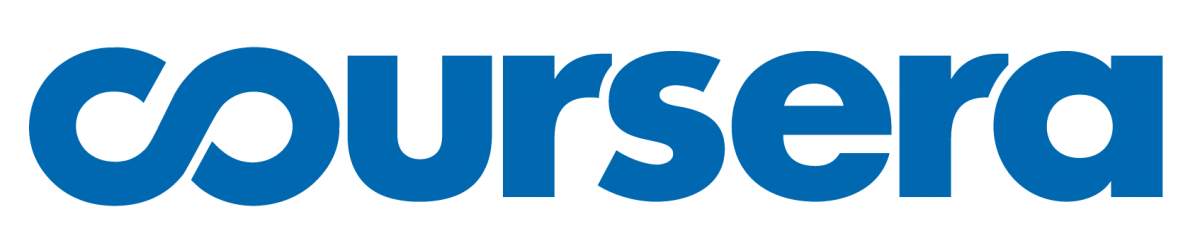
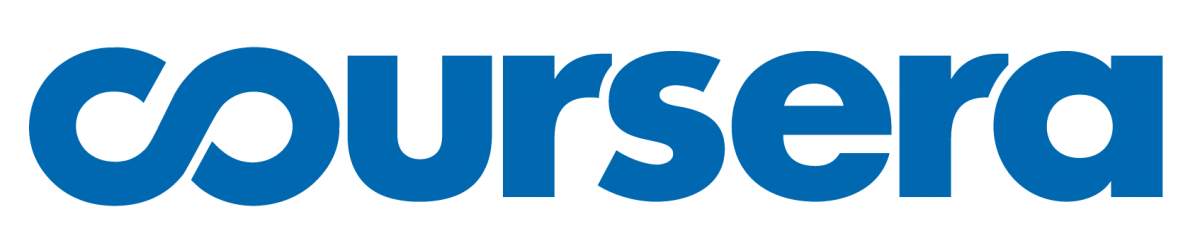
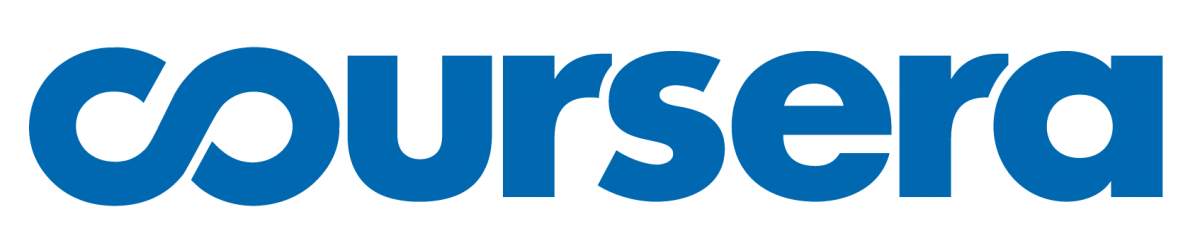
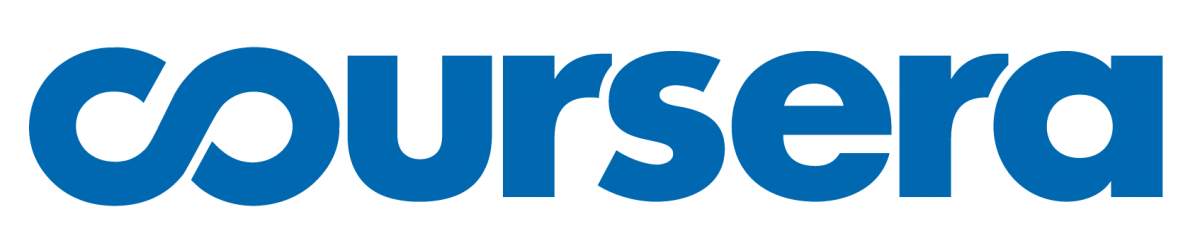
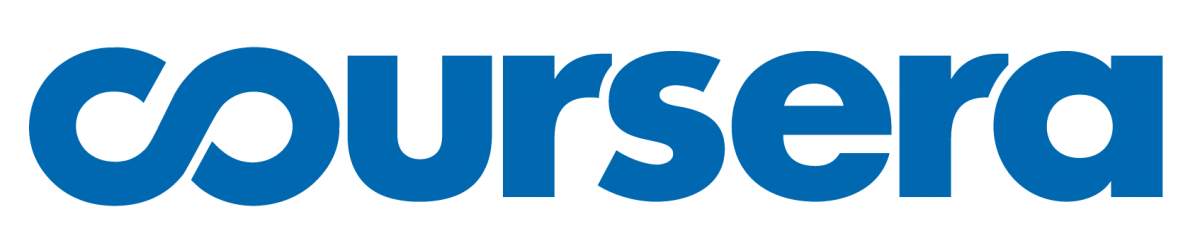
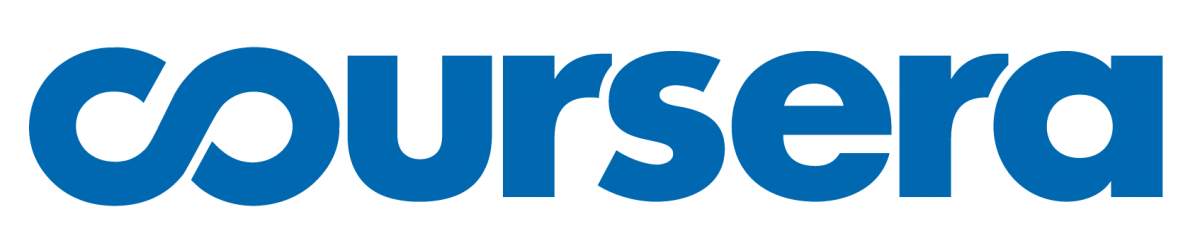
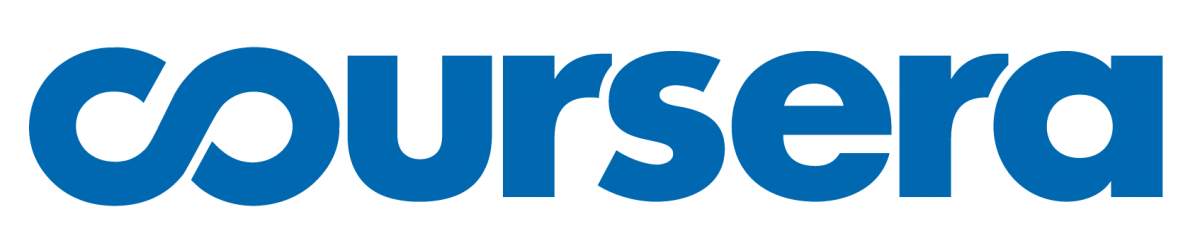
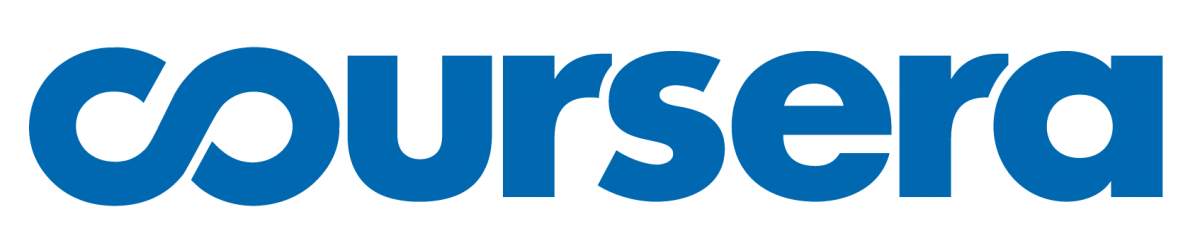
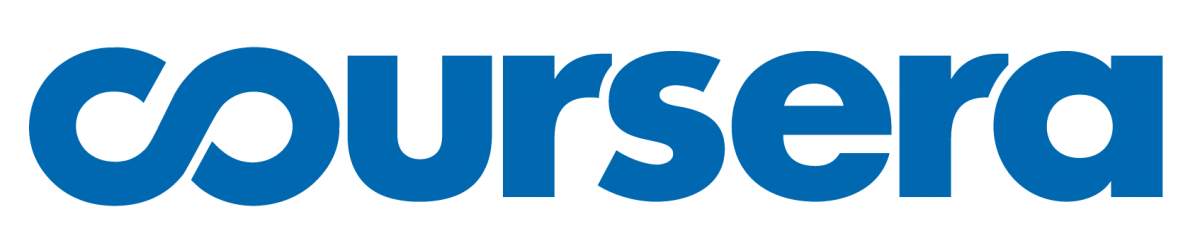
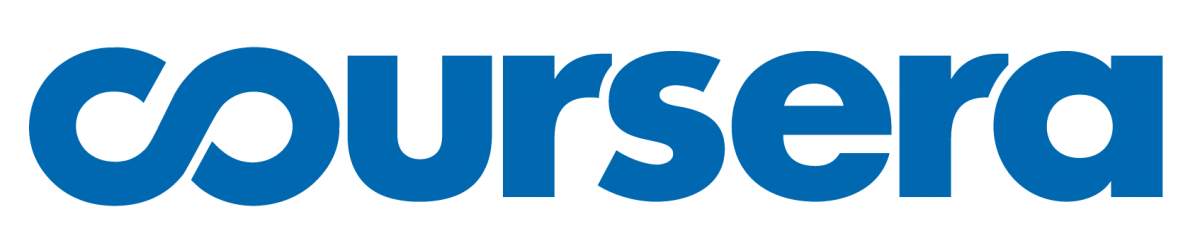
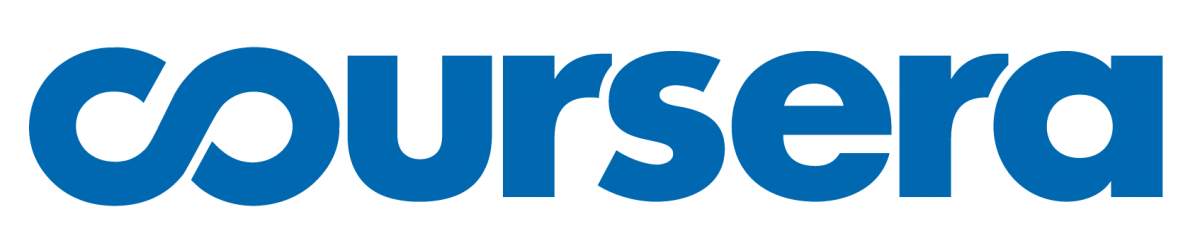
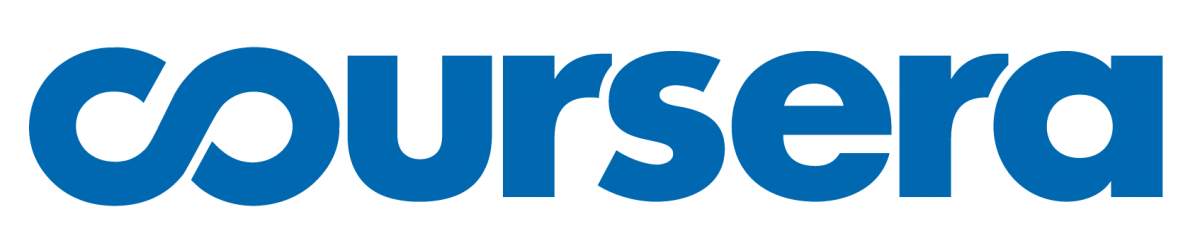
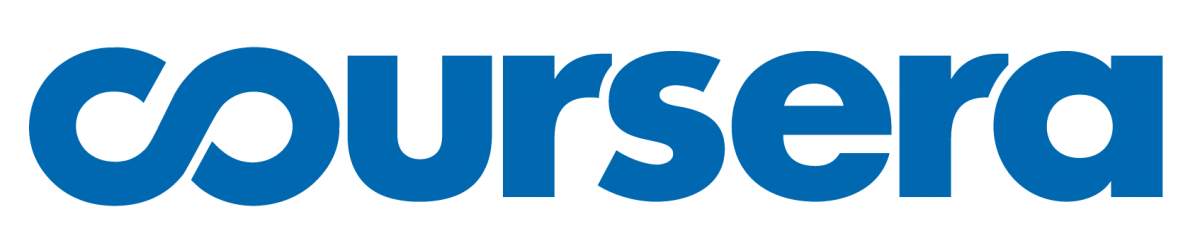
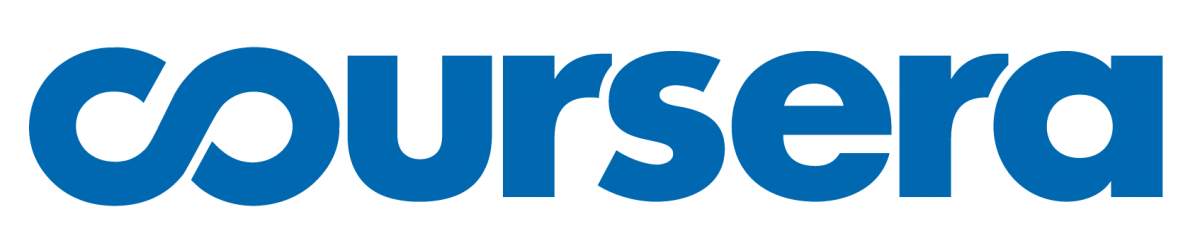
Description
A beginner course for learning programming. The fundamental building blocks of programming and teaches you how to write fun and useful programs using the Python language. A course is a great option for complete beginners in programming with Python. It covers many programming concepts as well as the Python language syntax.
Teachers:
- University of Toronto: Jennifer Campbell Associate Professor
- :
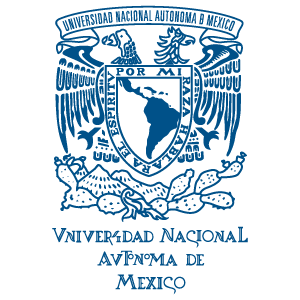
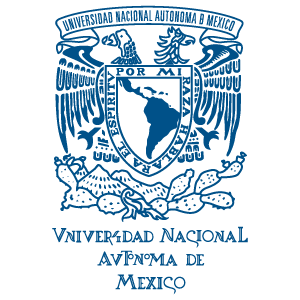
Database Systems
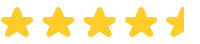
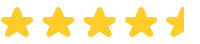
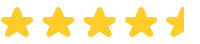
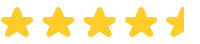
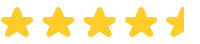
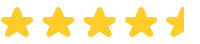
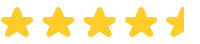
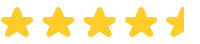
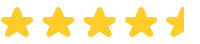
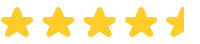
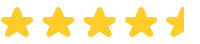
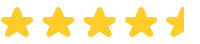
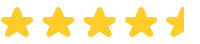
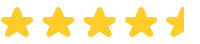
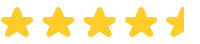
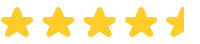
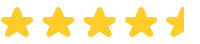
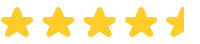
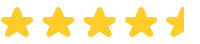
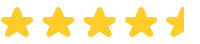
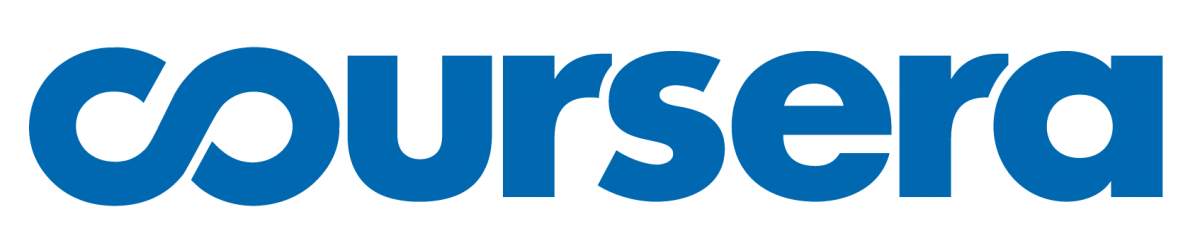
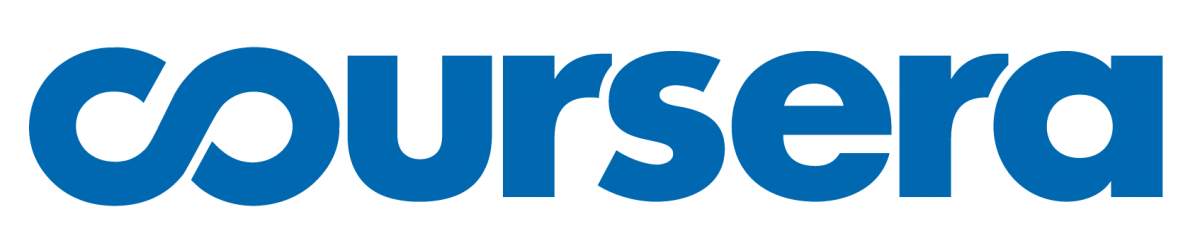
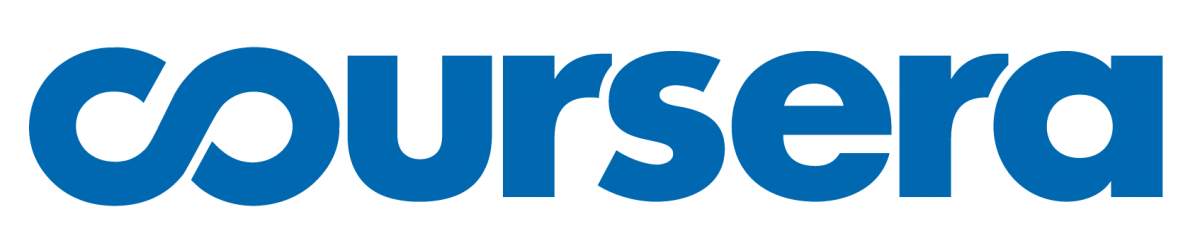
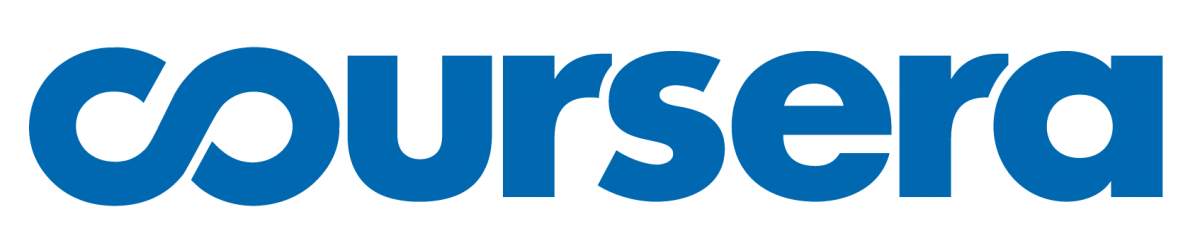
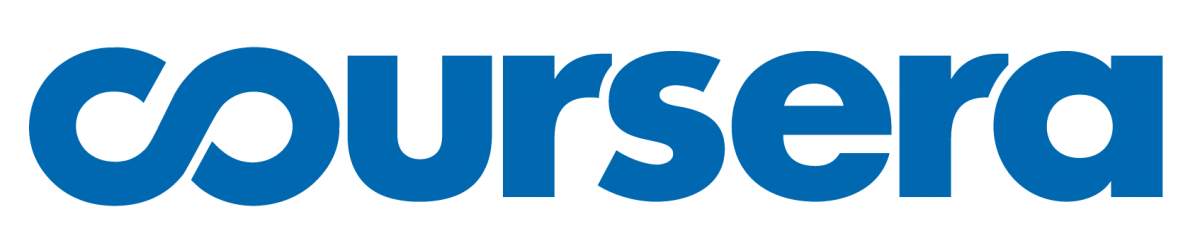
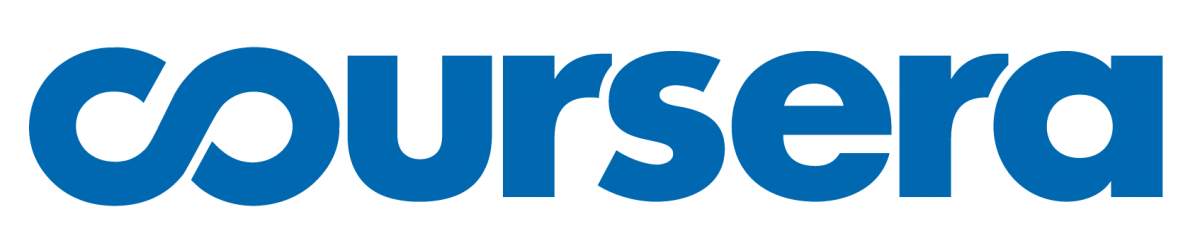
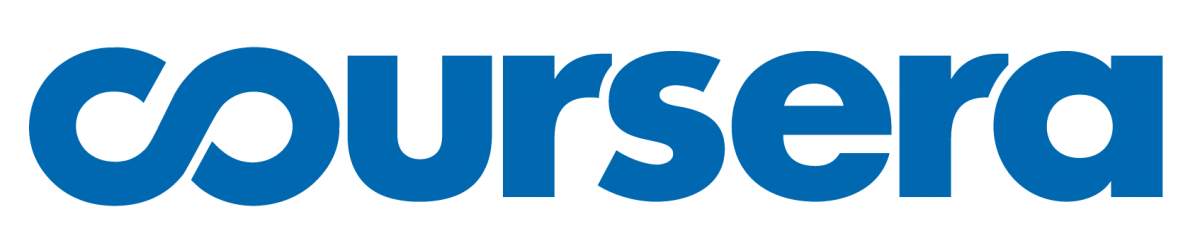
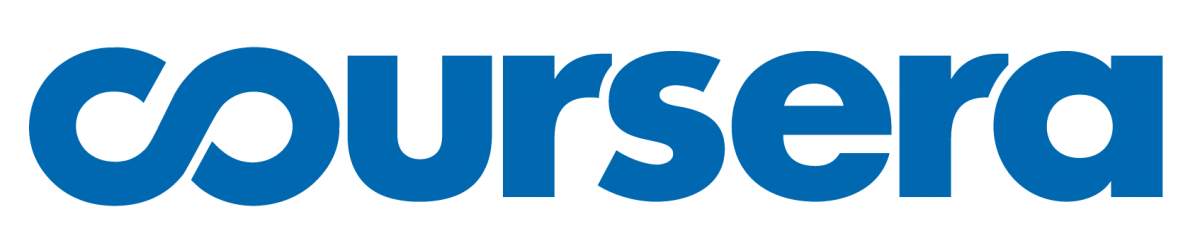
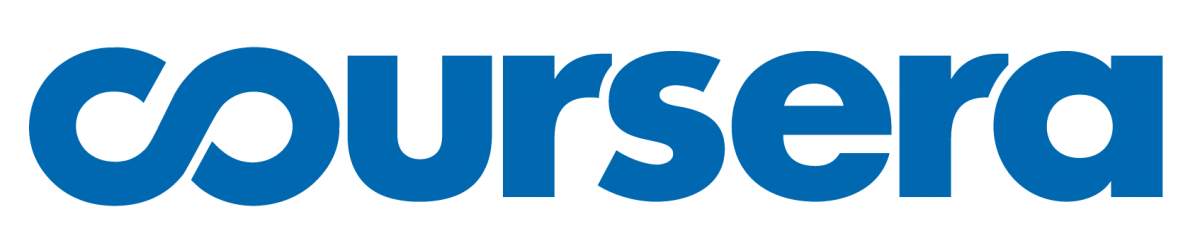
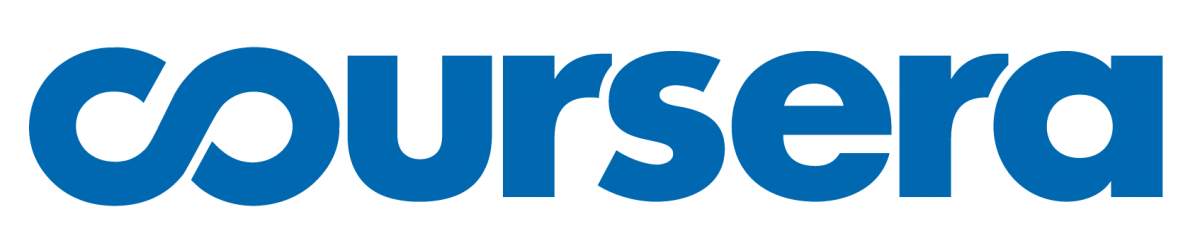
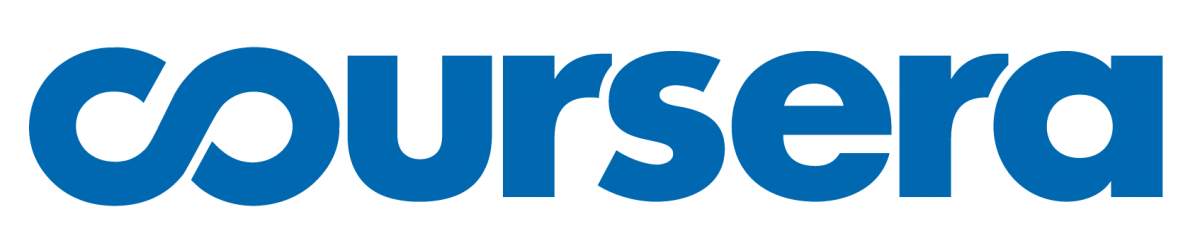
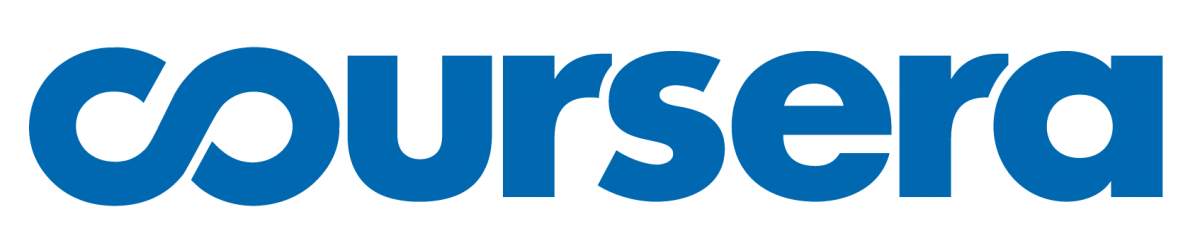
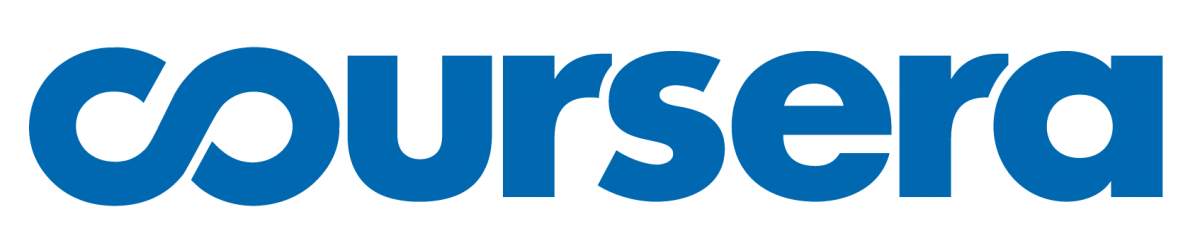
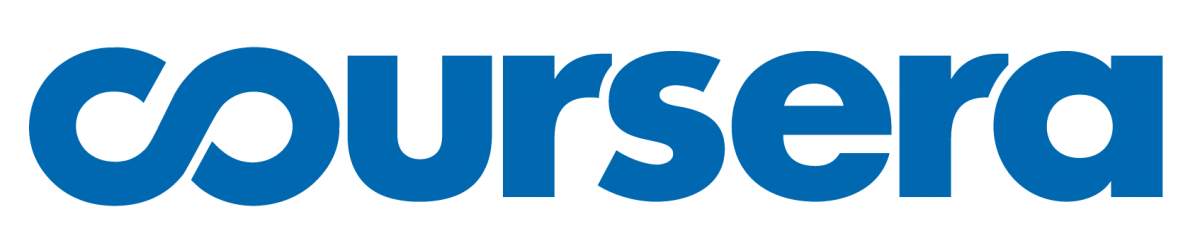
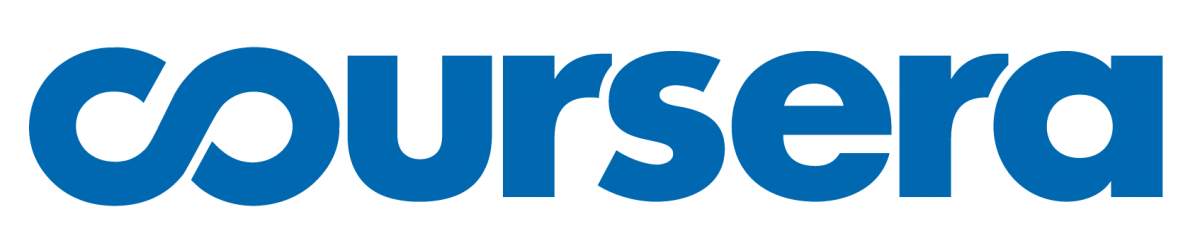
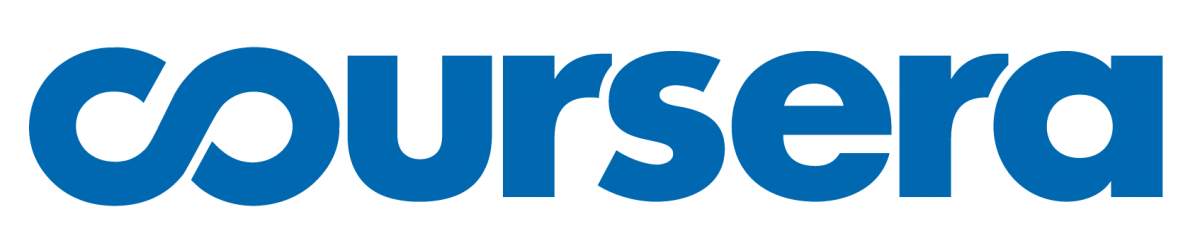
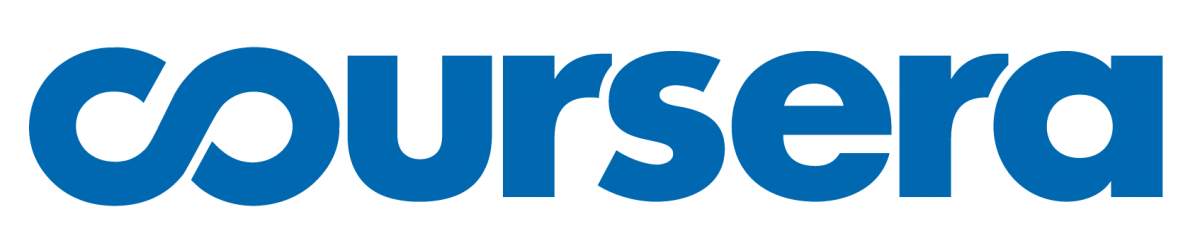
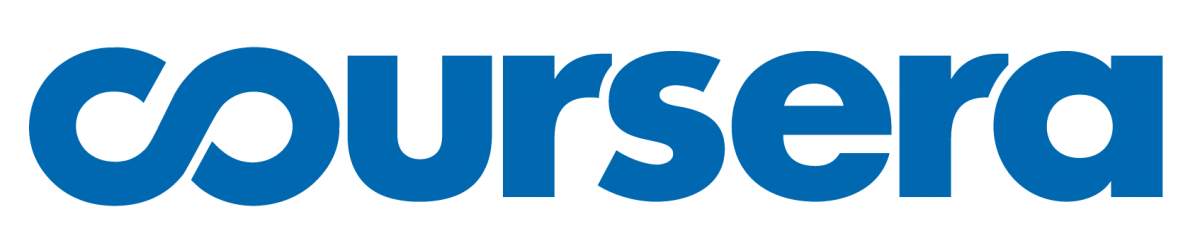
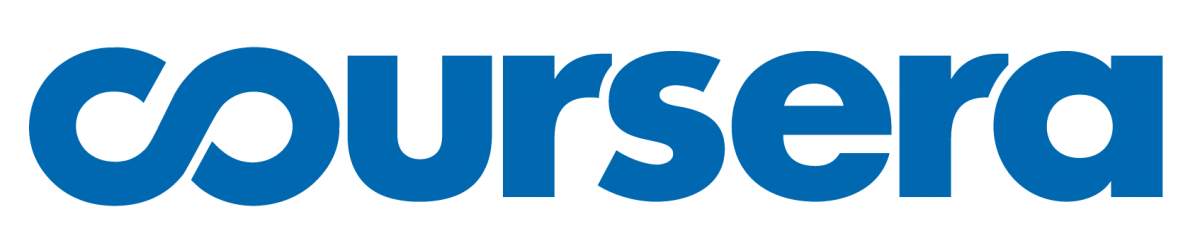
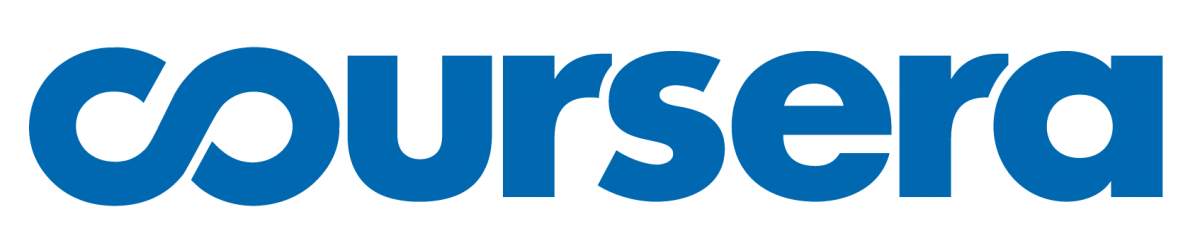
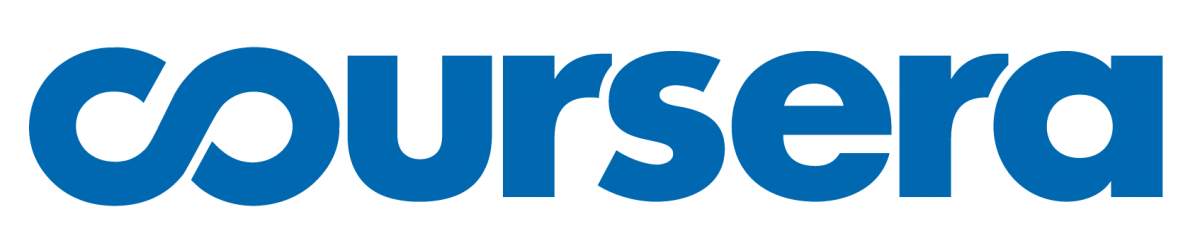
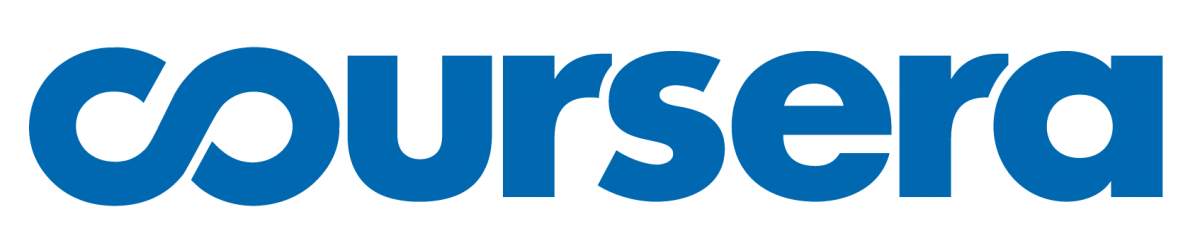
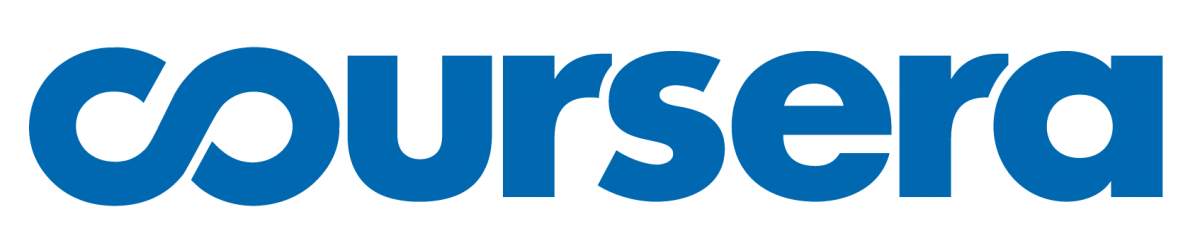
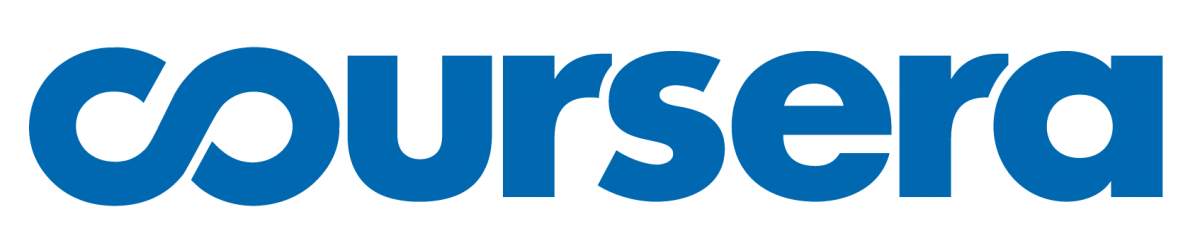
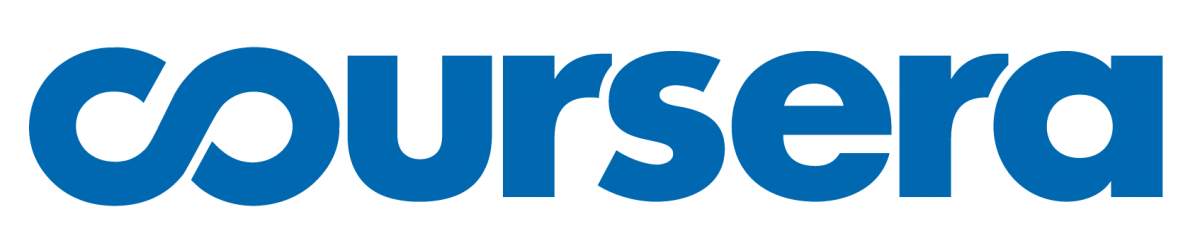
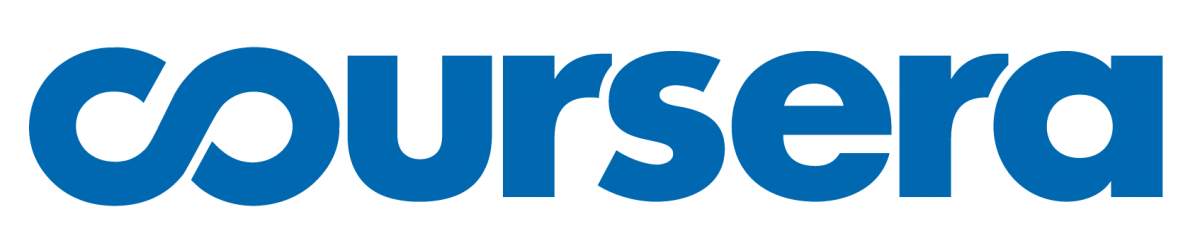
Description
Students will learn about transactional relational databases, business intelligence and Data warehousing, NoSQL technologies, and reliable, scalable, and maintainable data-intensive applications. It is intended to help students become specialized information system consultants or data scientists. The specialization consists of 4 courses: Relational database systems, Business intelligence, and data warehousing, NoSQL systems, Designing data-intensive applications.
Teachers:
- UNAM: María del Pilar Ángeles Full time professor
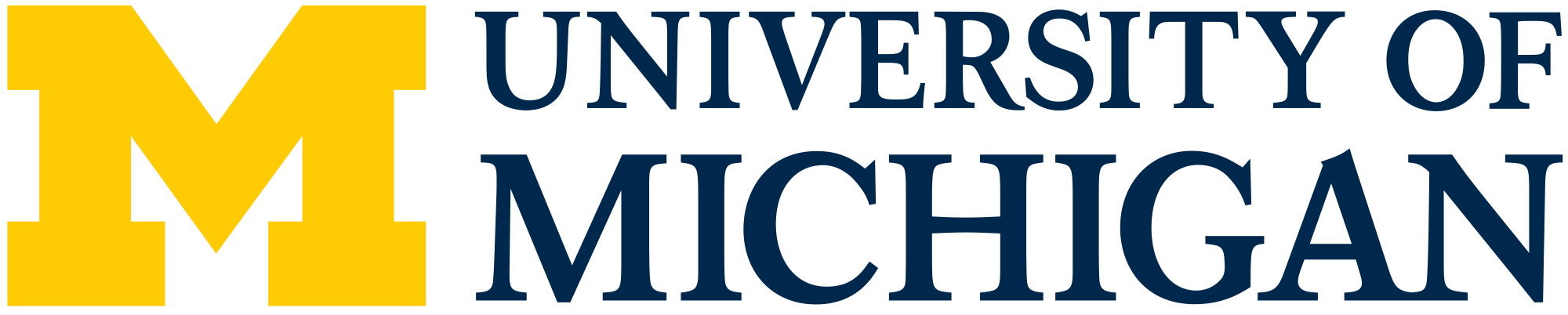
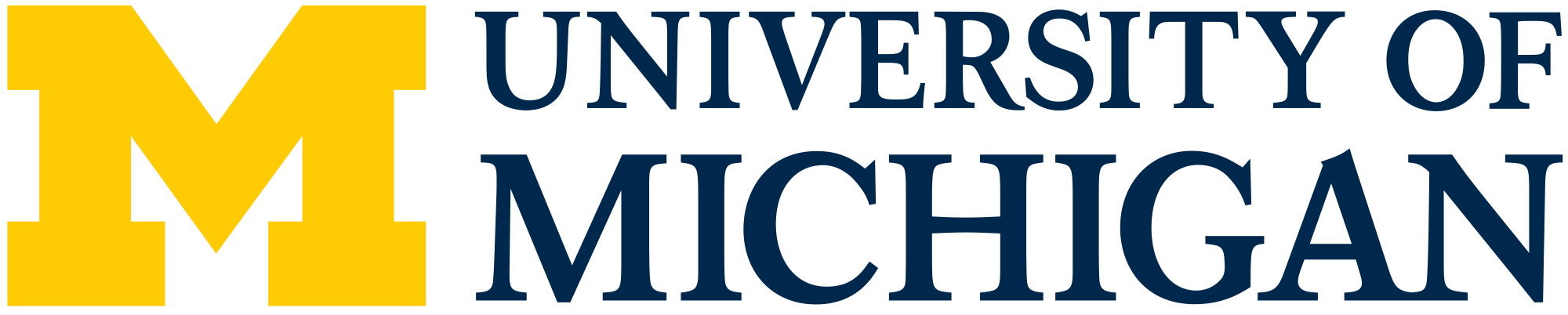
Statistics with Python
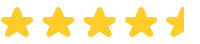
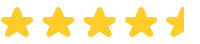
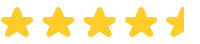
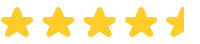
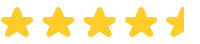
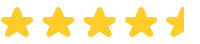
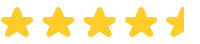
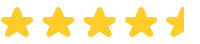
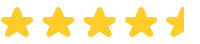
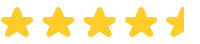
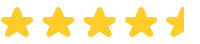
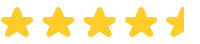
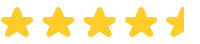
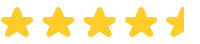
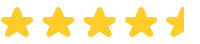
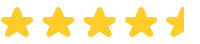
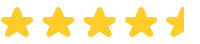
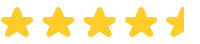
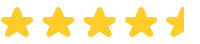
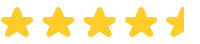
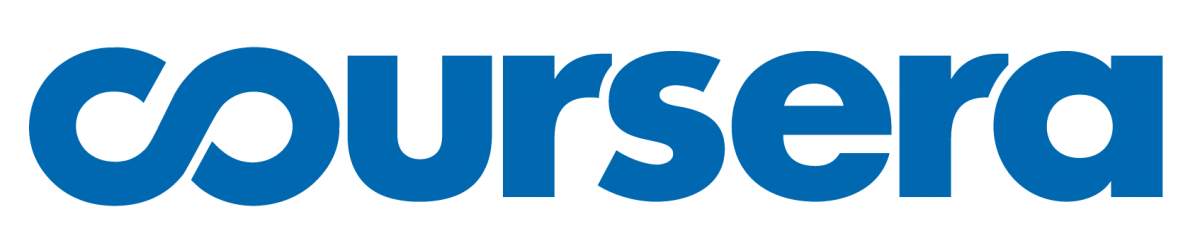
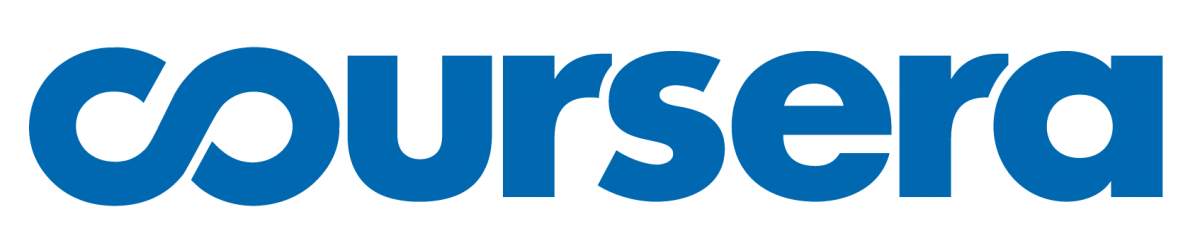
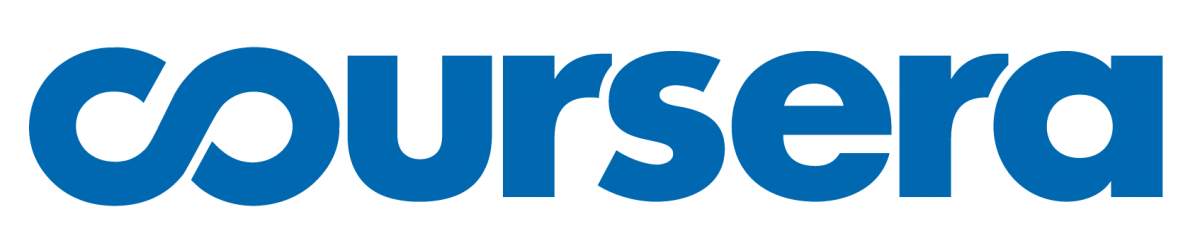
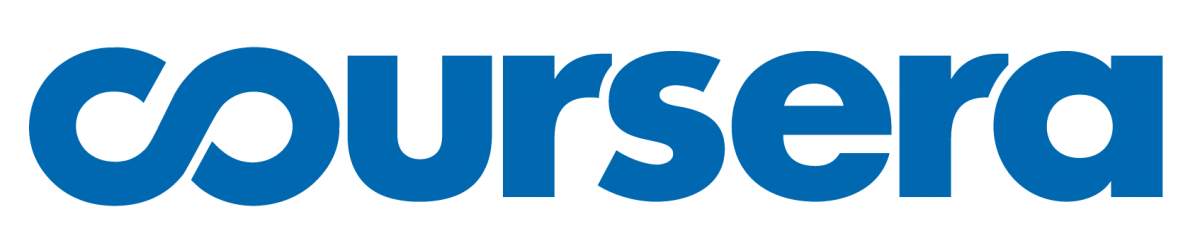
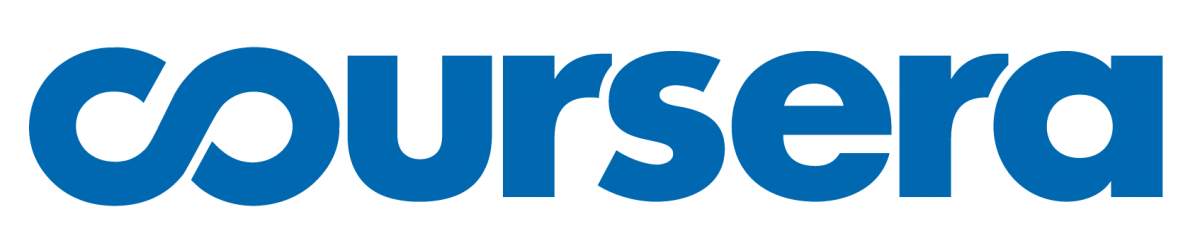
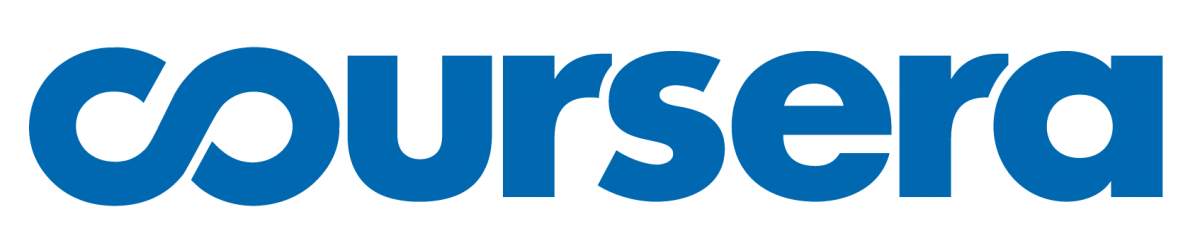
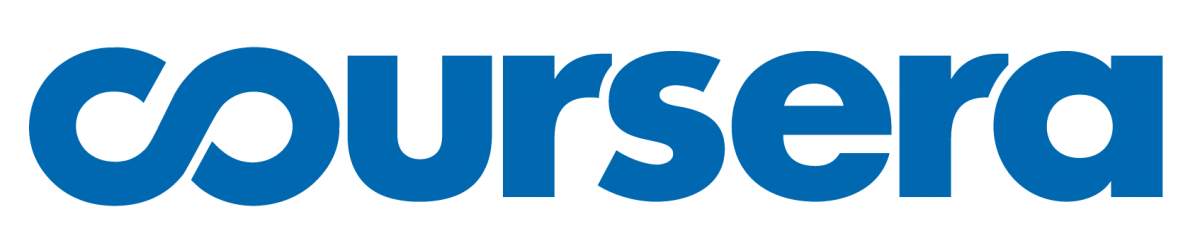
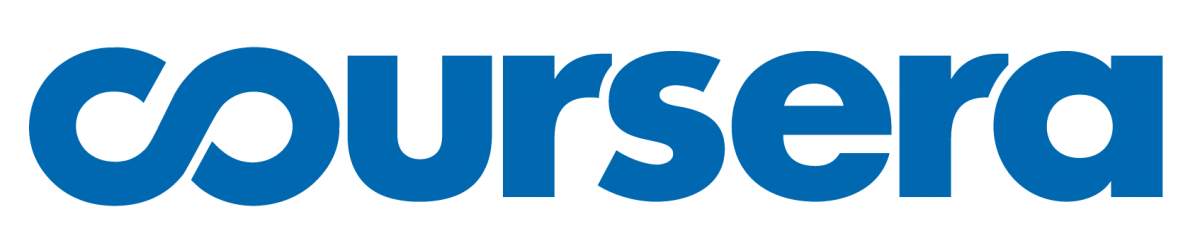
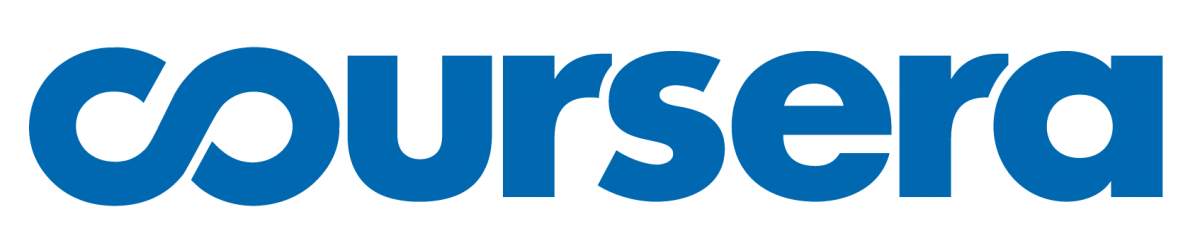
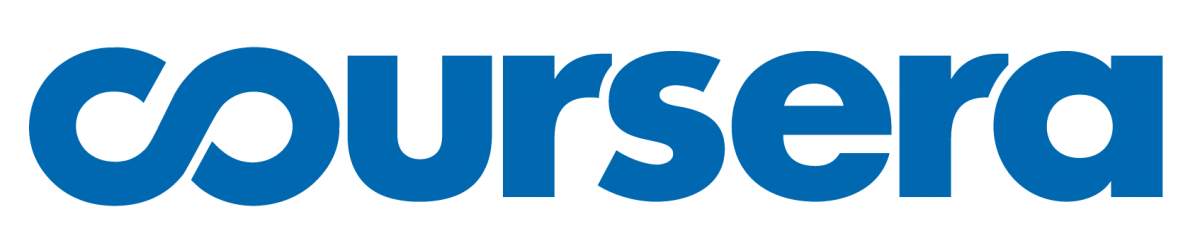
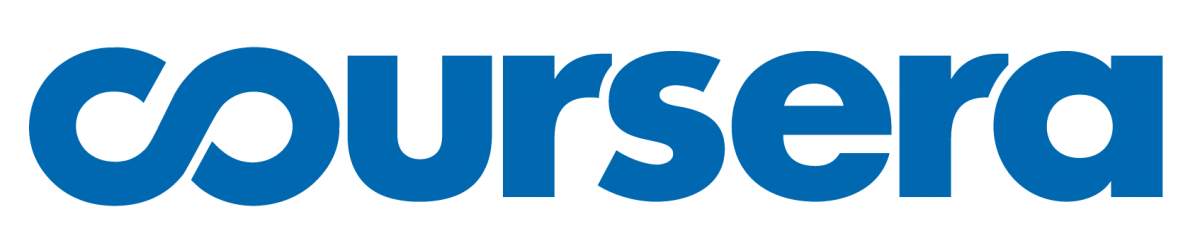
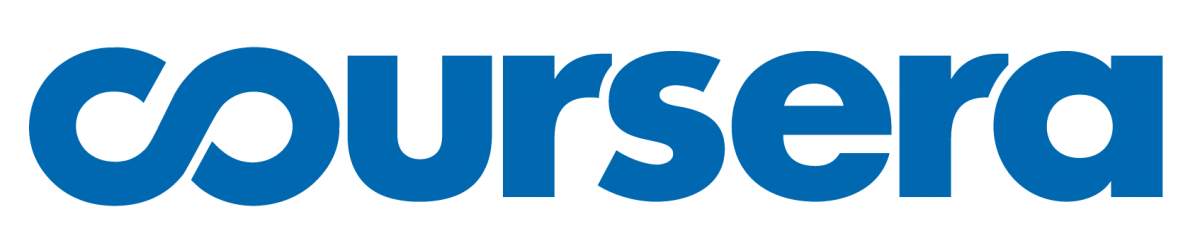
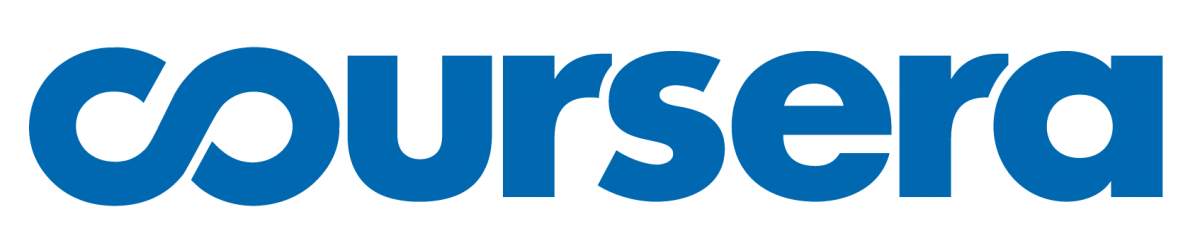
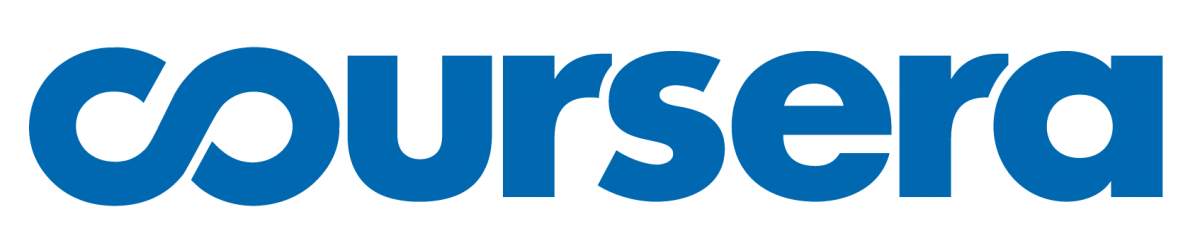
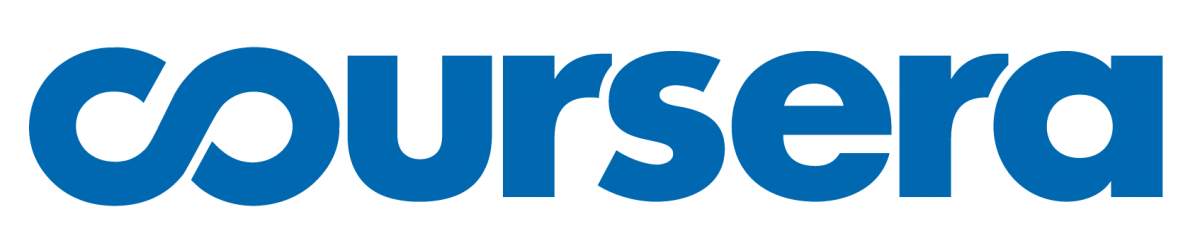
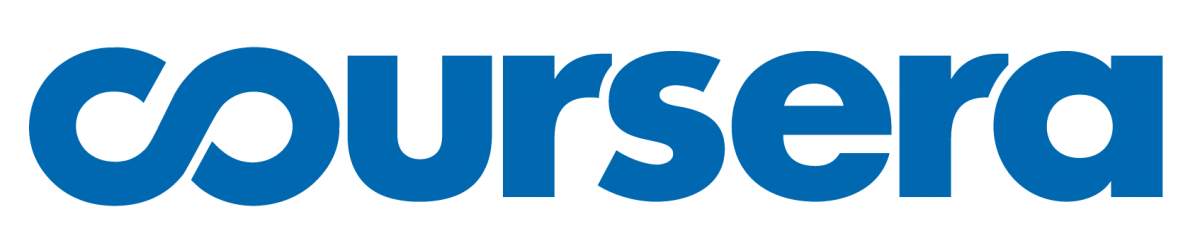
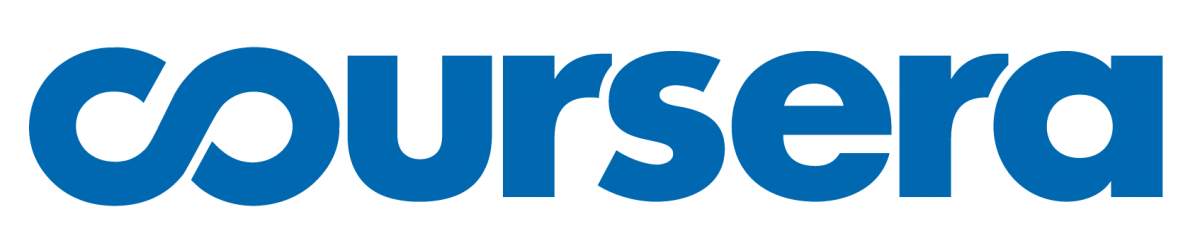
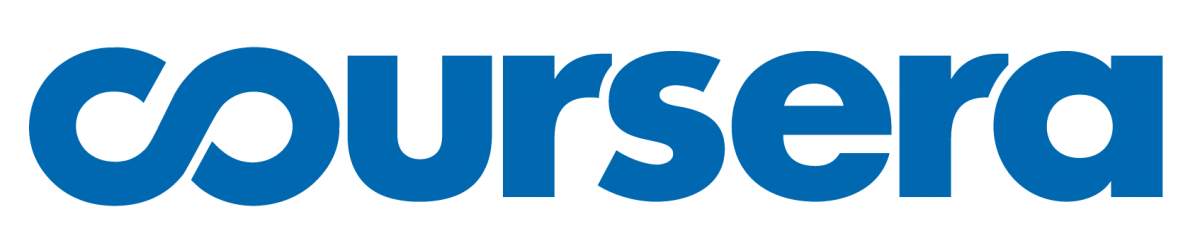
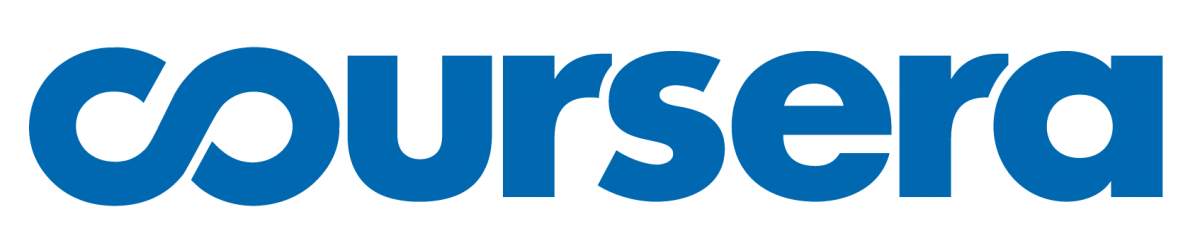
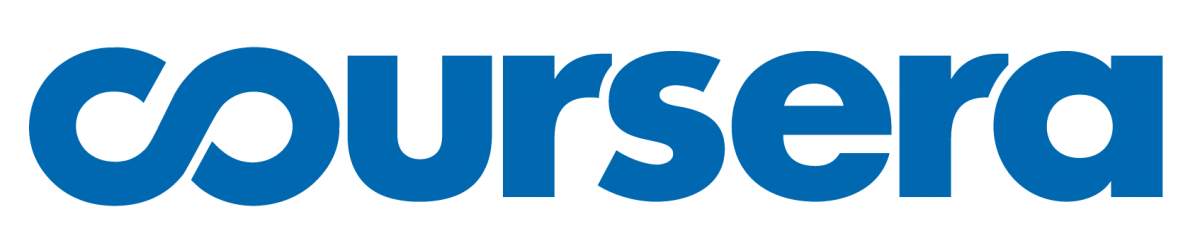
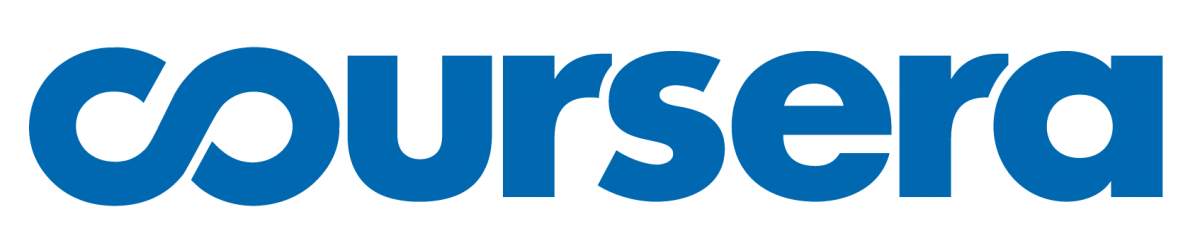
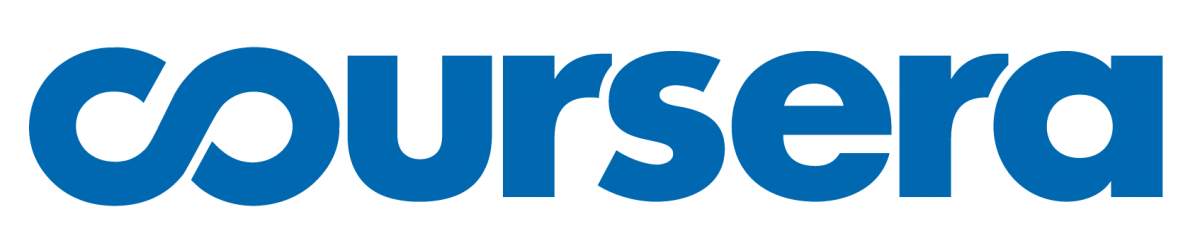
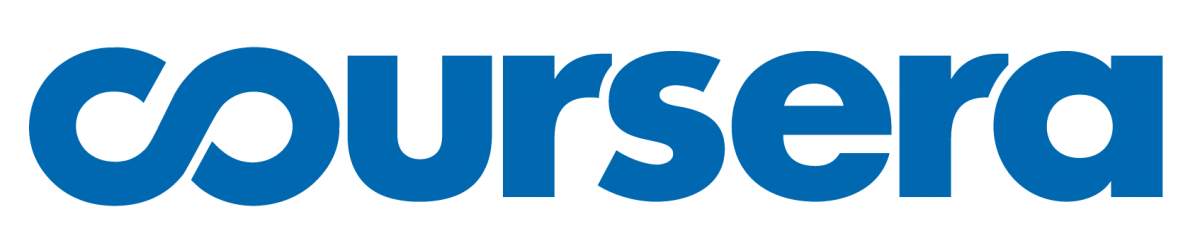
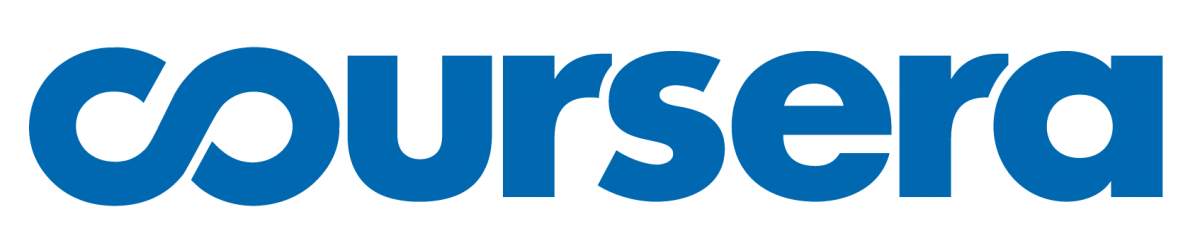
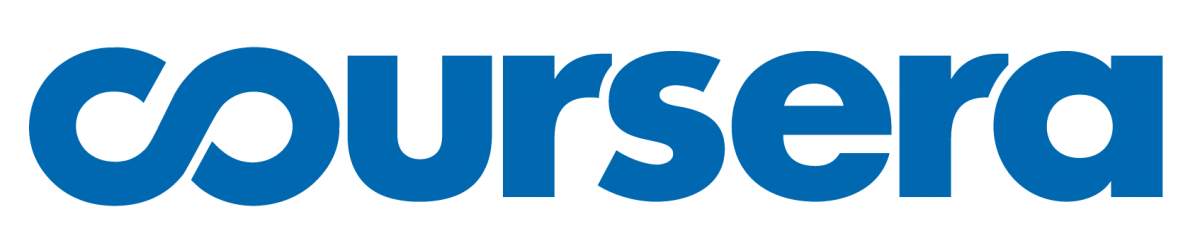
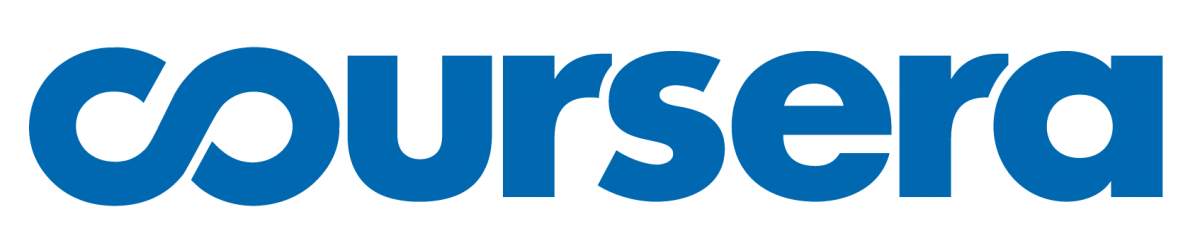
Description
The specialization offers students to learn the beginning and intermediate concepts of statistical analysis, and use of the Python programming language to conduct data analysis. It consists of 3 courses: Understanding and Visualizing Data with Python, Inferential Statistics Analysis with Python, Fitting Statistical Models to data with Python.
Teachers:
- University of Michigan: Kerby Shedden Professor
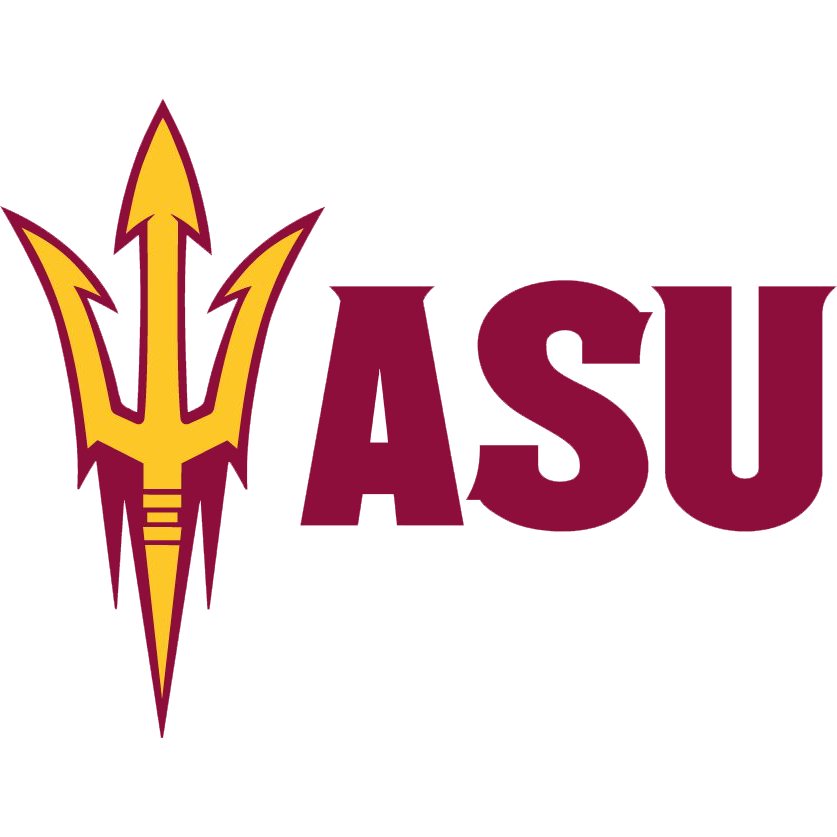
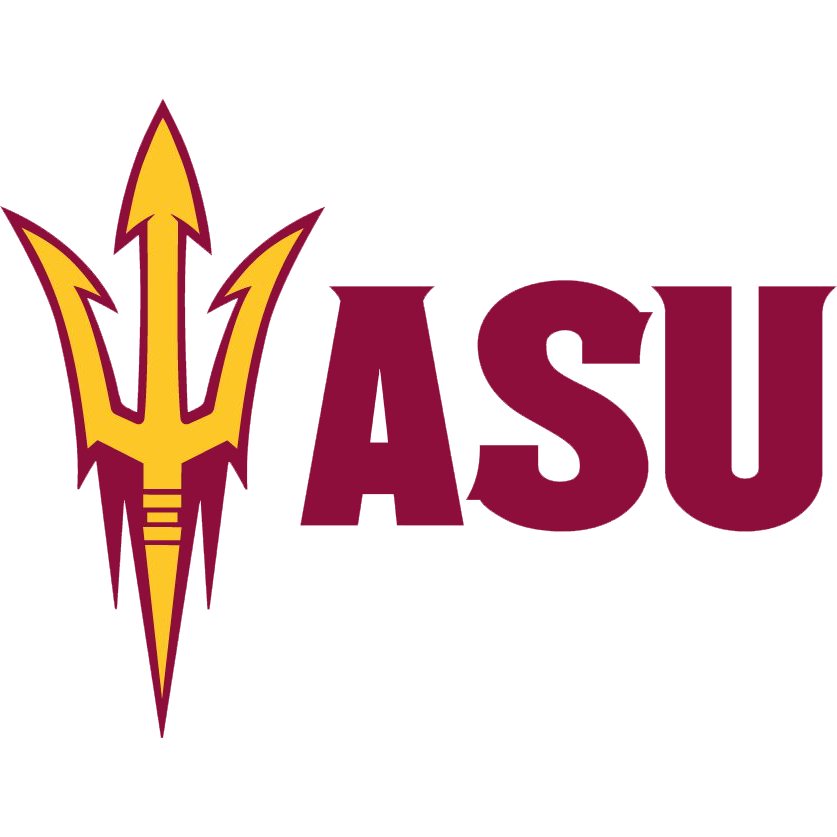
Data Visualization
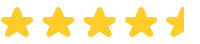
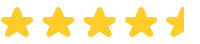
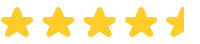
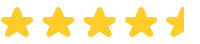
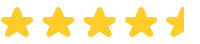
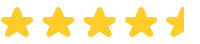
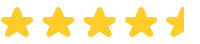
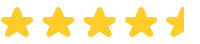
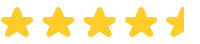
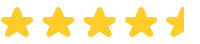
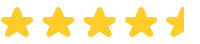
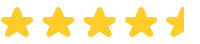
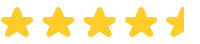
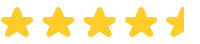
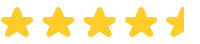
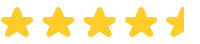
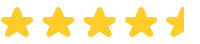
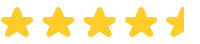
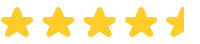
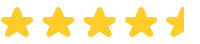
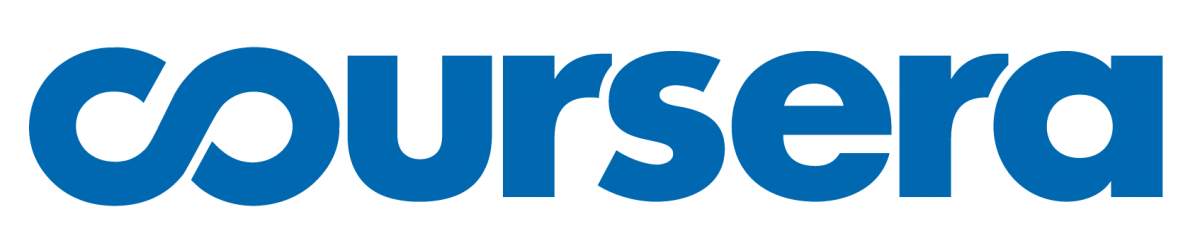
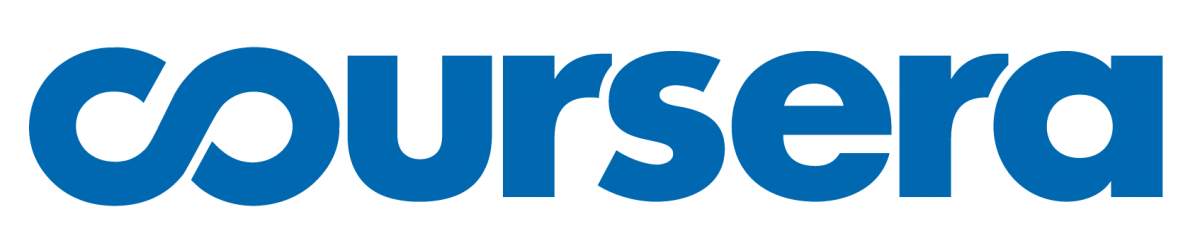
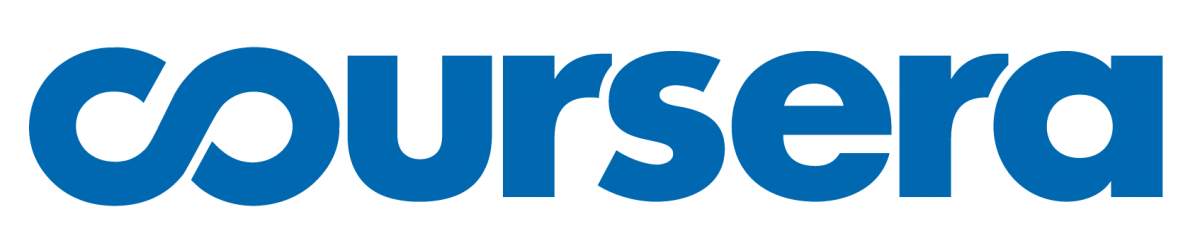
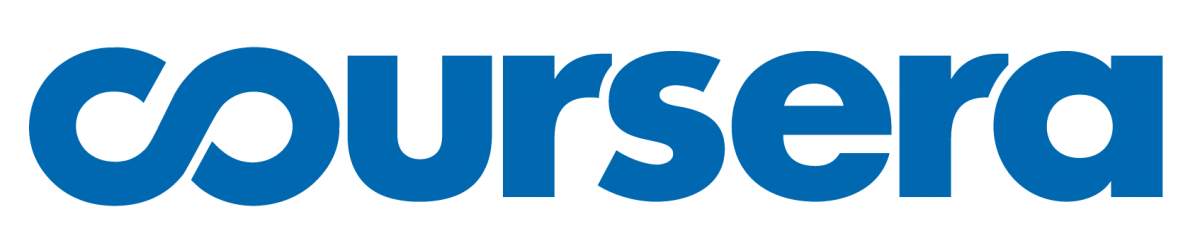
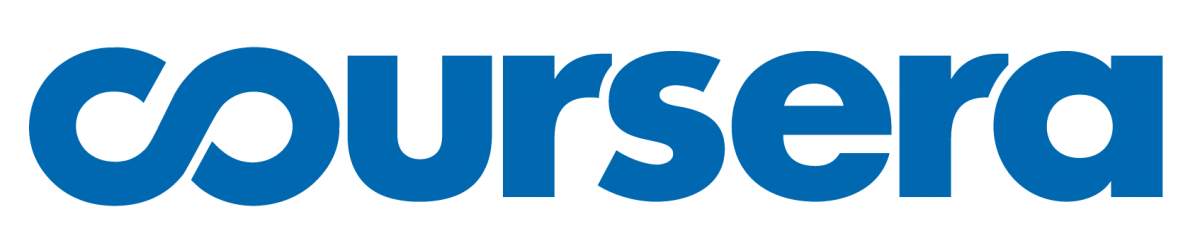
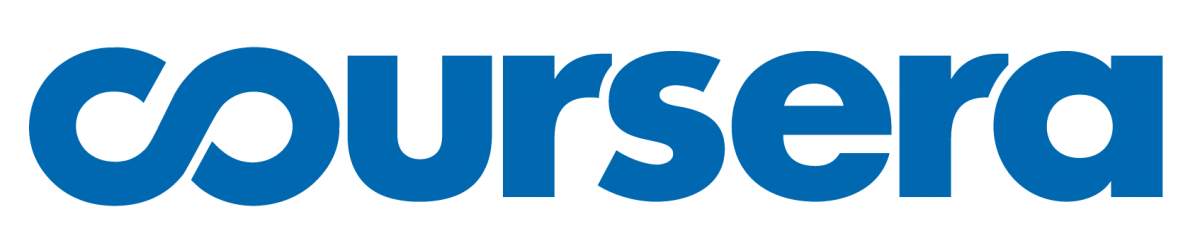
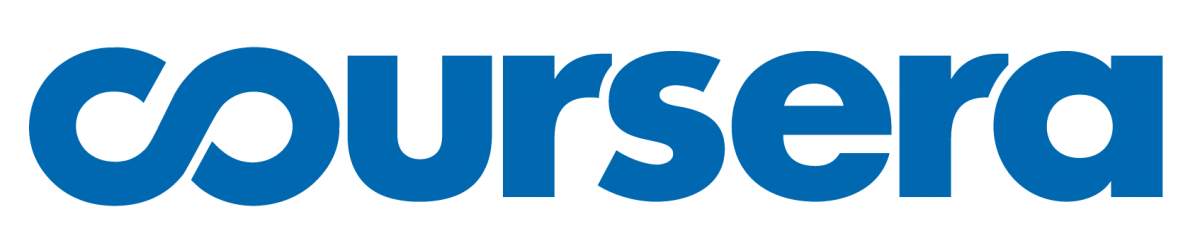
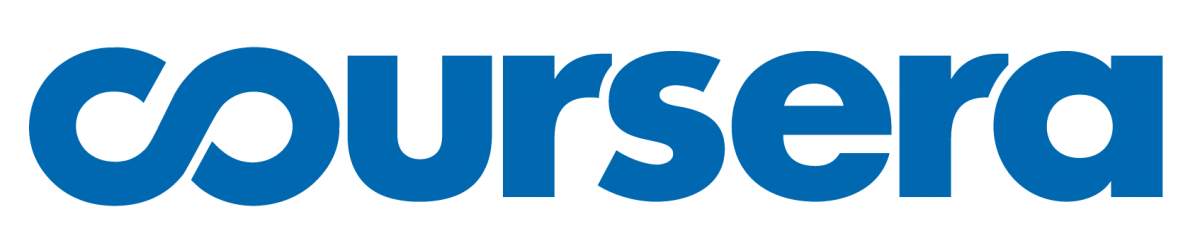
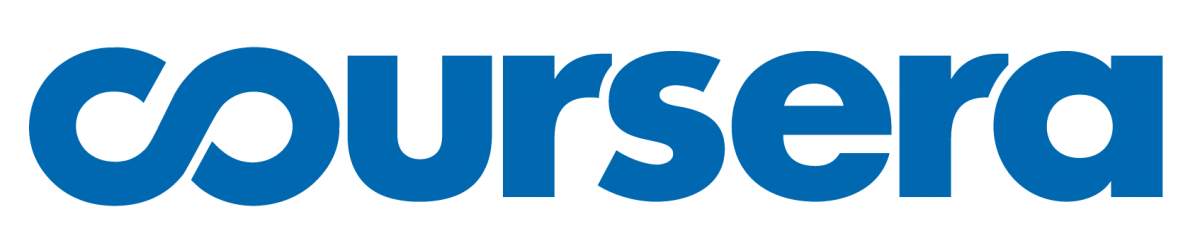
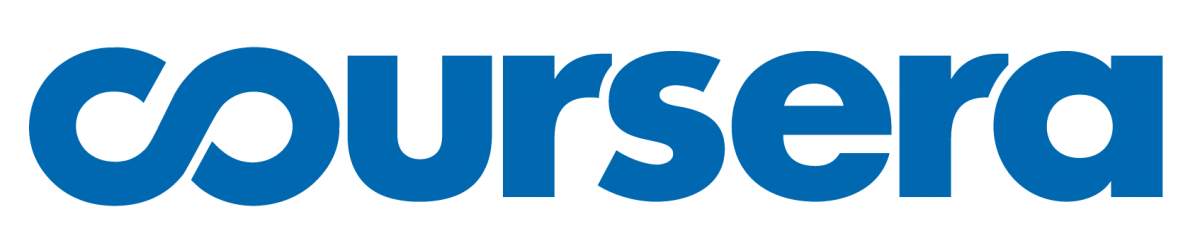
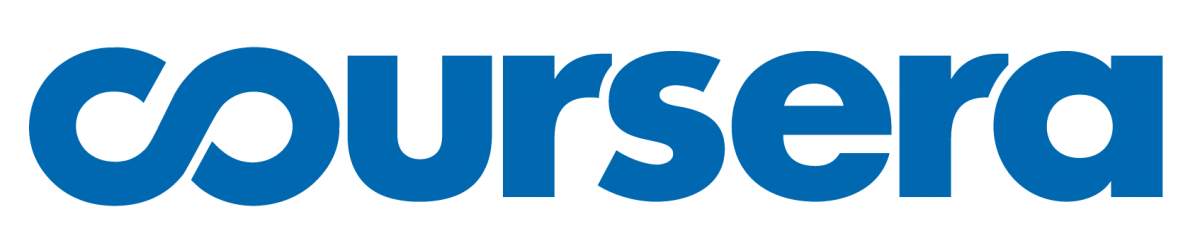
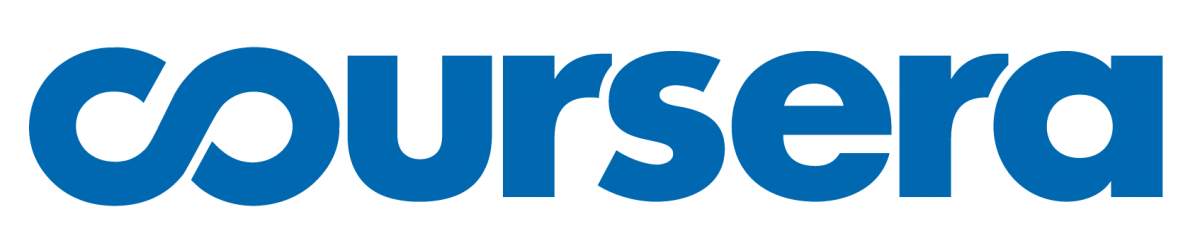
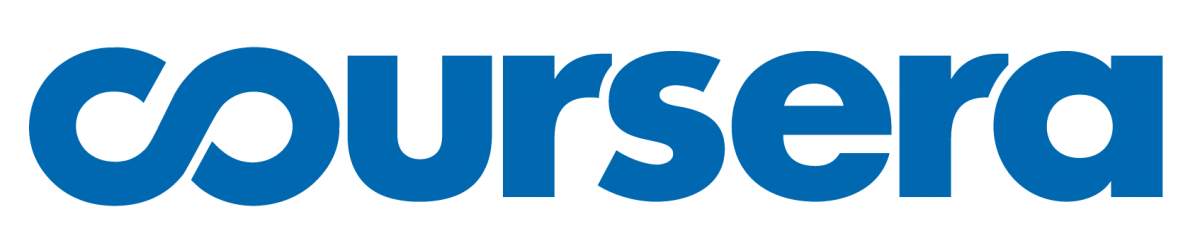
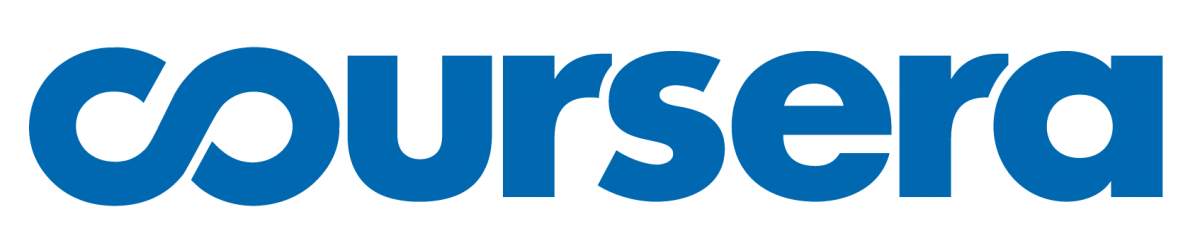
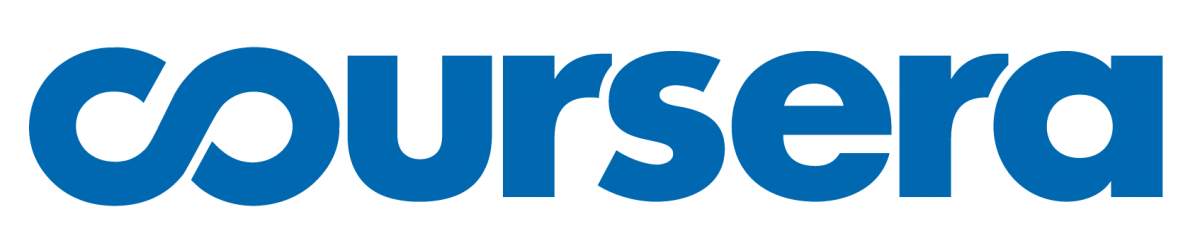
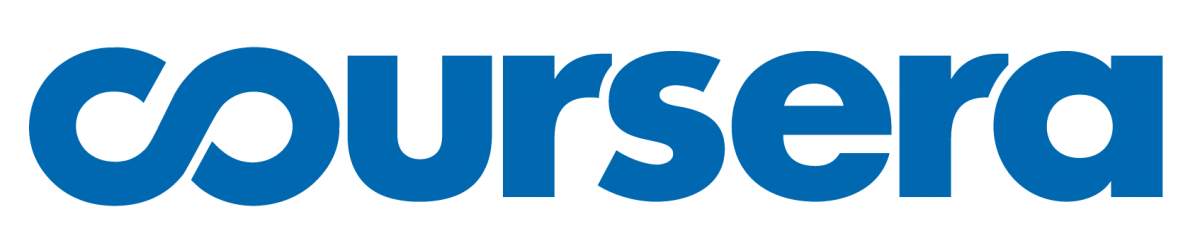
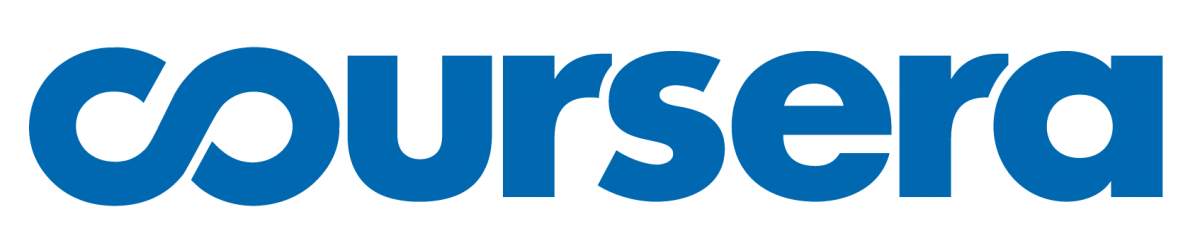
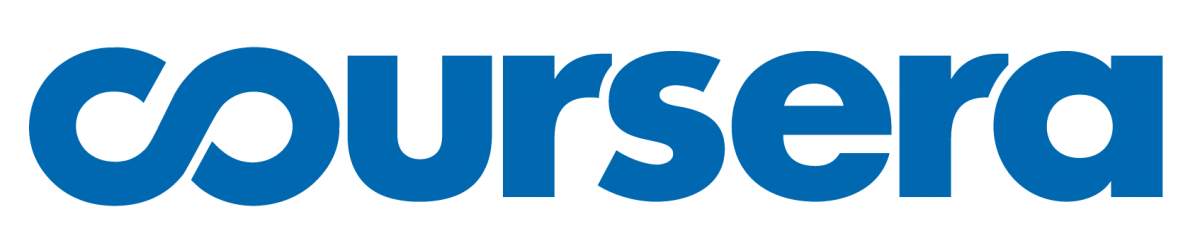
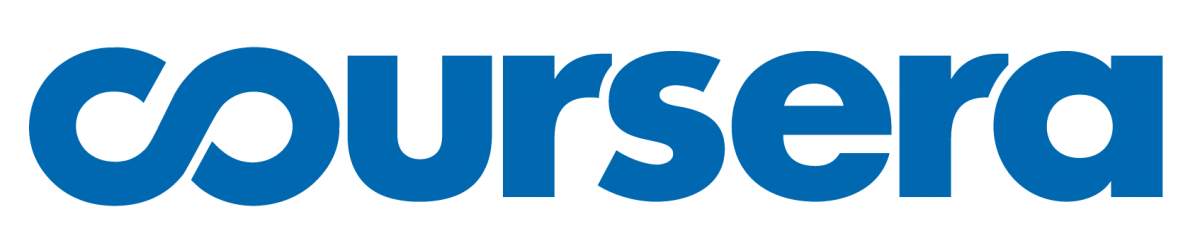
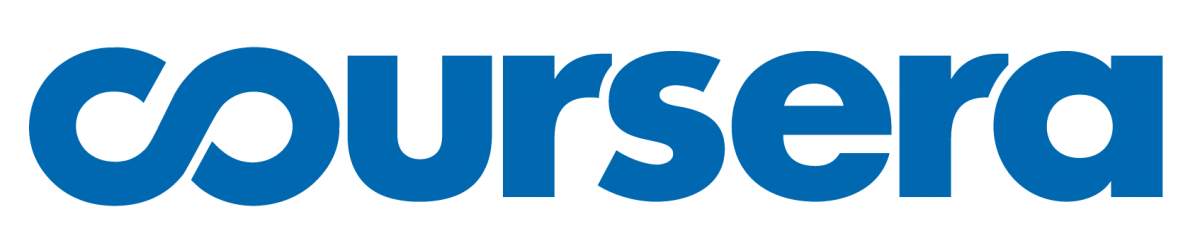
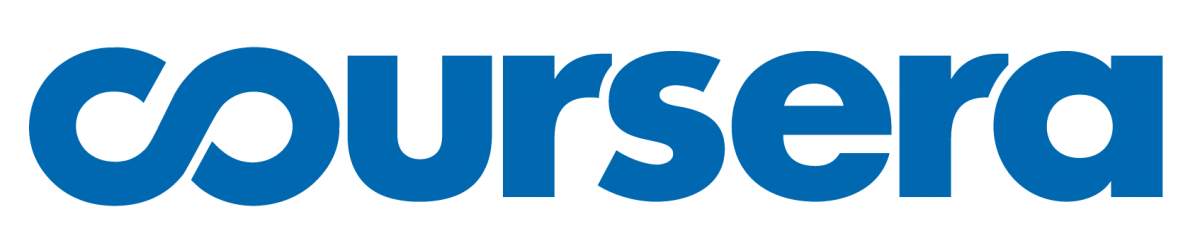
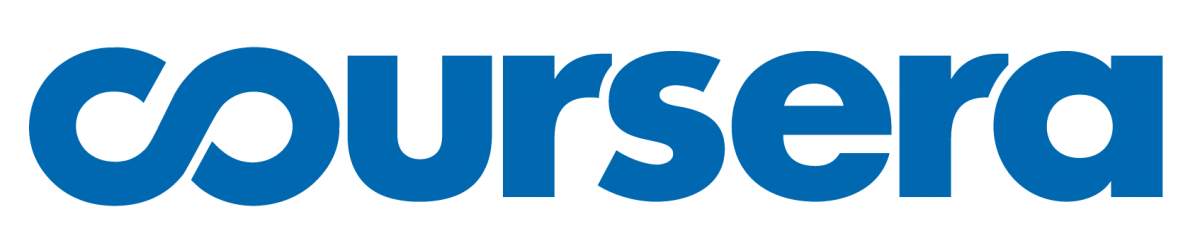
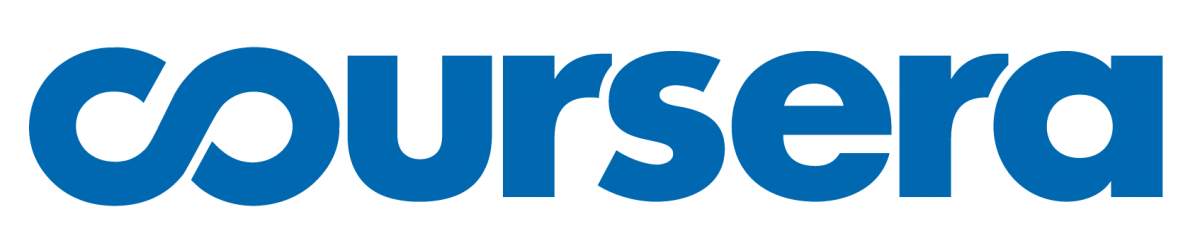
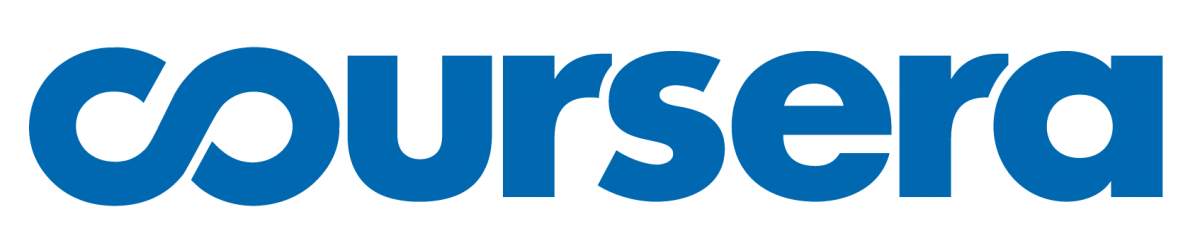
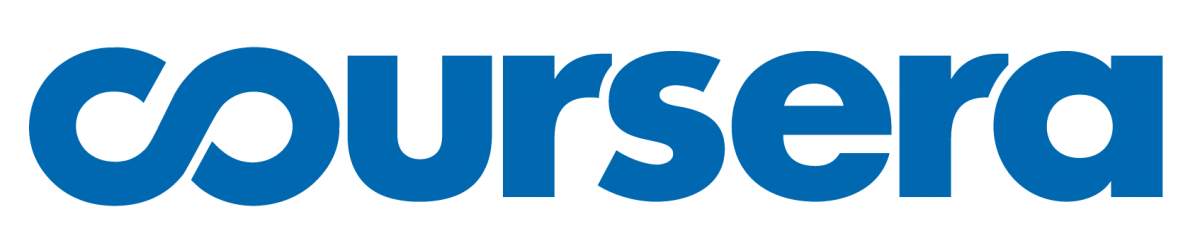
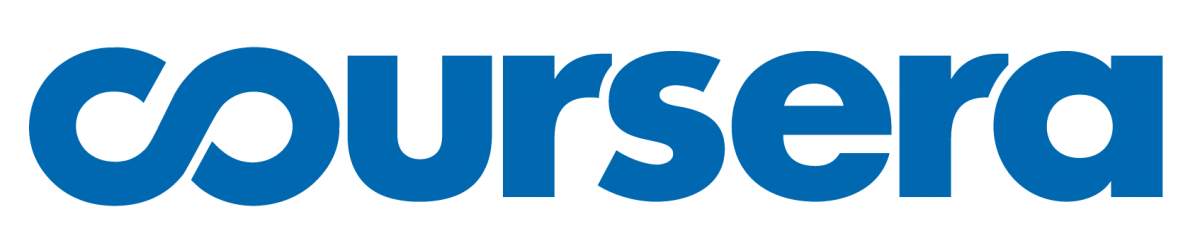
Description
Techniques and algorithms for creating effective visualizations based on principles from graphic design, visual art, perceptual psychology, and cognitive science to enhance the understanding of complex data.
Teachers:
- Arizona State University: Ross Maciejewsk, Associate Professor
- :
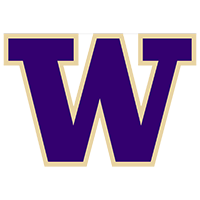
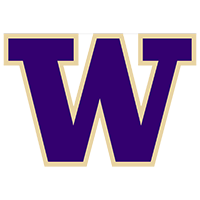
Data Science at Scale
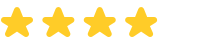
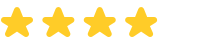
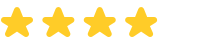
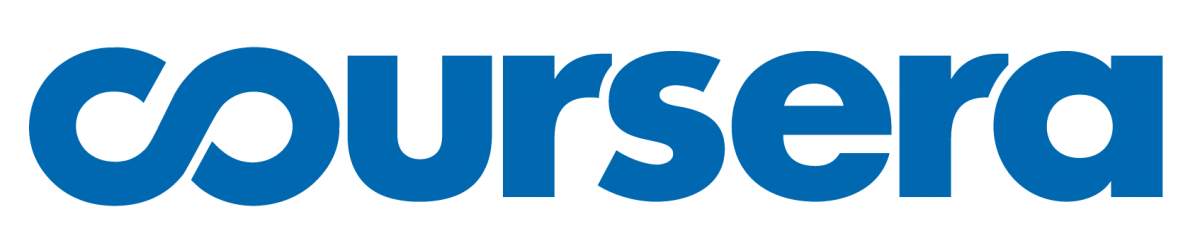
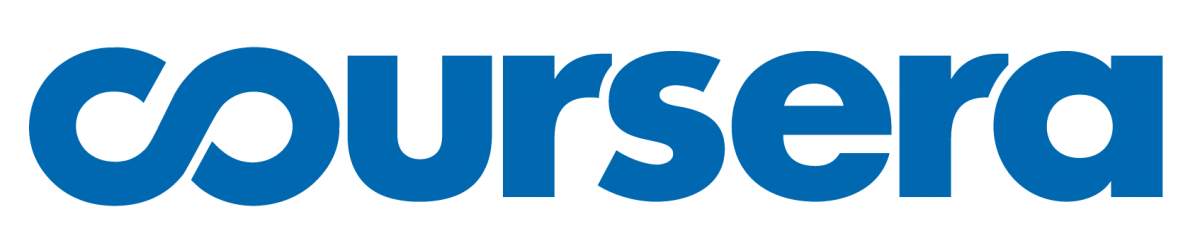
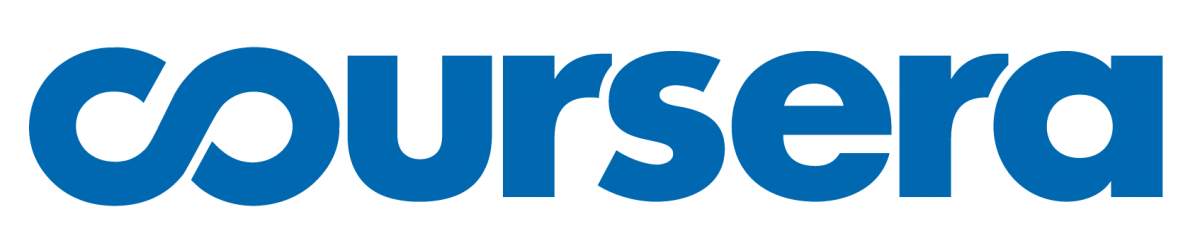
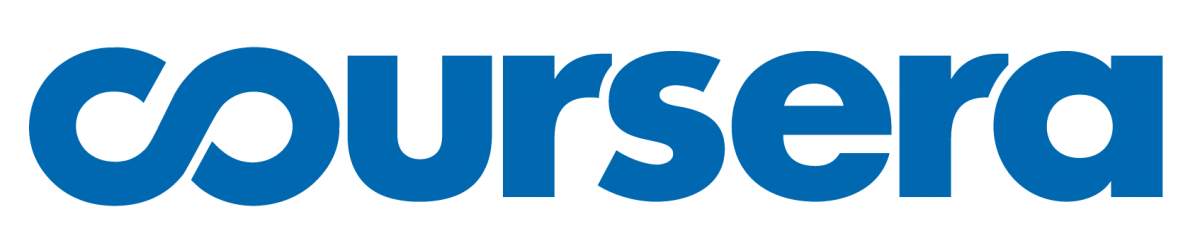
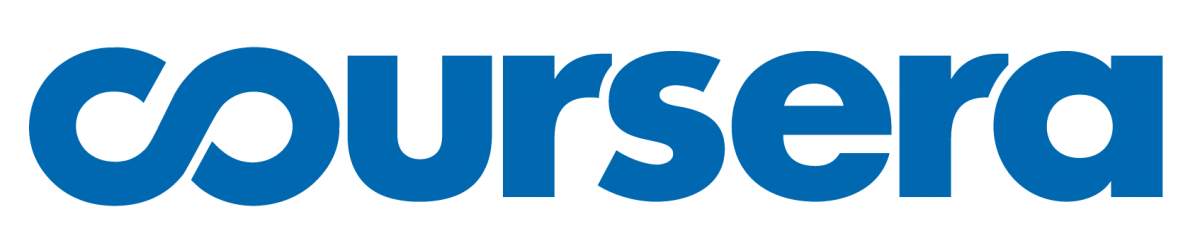
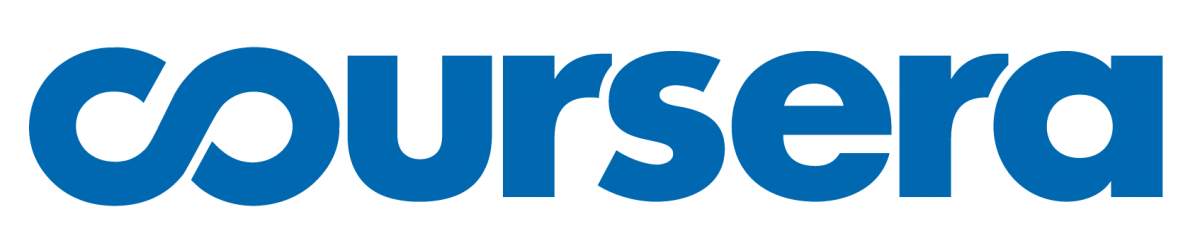
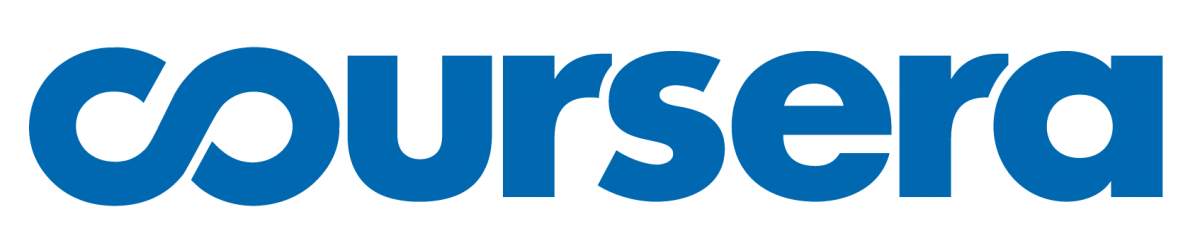
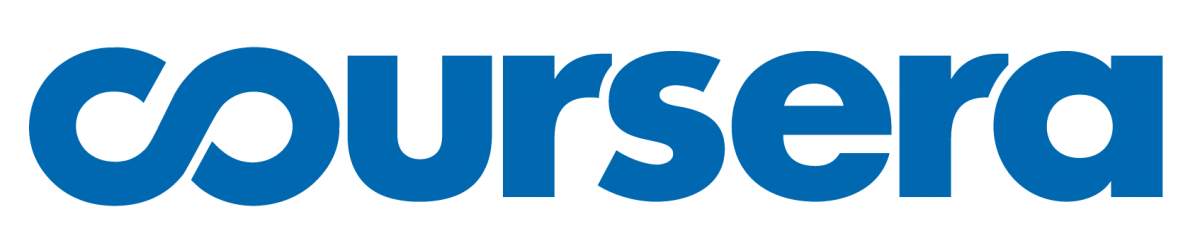
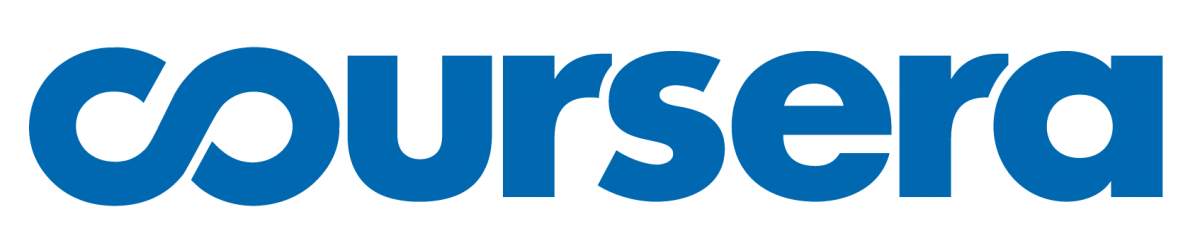
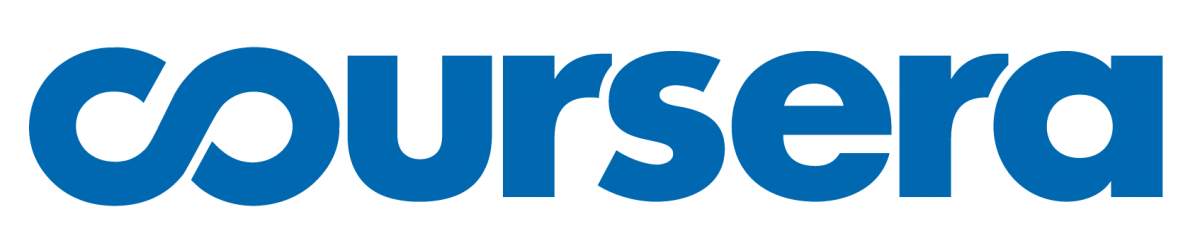
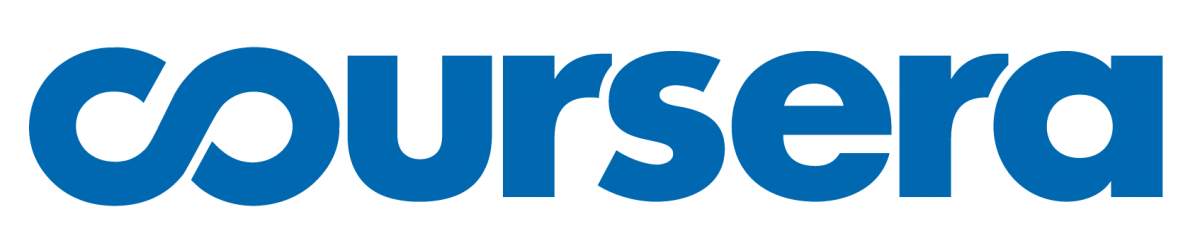
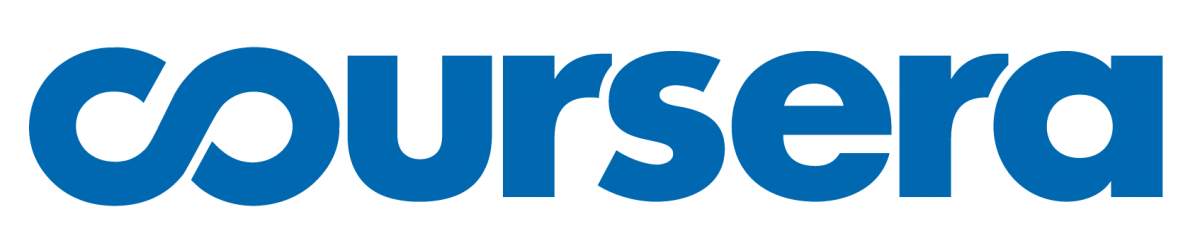
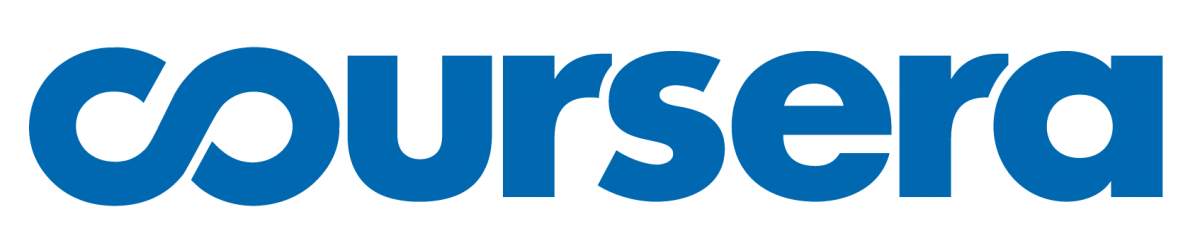
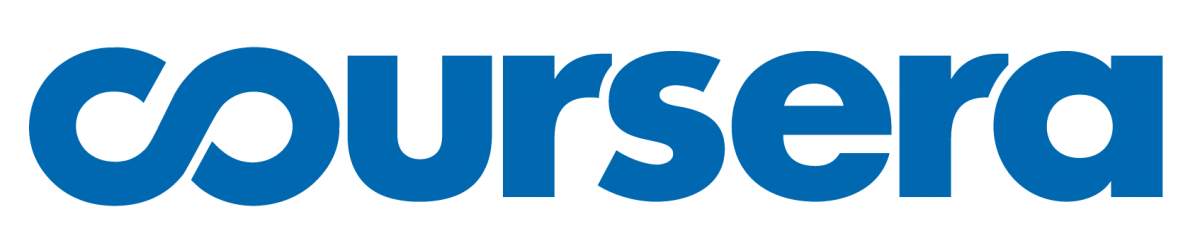
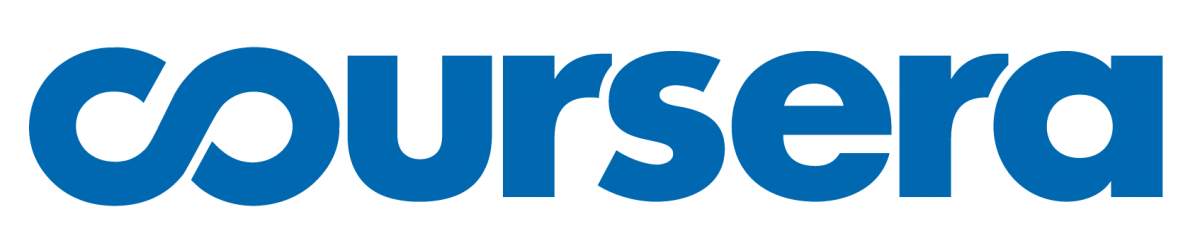
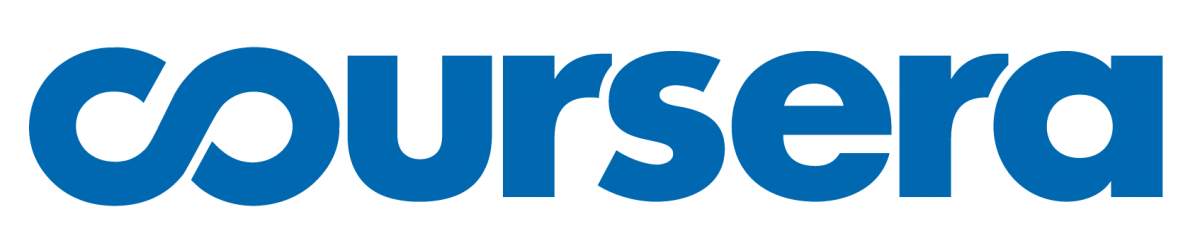
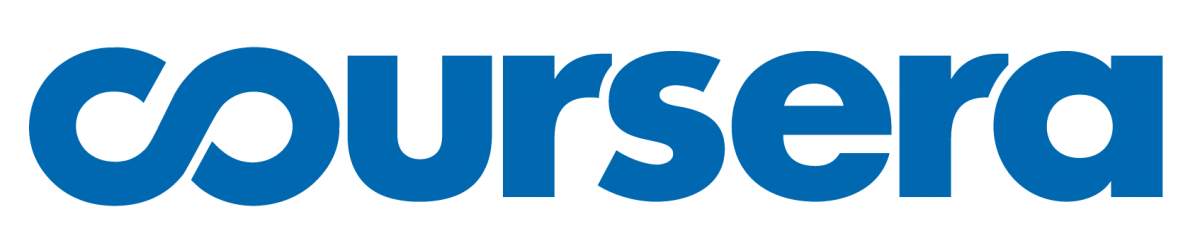
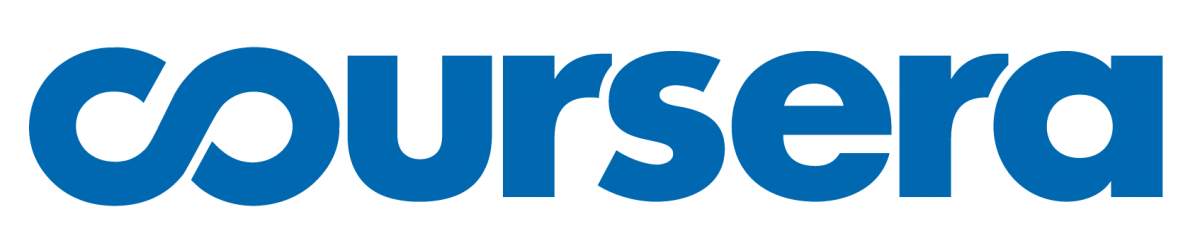
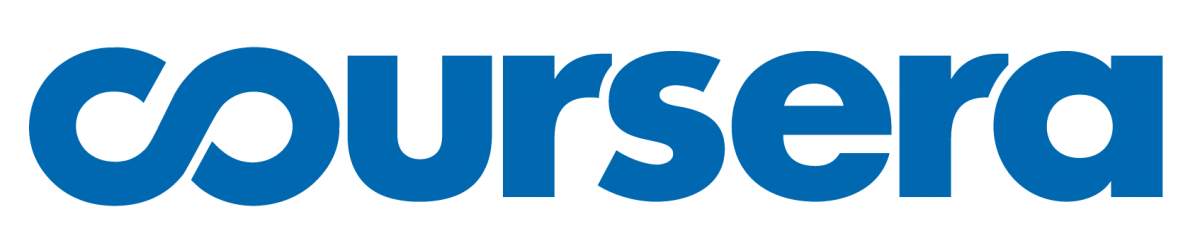
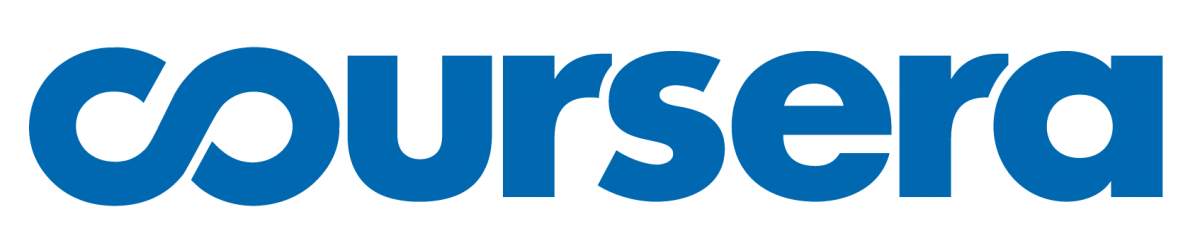
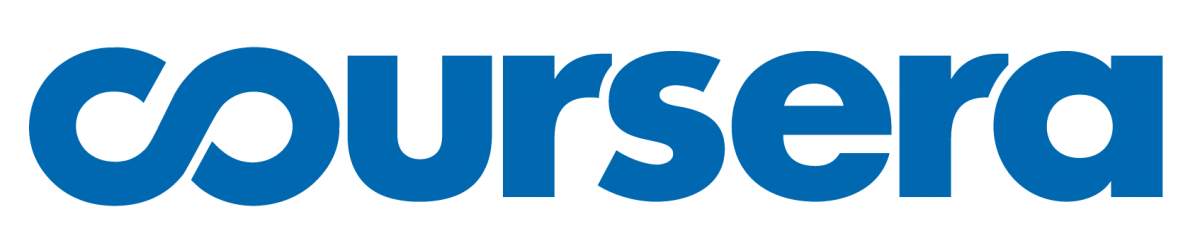
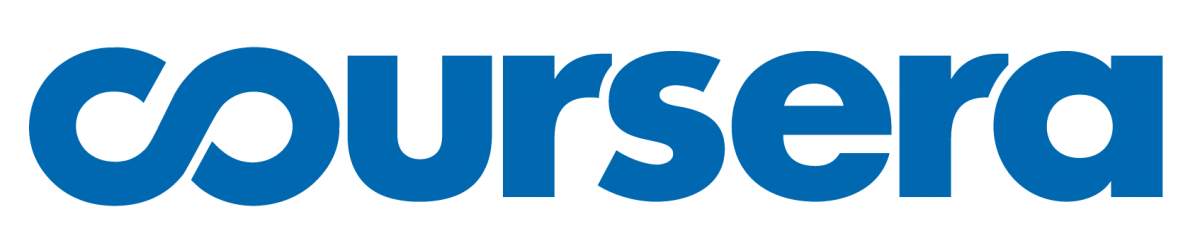
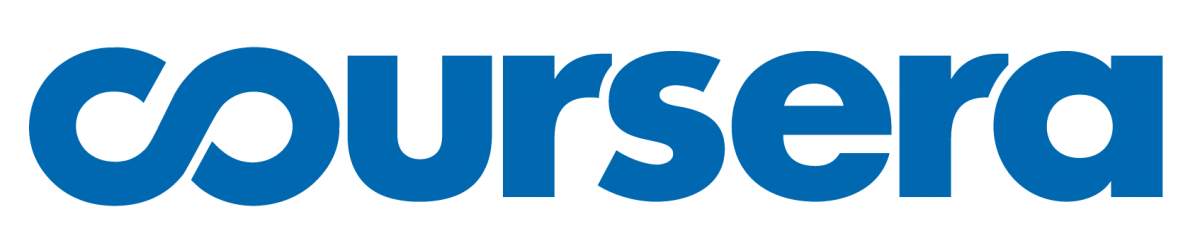
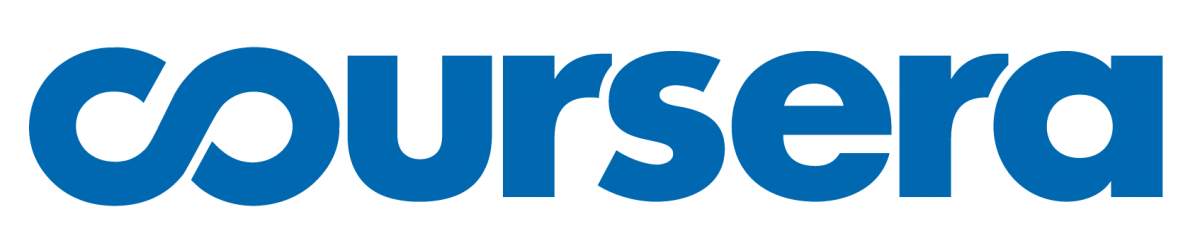
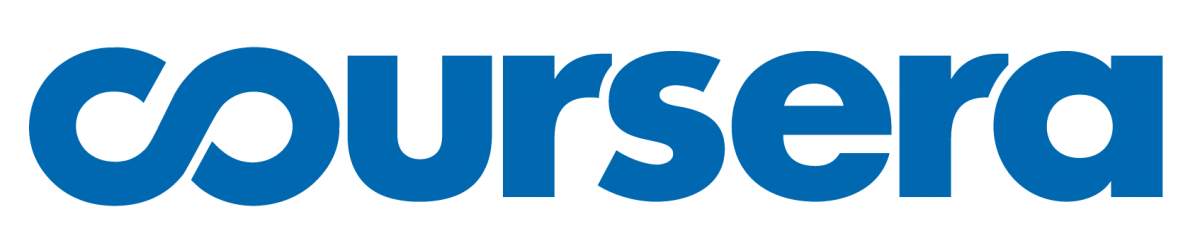
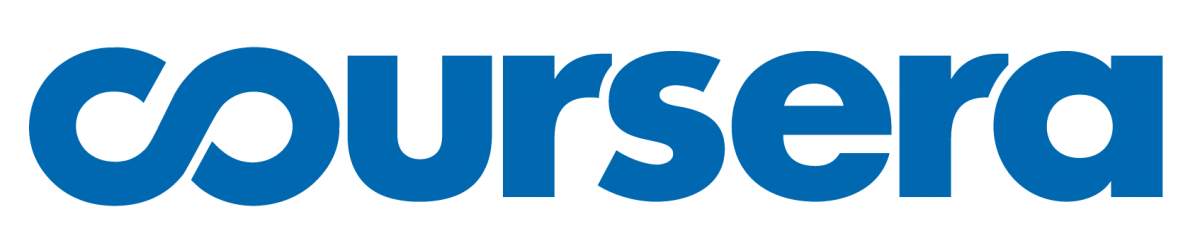
Description
4-course Specialization that covers: scalable data management, evaluation of big data technologies, and design of effective visualizations. Students will learn intermediate concepts from Data Science along with how to effectively visualize and present data, considering all legal and ethical issues that might arise.
Teachers:
- University of Washington: Bill Howe, Director of Research
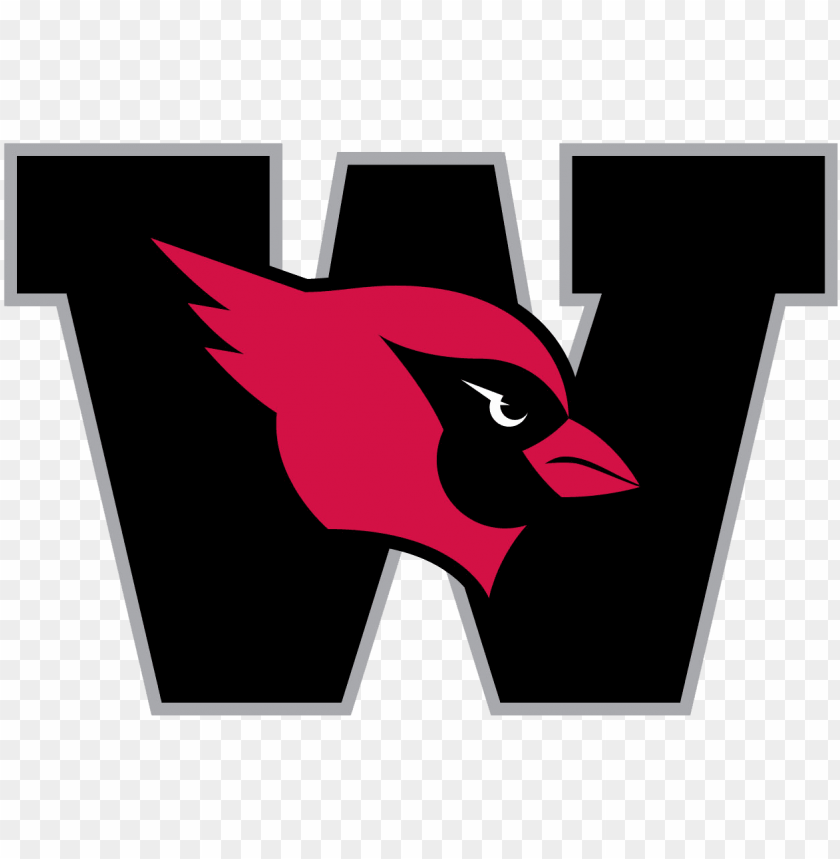
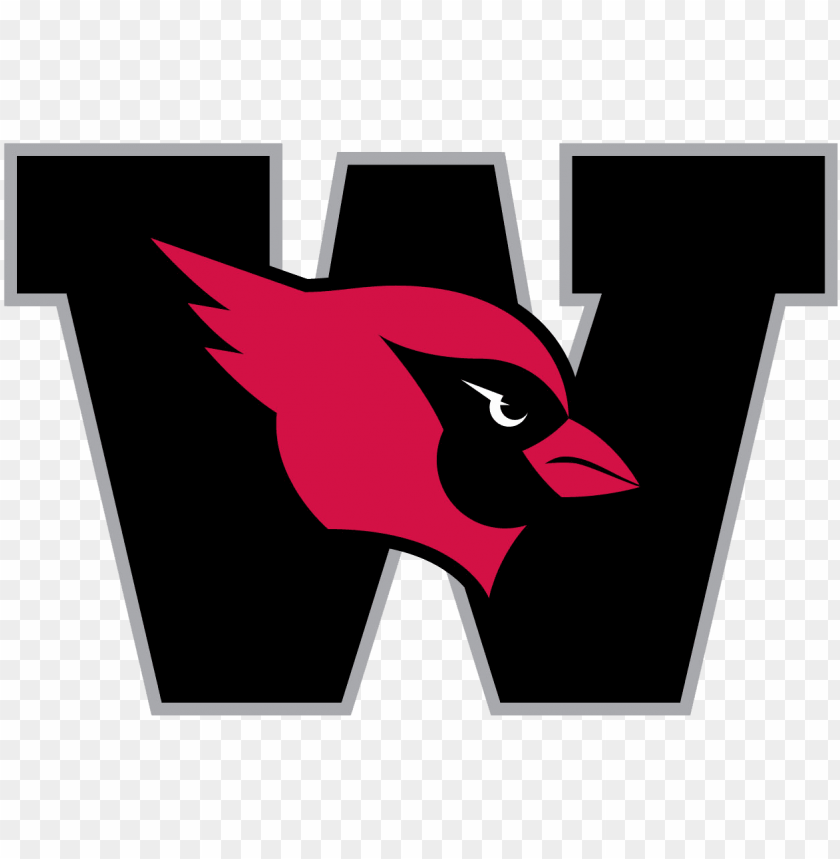
Data Analysis and Interpretation
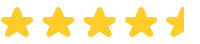
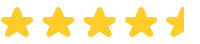
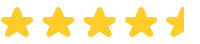
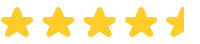
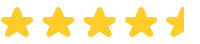
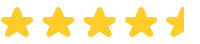
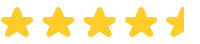
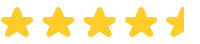
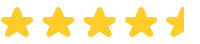
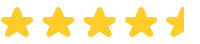
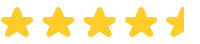
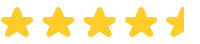
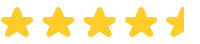
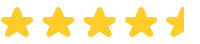
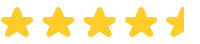
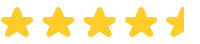
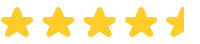
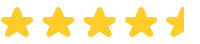
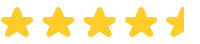
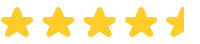
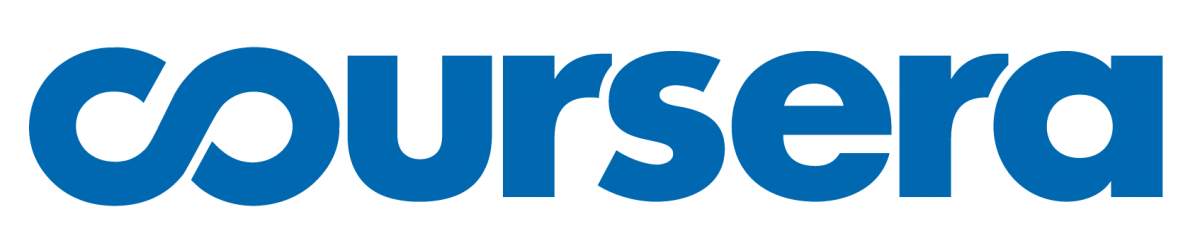
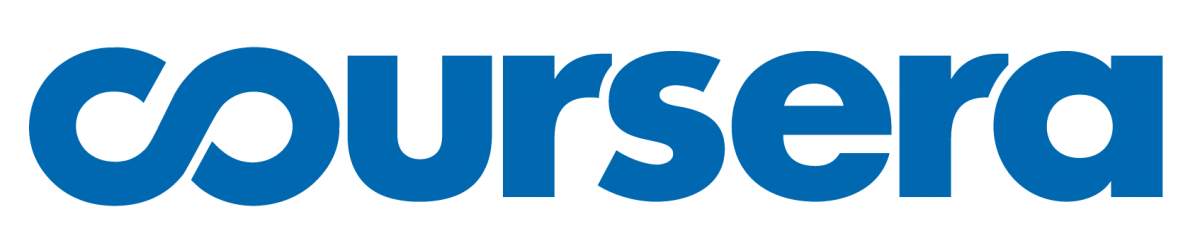
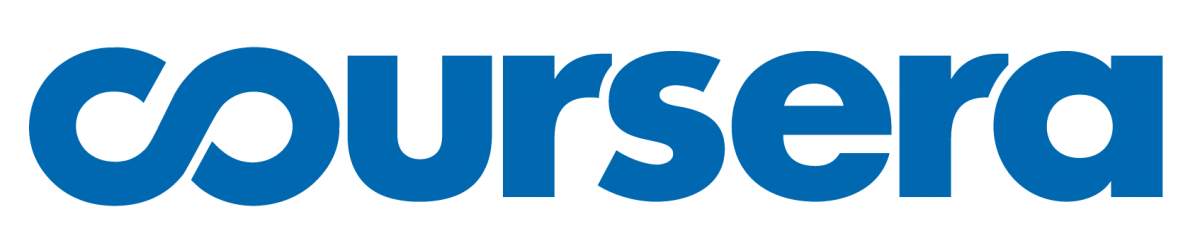
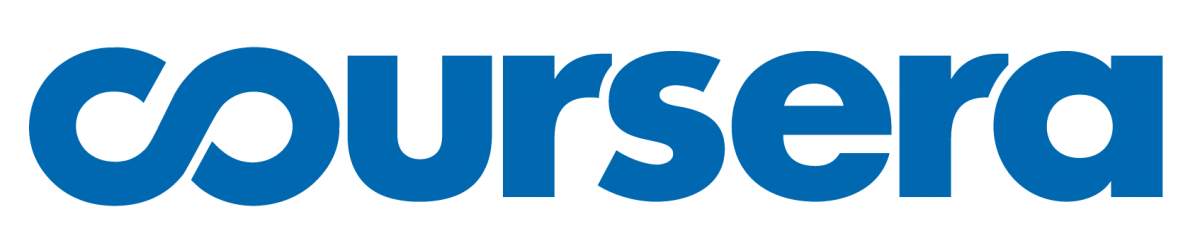
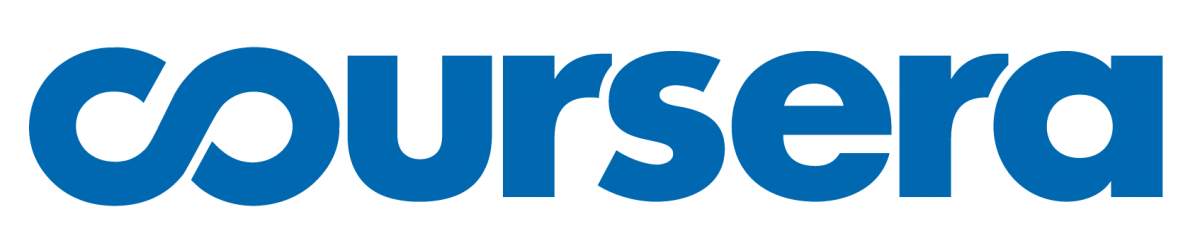
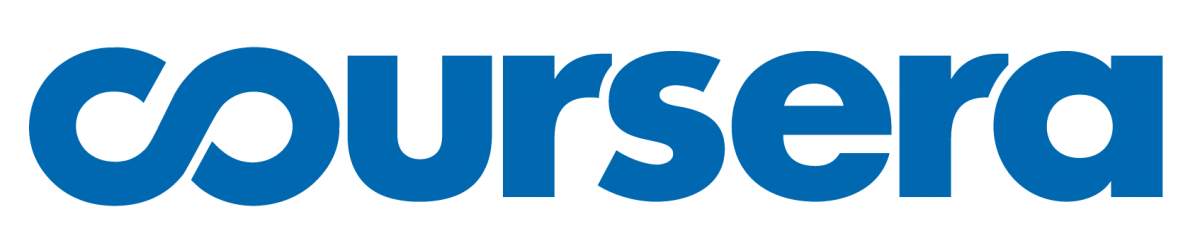
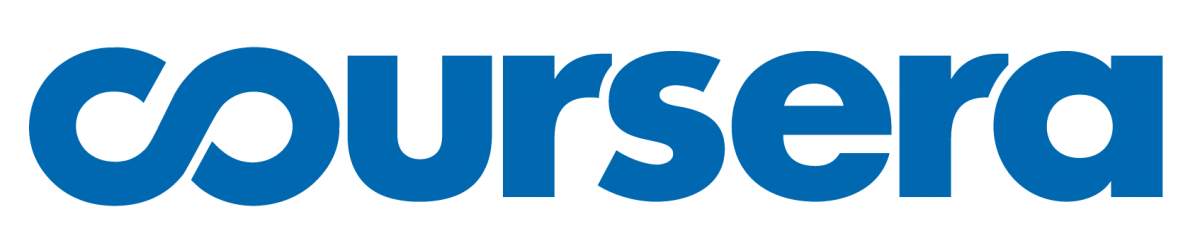
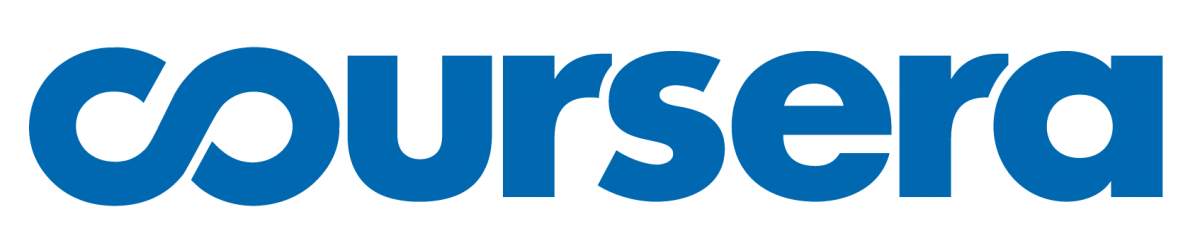
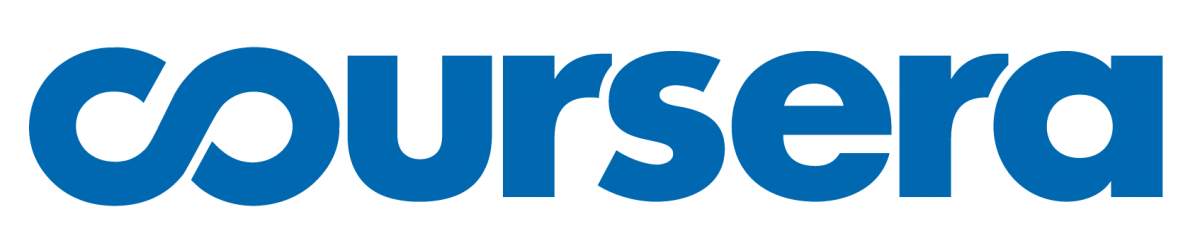
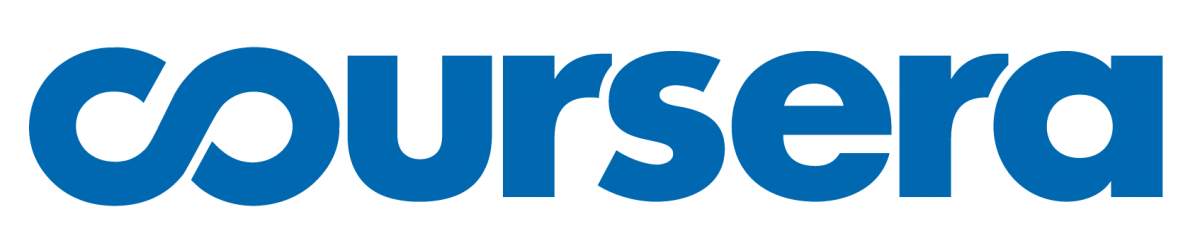
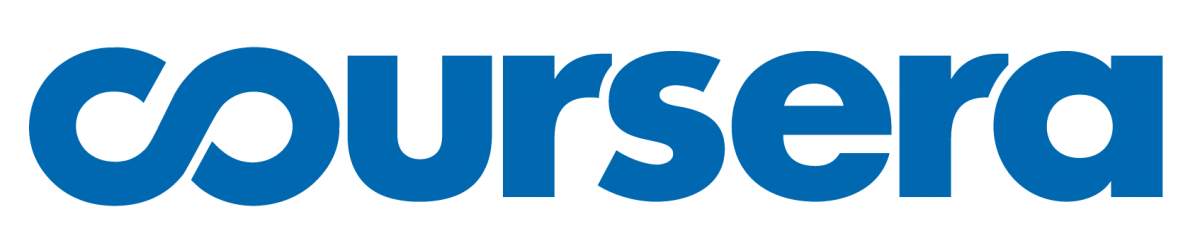
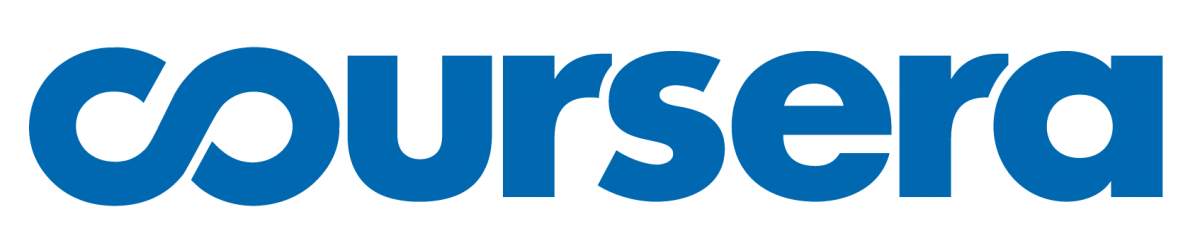
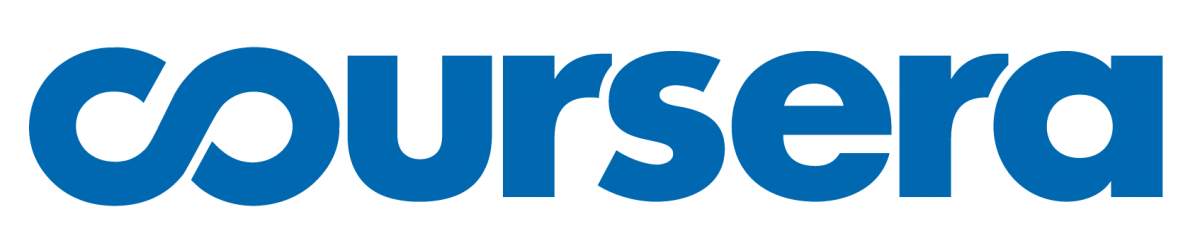
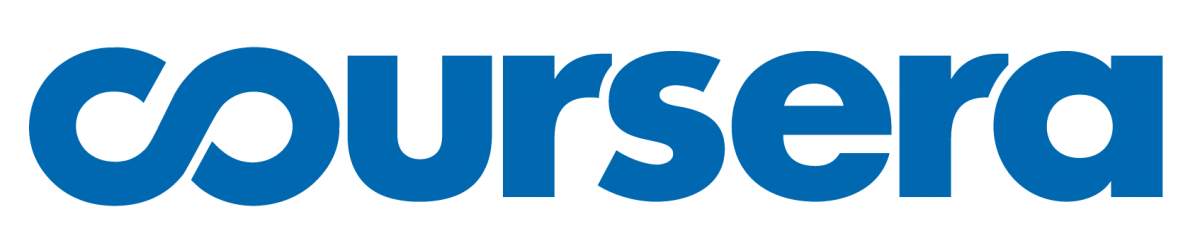
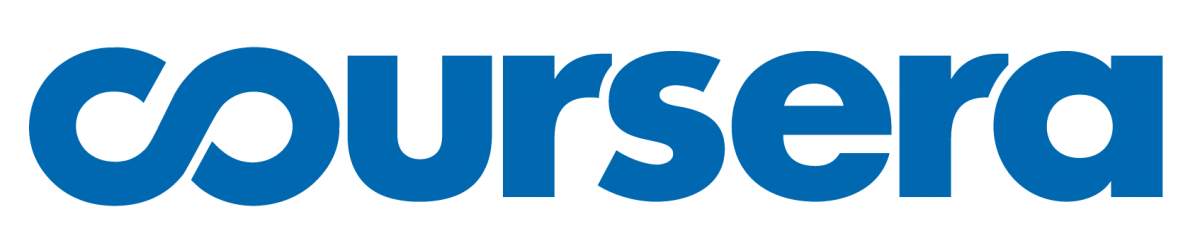
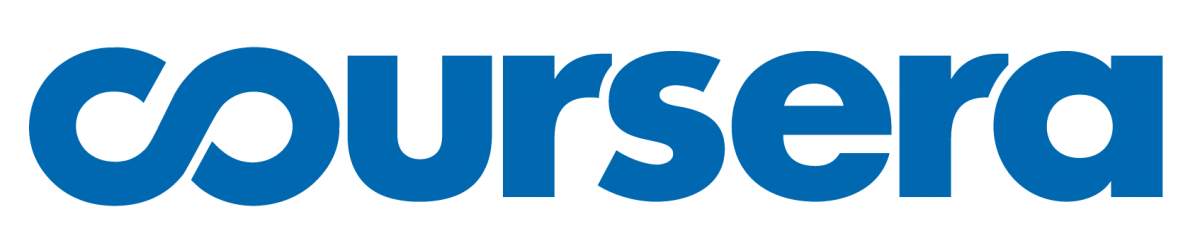
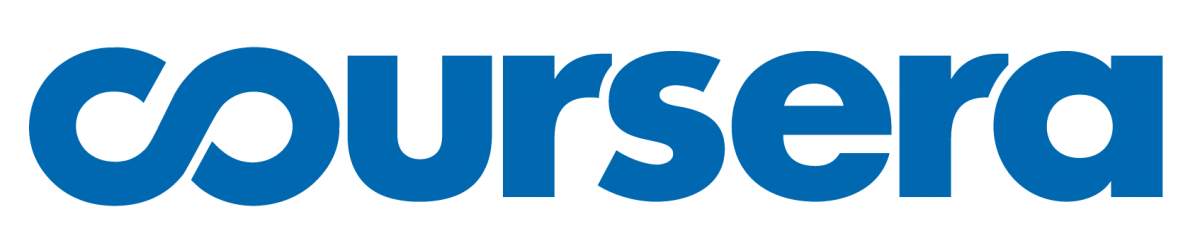
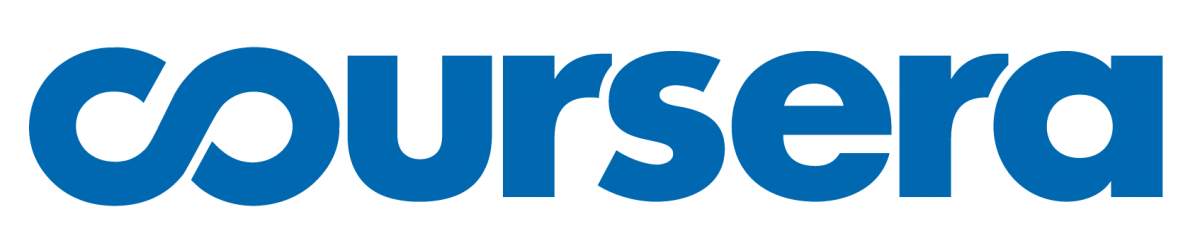
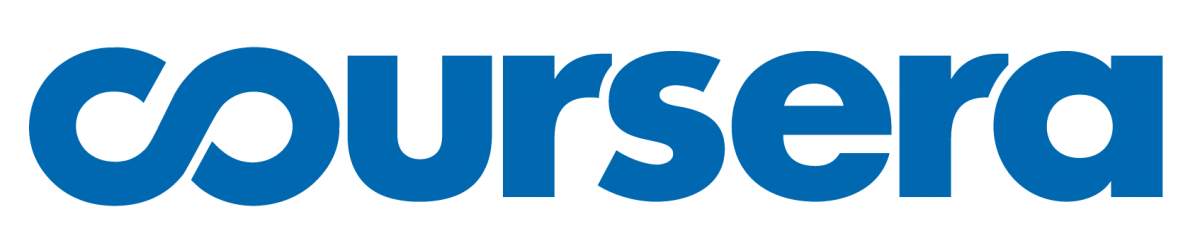
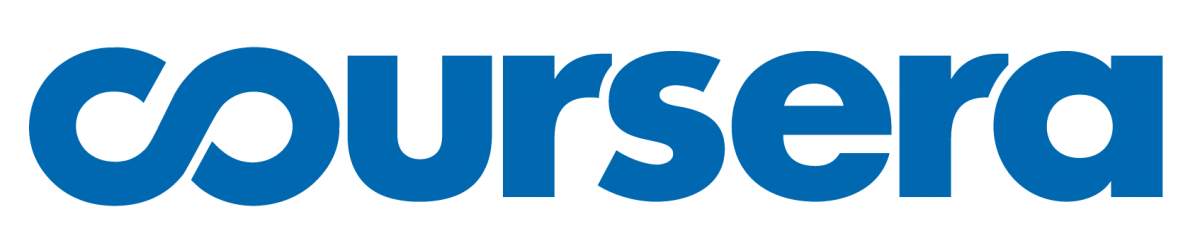
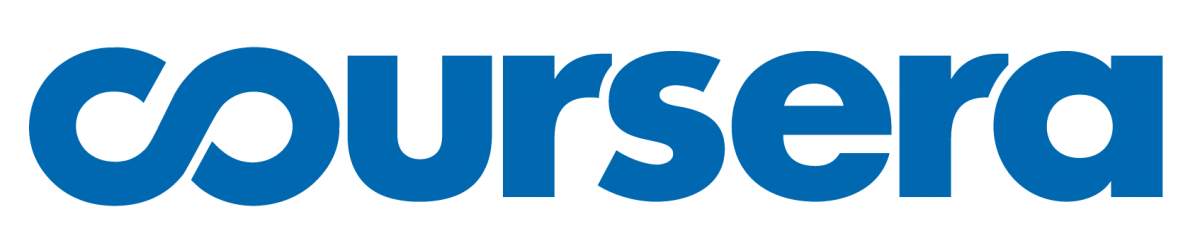
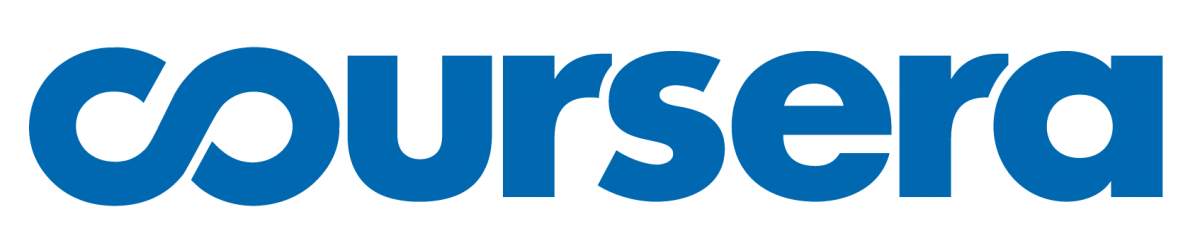
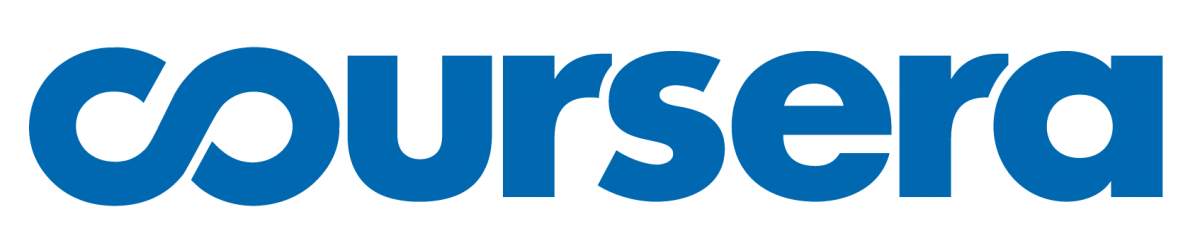
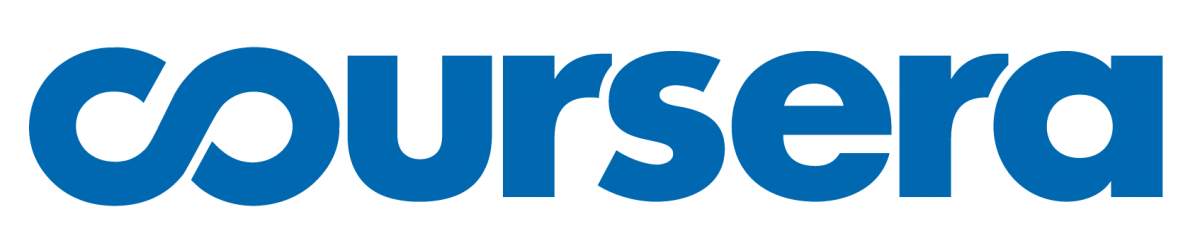
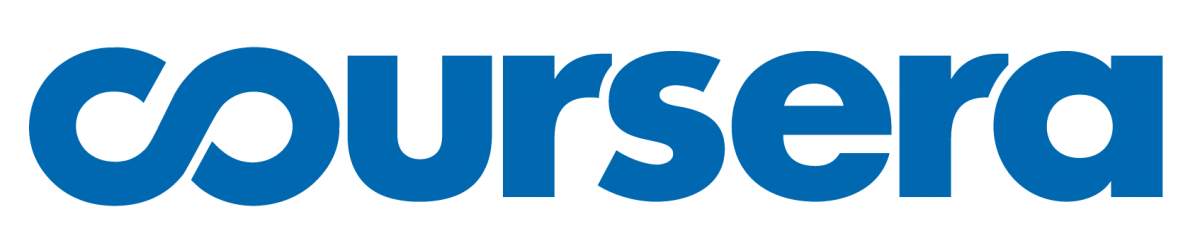
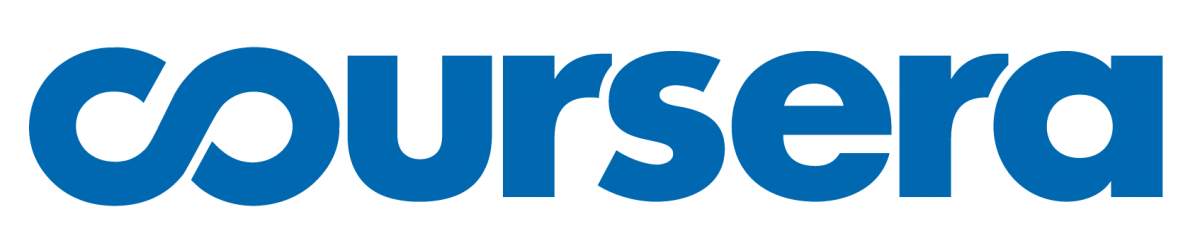
Description
Beginner-level specialization that covers basic data science tools, including data management and visualization, modeling, and machine learning. Students can choose from using either SAS or Python, including pandas and Scikit-learn to perform the exercises and projects in the courses. The specialization consists of 5 beginner courses in total.
Teachers:
- Wesleyan University: Lisa Dierker, Professor Psychology
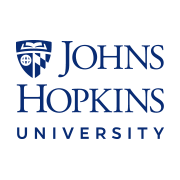
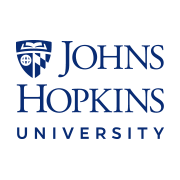
Mastering Software Development in R
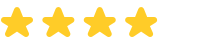
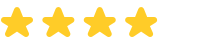
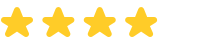
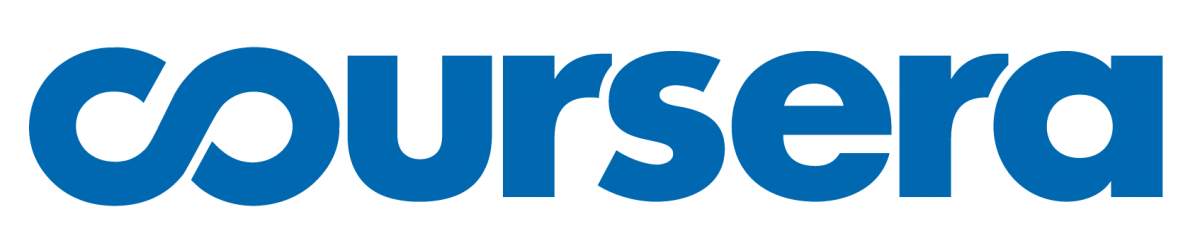
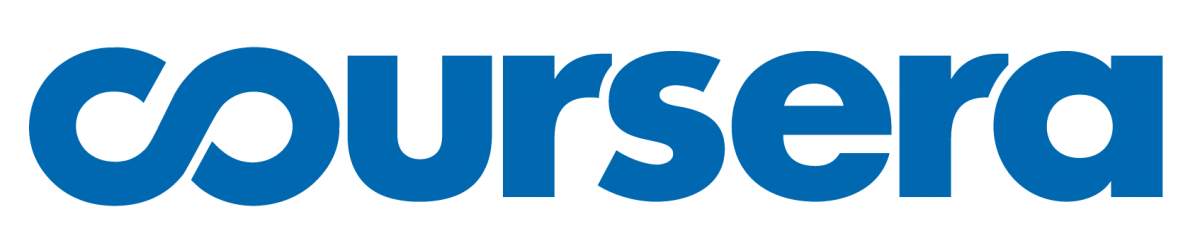
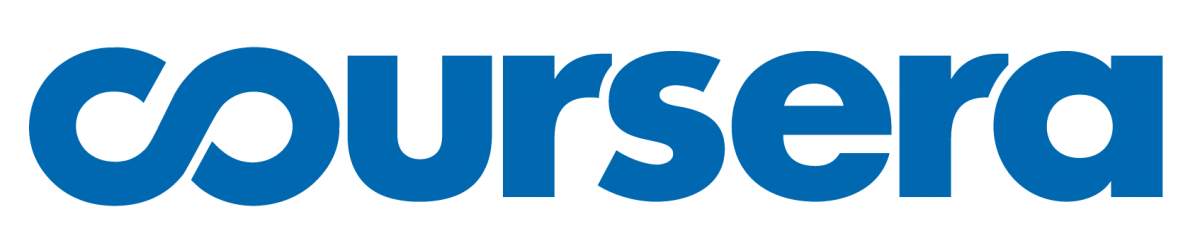
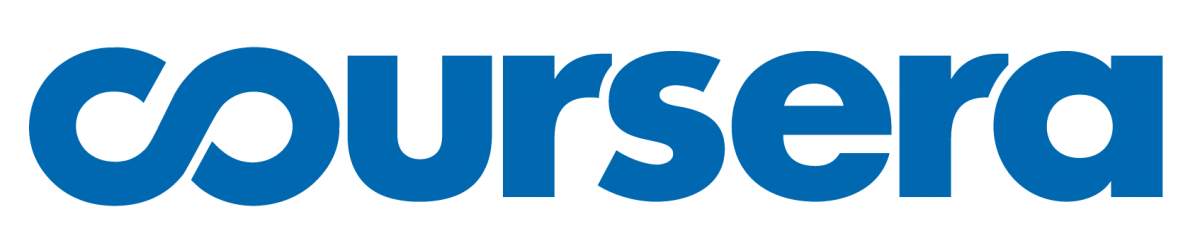
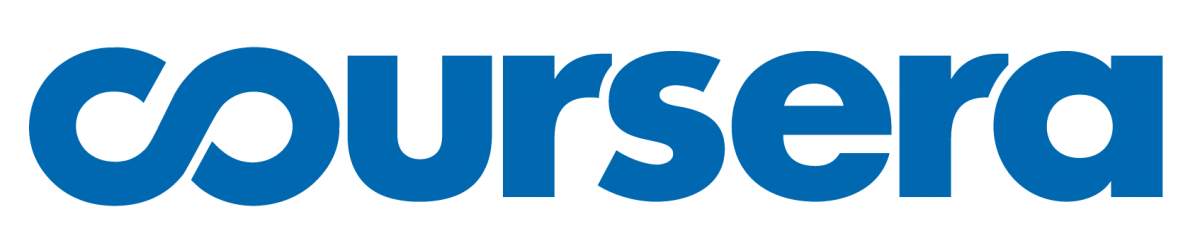
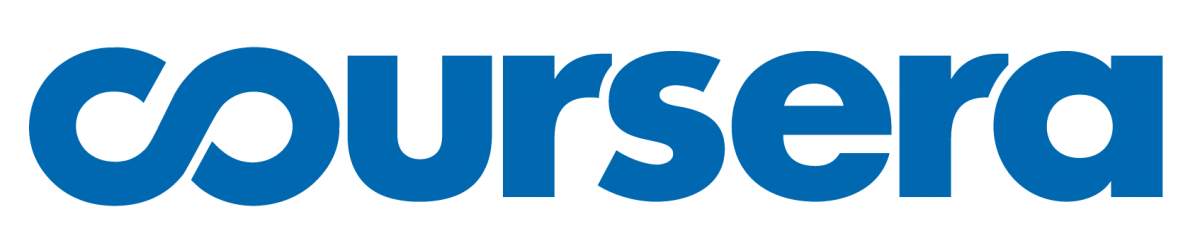
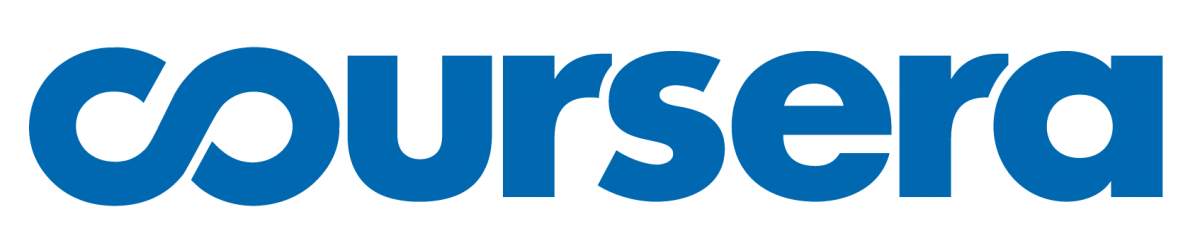
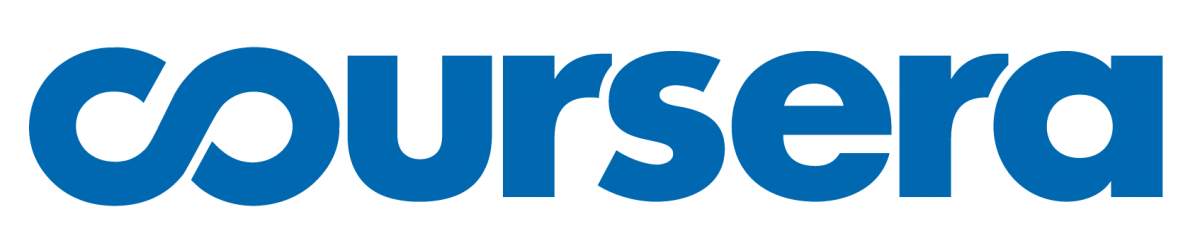
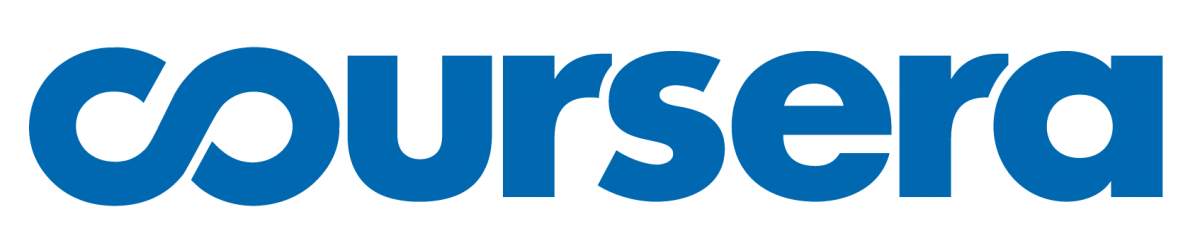
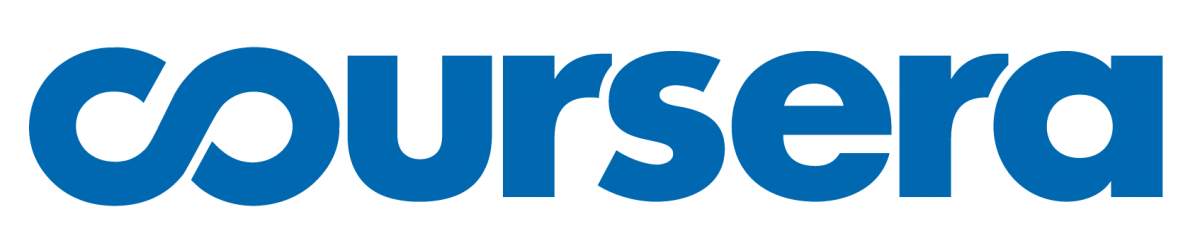
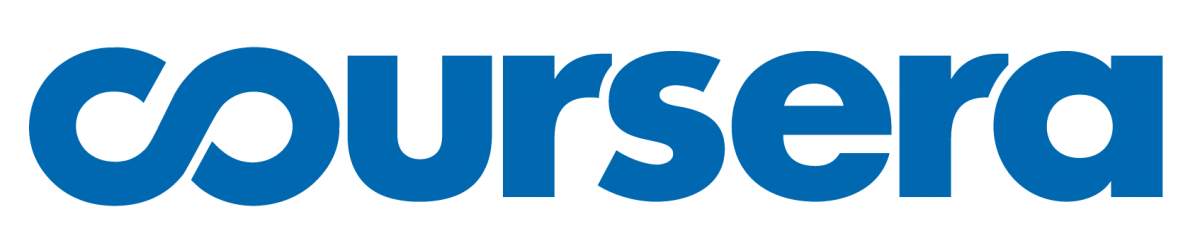
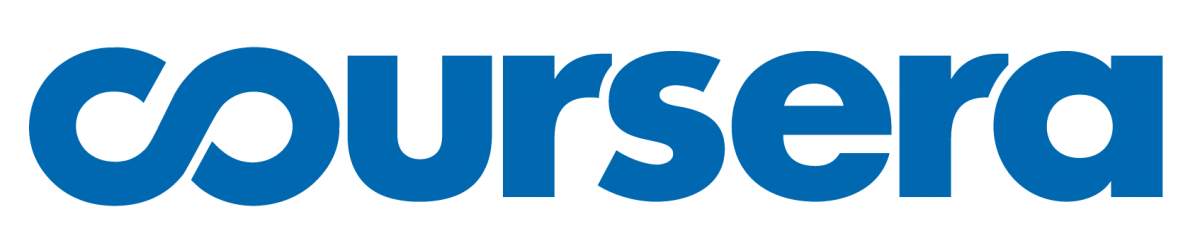
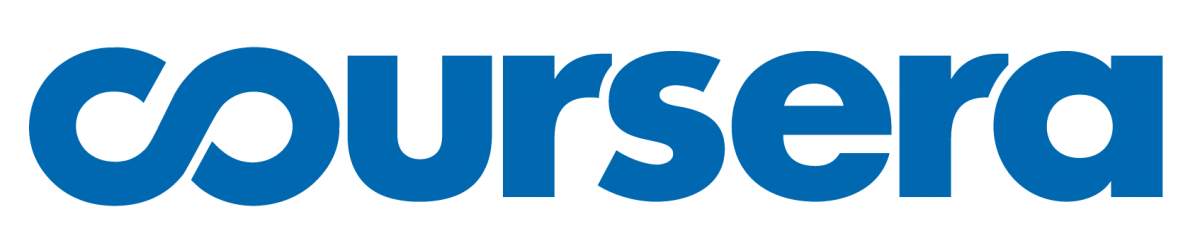
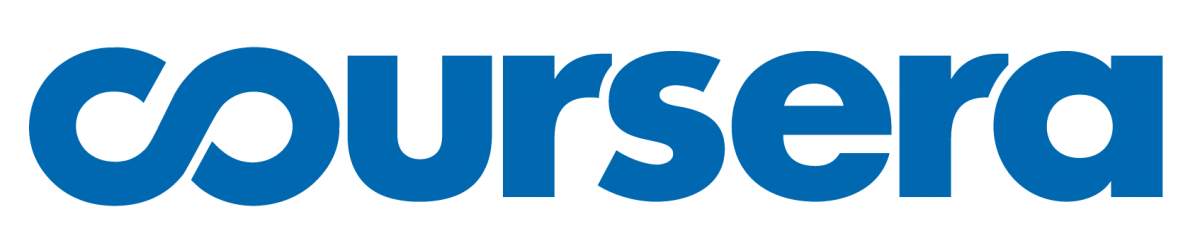
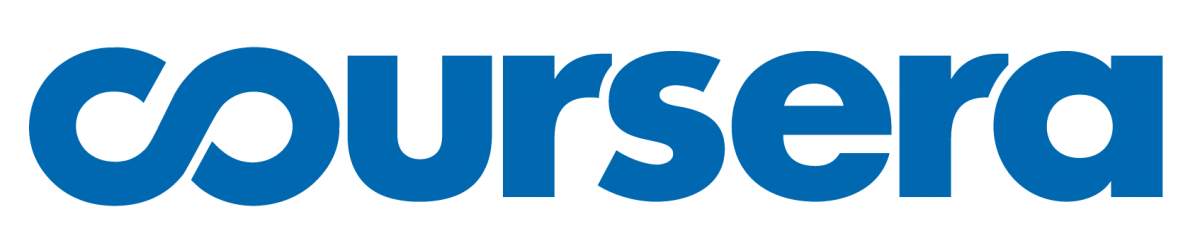
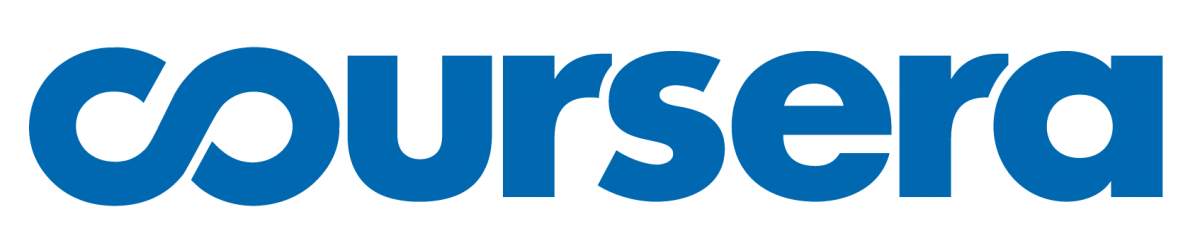
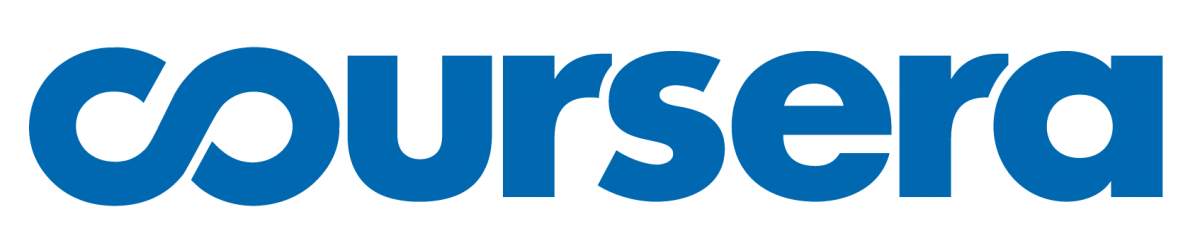
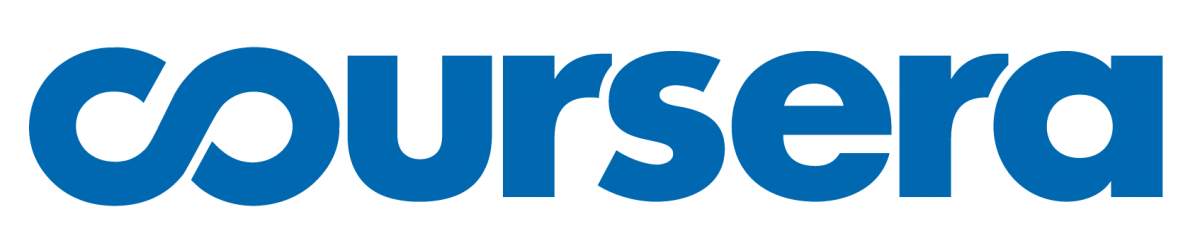
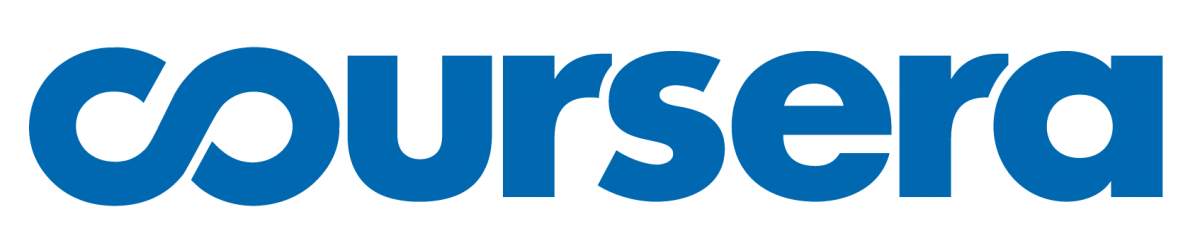
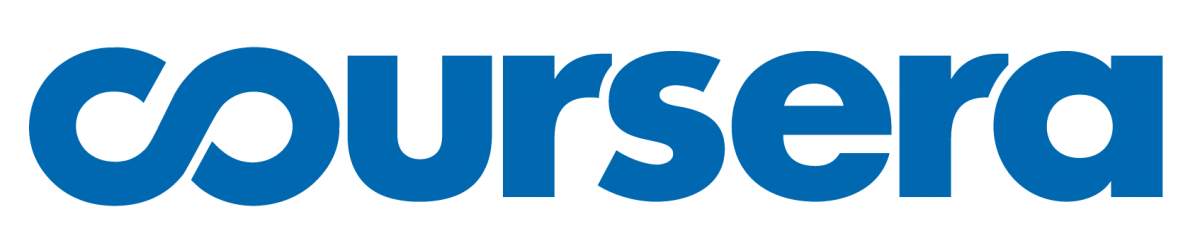
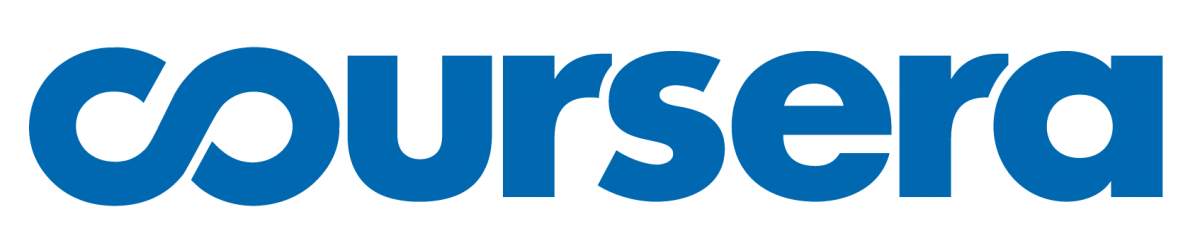
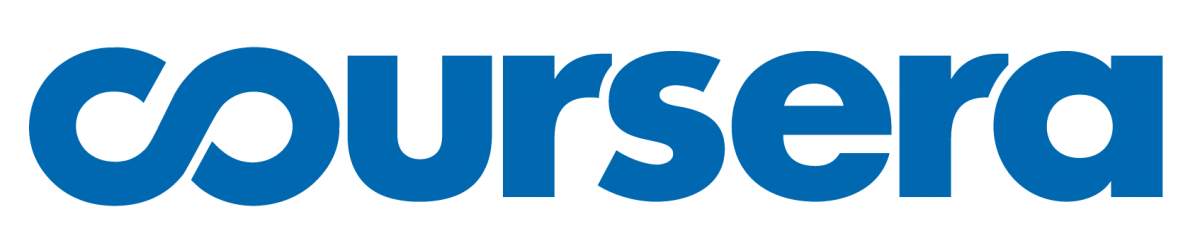
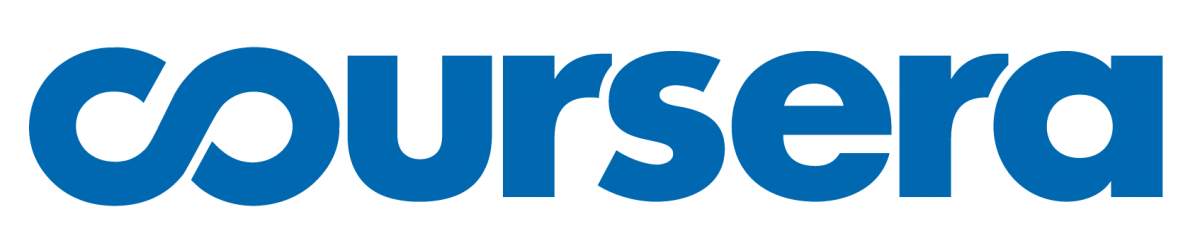
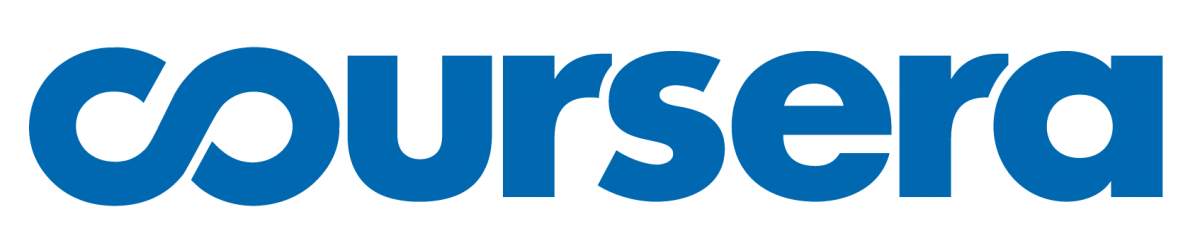
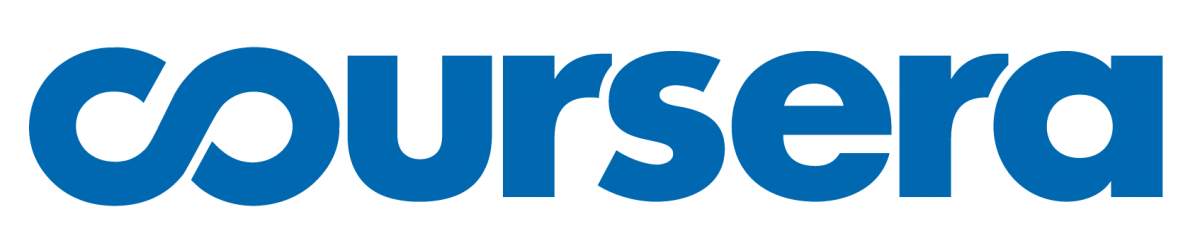
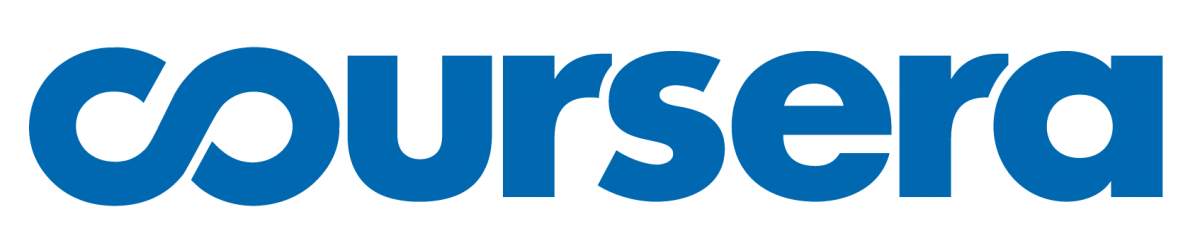
Description
This Coursera specialization offers students to get rigorous training in programming with the R programming language for statistical analysis. The courses that are part of this specialization will enable the student learn about: handling complex data in R, building R packages, create custom visualizations and finally produce and scale data science products
Teachers:
- Johns Hopkins University: Roger D. Peng, PhD Associate Professor, Biostatistics
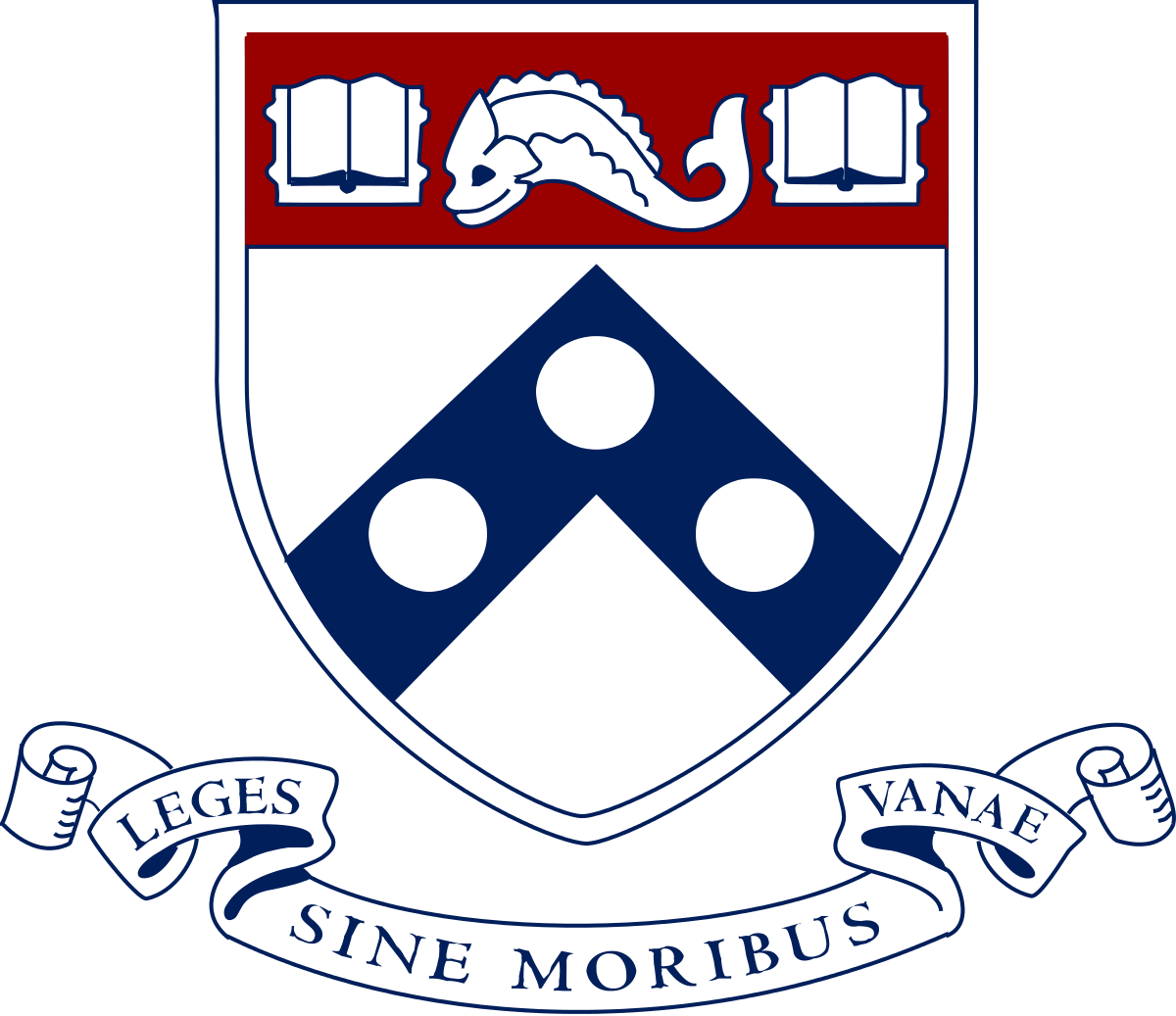
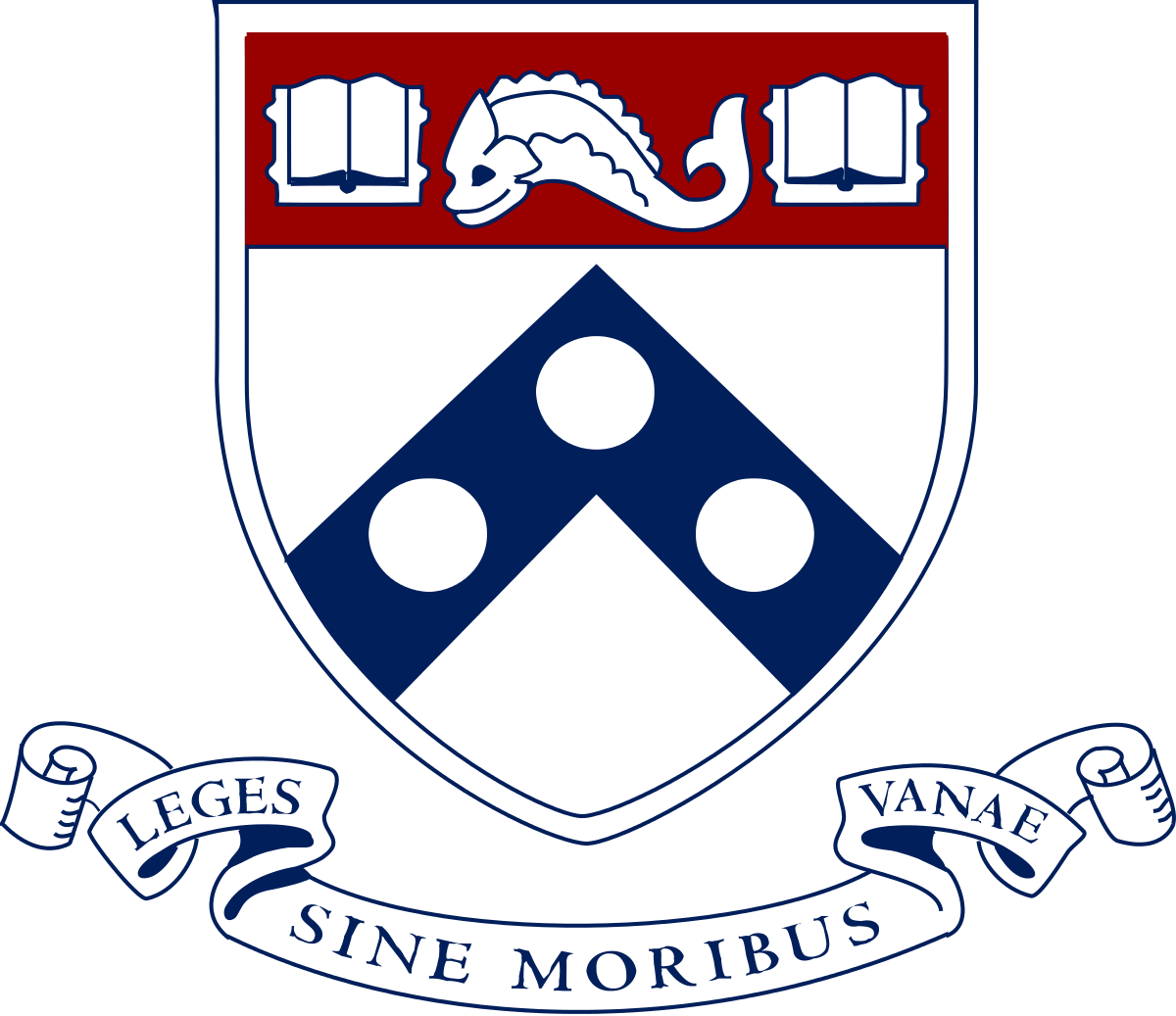
Business Analytics
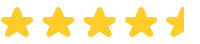
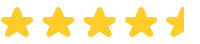
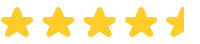
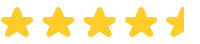
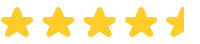
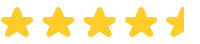
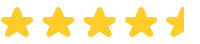
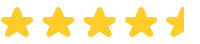
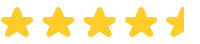
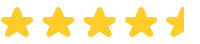
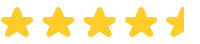
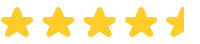
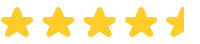
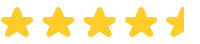
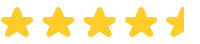
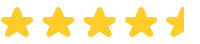
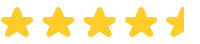
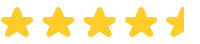
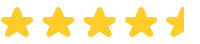
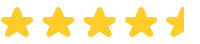
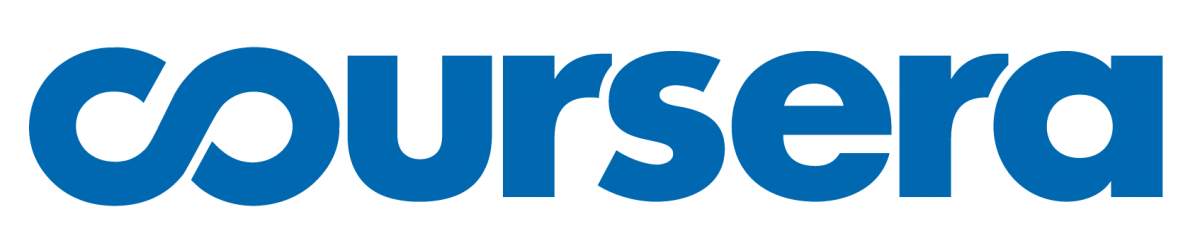
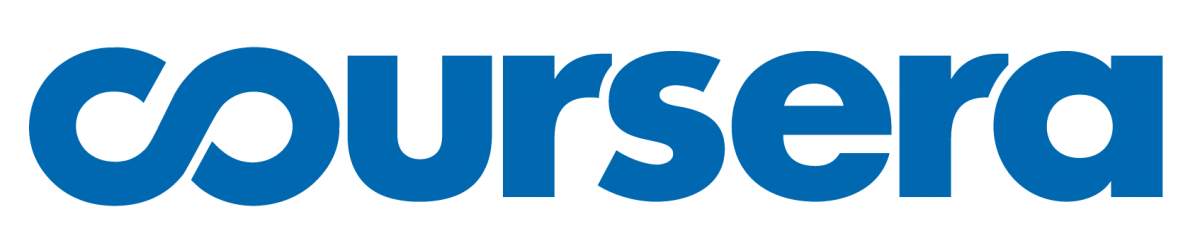
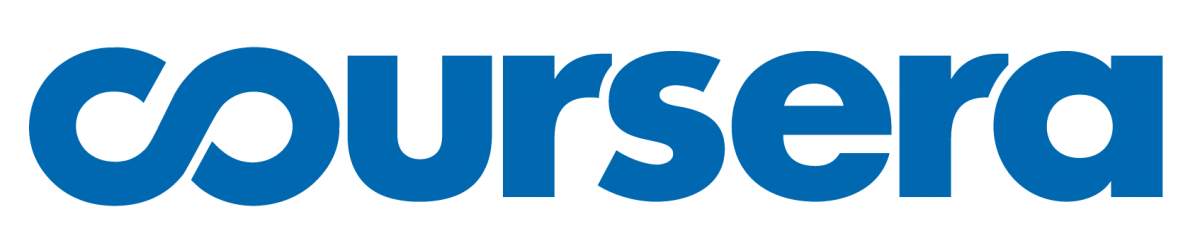
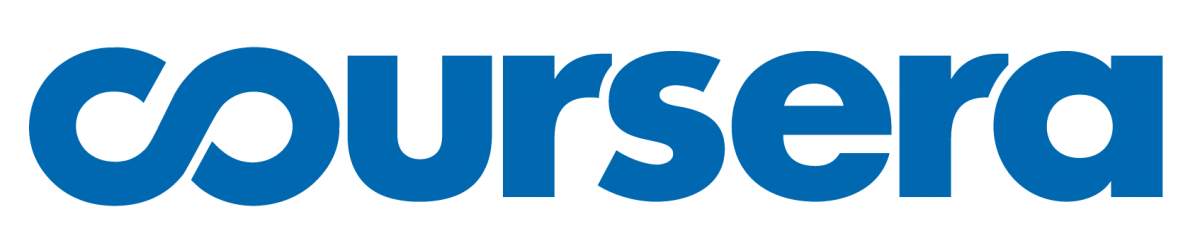
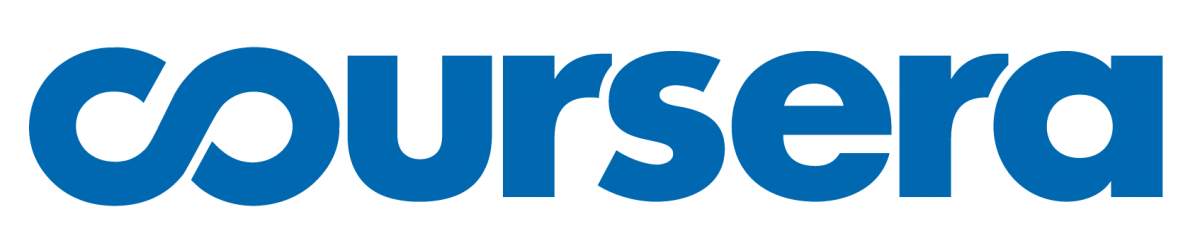
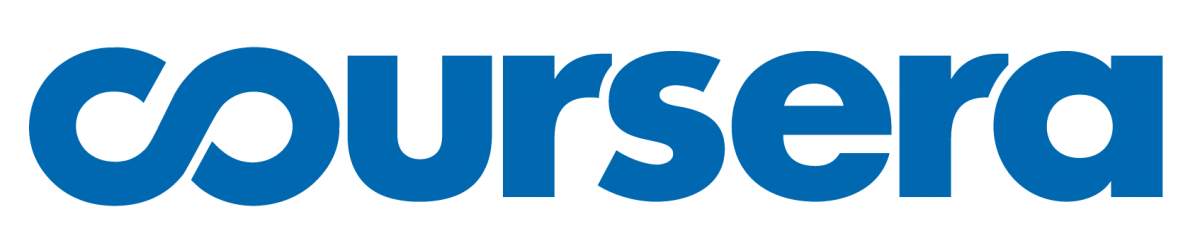
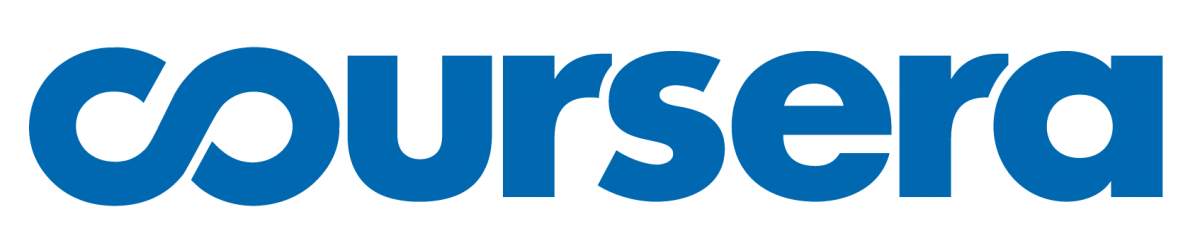
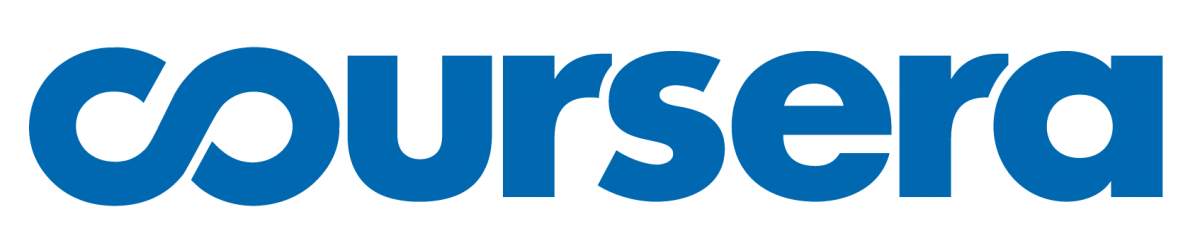
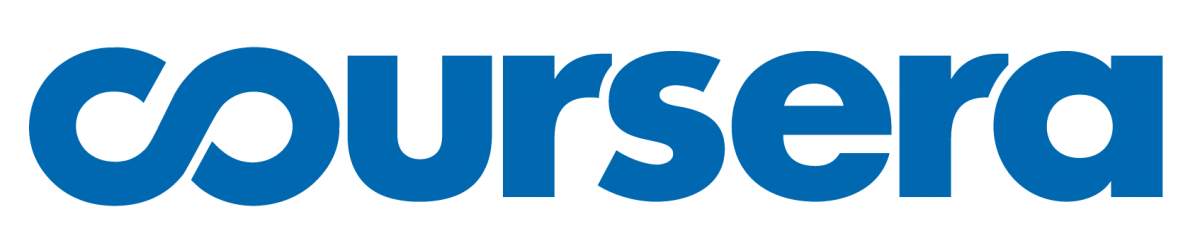
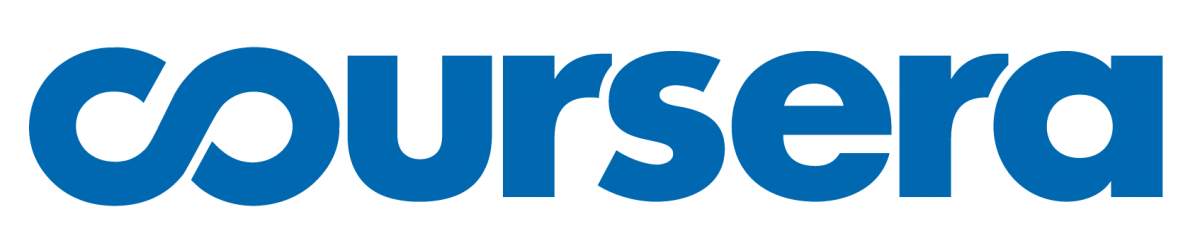
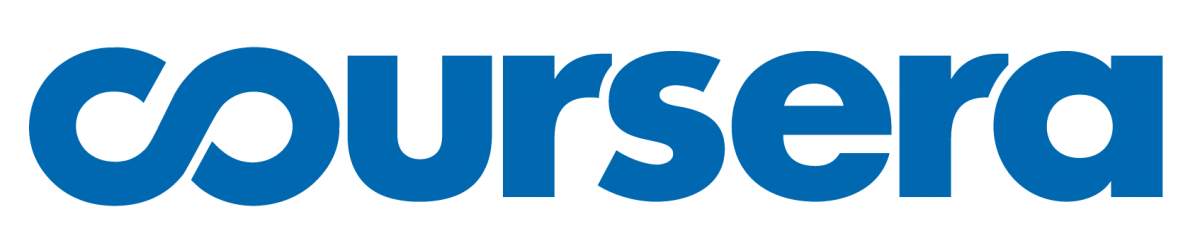
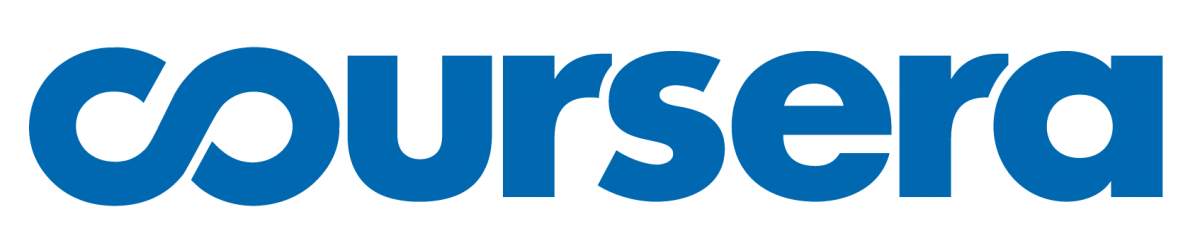
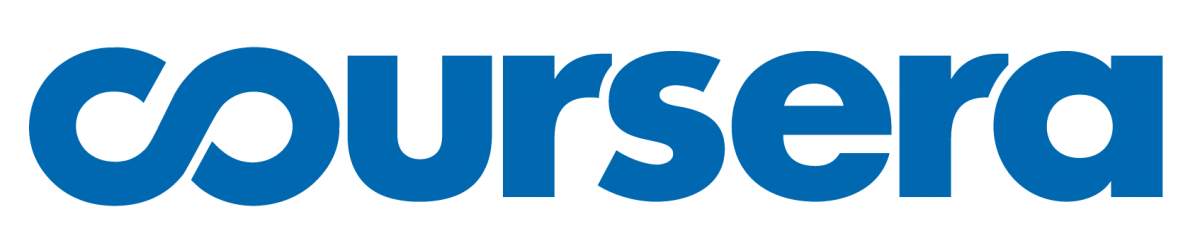
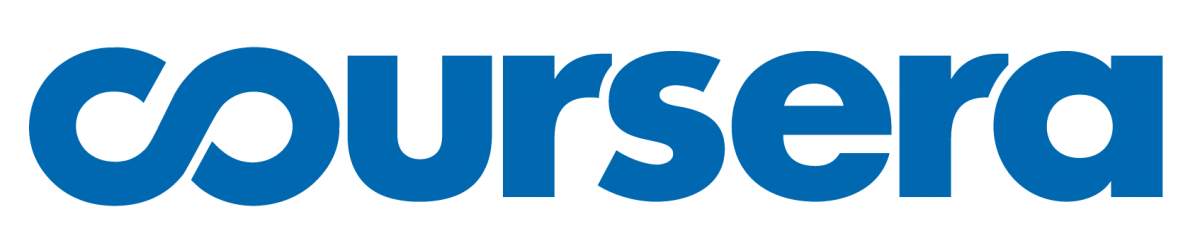
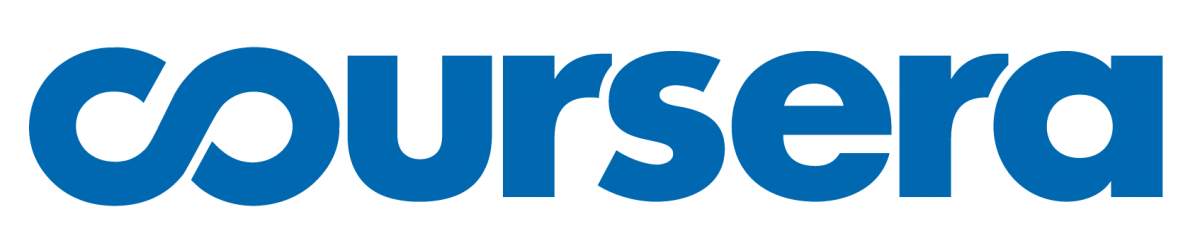
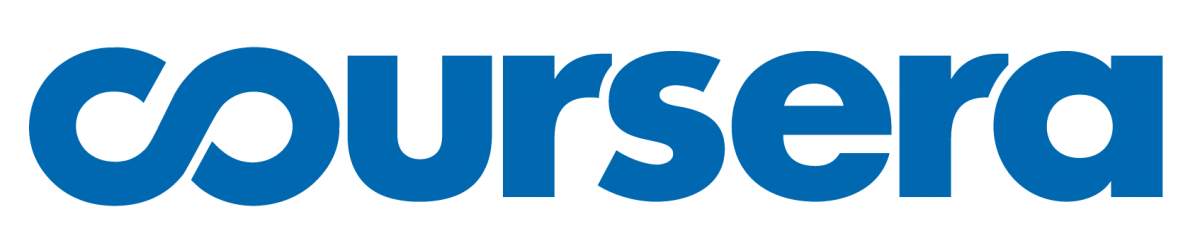
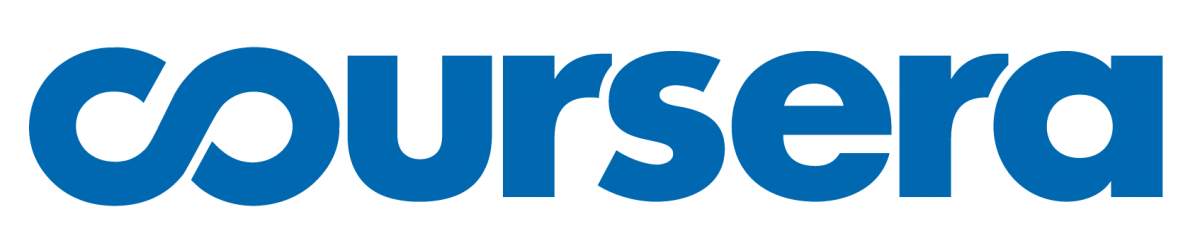
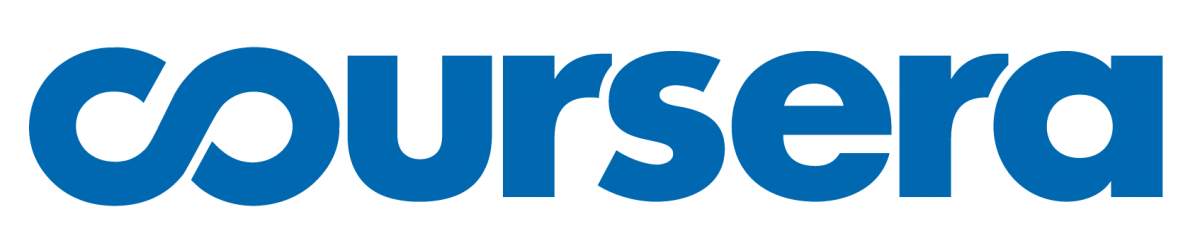
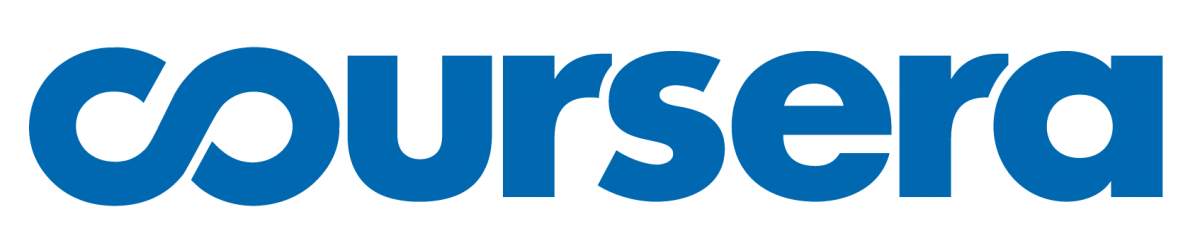
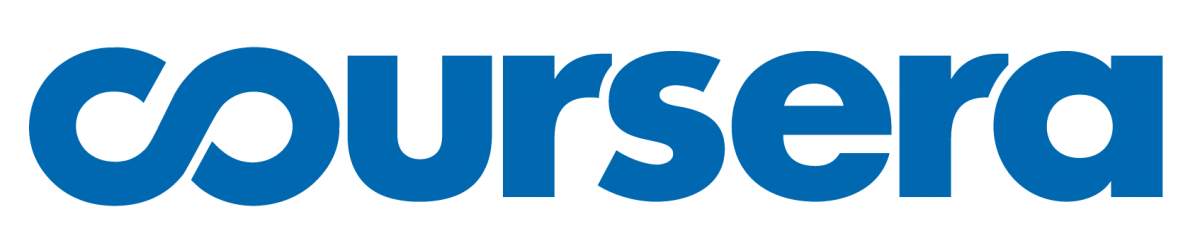
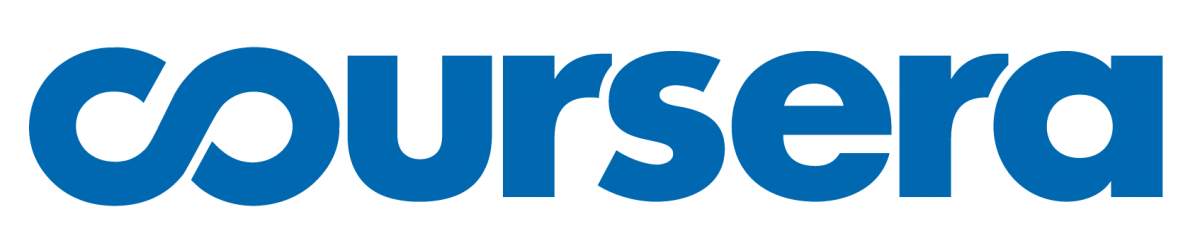
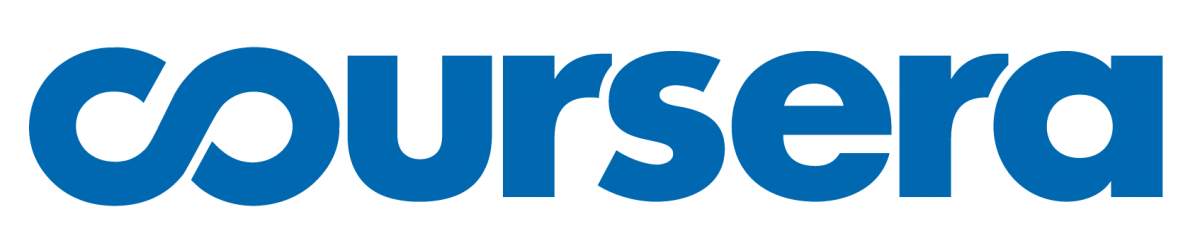
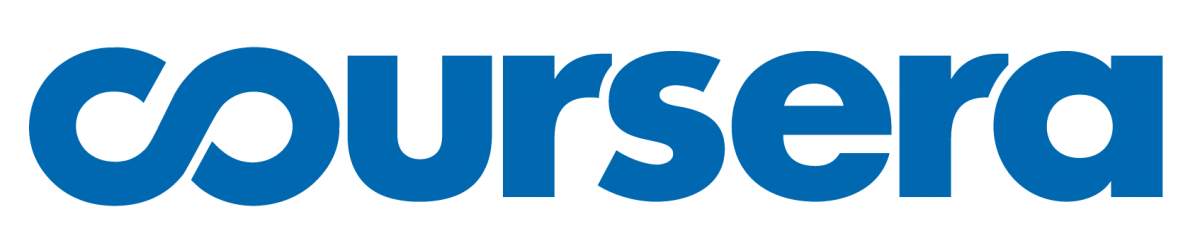
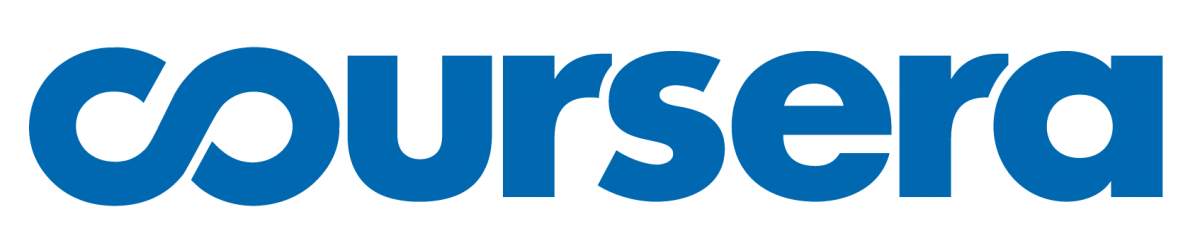
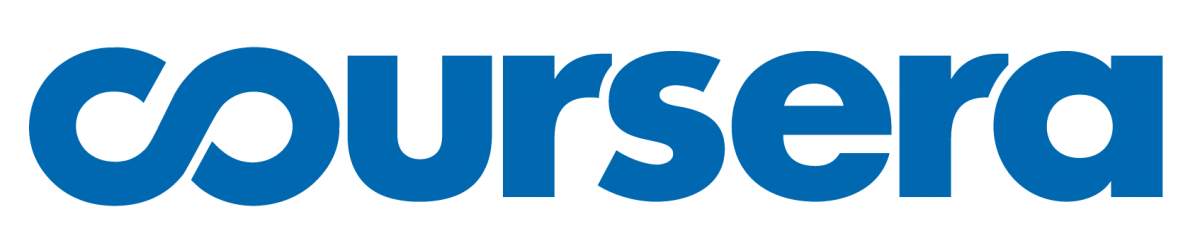
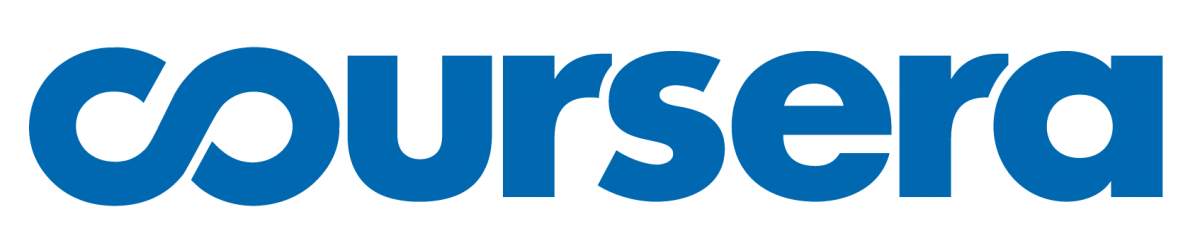
Description
Data-driven decision making specialization. Students will learn how data analysts describe, predict, and inform business decisions in the specific areas of marketing, human resources, finance, and operations. This specialization will enable students to learn how to solve business problems using data-driven approach.
Teachers:
- University of Pennsylvania: Ron Berman Assistant Professor of Marketing
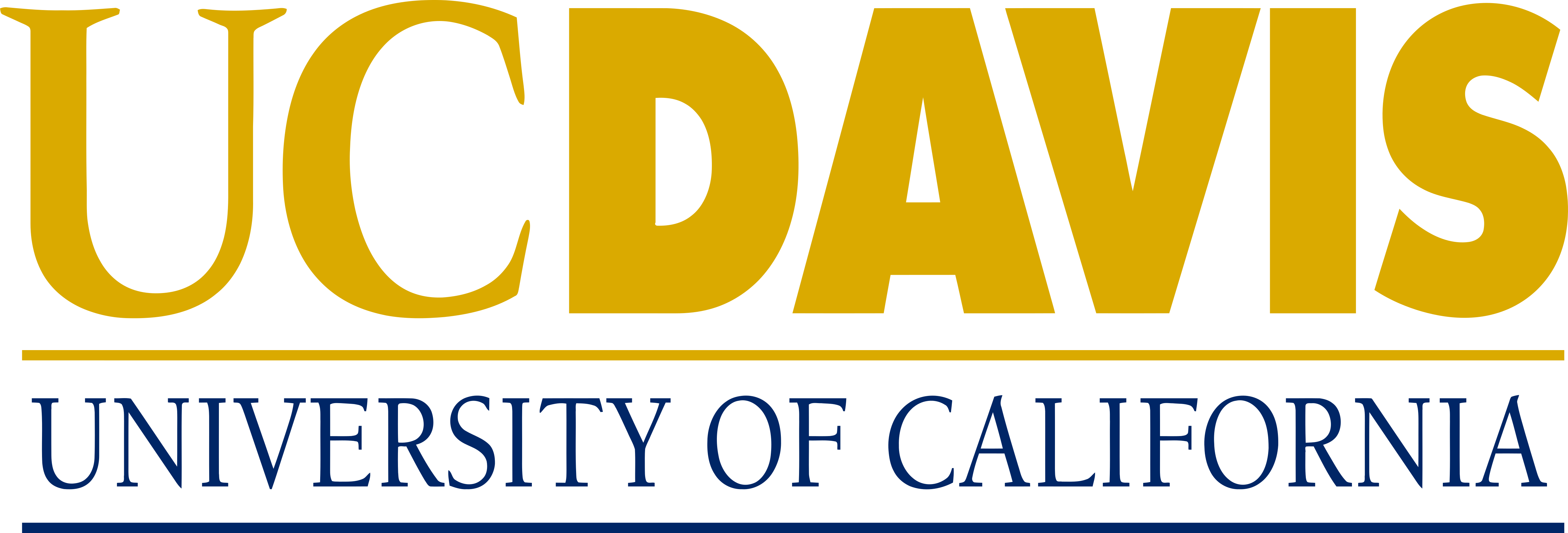
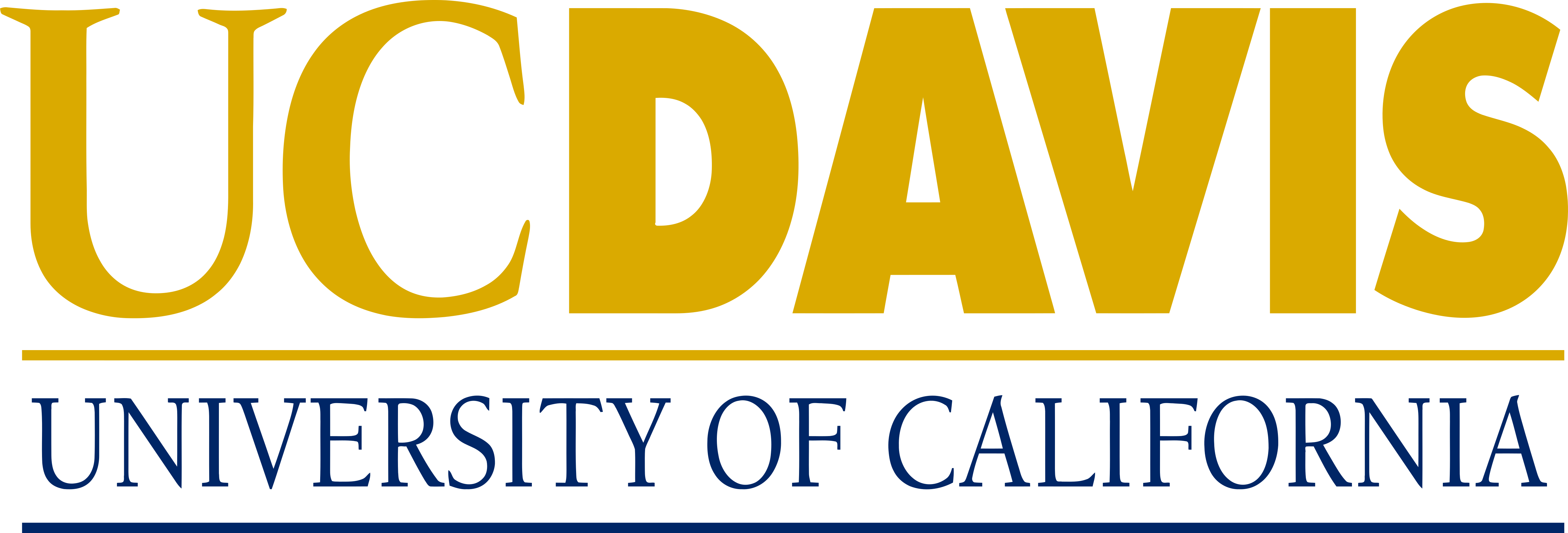
Data Visualization with Tableau
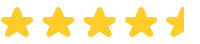
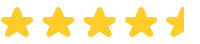
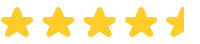
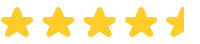
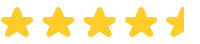
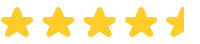
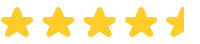
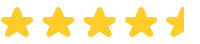
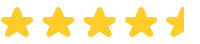
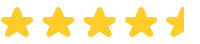
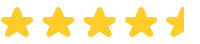
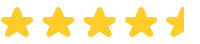
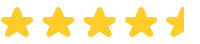
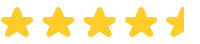
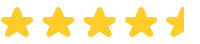
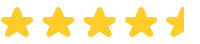
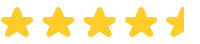
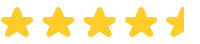
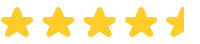
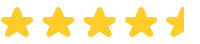
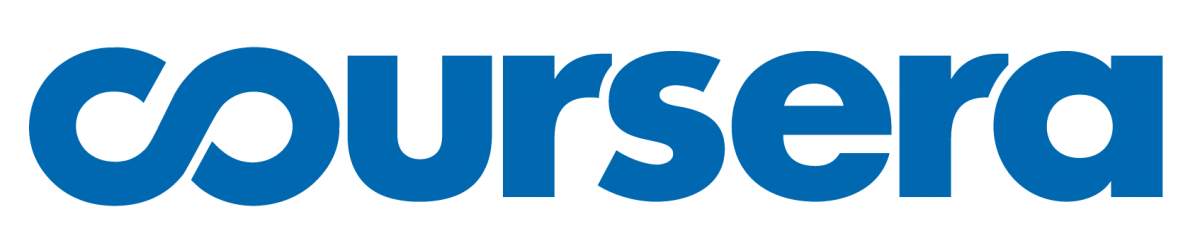
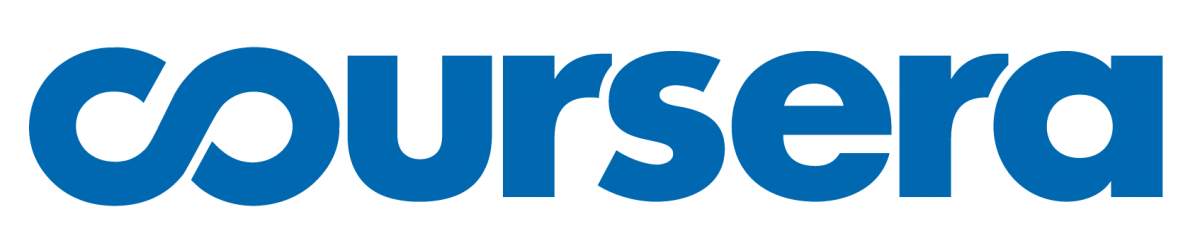
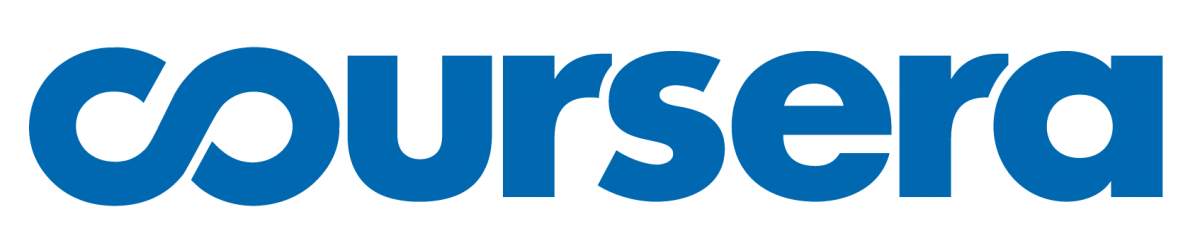
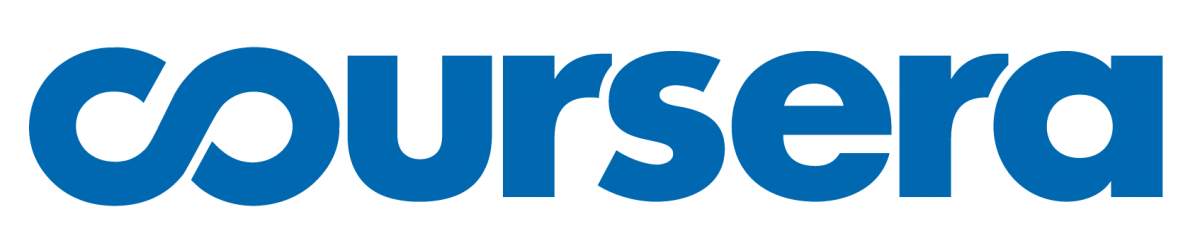
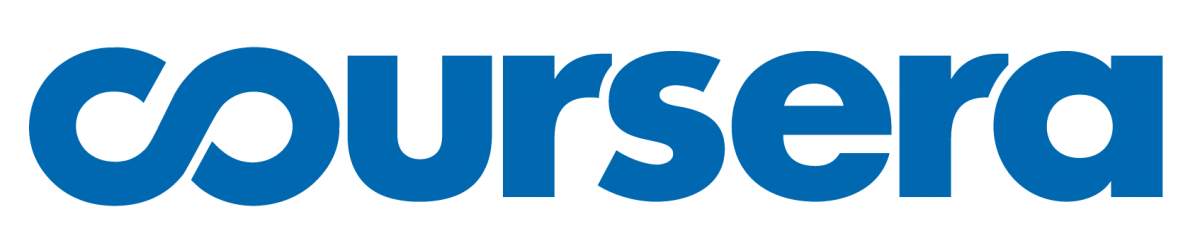
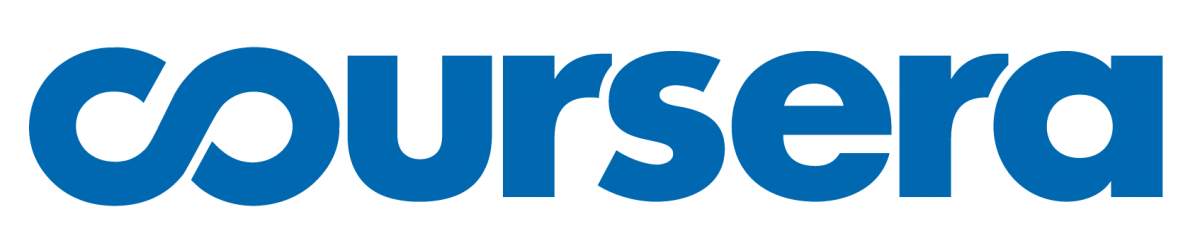
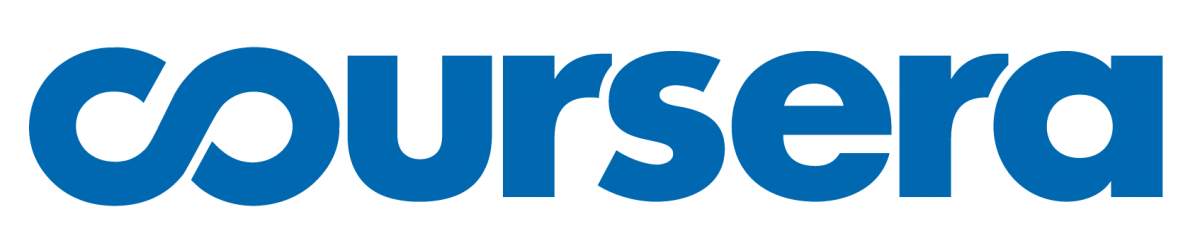
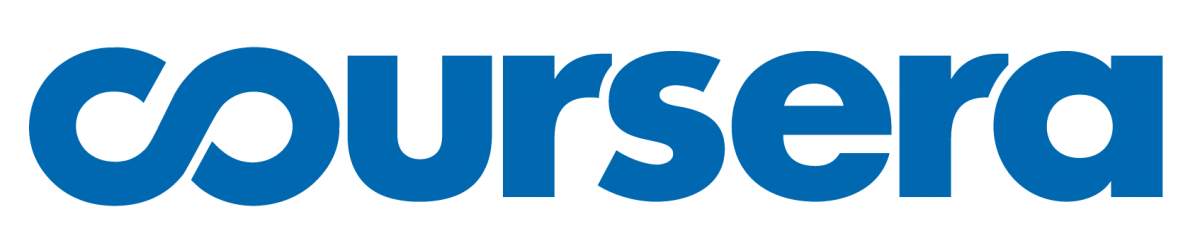
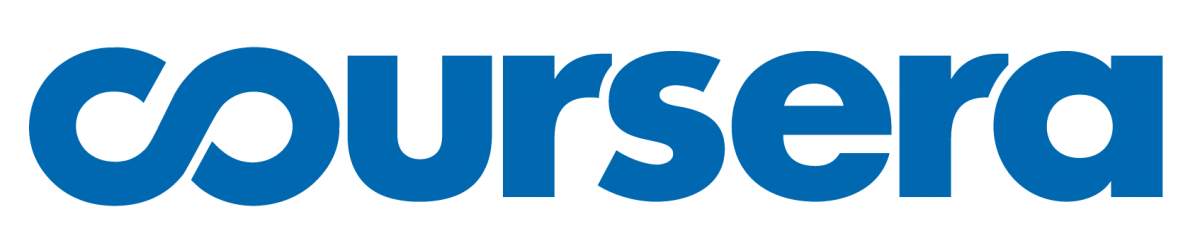
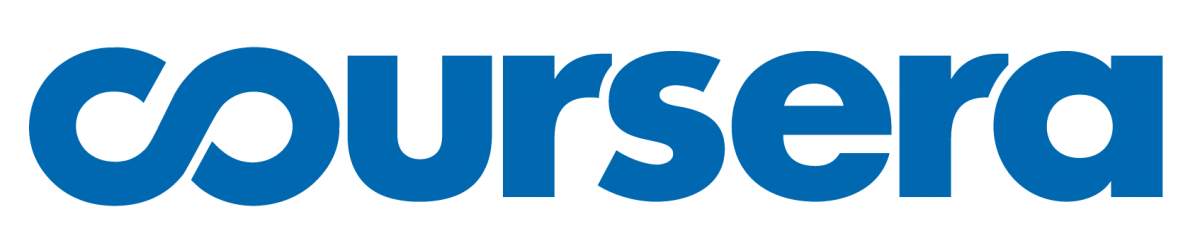
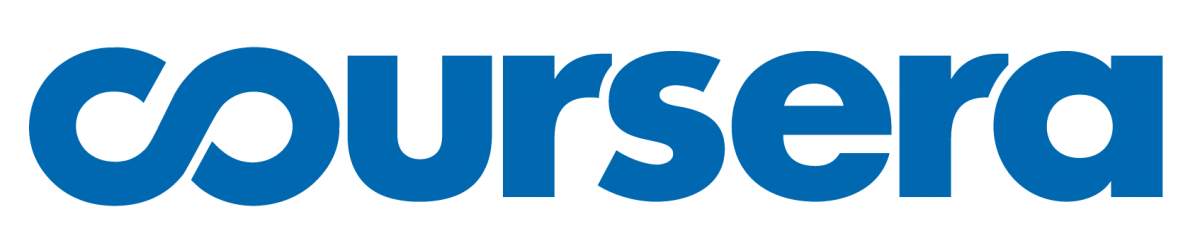
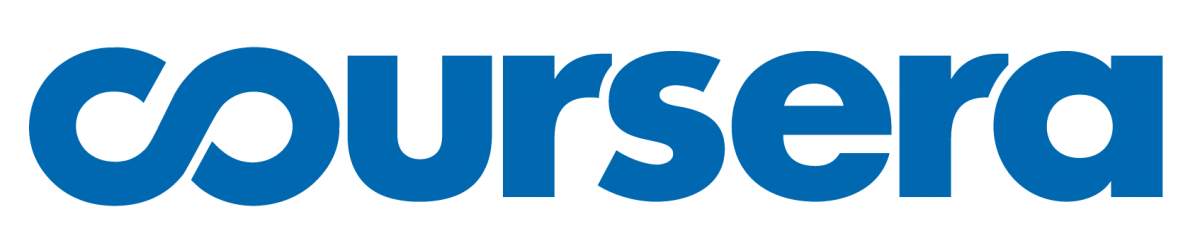
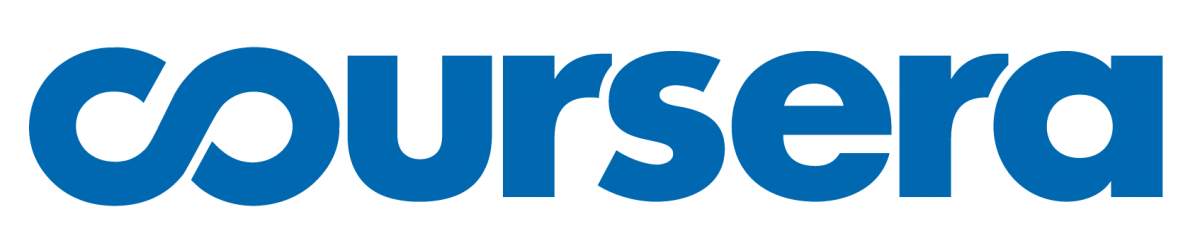
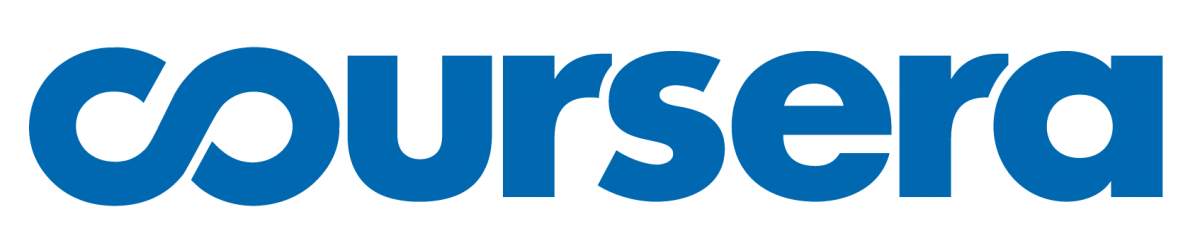
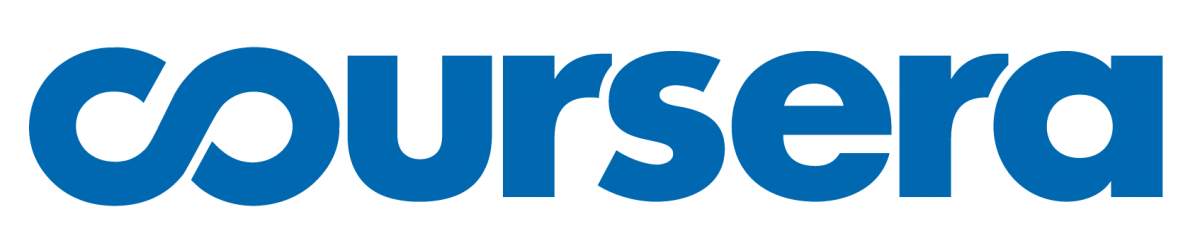
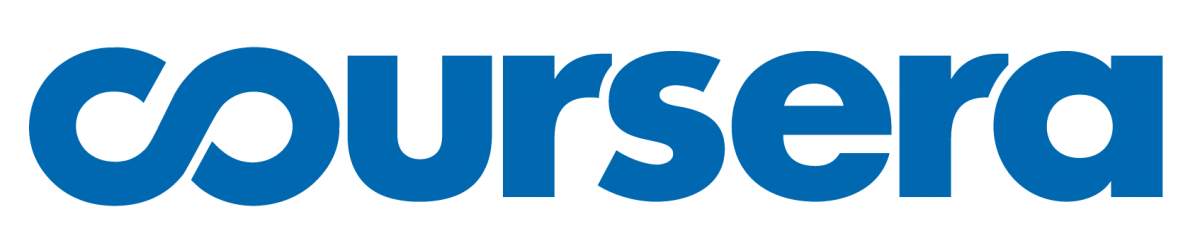
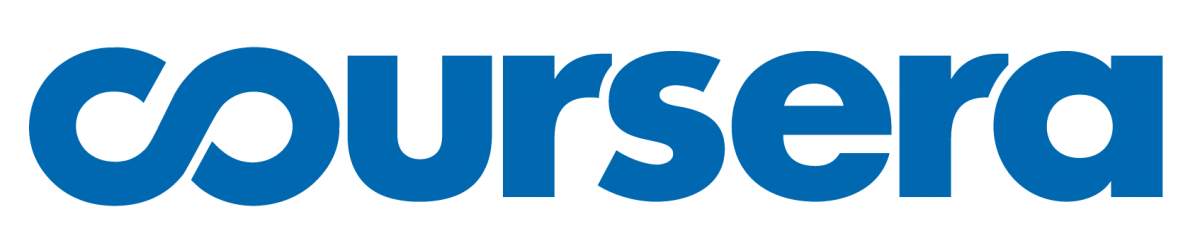
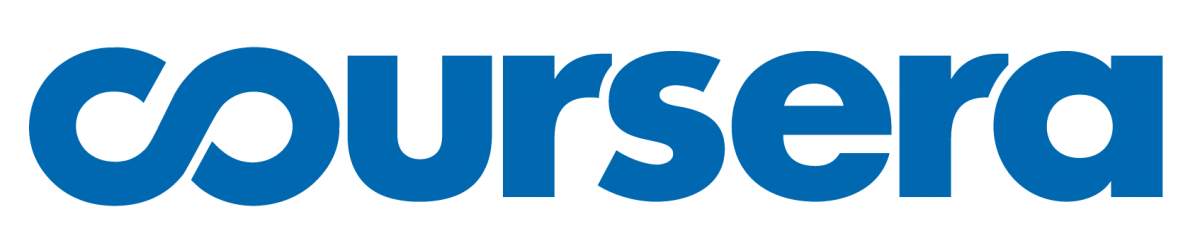
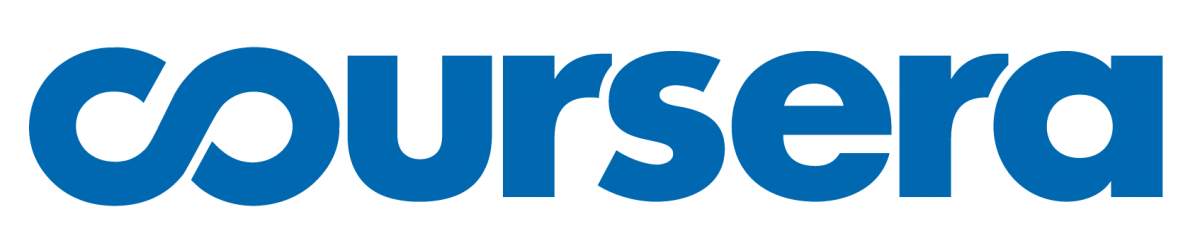
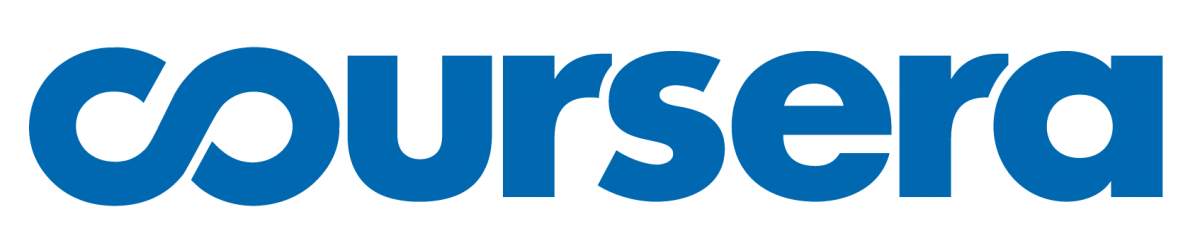
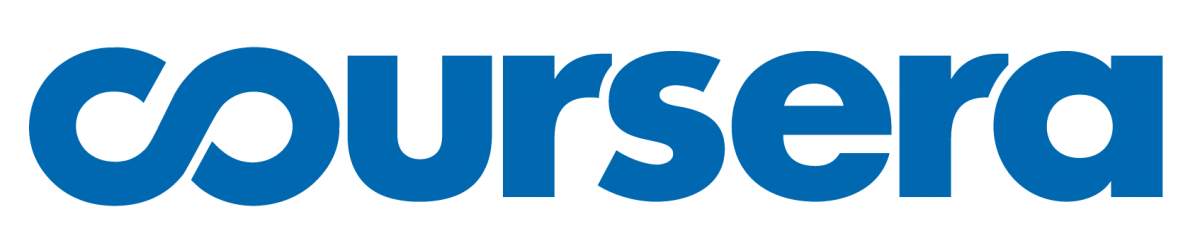
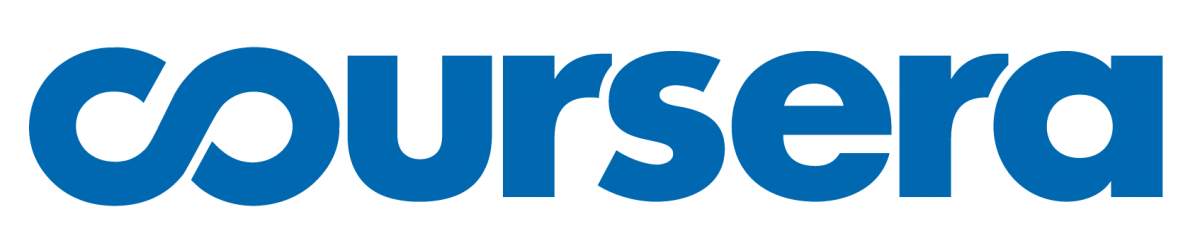
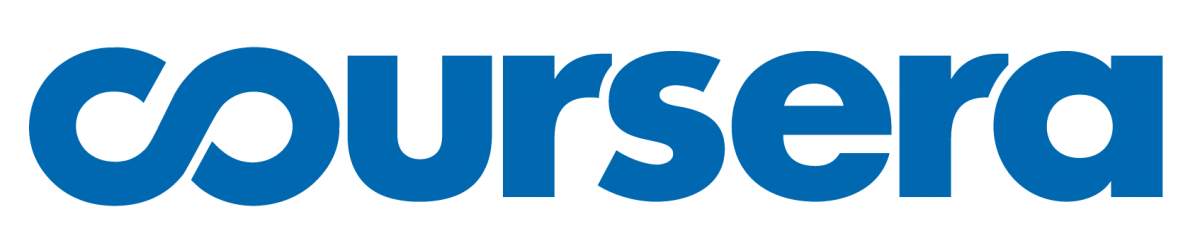
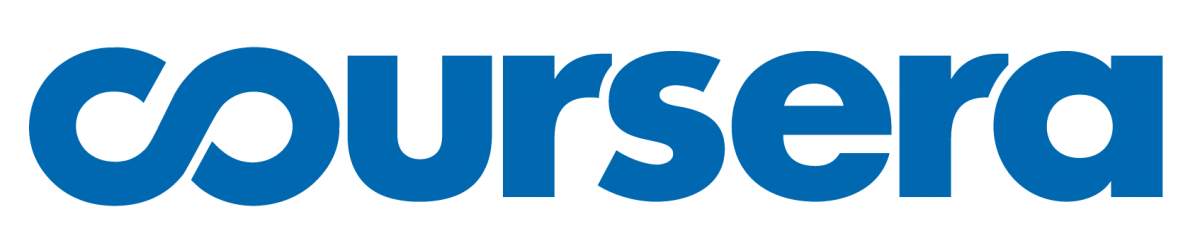
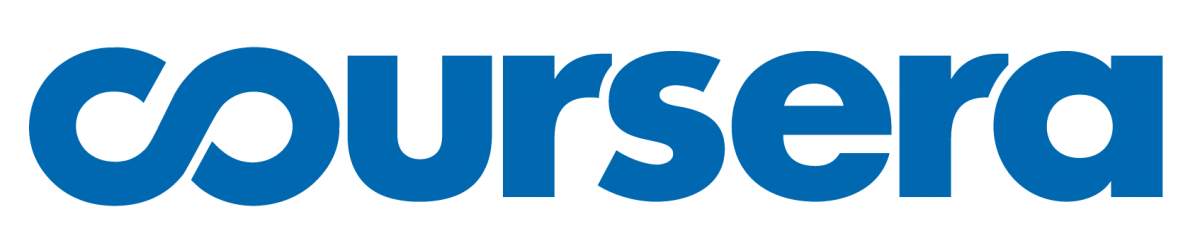
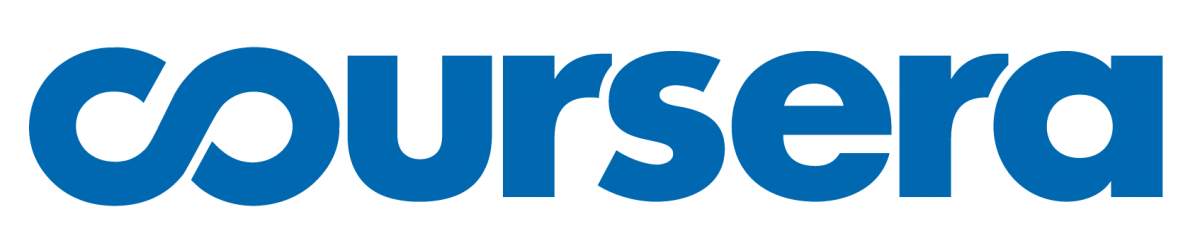
Description
This 5-course specialization offers students to learn how to use Tableau, perform exploratory data analysis and create visualizations and dashboards in an intuitive way. The specialization is offered in collaboration with Tableau, for newcomers to data visualization with no prior experience using Tableau.
Teachers:
- UC Davis: Suk S. Brar, M.B.A.
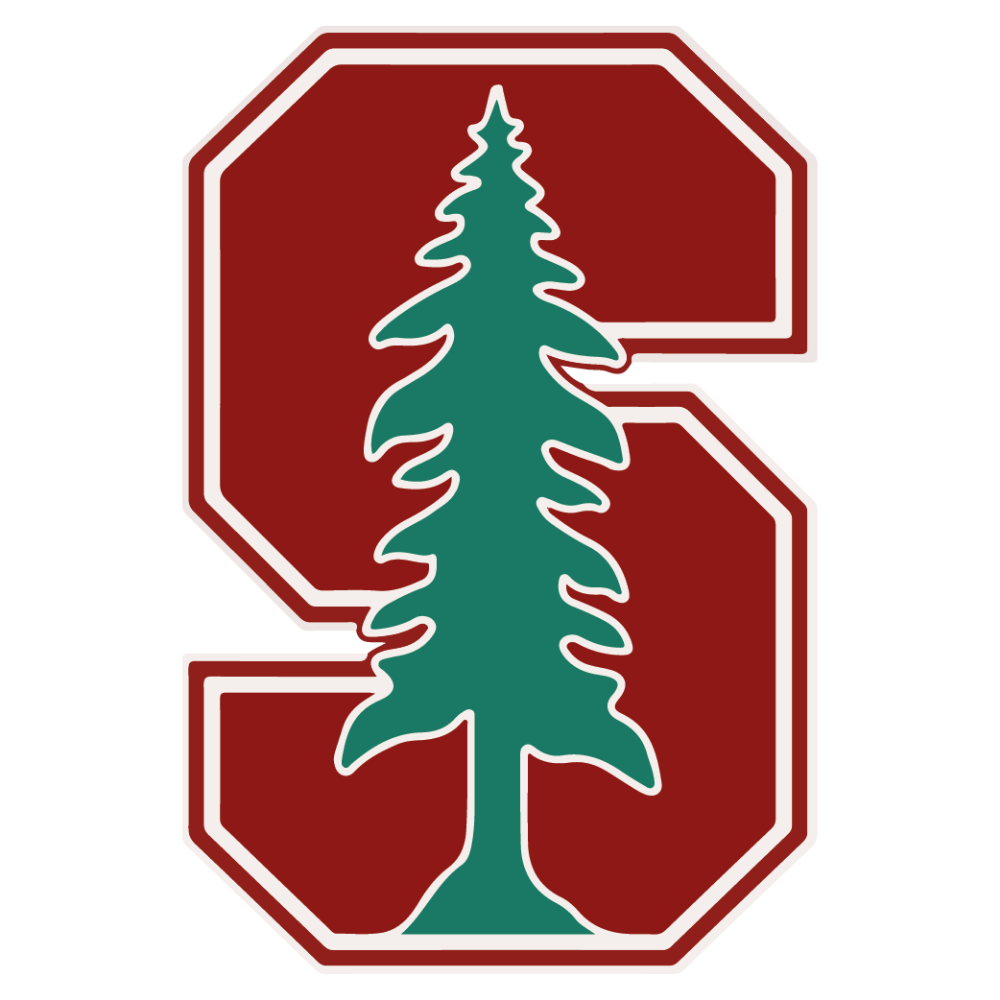
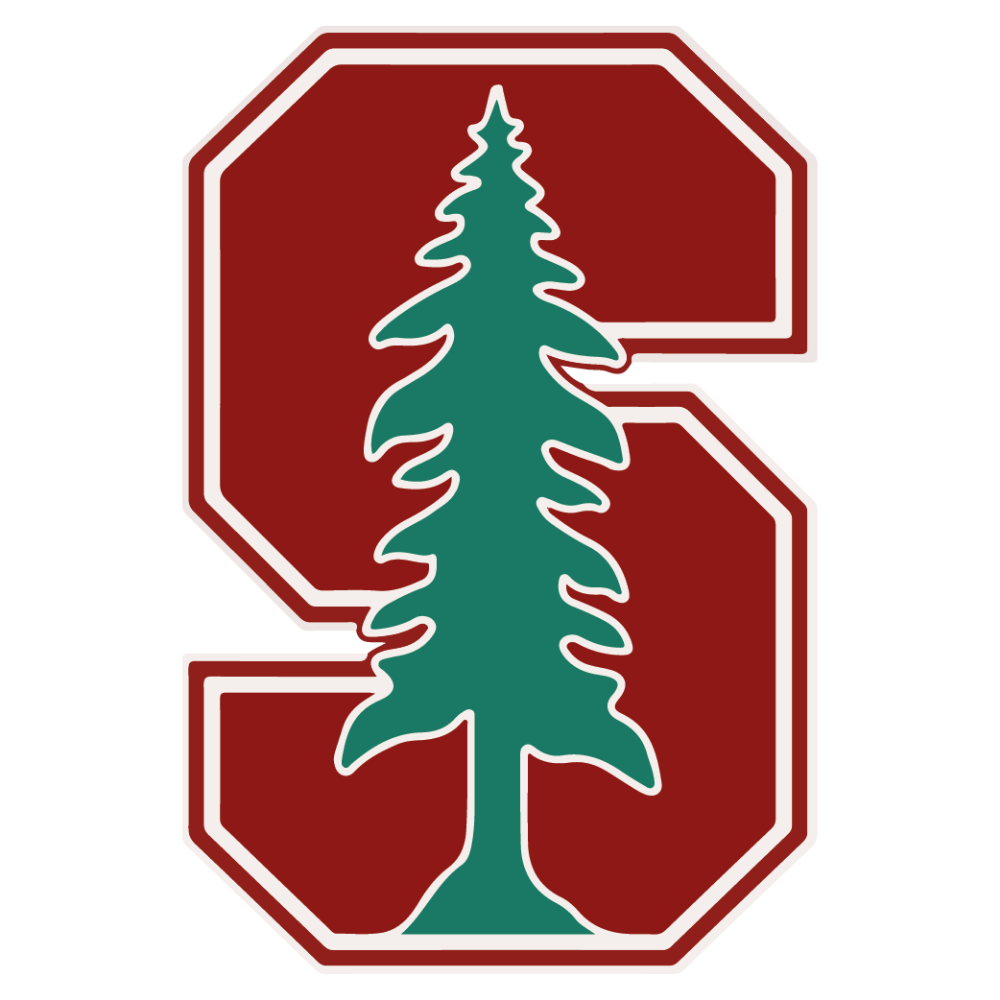
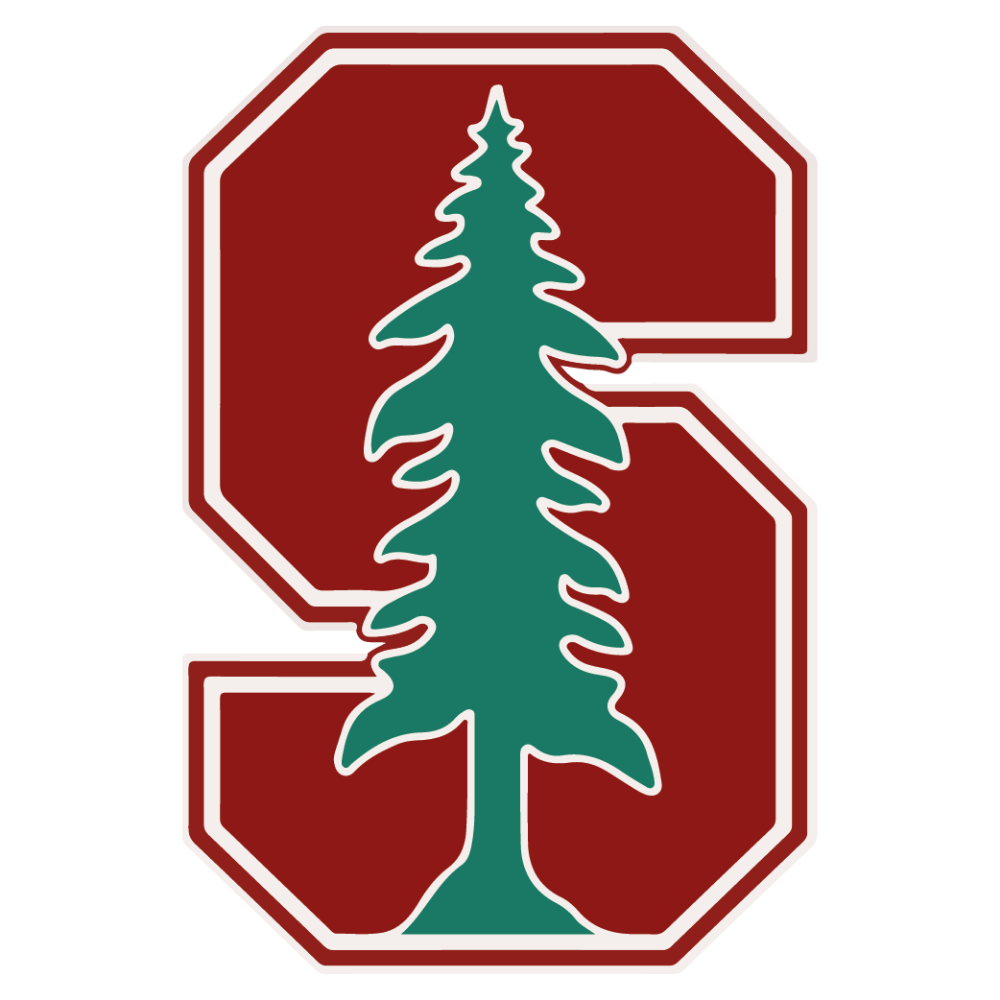
Machine Learning
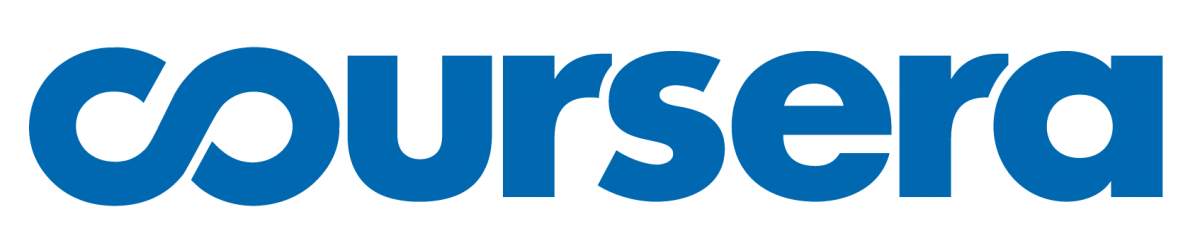
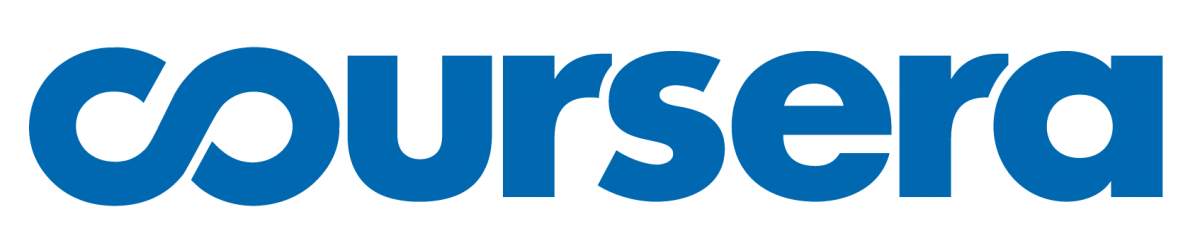
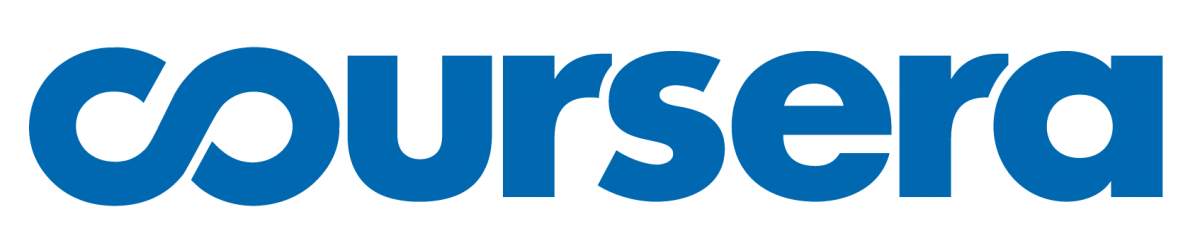
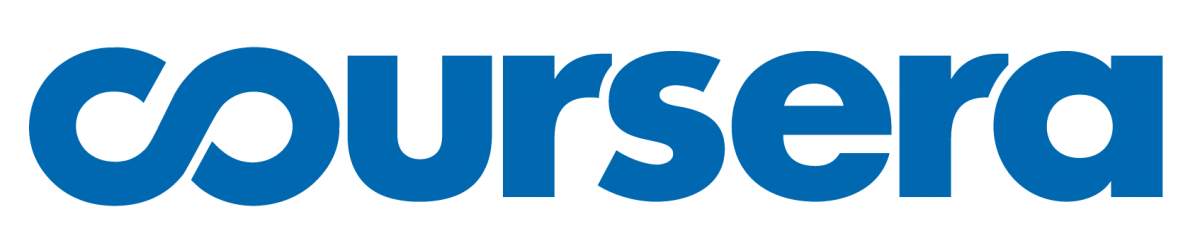
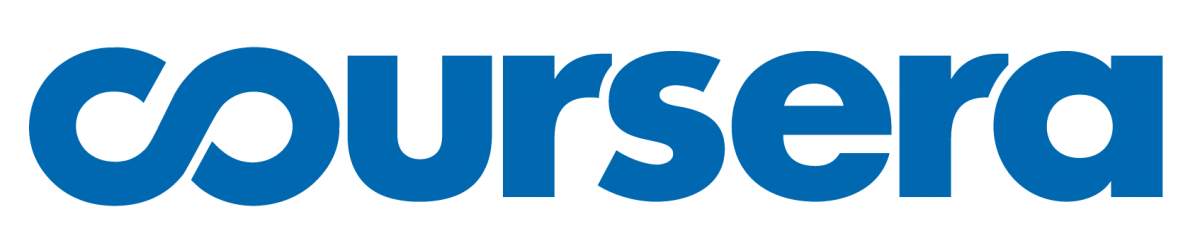
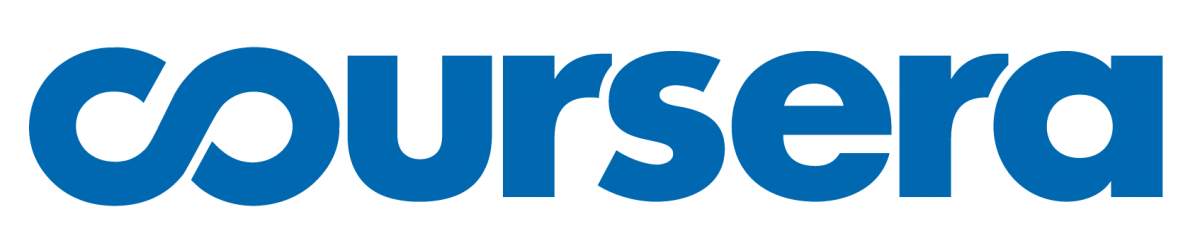
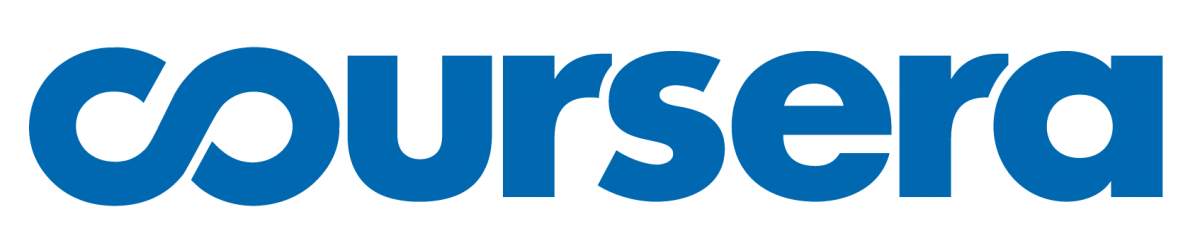
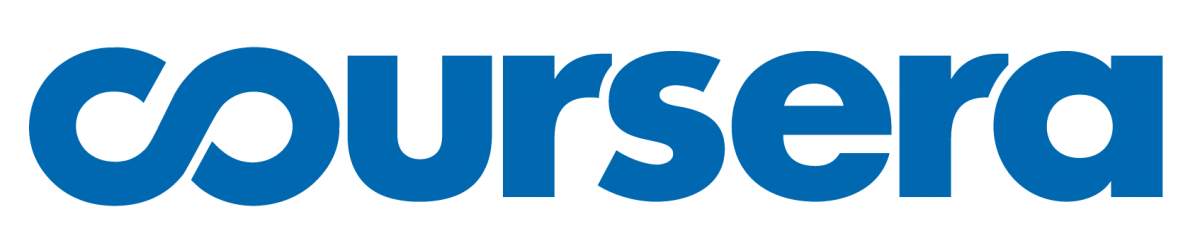
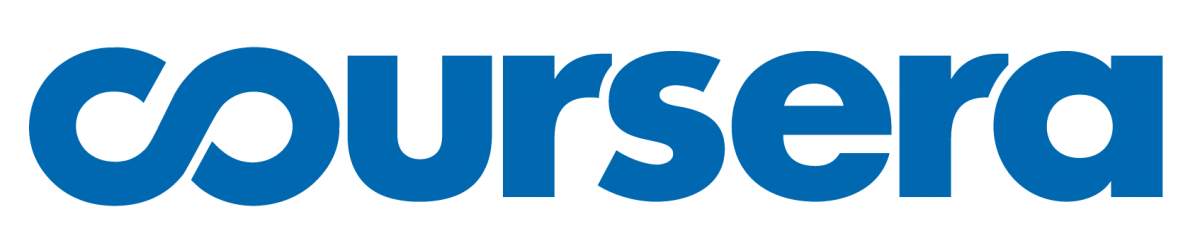
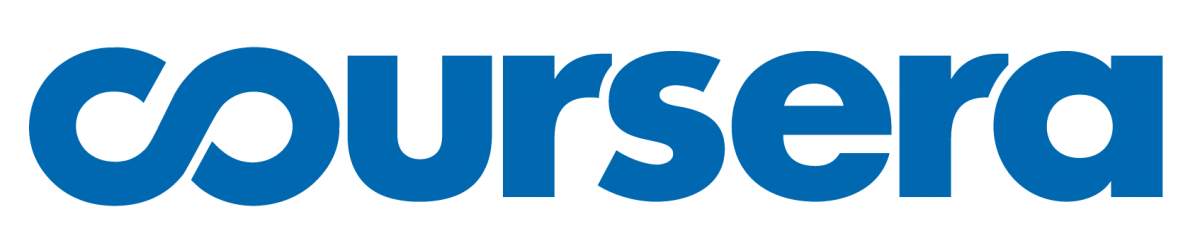
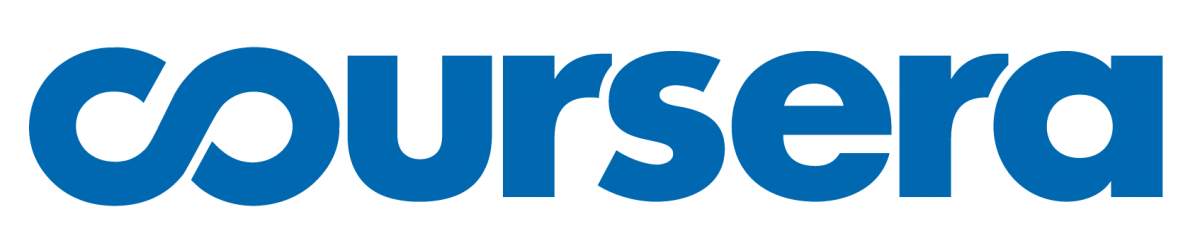
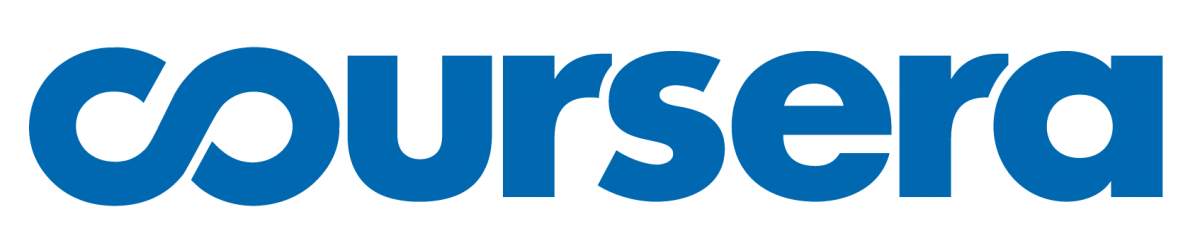
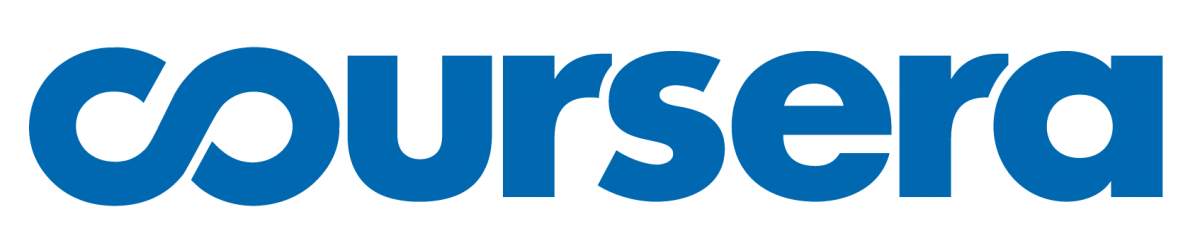
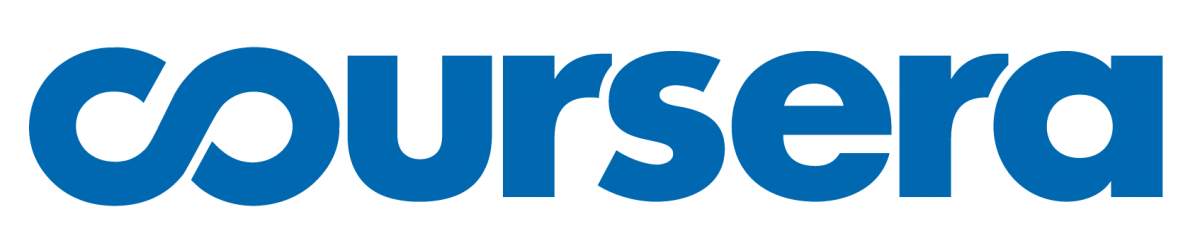
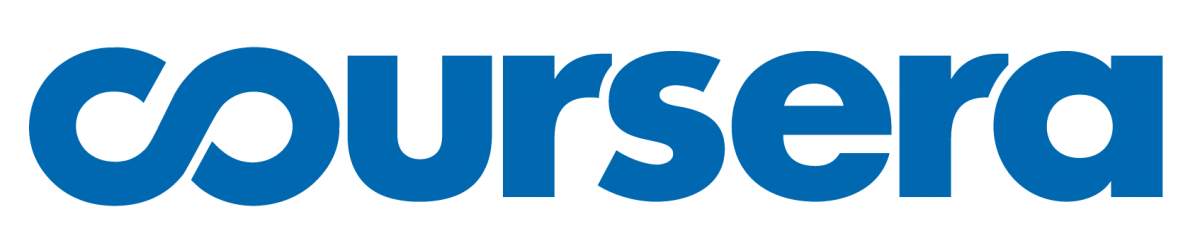
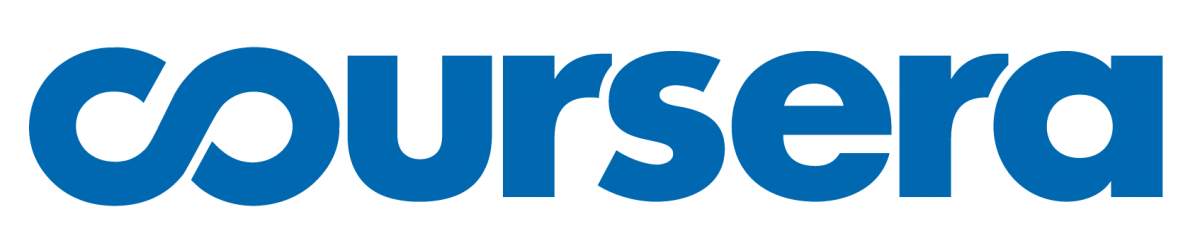
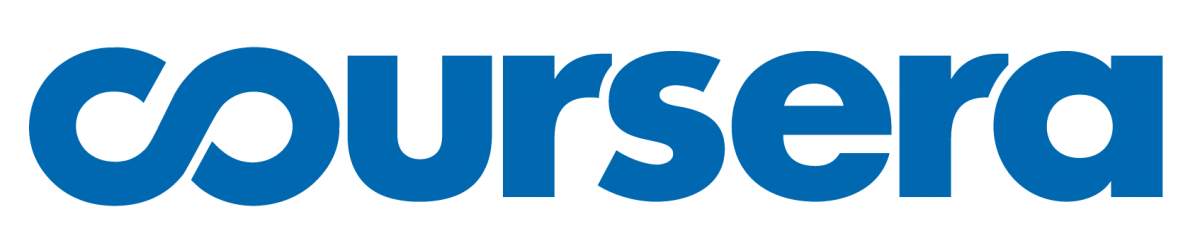
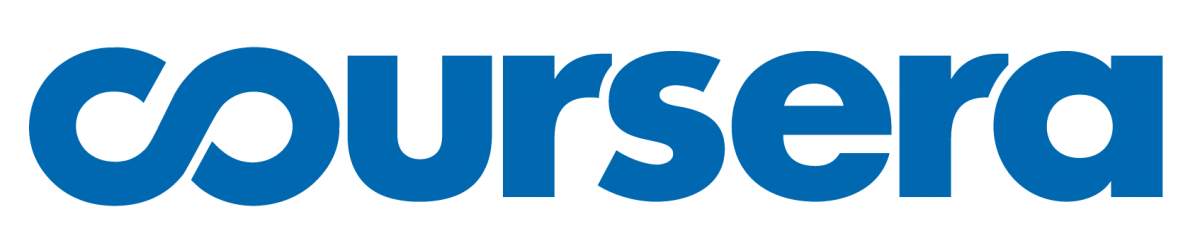
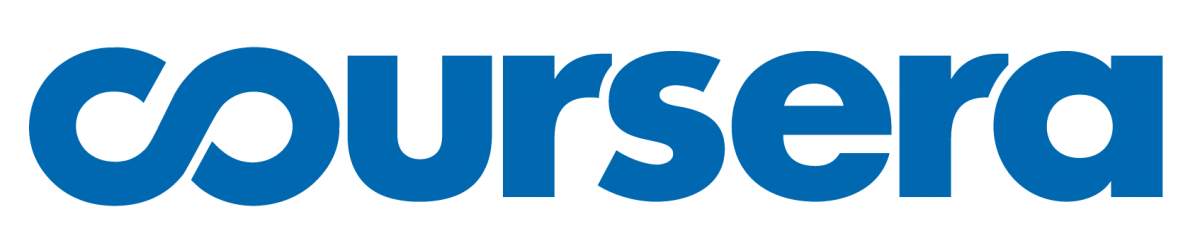
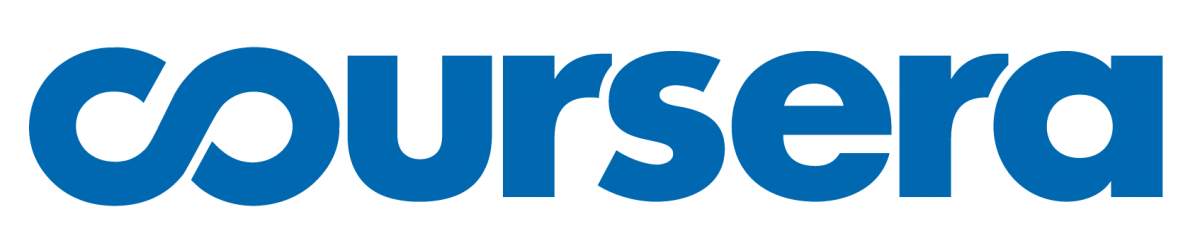
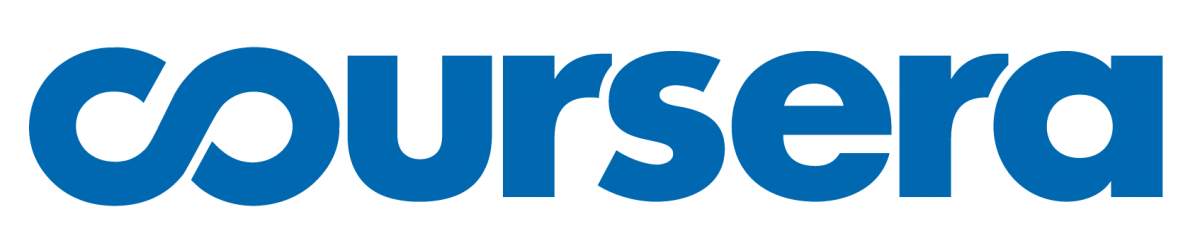
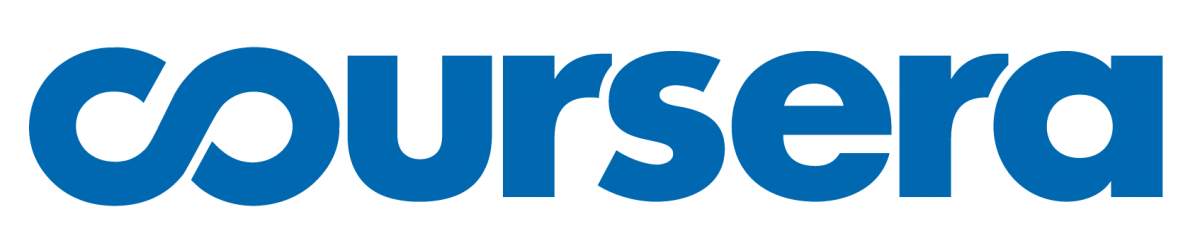
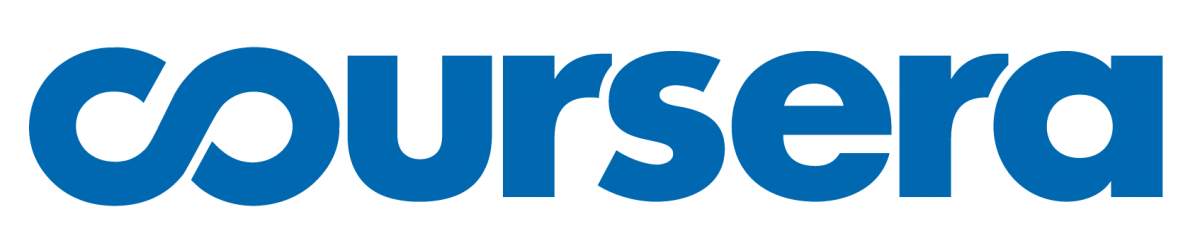
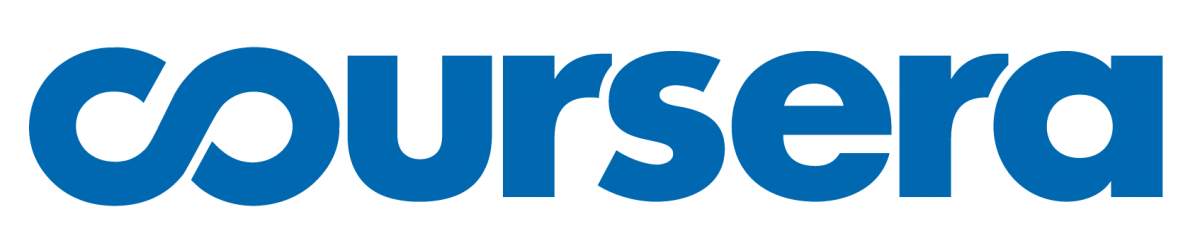
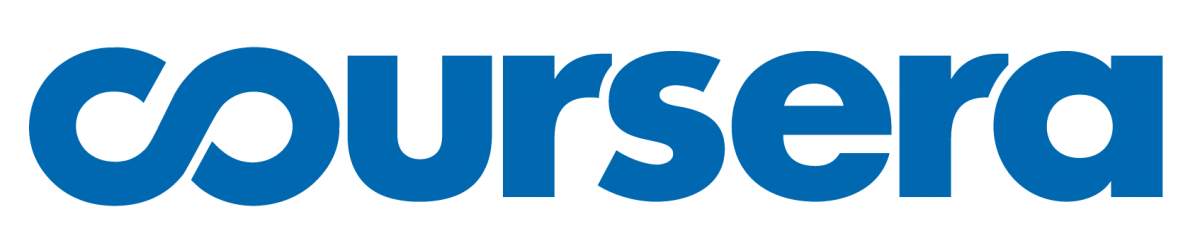
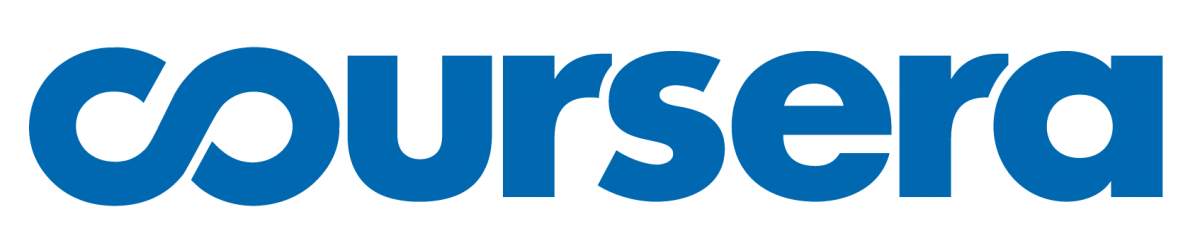
Description
This beginner course thought by AI Expert and Coursera’s founder Andrew Ng, offers students to understand many important aspects of machine learning. The course involves: introduction to machine learning, data mining, and statistical pattern recognition and it covers best practices in machine learning as well. The course outlines numerous case studies and applications of machine learning in the real world.
Teachers:
- Stanford: Andrew NG
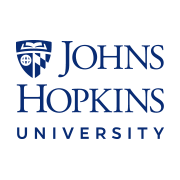
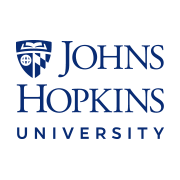
Genomic Data Science
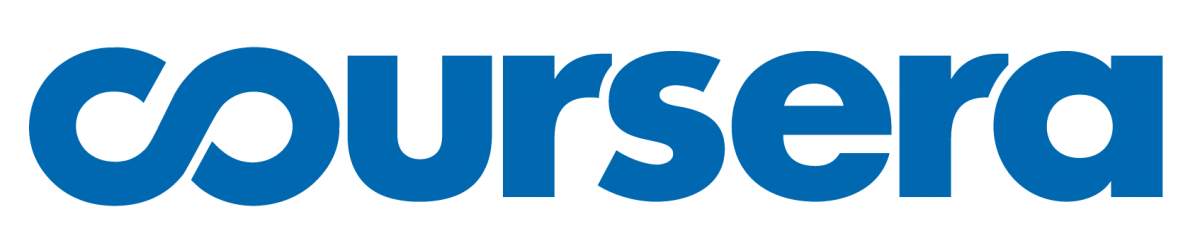
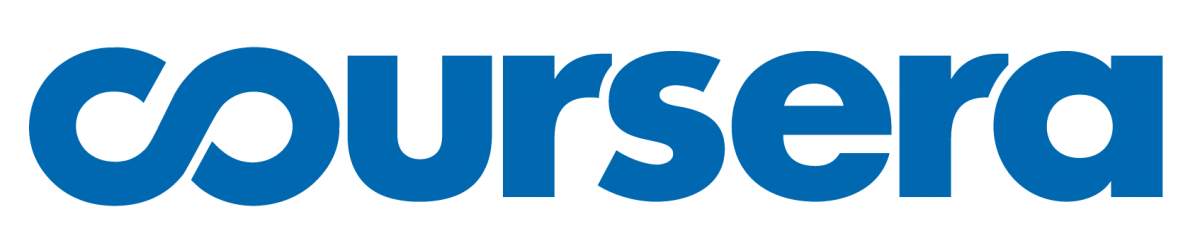
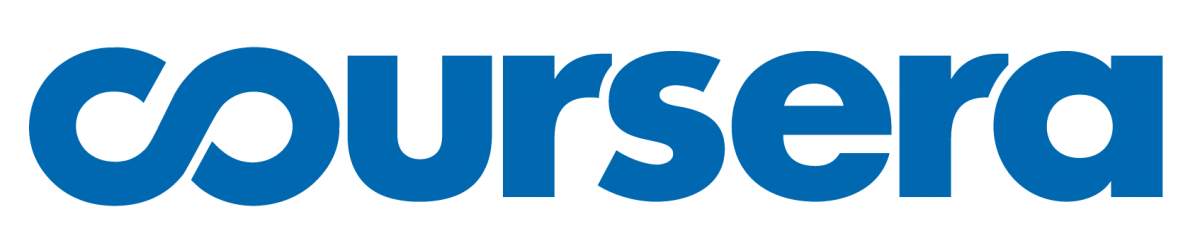
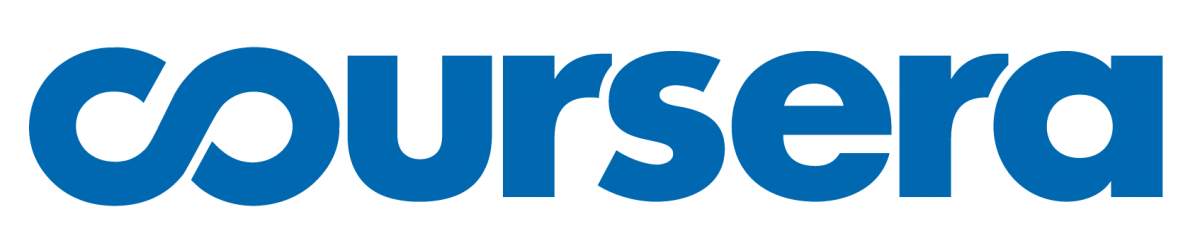
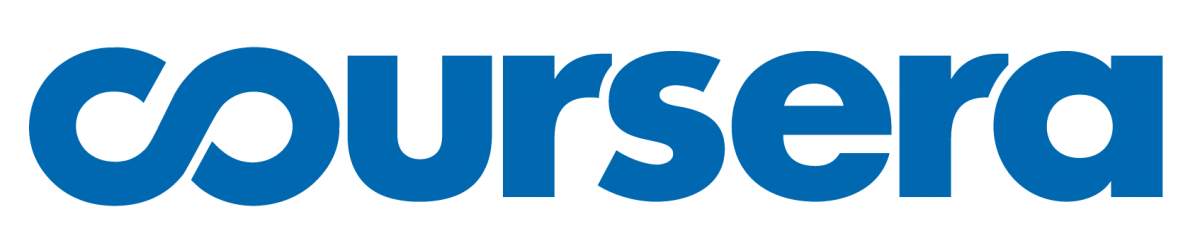
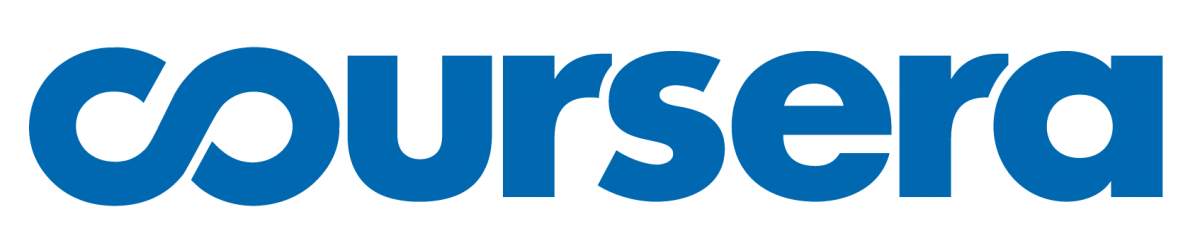
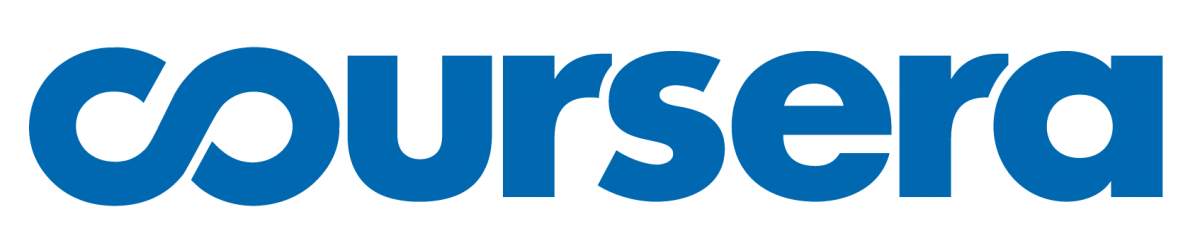
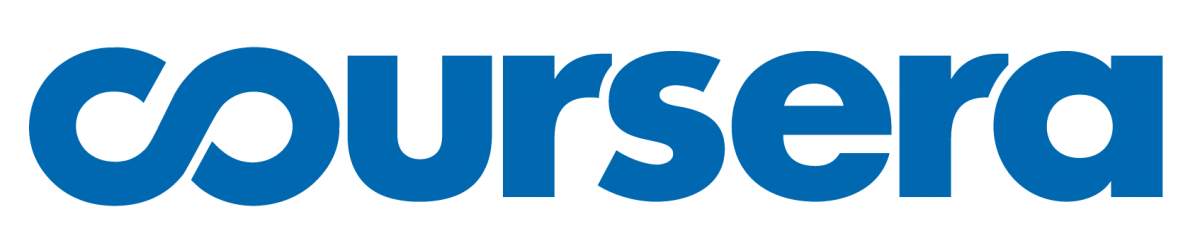
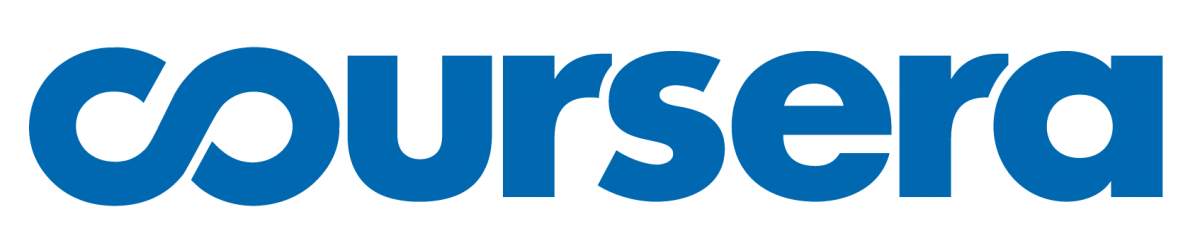
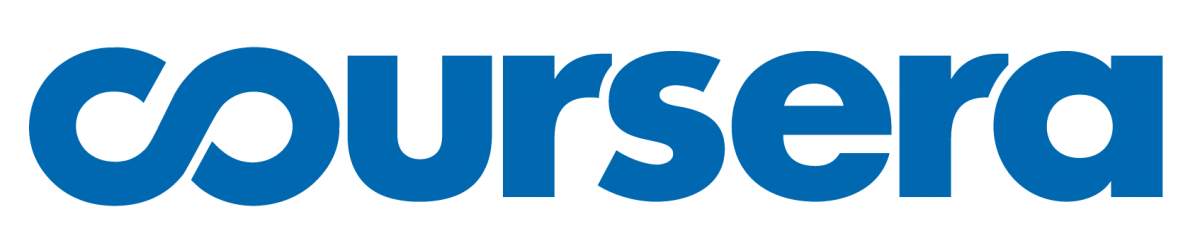
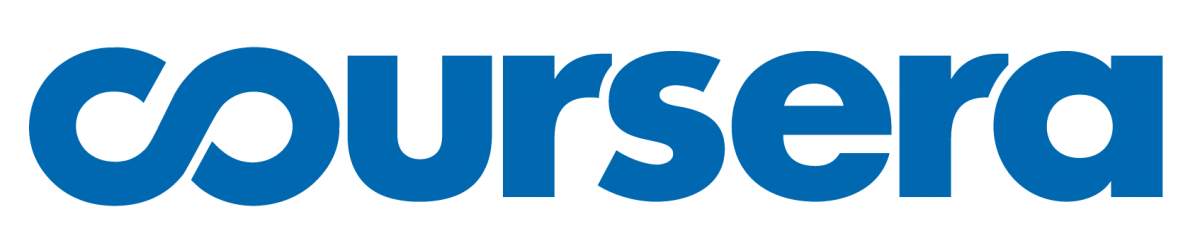
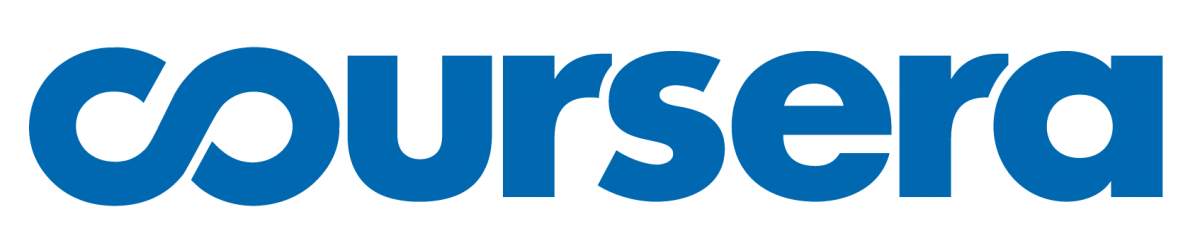
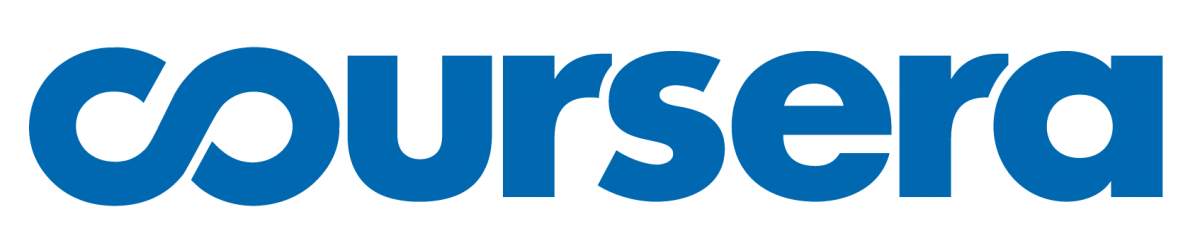
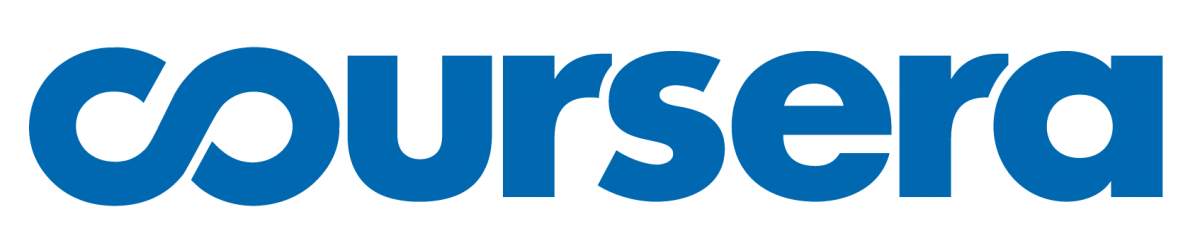
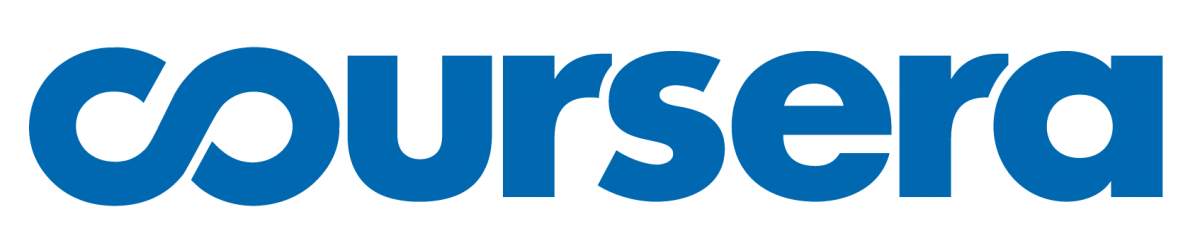
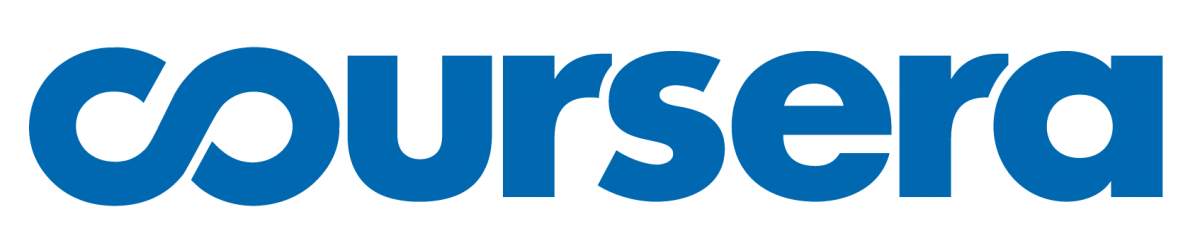
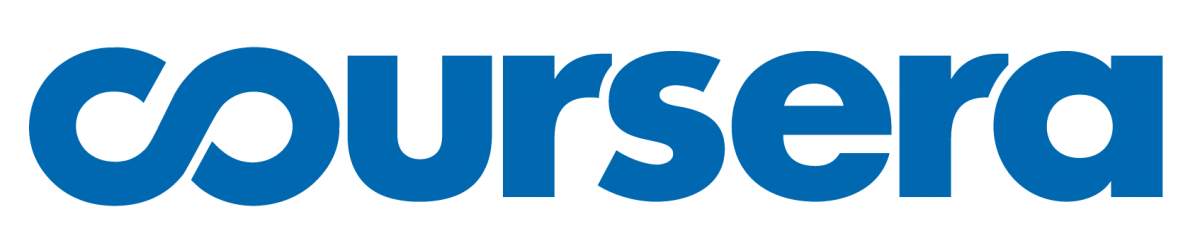
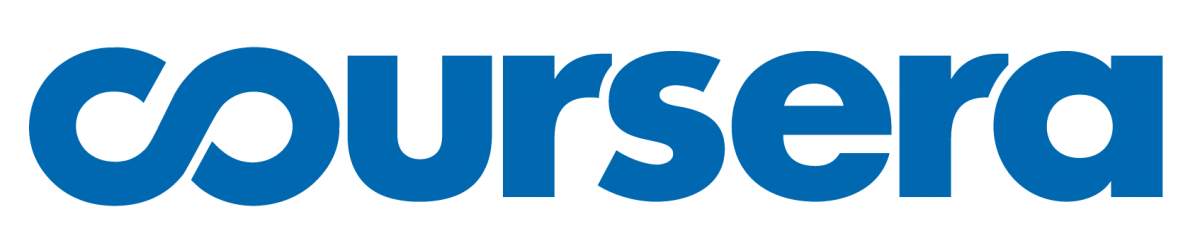
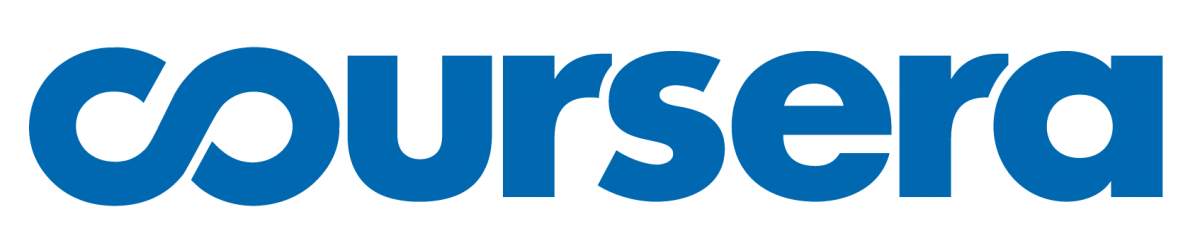
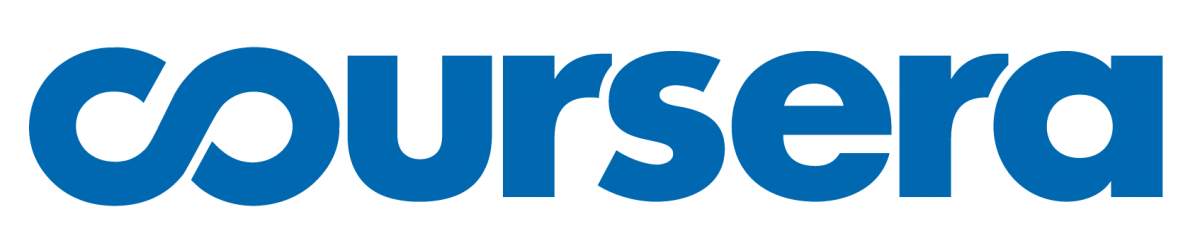
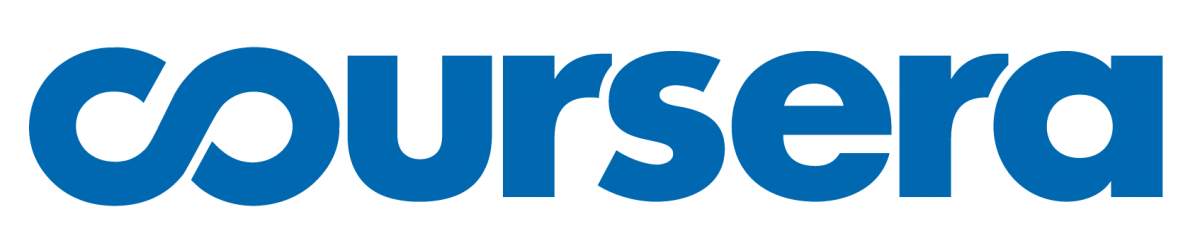
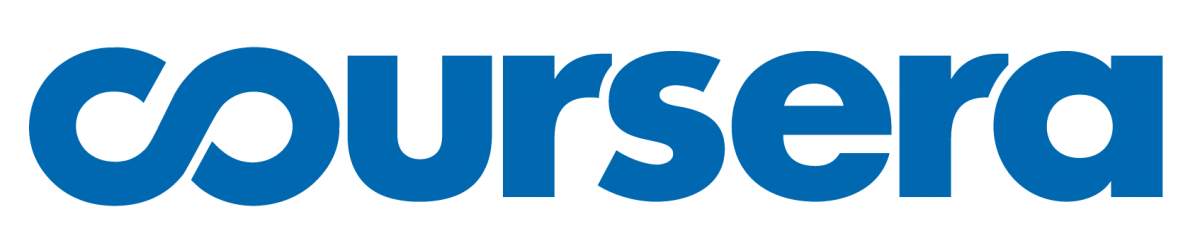
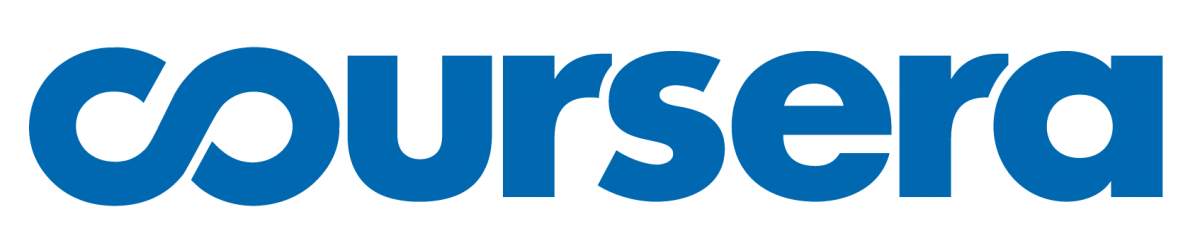
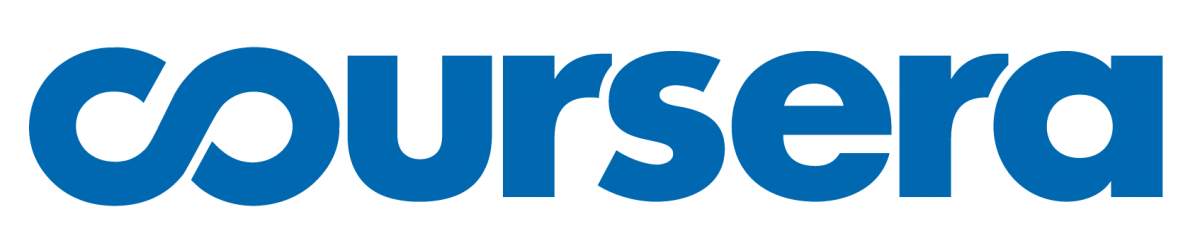
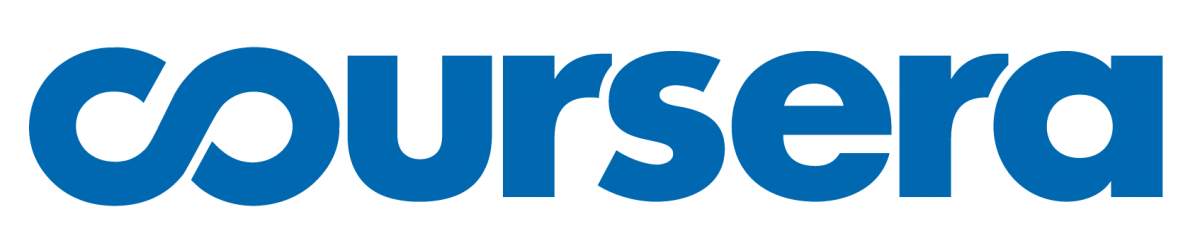
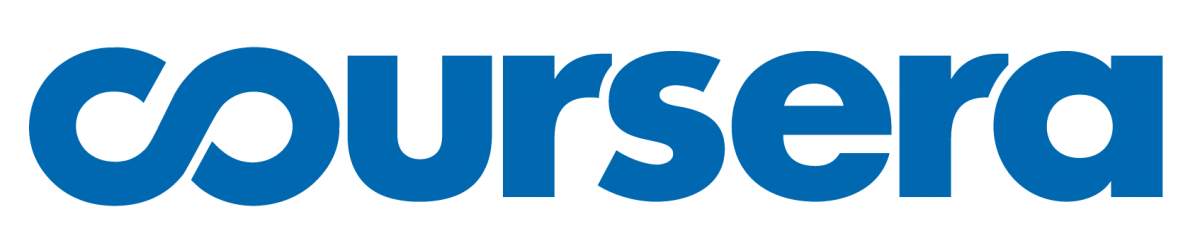
Description
As part of this specialization, students will learn genomic technologies and how to perform genome analysis using a wide variety of tools and concepts which are covered within the course. It consists of 8 courses which go from introduction to the basic biology of modern genomics all the way to genomic data science. The courses are thought by professors and researchers from the John Hopkins University.
Teachers:
- Jonhs Hopkins University: Jacob Pritt, Department of Computer Science
- Jonhs Hopkins University: James Taylor, PhD
- Jonhs Hopkins University: Liliana Florea, PhD, Assistant Professor
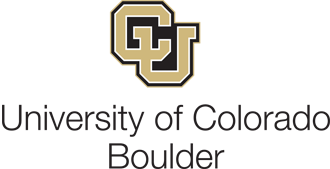
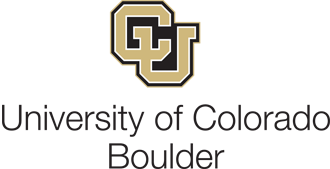
Data Warehousing for Business Intelligence
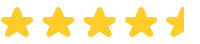
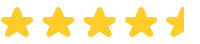
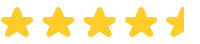
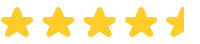
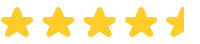
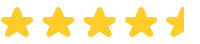
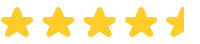
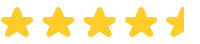
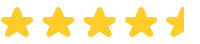
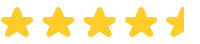
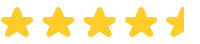
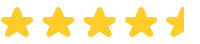
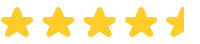
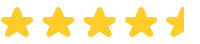
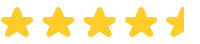
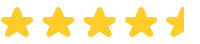
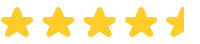
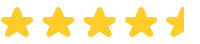
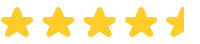
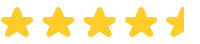
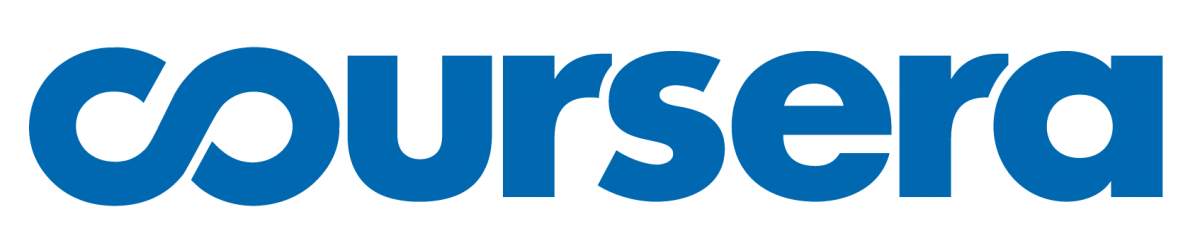
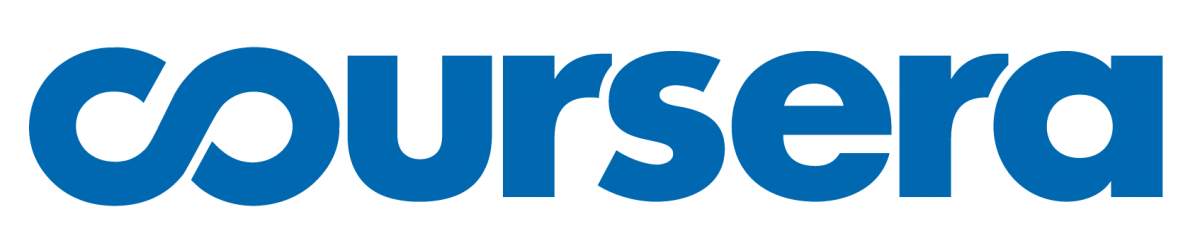
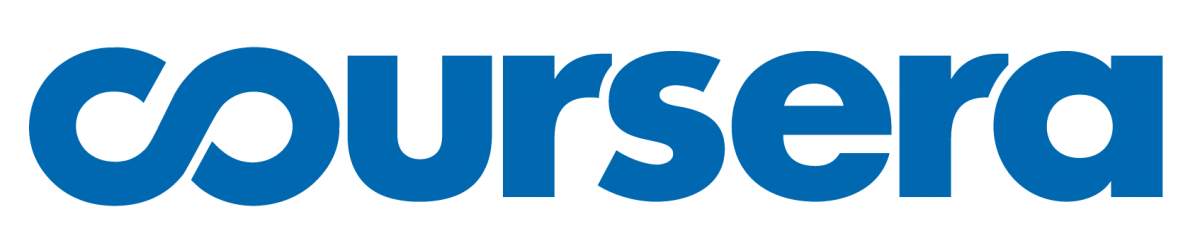
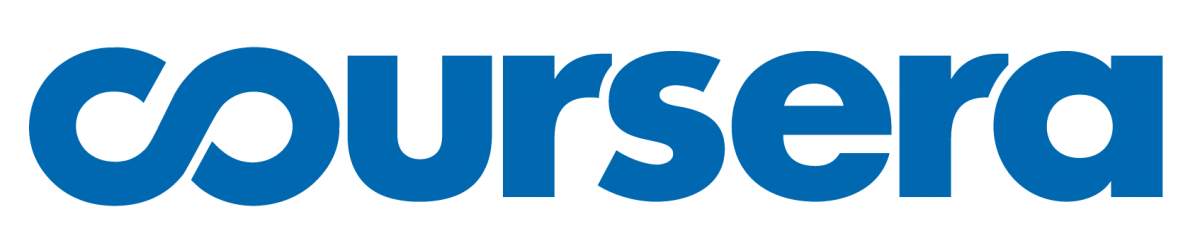
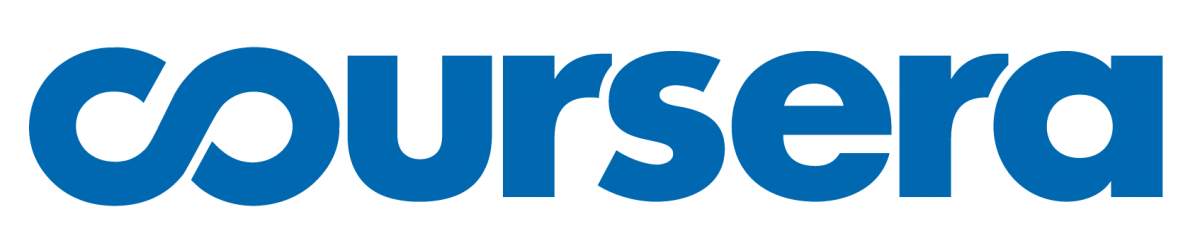
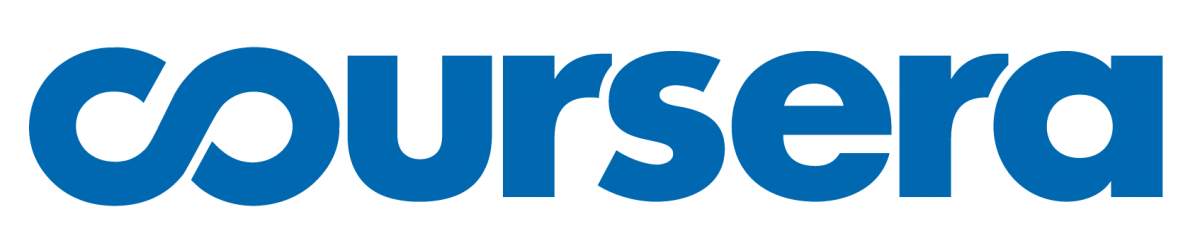
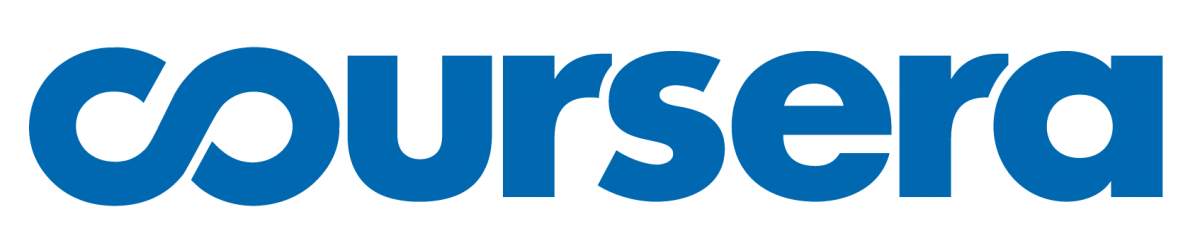
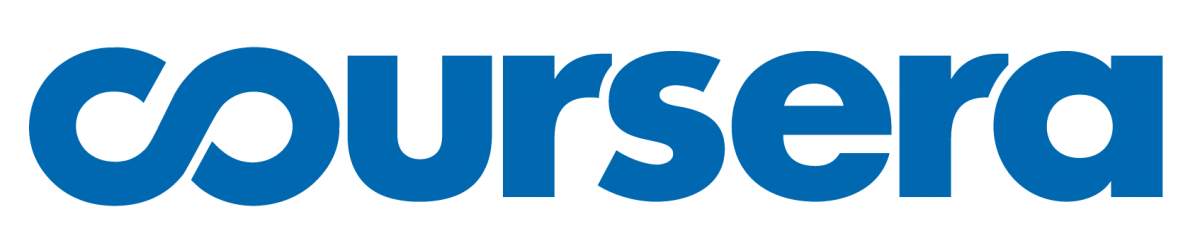
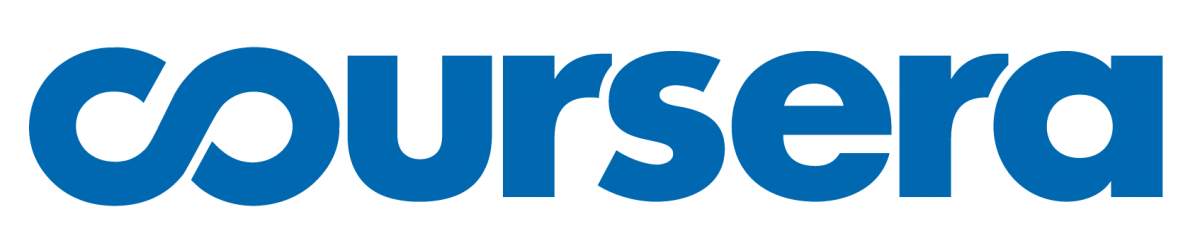
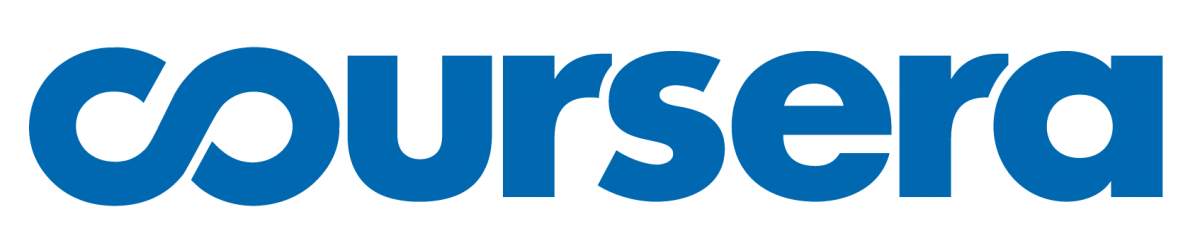
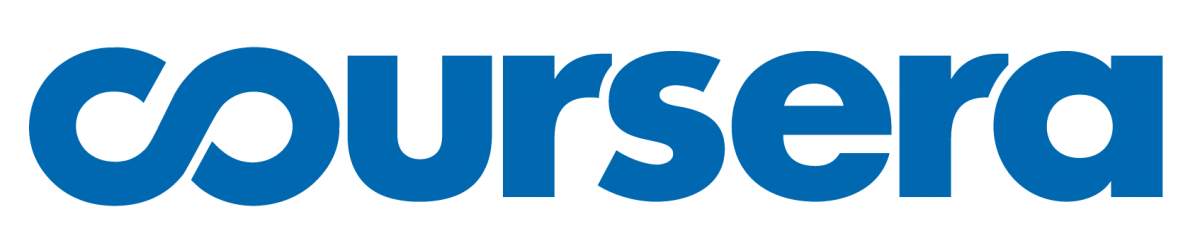
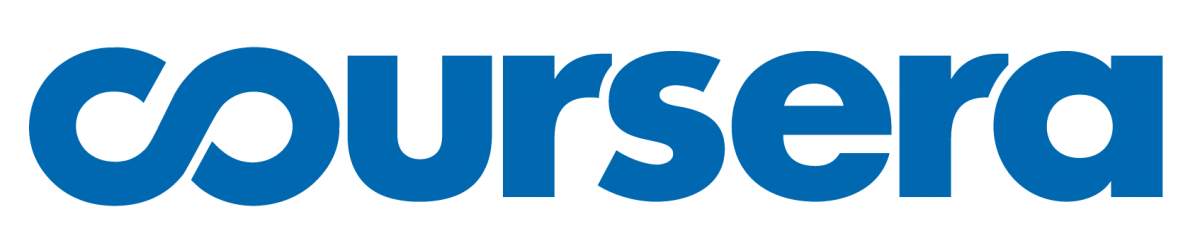
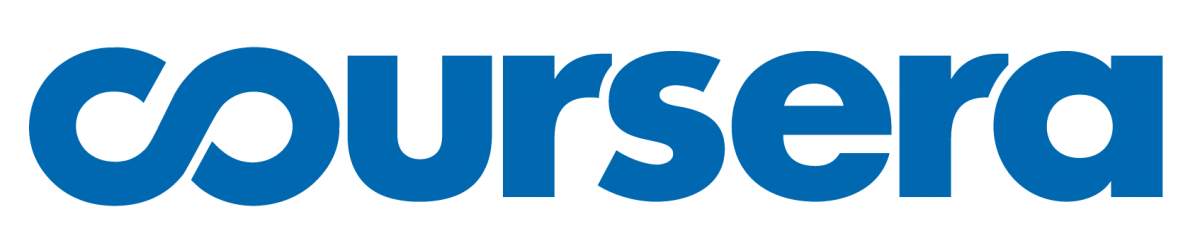
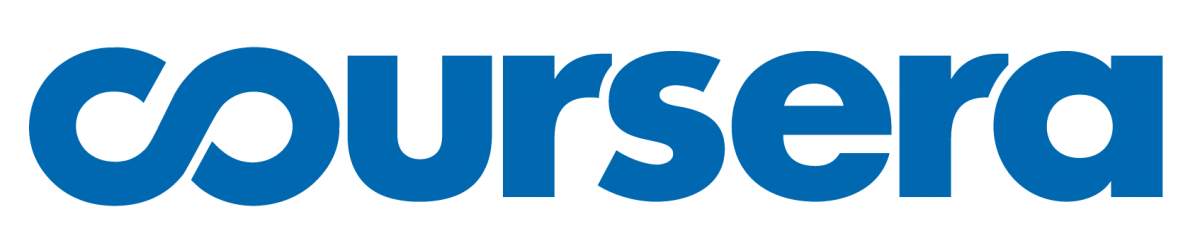
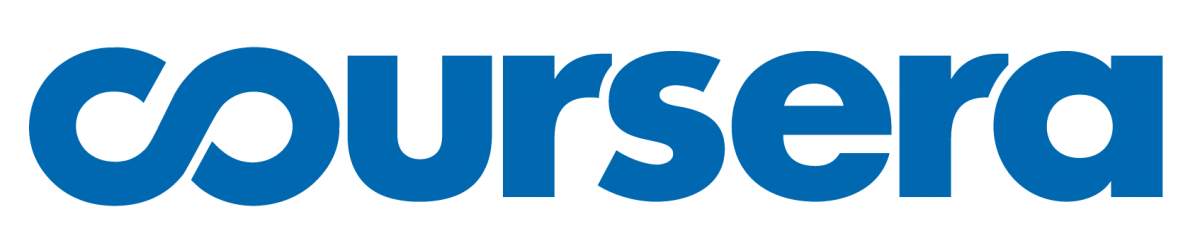
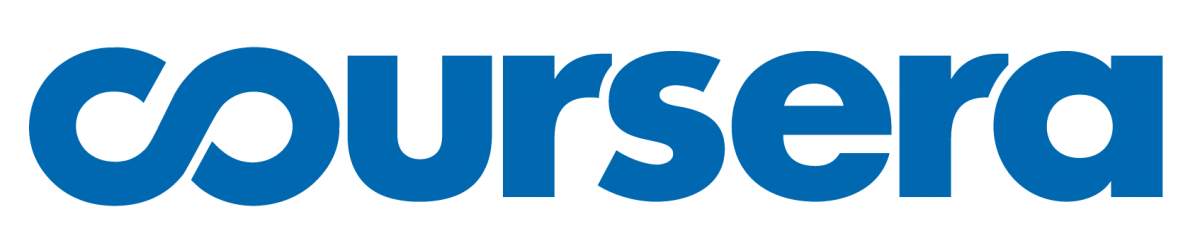
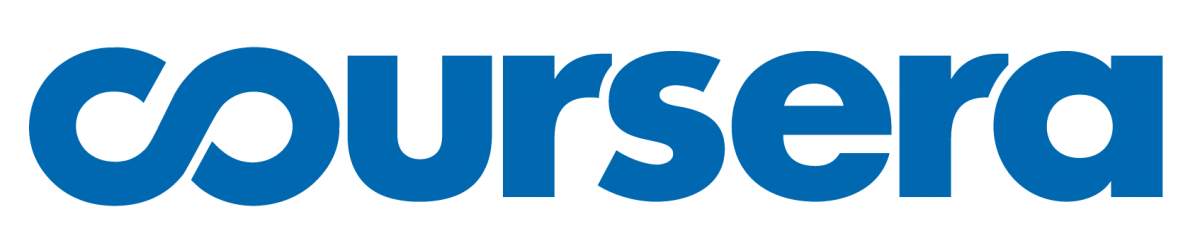
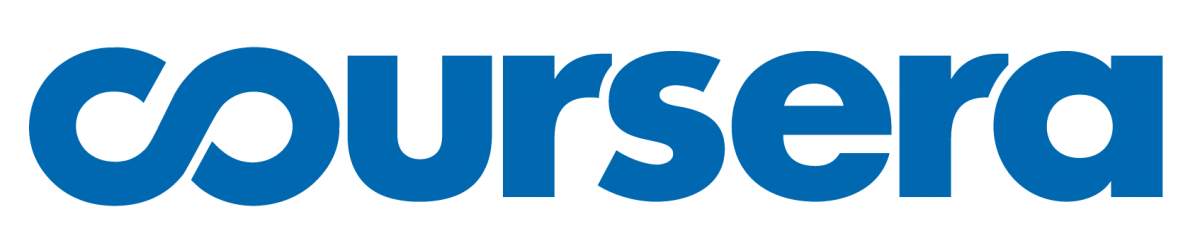
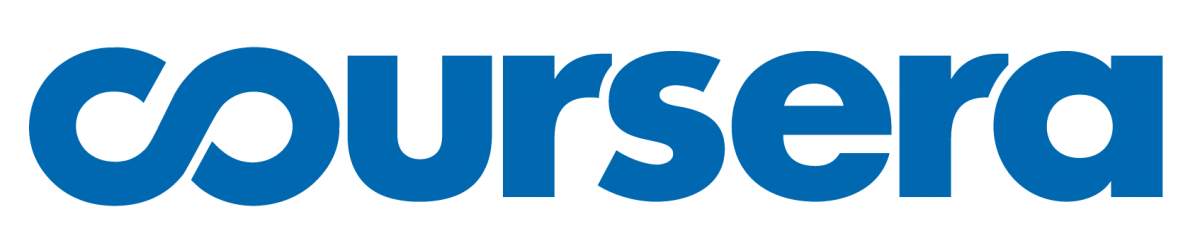
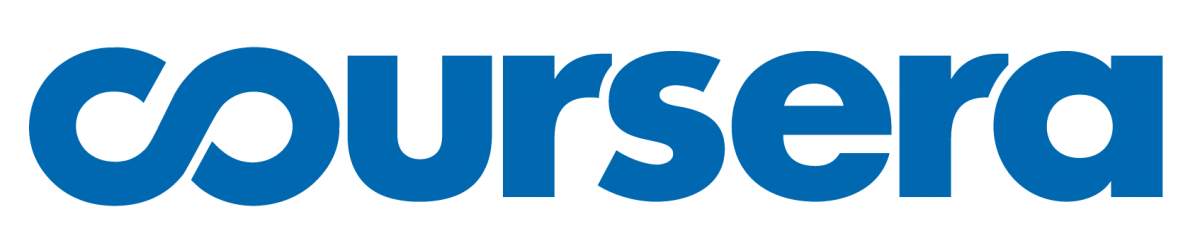
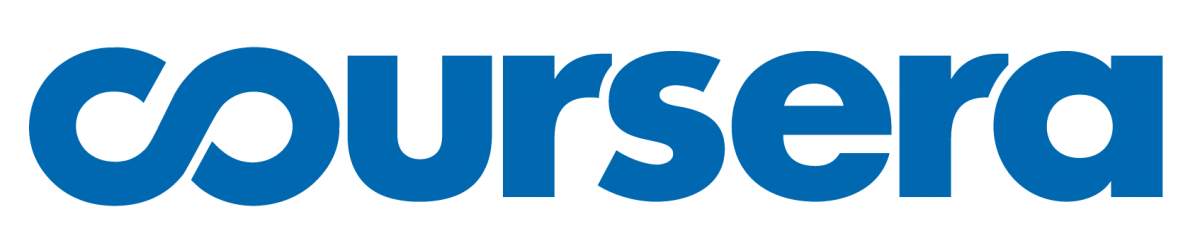
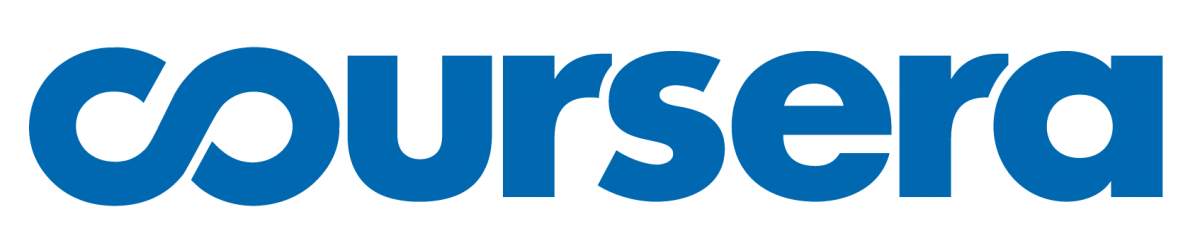
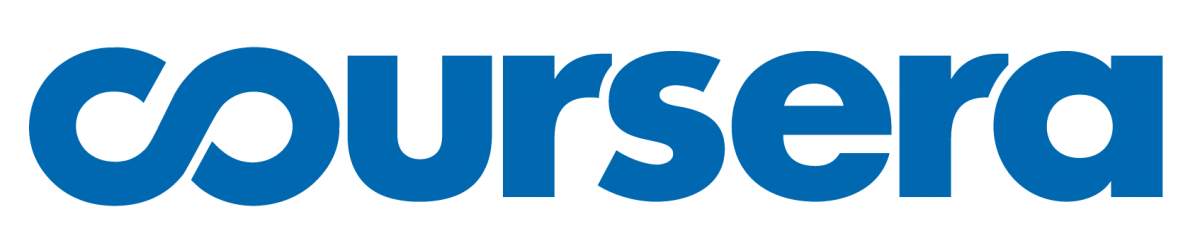
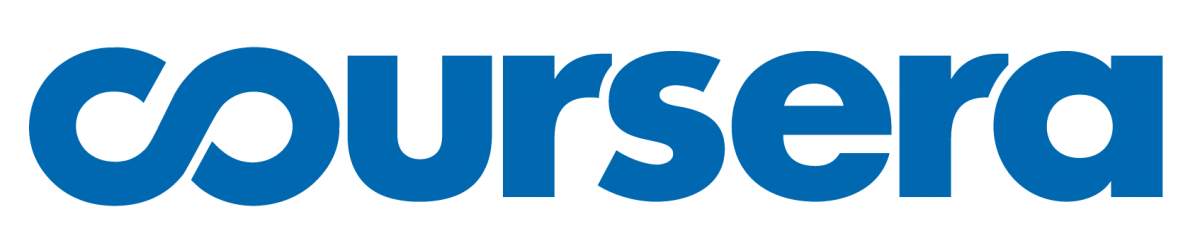
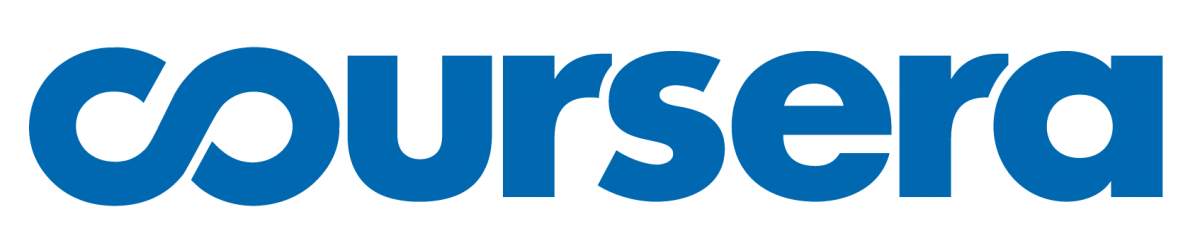
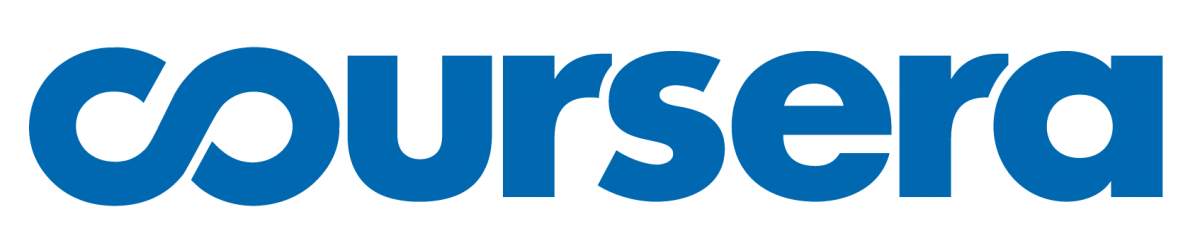
Description
This specialization offers students to gain data architecture skills that are increasingly critical across a broad range of technology fields. Students will learn the basics of structured data modeling with SQL and develop understanding of data warehouse designs and data manipulation. This specialization is labeled as an advanced level since it requires considerable knowledge of the background of databases. The 5 courses that are part of this specialization cover many concepts such as ER Model, DBMS, SQL, ETL processes, data visualization etc.
Teachers:
- University of Colorado: Michael Mannino, Associate Professor
- University of Colorado: Jahangir Karimi, Professor
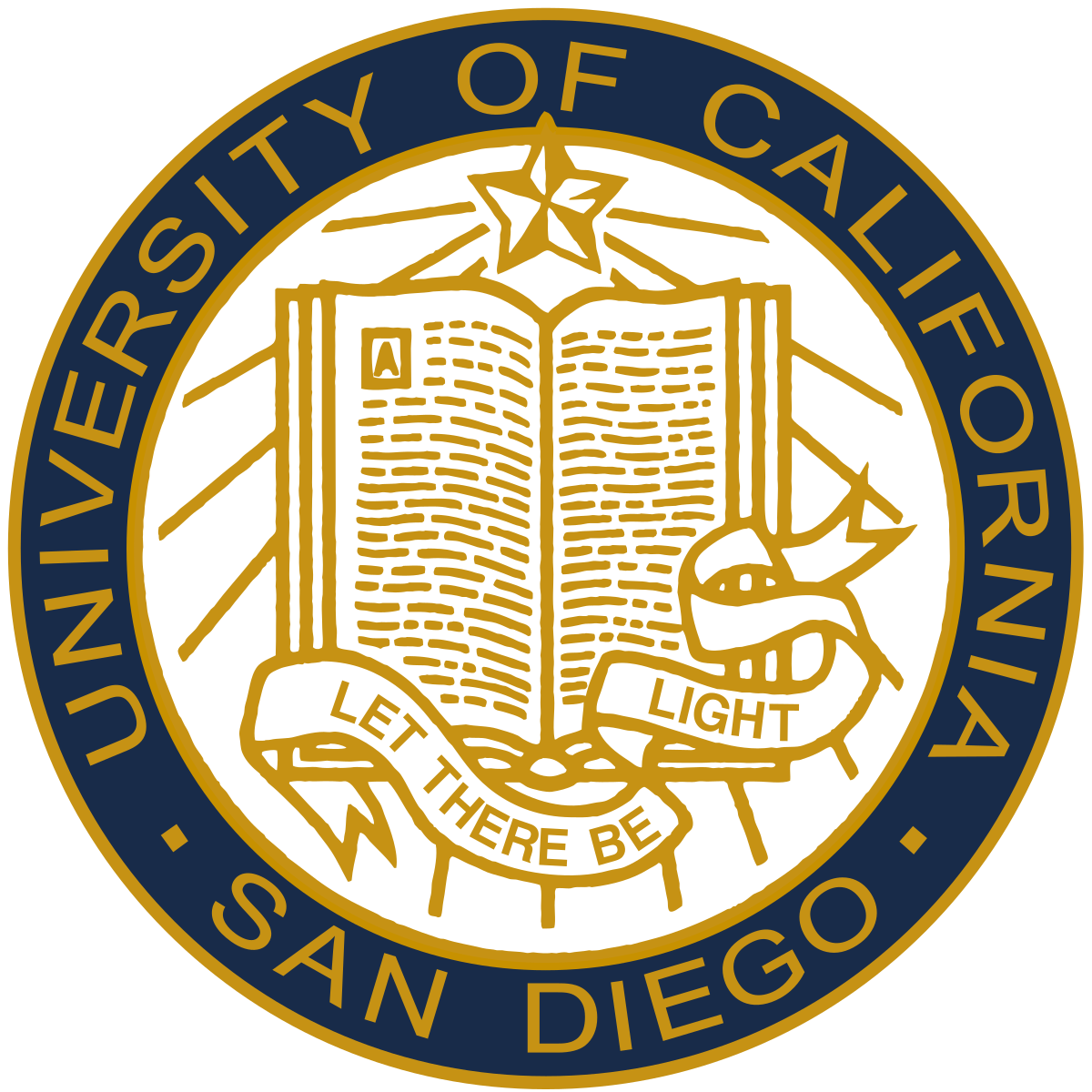
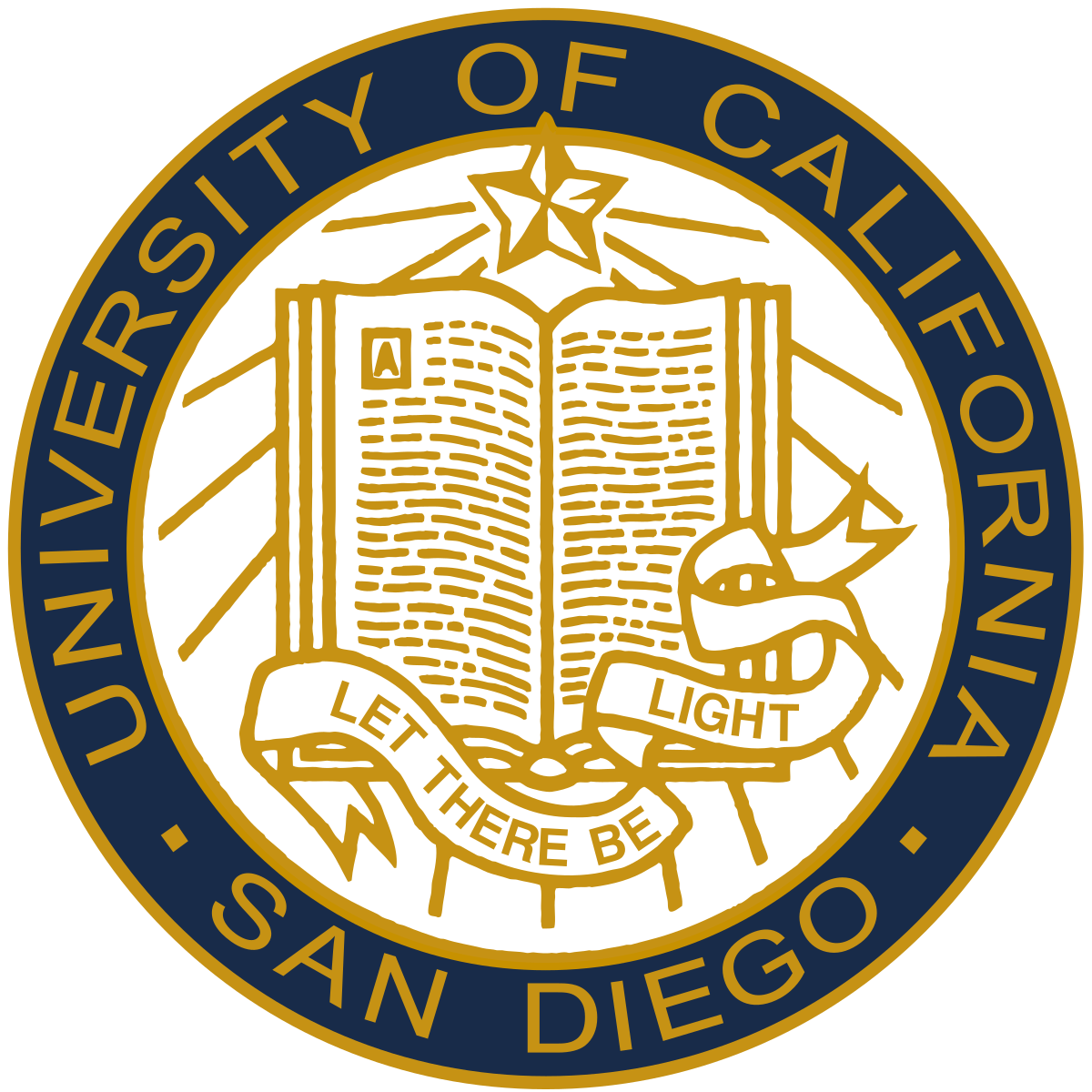
Big Data
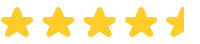
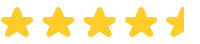
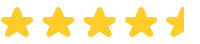
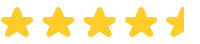
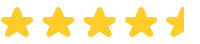
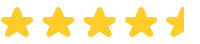
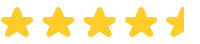
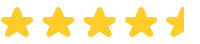
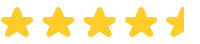
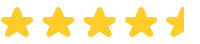
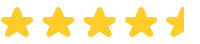
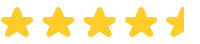
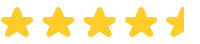
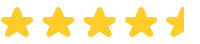
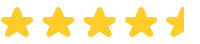
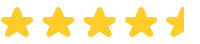
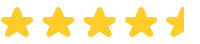
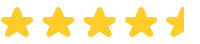
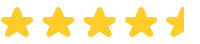
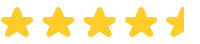
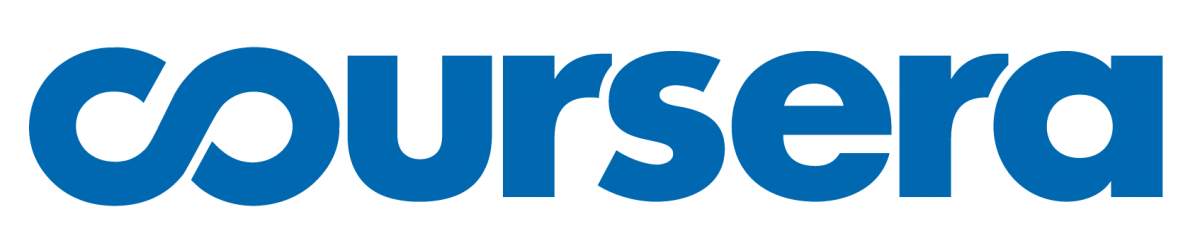
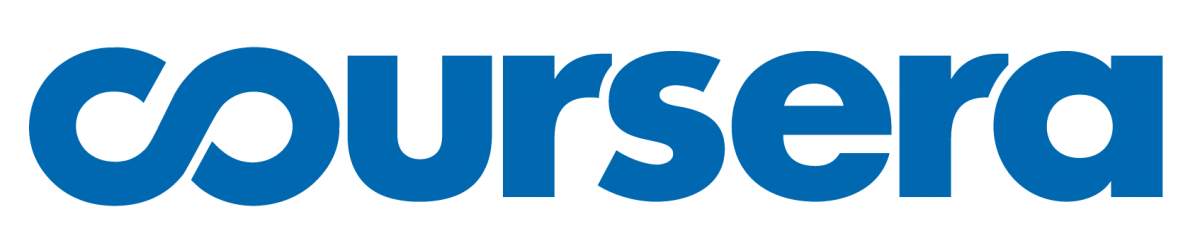
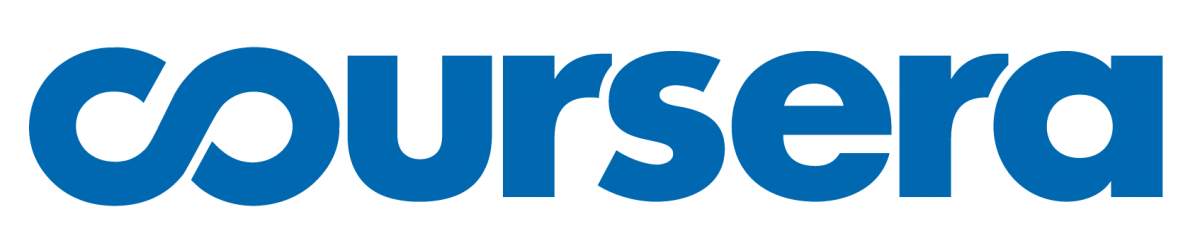
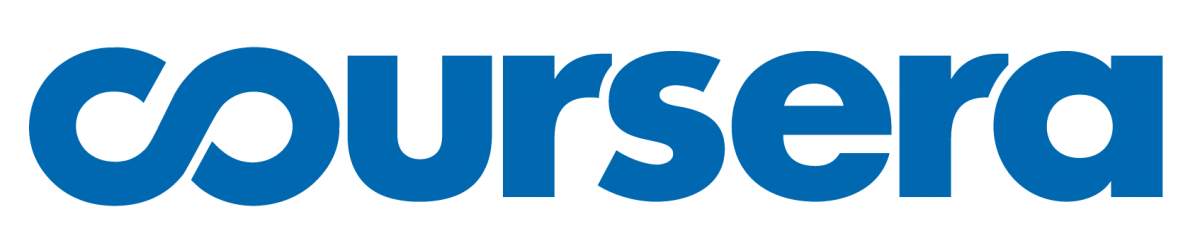
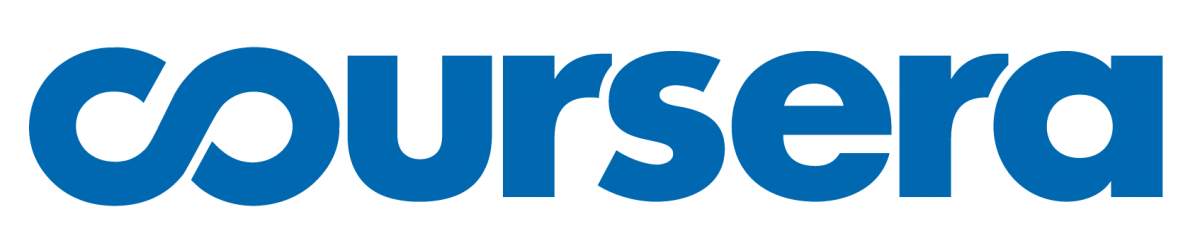
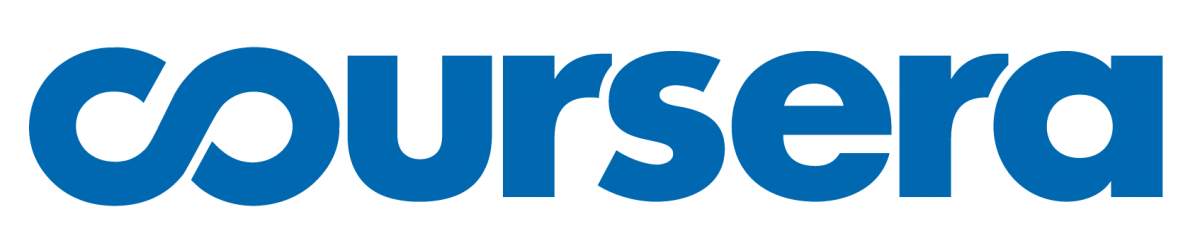
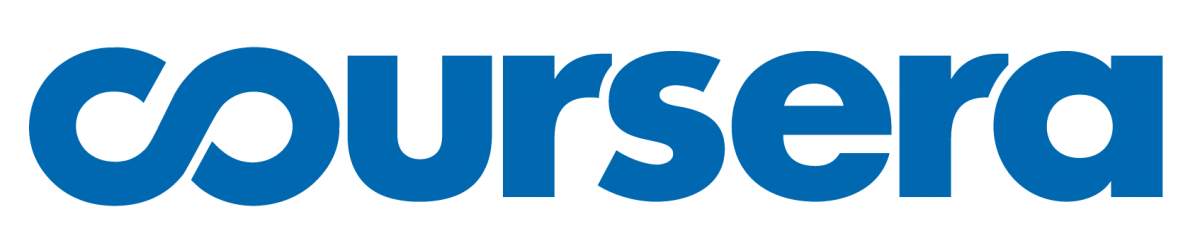
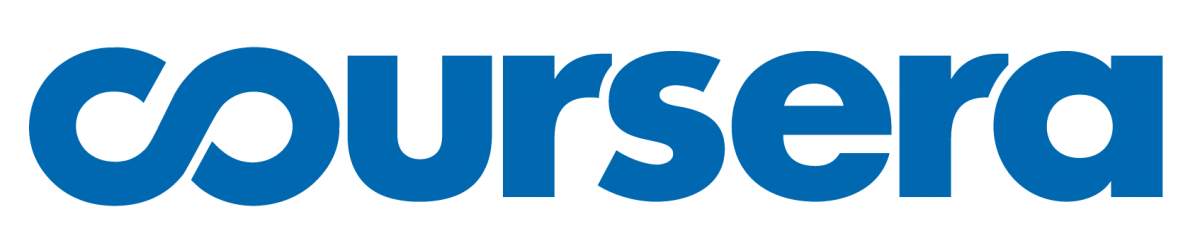
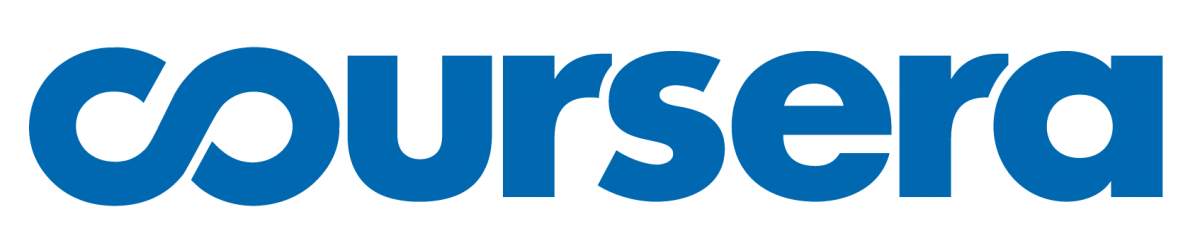
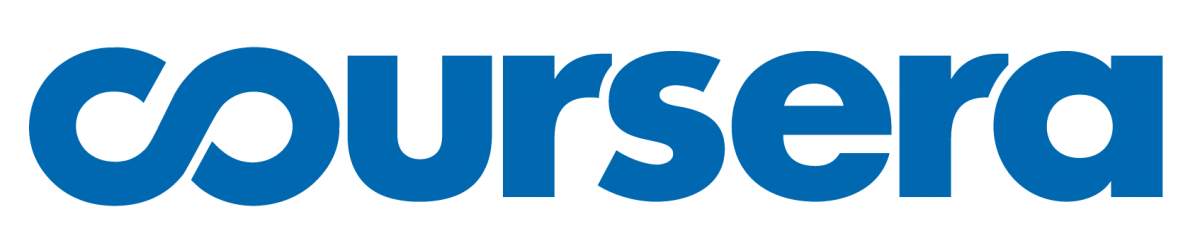
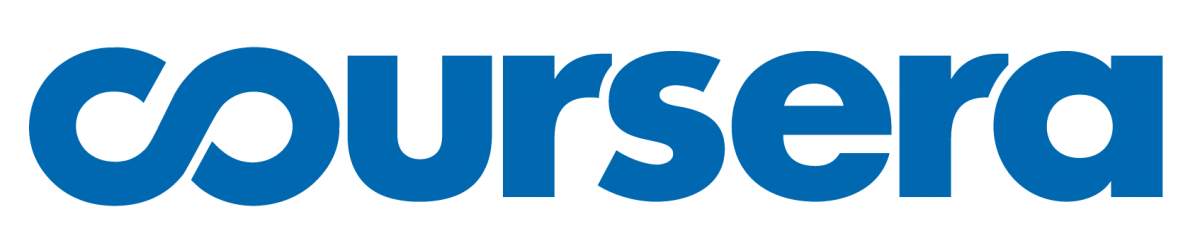
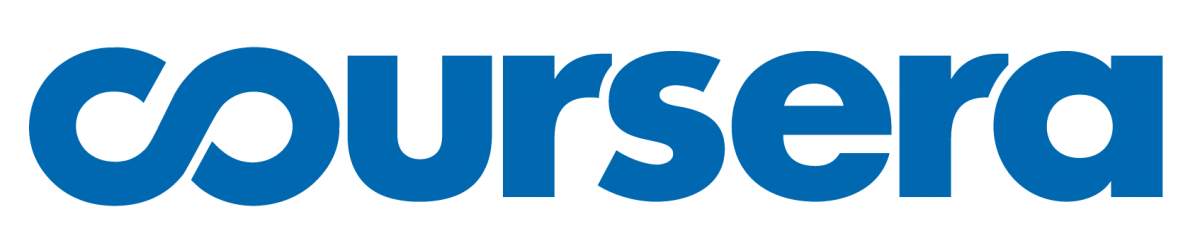
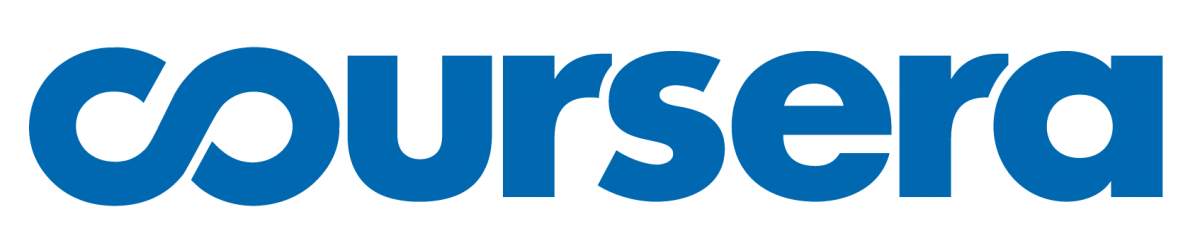
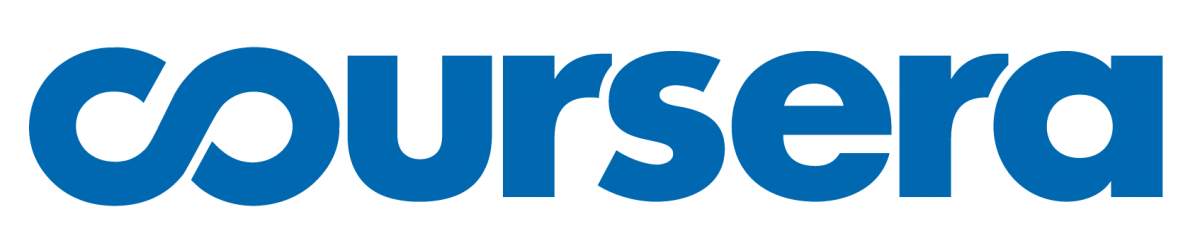
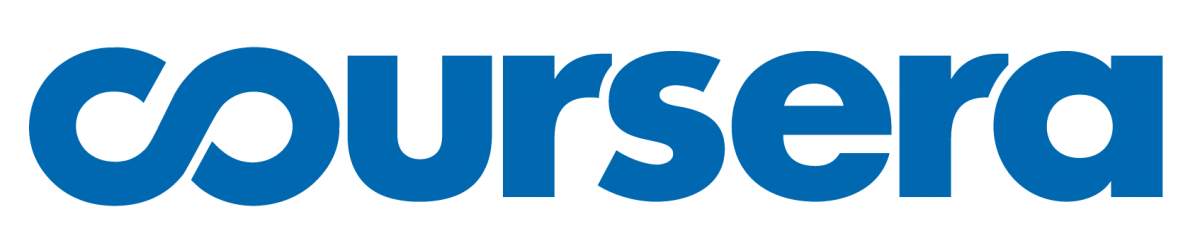
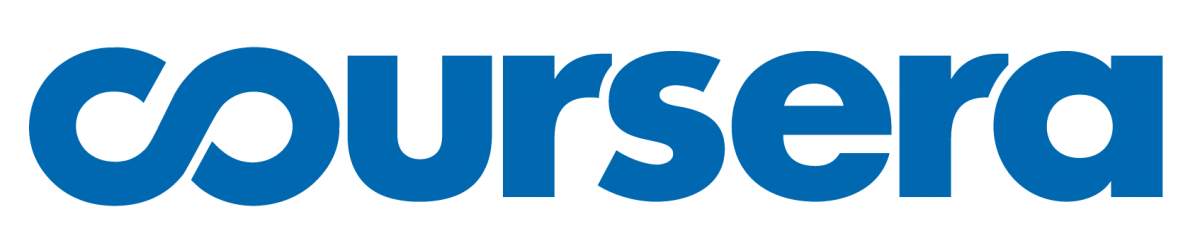
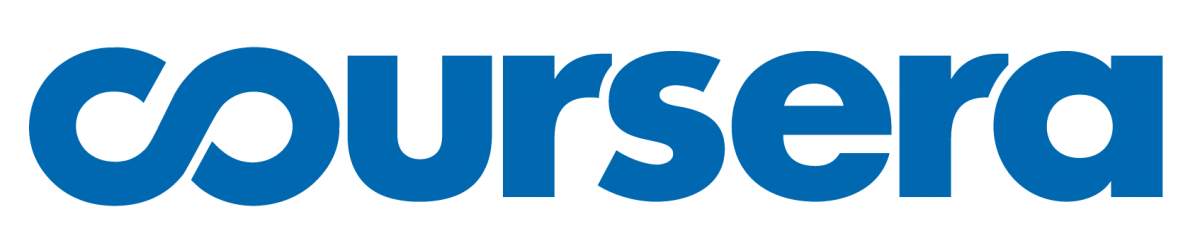
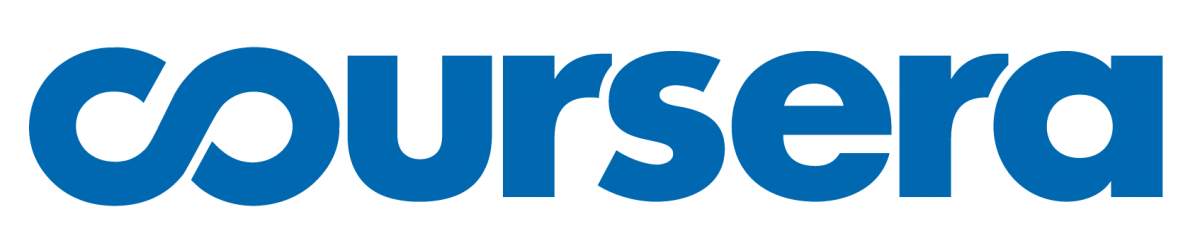
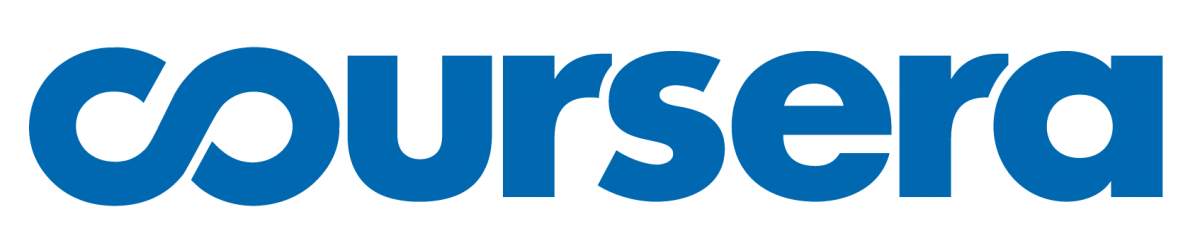
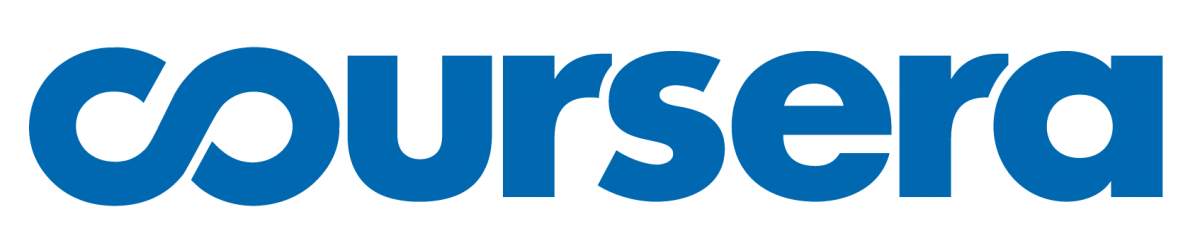
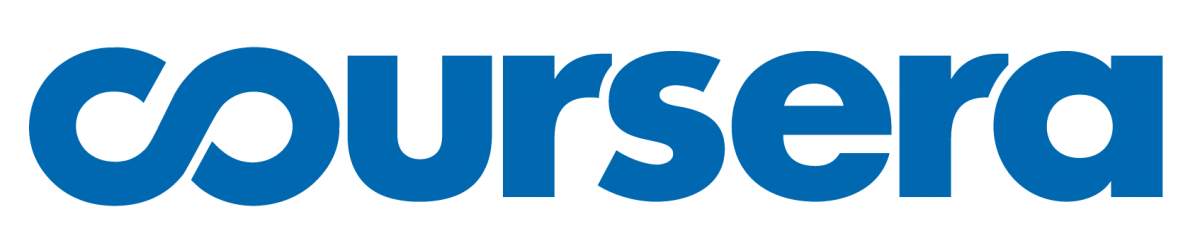
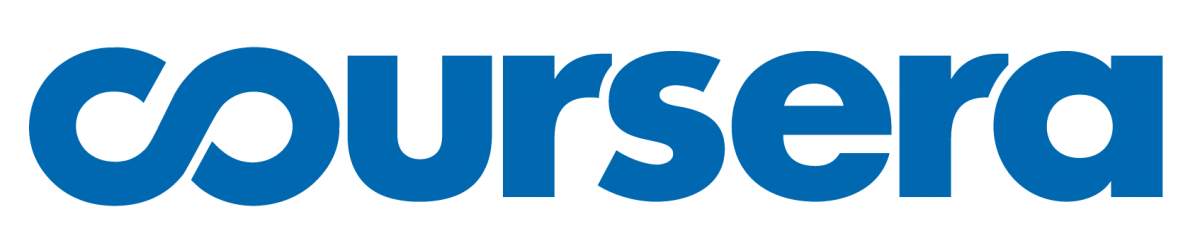
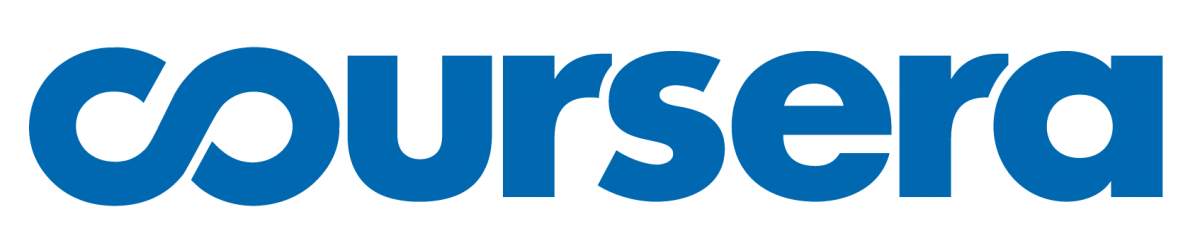
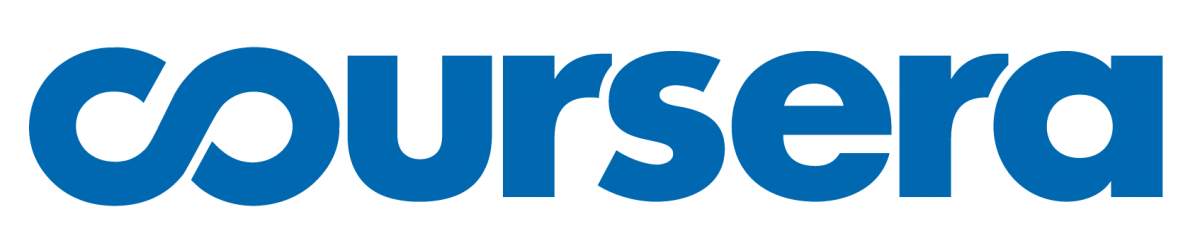
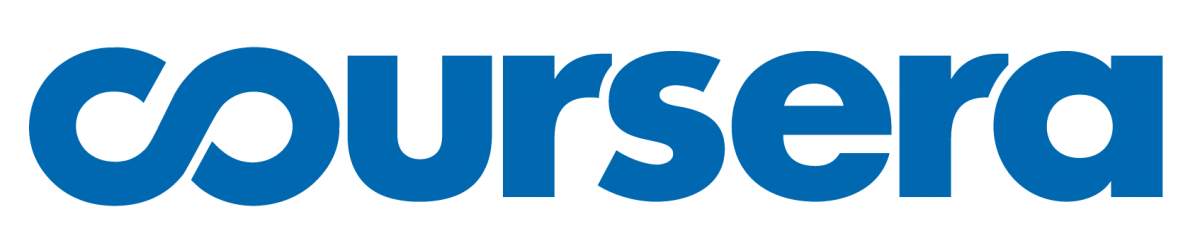
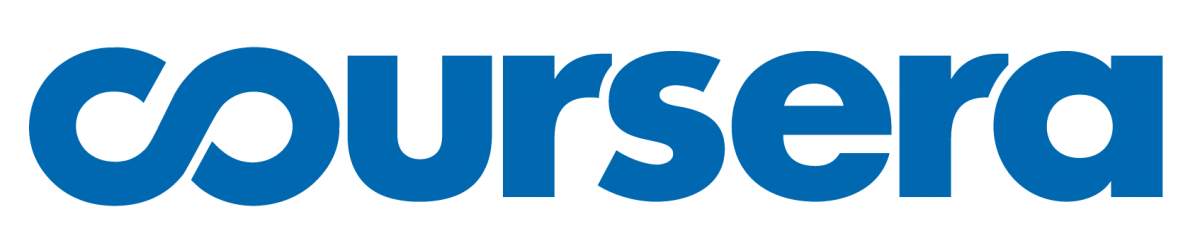
Description
A 6-course specialization that will enable students to gain an understanding of what insights big data can provide through hands-on experience with the tools and systems used by big data scientists and engineers. The specialization starts with introduction to bid data and covers big data processing, machine learning as well as graph analytics. The last course of the specialization is a final “Capstone Big Data Project”.
Teachers:
- San Diego Supercomputer Center (SDSC): Ilkay Altintas, Chief Data Science Officer
- San Diego Supercomputer Center (SDSC): Amarnath Gupta, Director, Advanced Query Processing Lab
- San Diego Supercomputer Center (SDSC): Mai Nguyen, Lead for Data Analytics
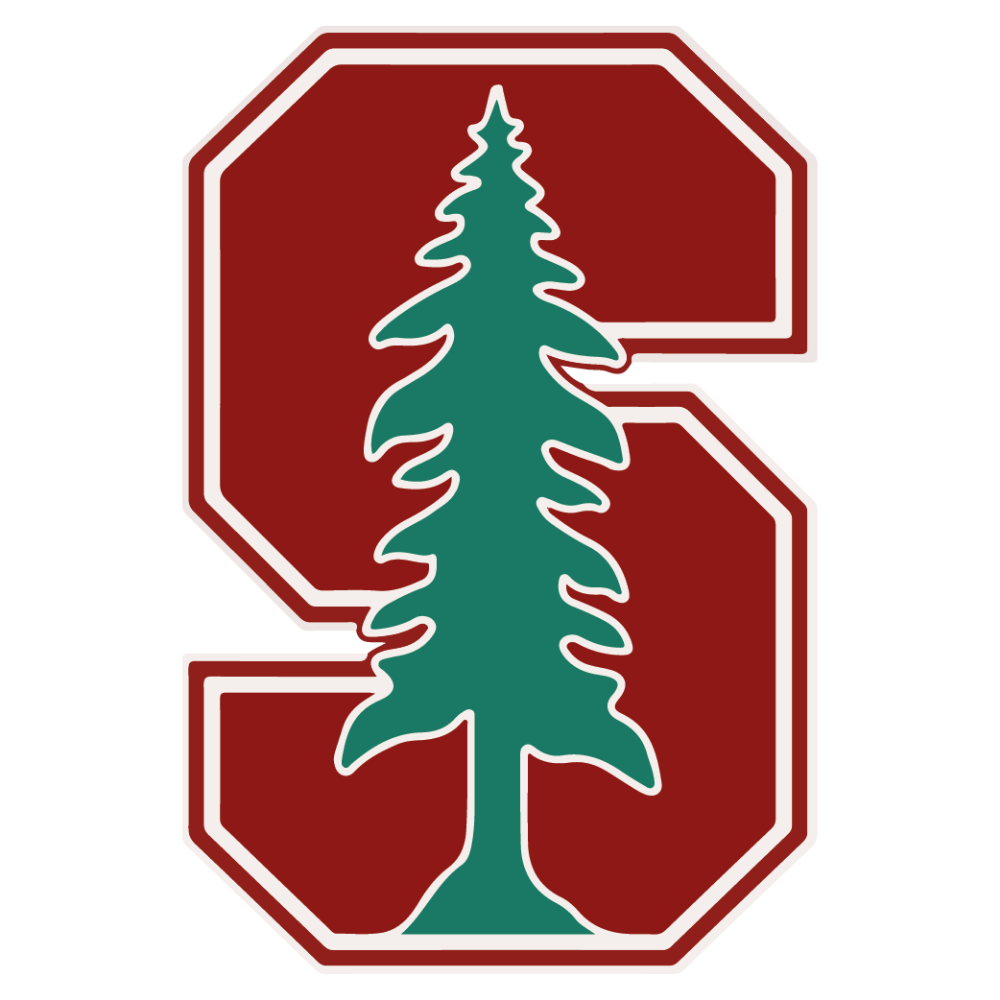
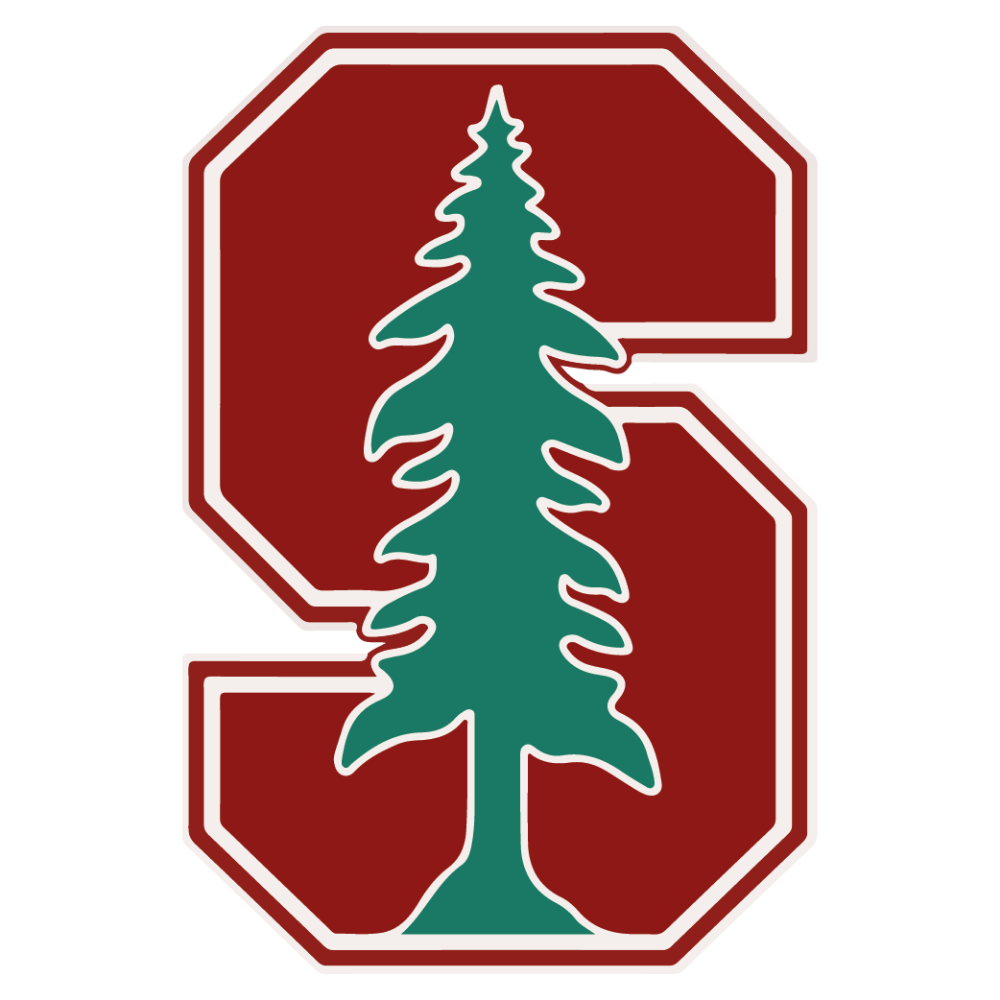
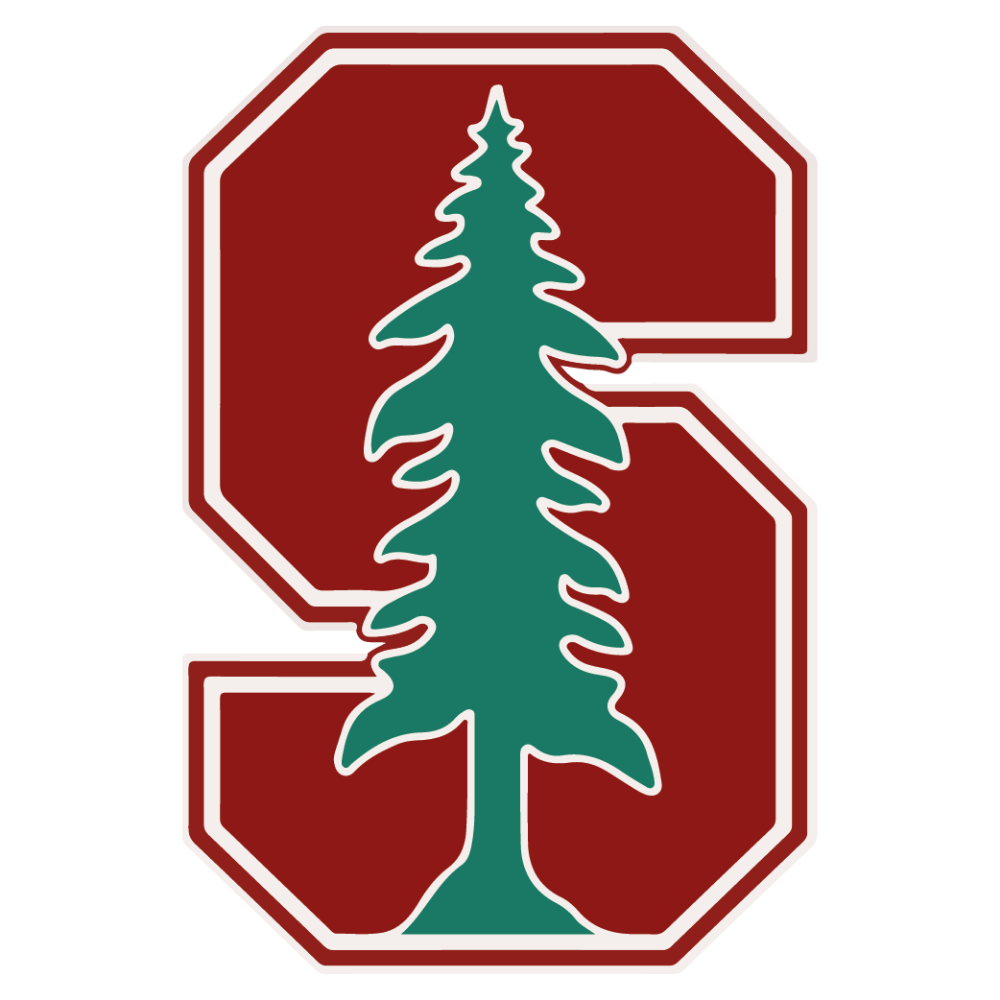
Probabilistic Graphical Models
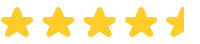
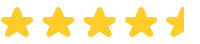
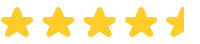
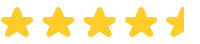
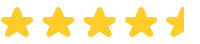
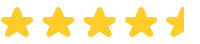
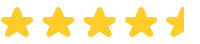
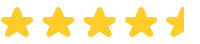
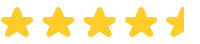
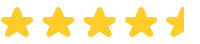
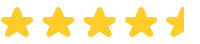
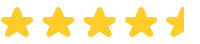
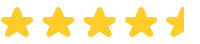
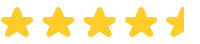
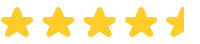
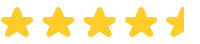
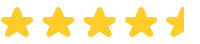
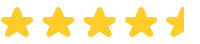
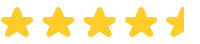
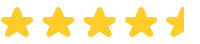
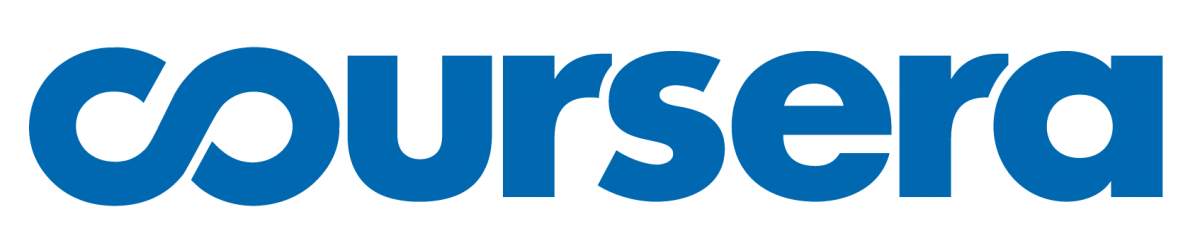
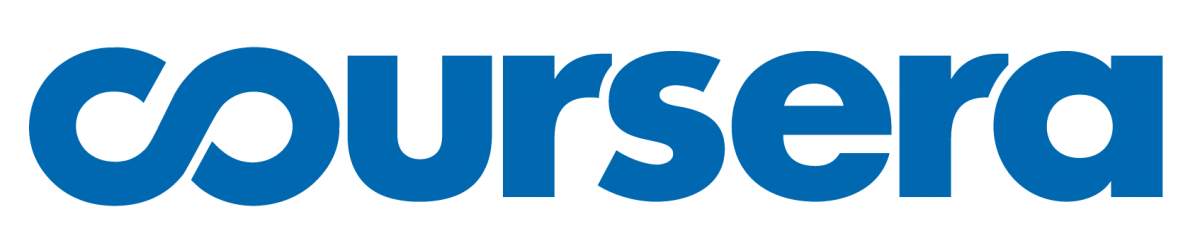
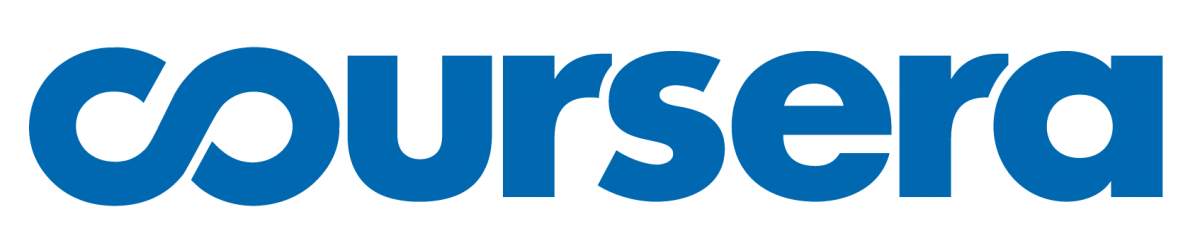
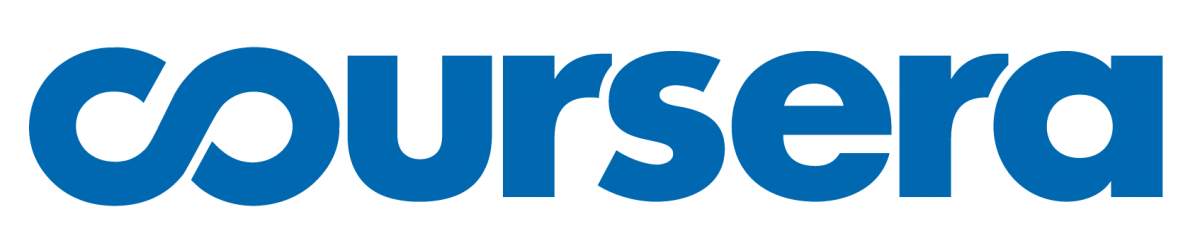
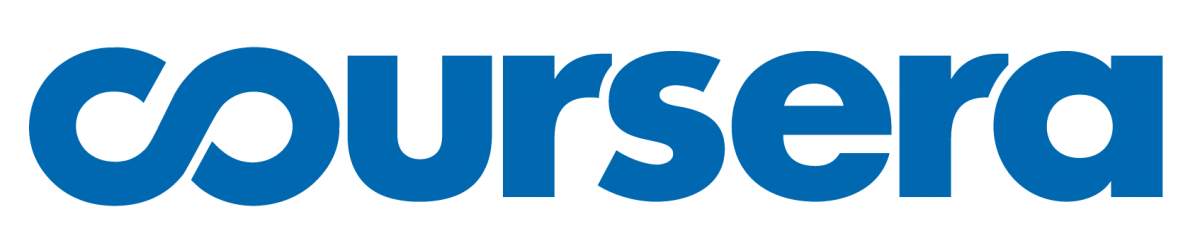
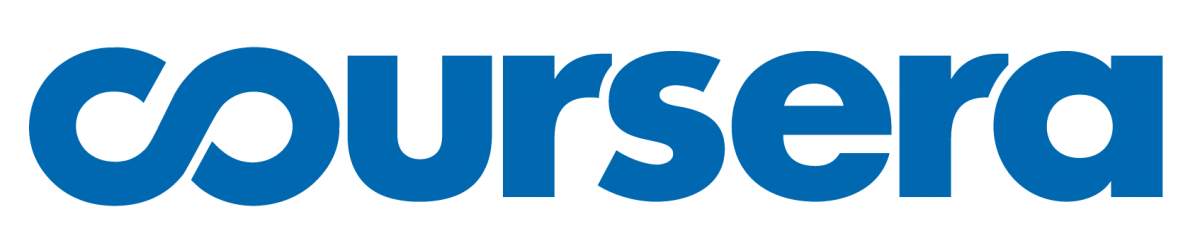
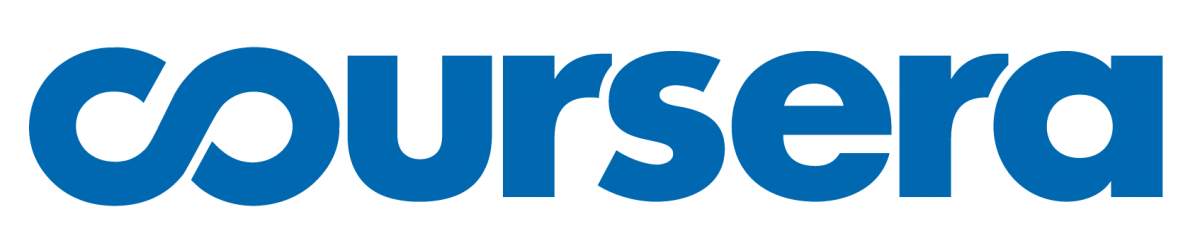
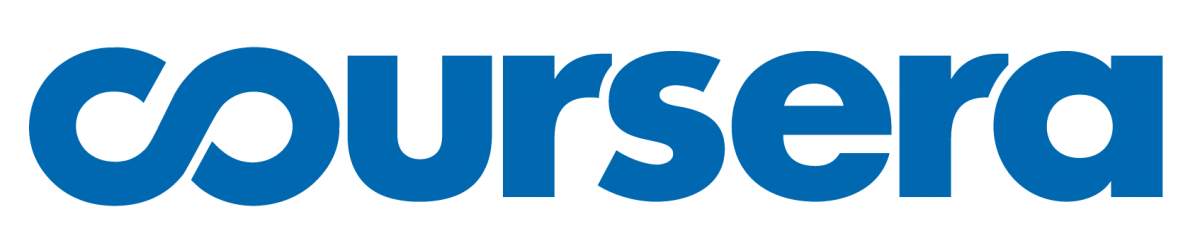
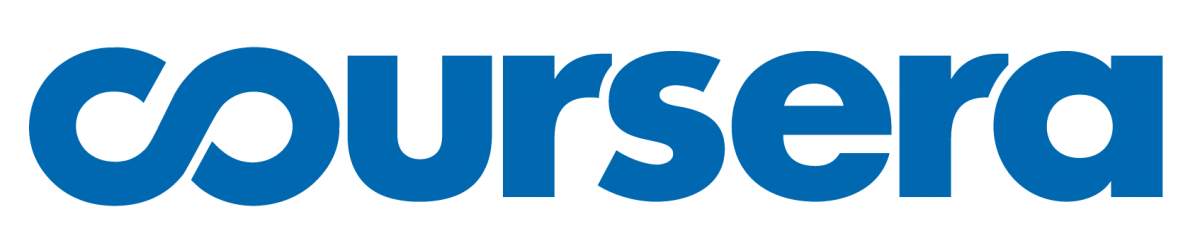
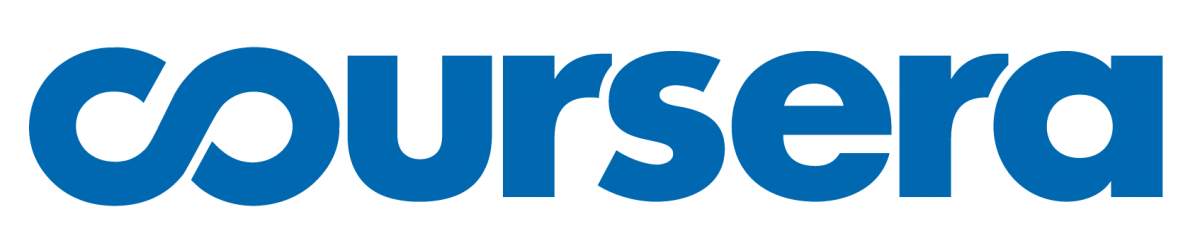
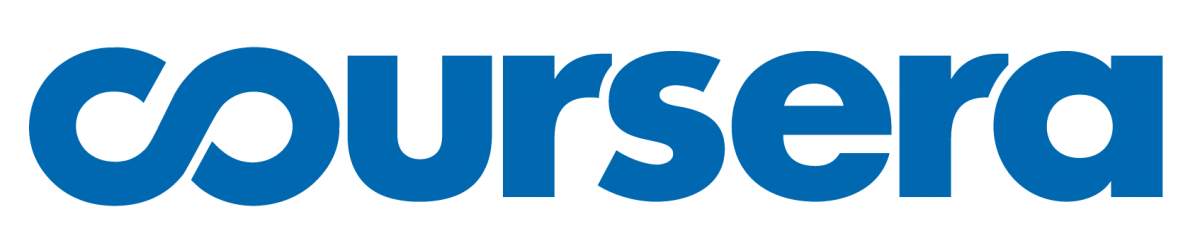
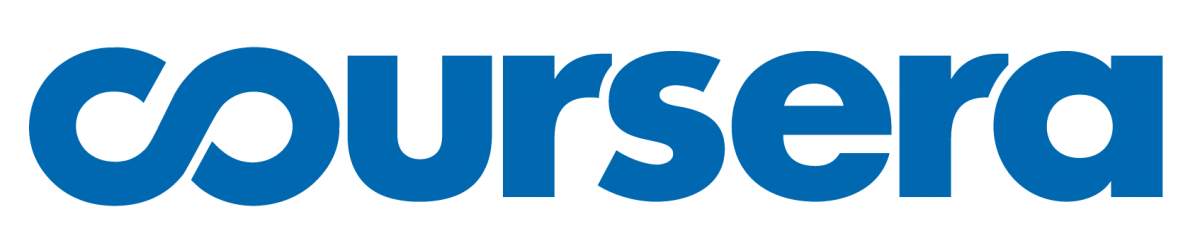
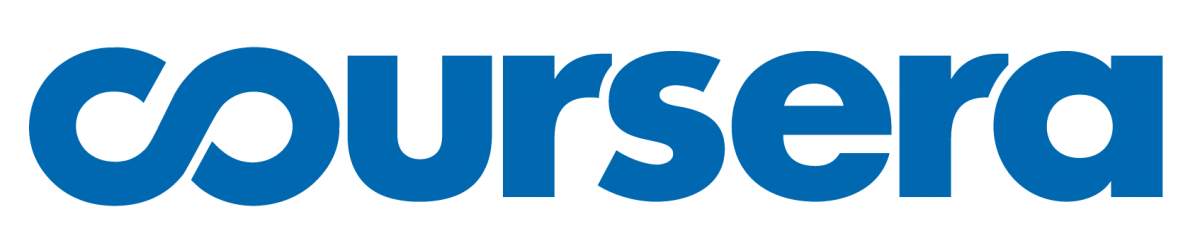
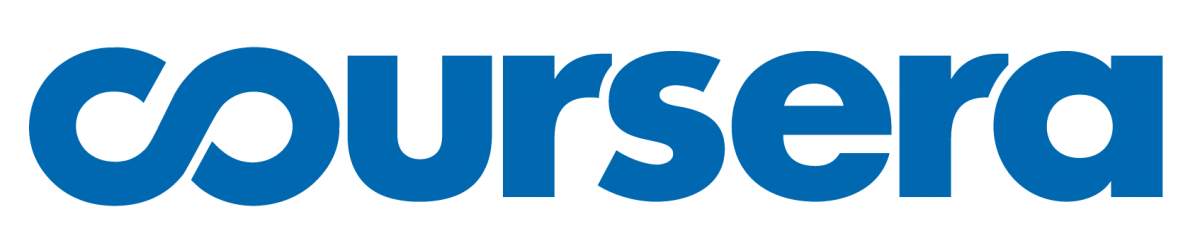
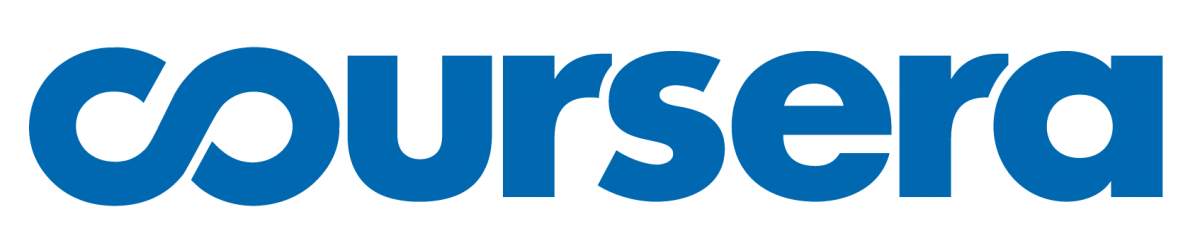
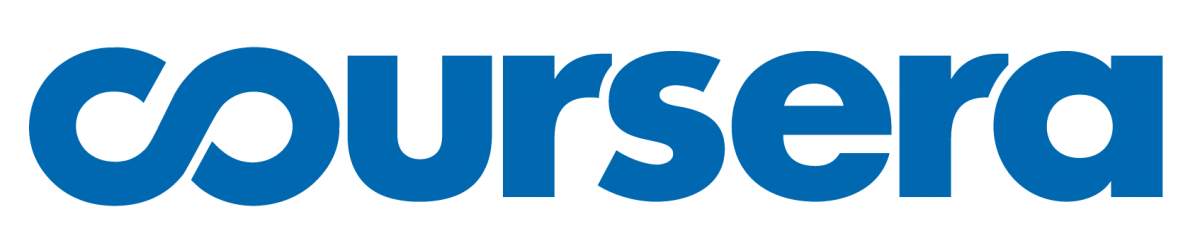
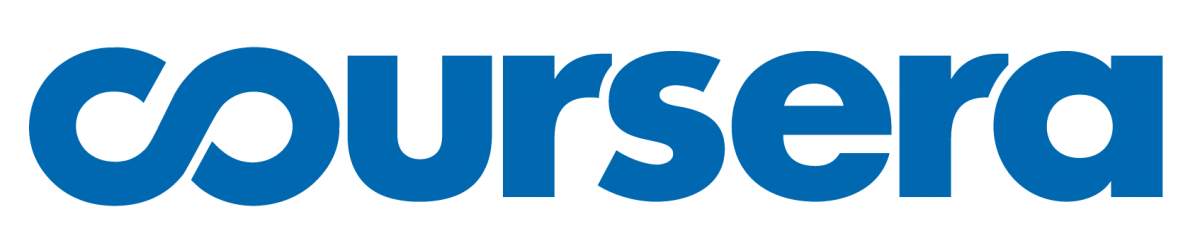
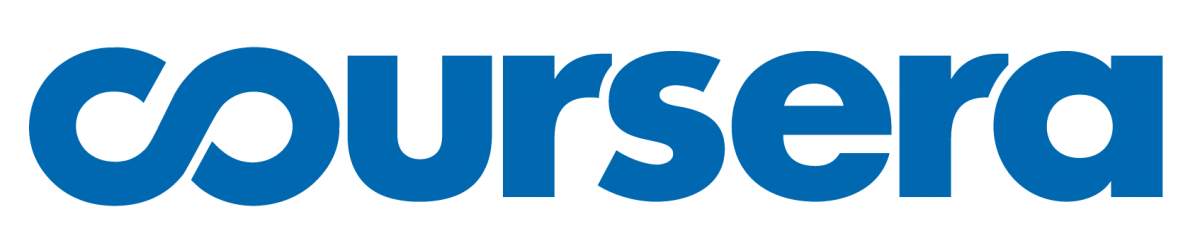
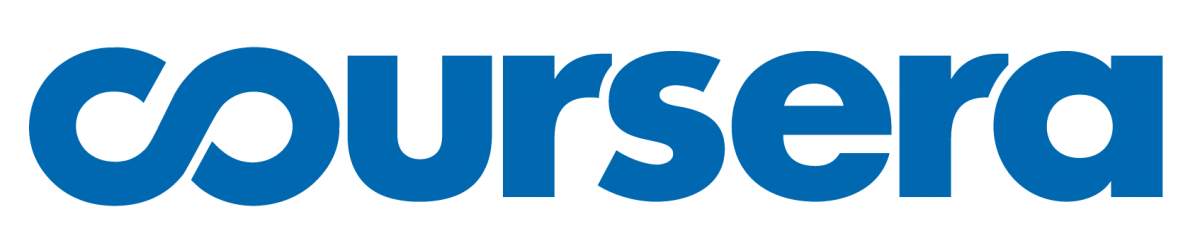
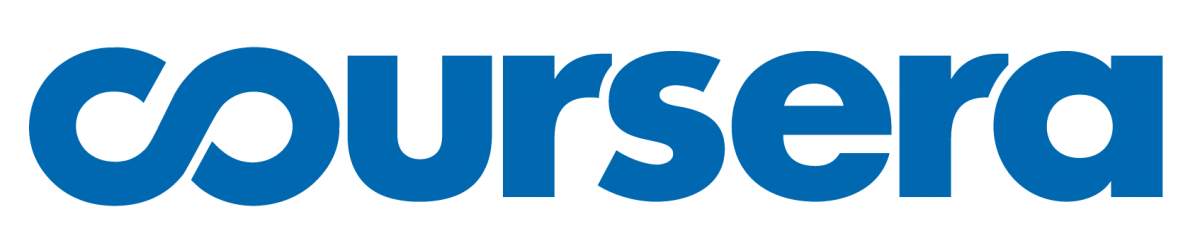
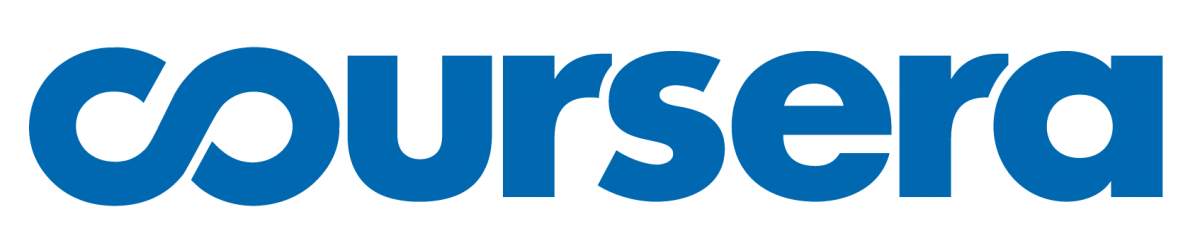
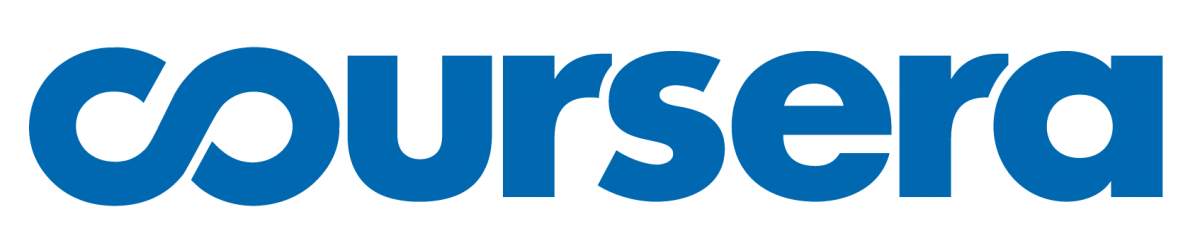
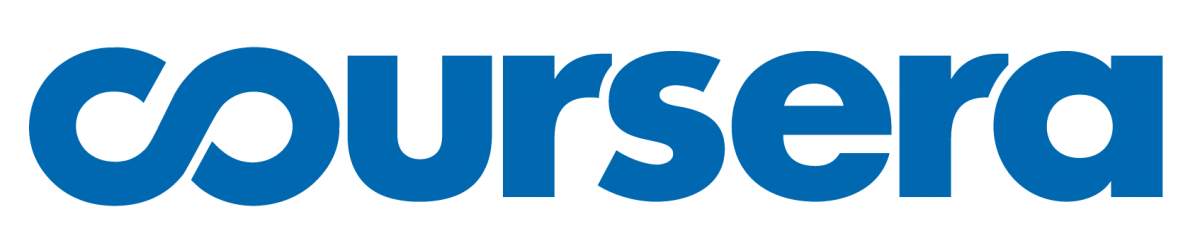
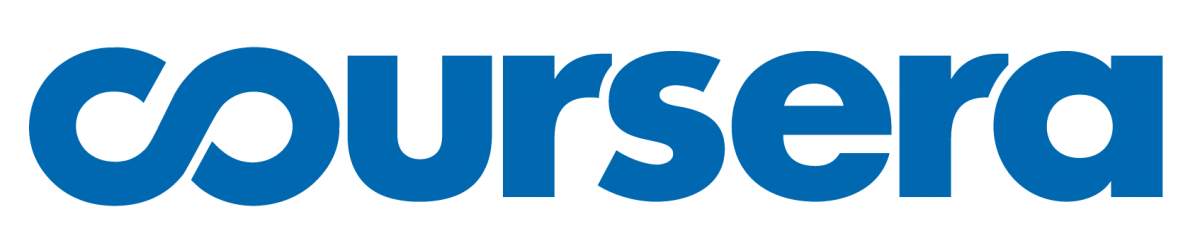
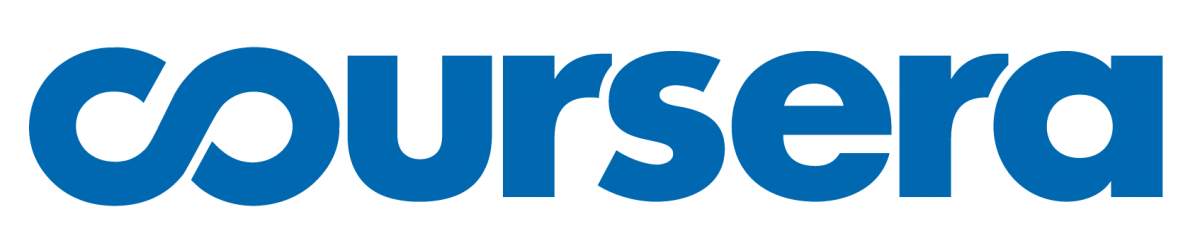
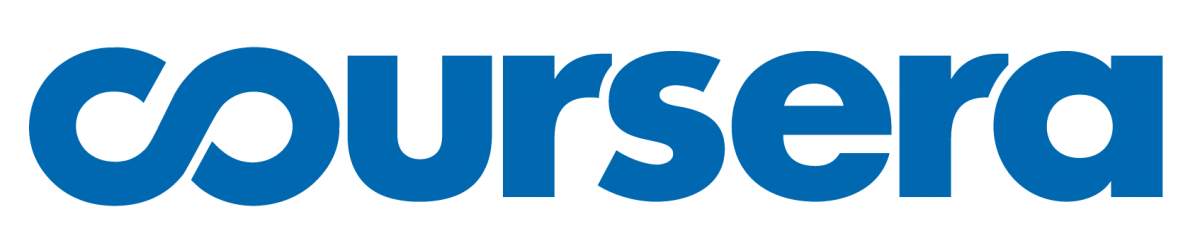
Description
An advanced-level specialization that covers the topic of Probabilistic Graphical Models. Students have the possibility to practice and master the fundamentals of probabilistic graphical models while working with various lectures quizzes , practical assignments and one final applied learning project. The specialization consists of 3 courses.
Teachers:
- Stanford University: Daphne Koller, Professor
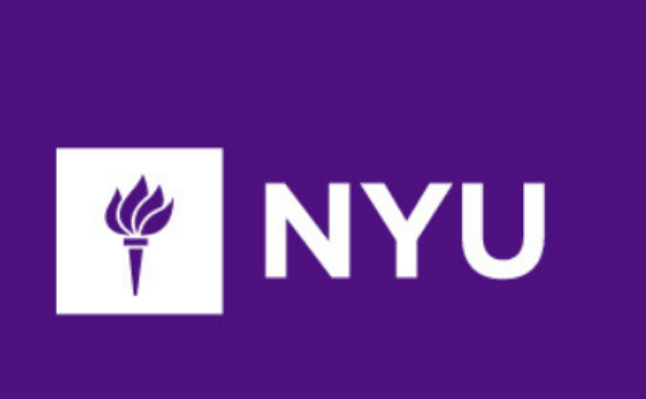
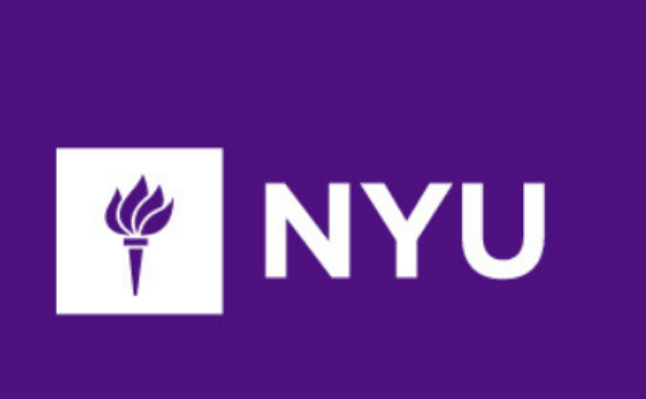
Machine Learning and Reinforcement Learning in Finance
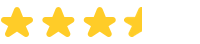
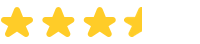
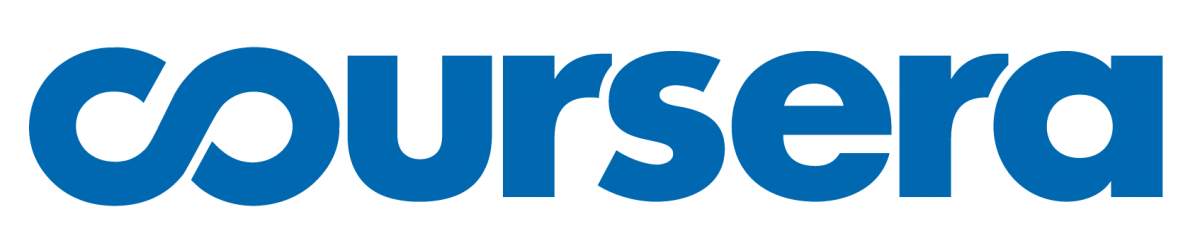
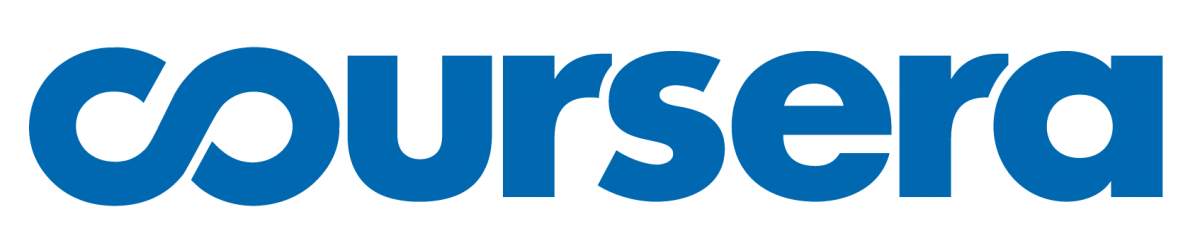
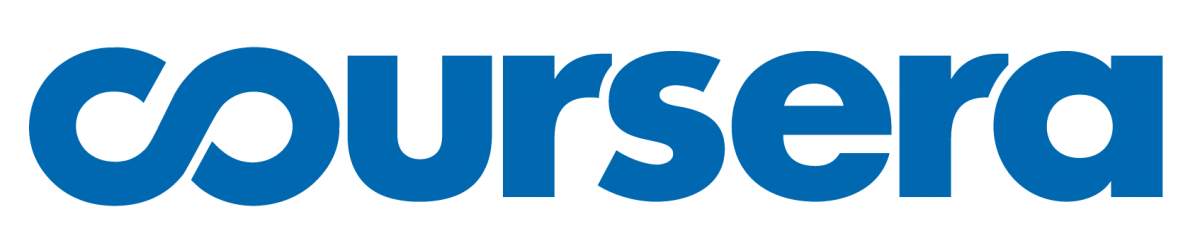
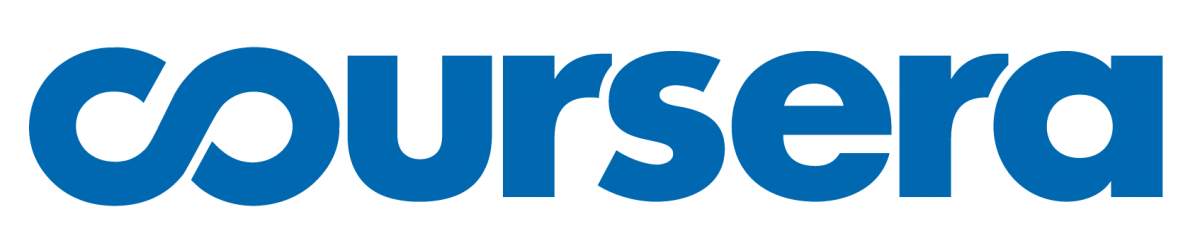
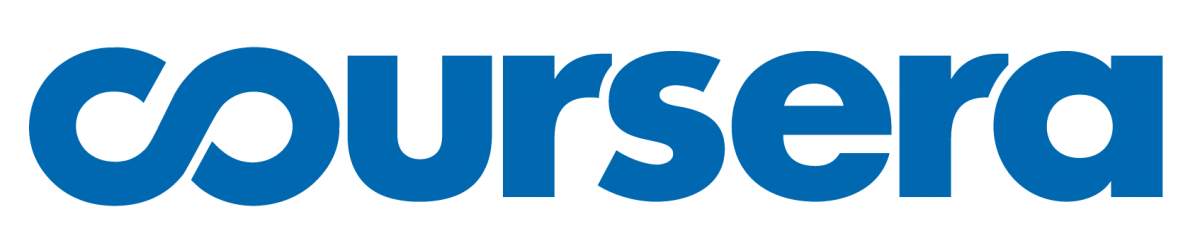
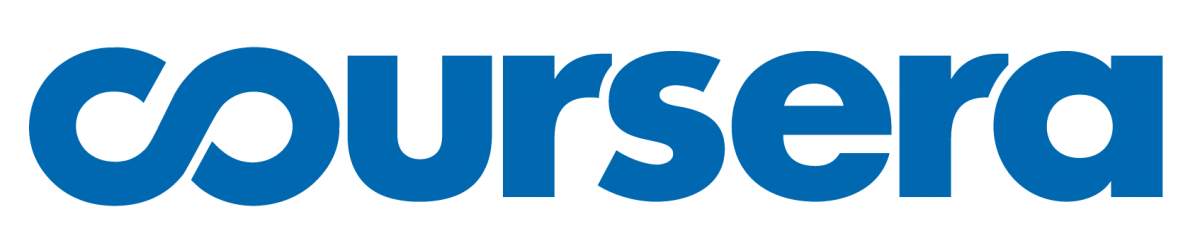
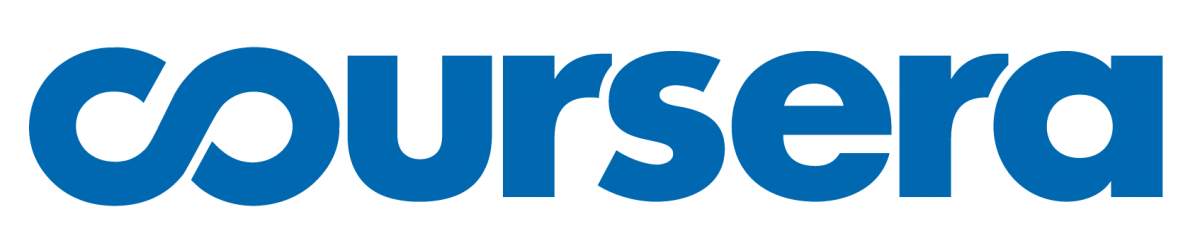
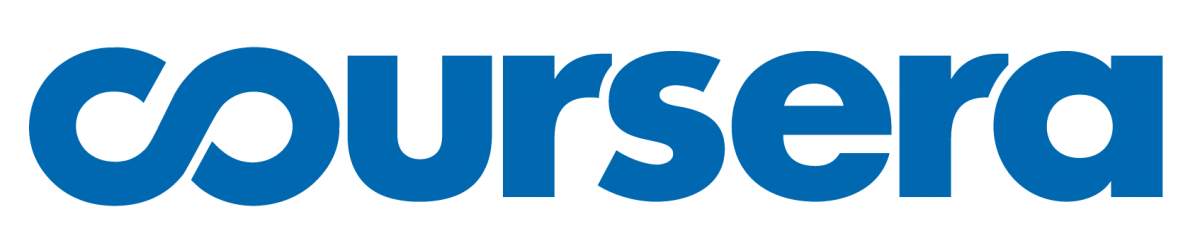
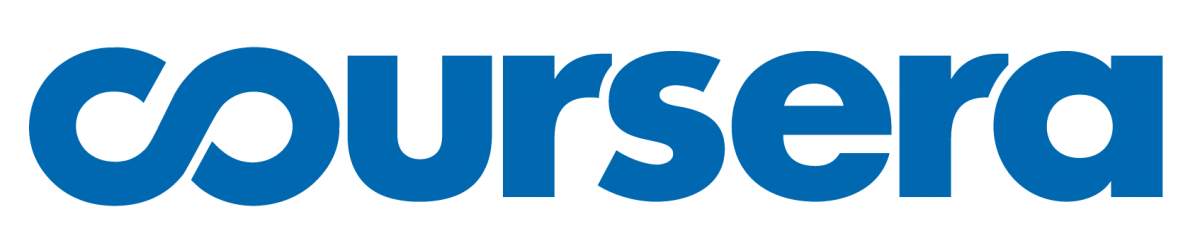
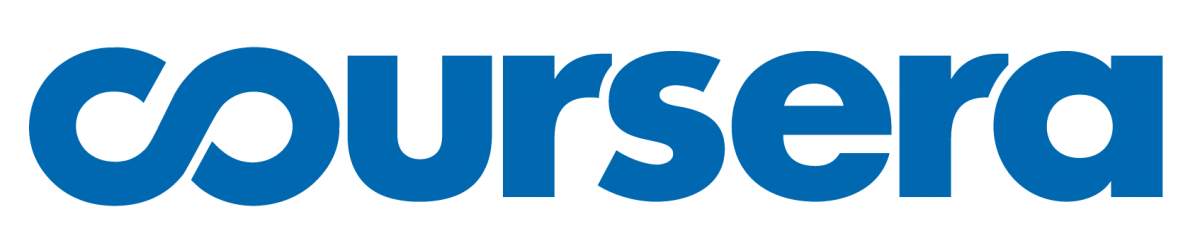
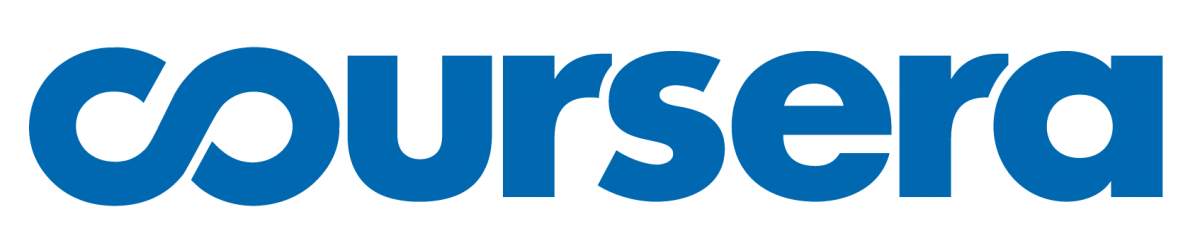
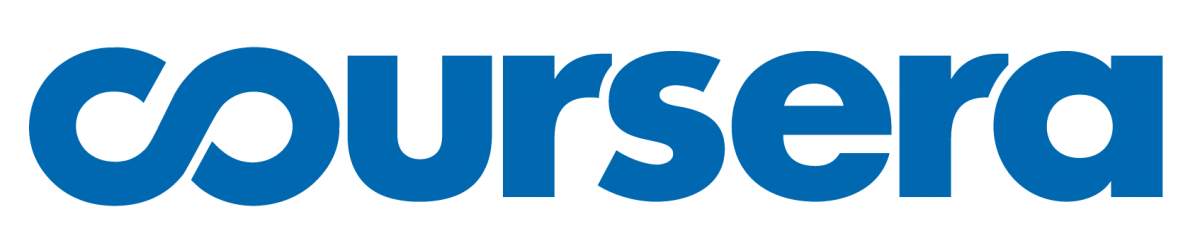
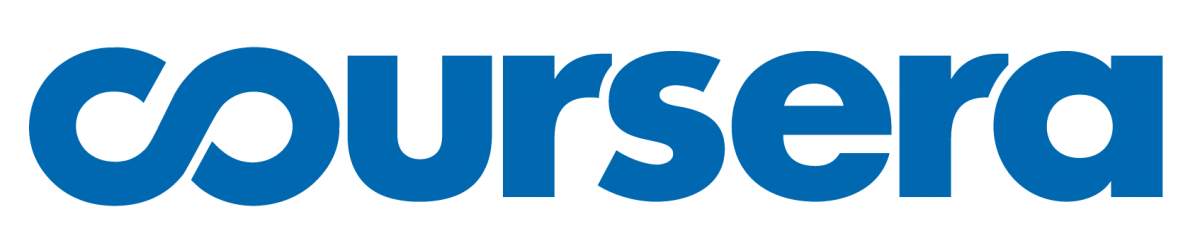
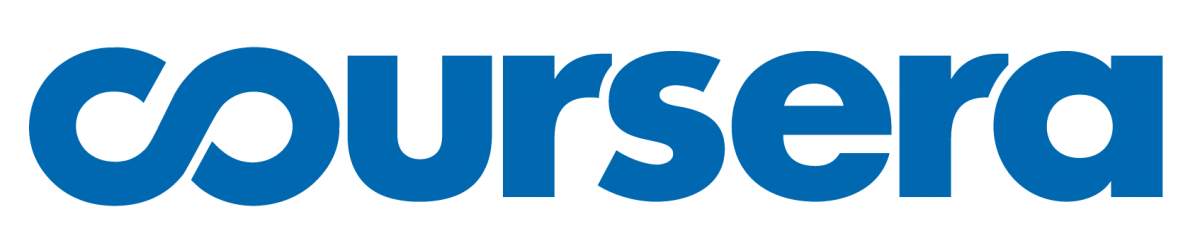
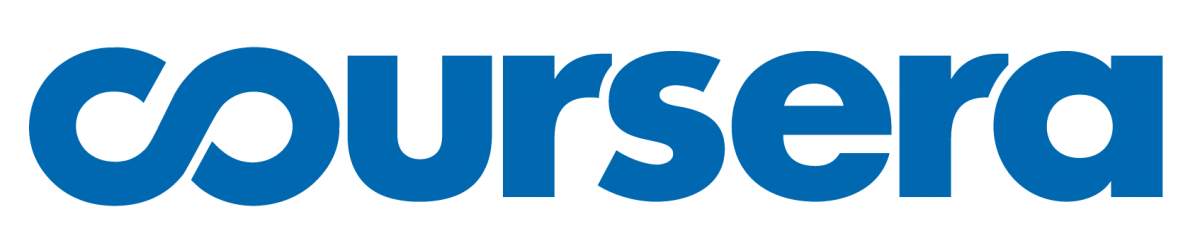
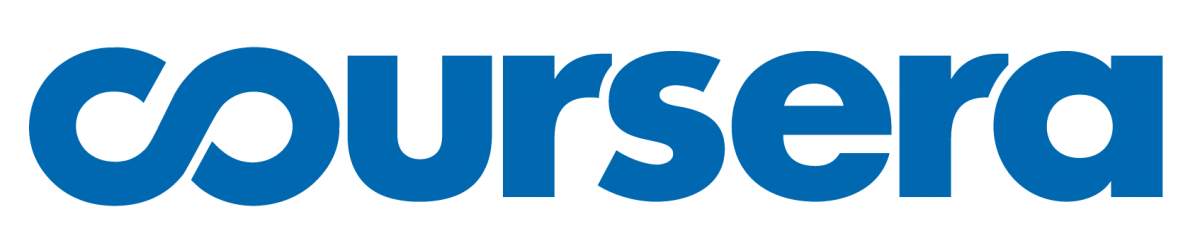
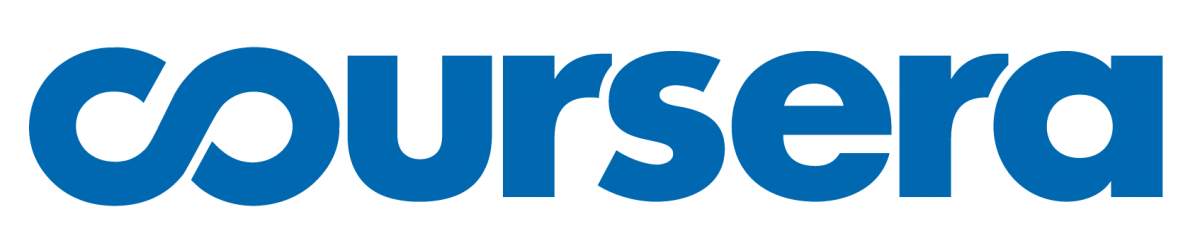
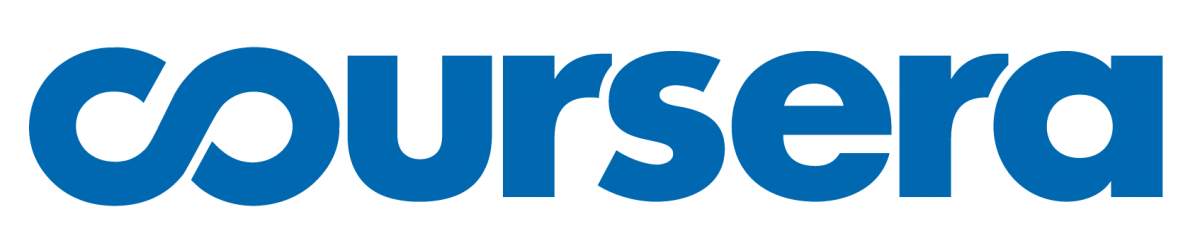
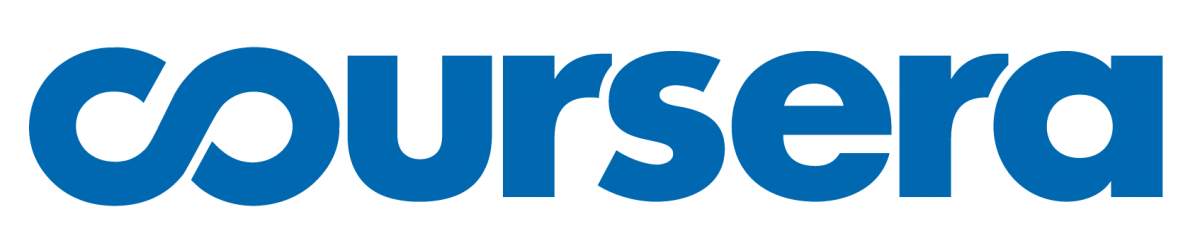
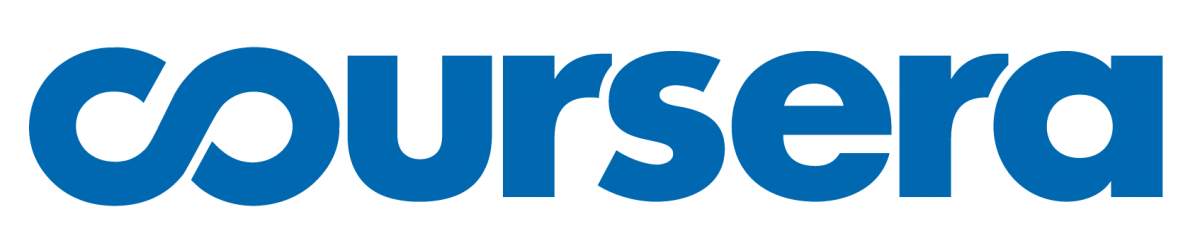
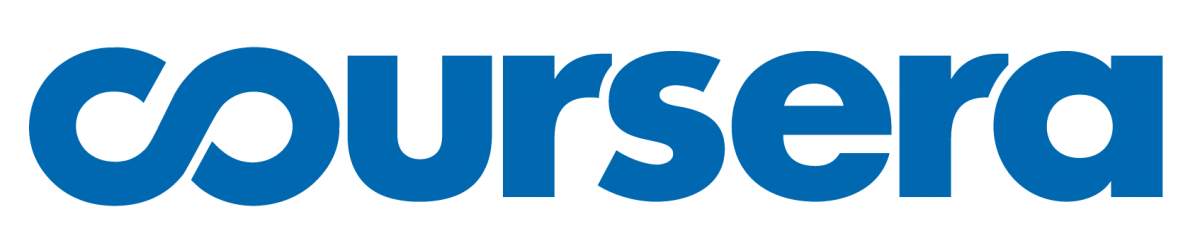
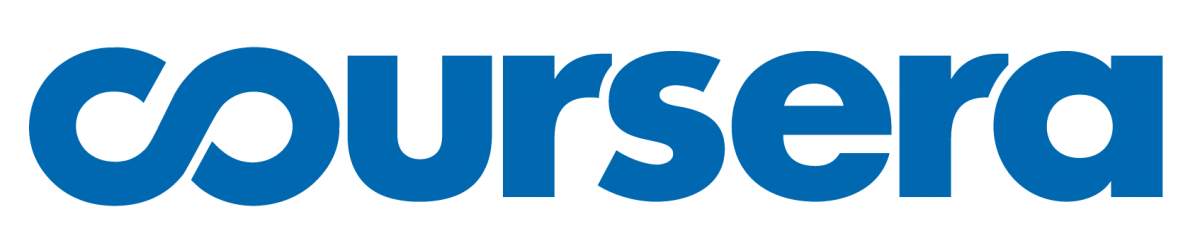
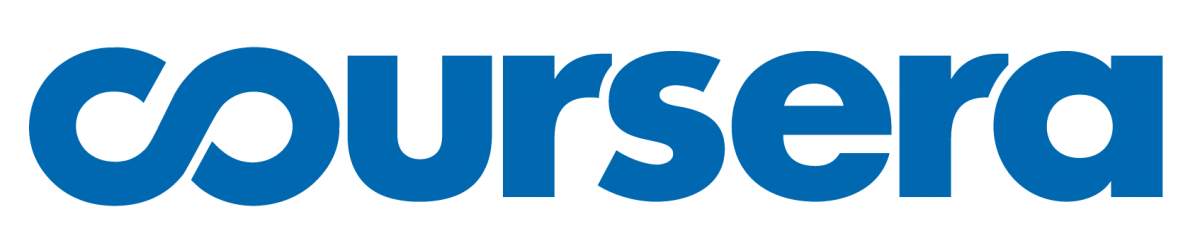
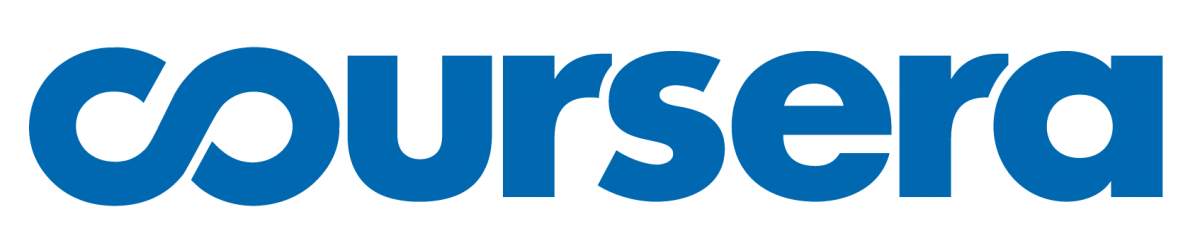
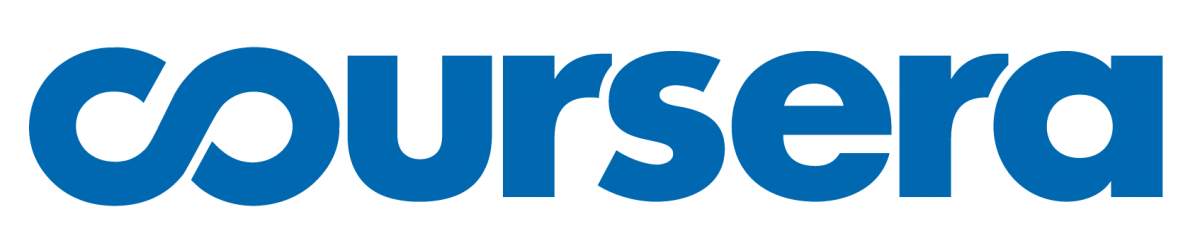
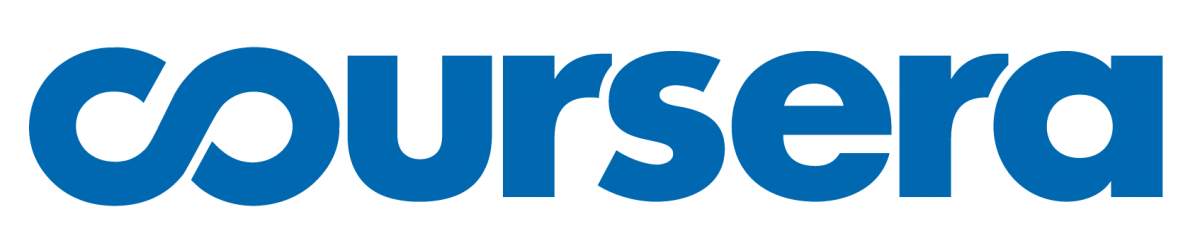
Description
This specialization covers the core topics and paradigms of Machine Learning with special emphasis on practical applications for problems in Finance. Students will learn how to choose the right approach and ML method for a particular finance problem and will also learn how to find and implement solutions to these problems.
Teachers:
- NYU: Igor Halperin
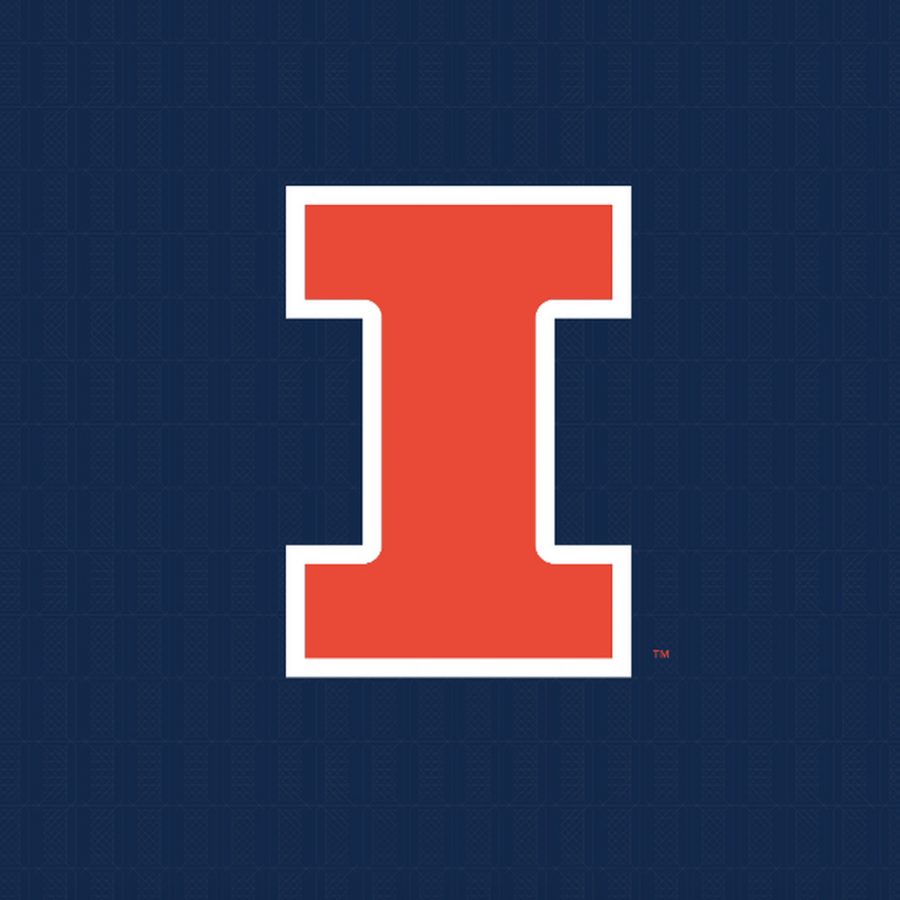
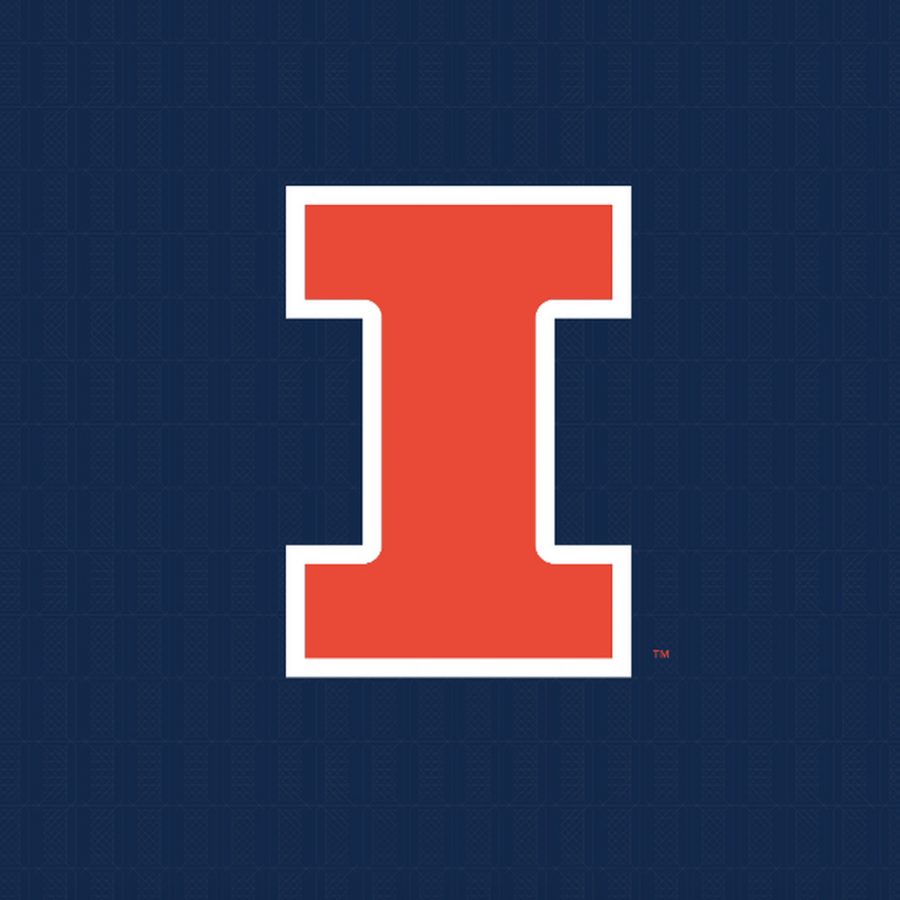
Data Mining
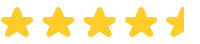
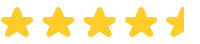
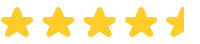
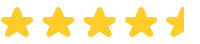
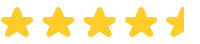
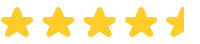
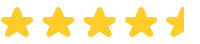
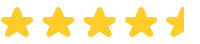
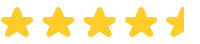
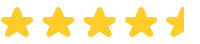
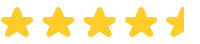
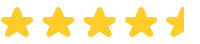
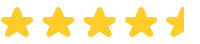
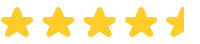
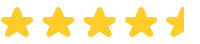
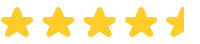
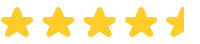
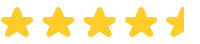
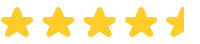
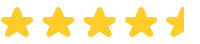
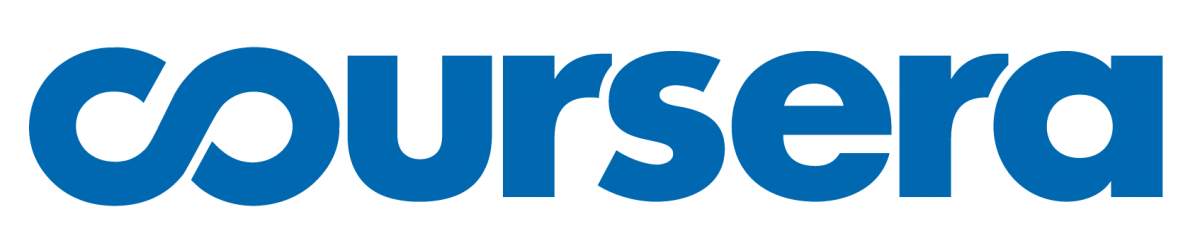
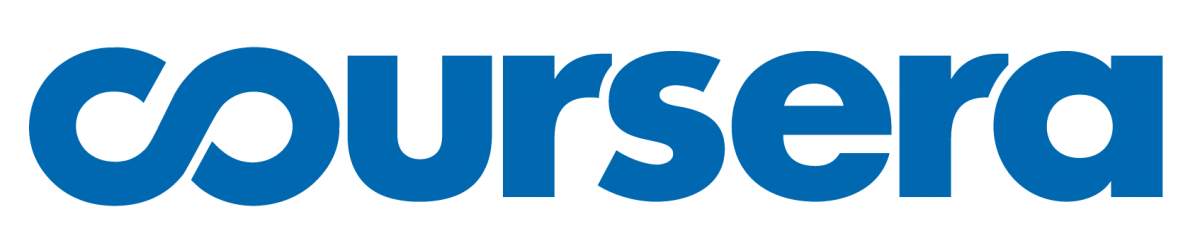
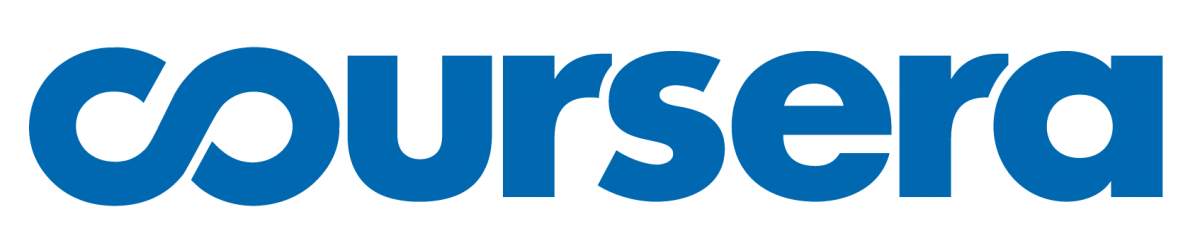
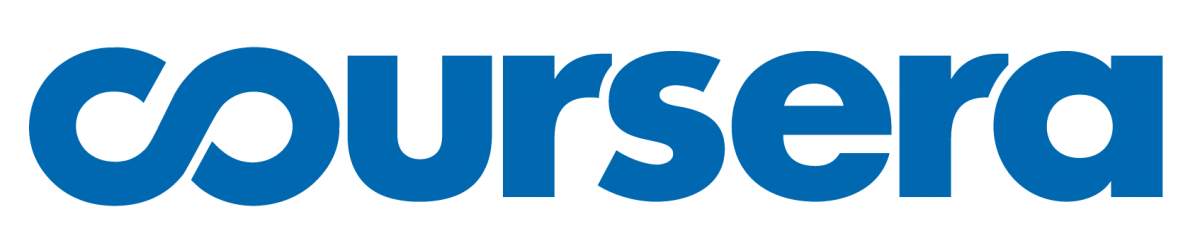
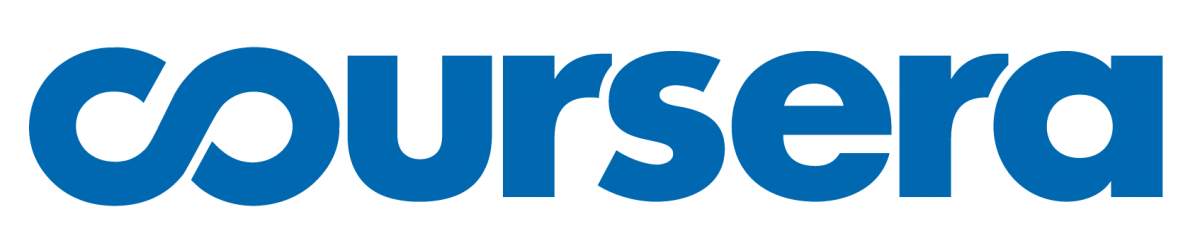
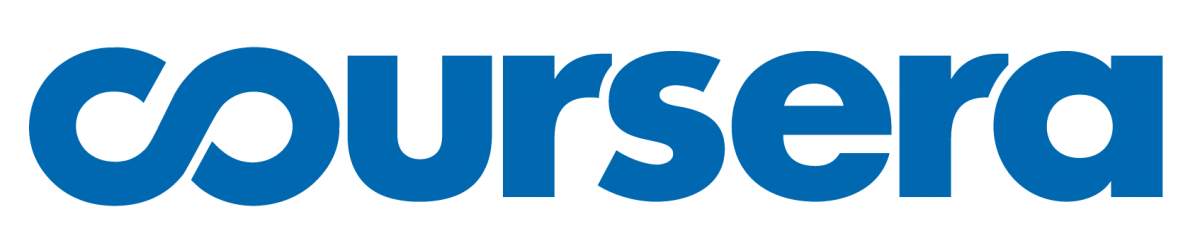
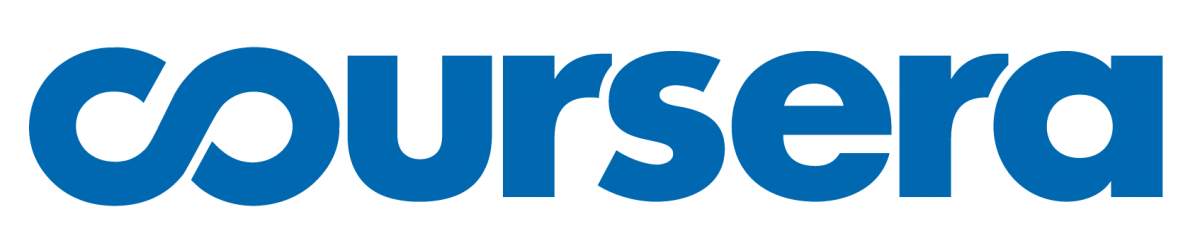
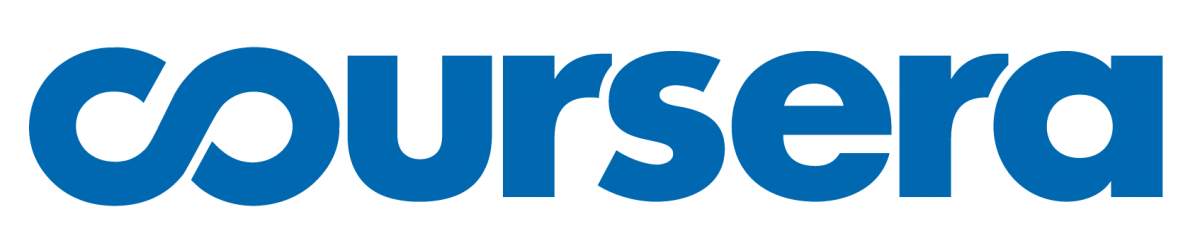
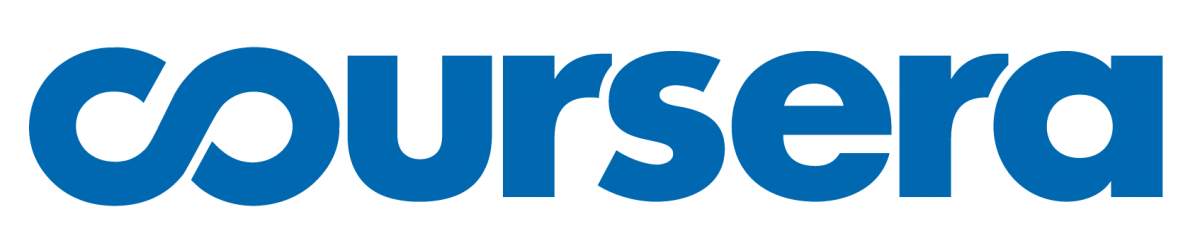
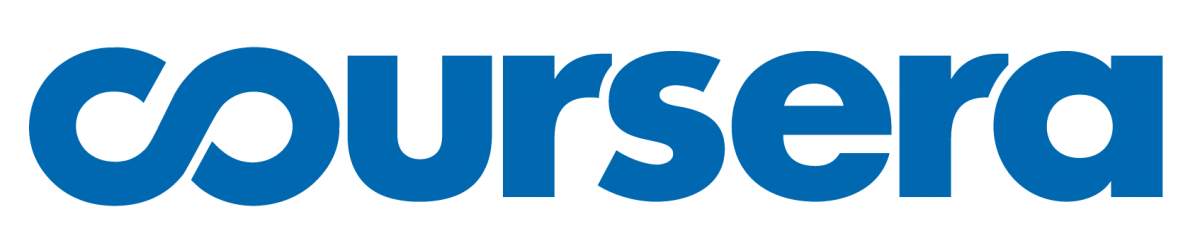
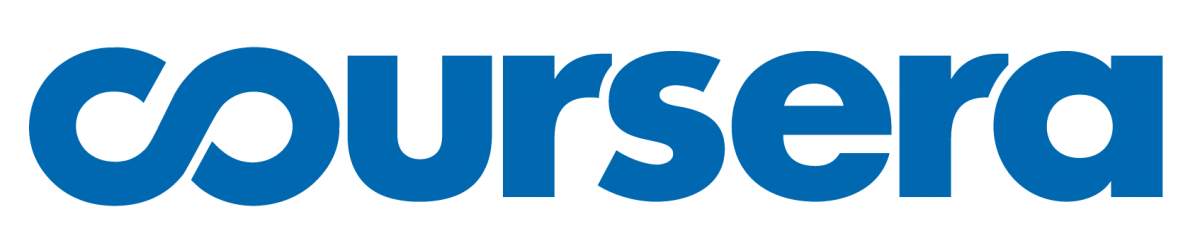
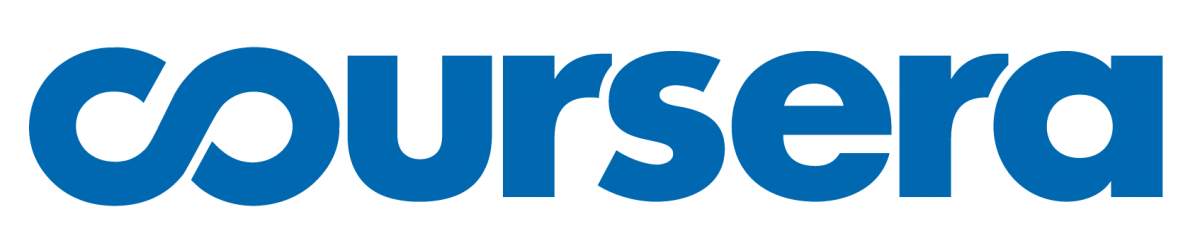
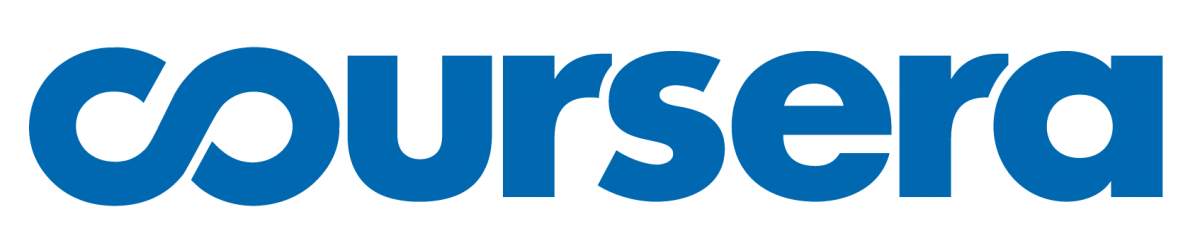
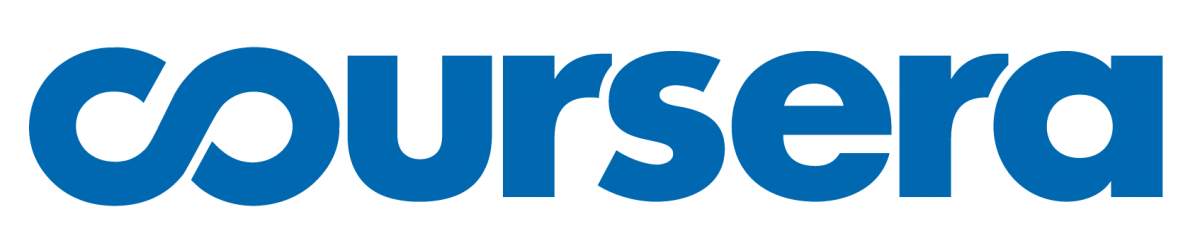
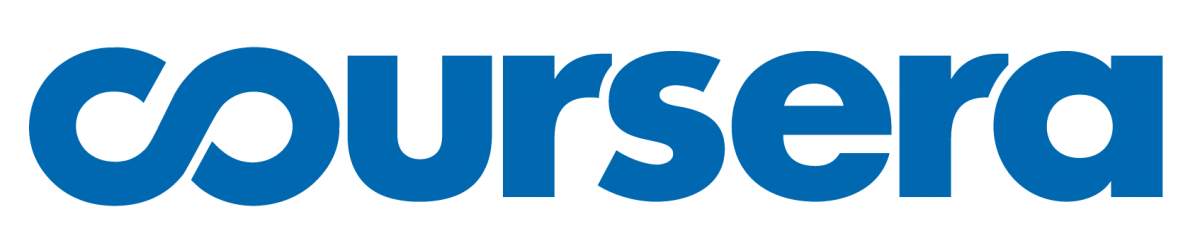
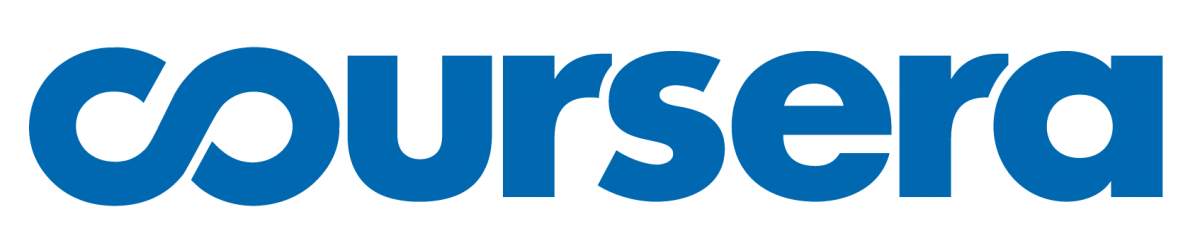
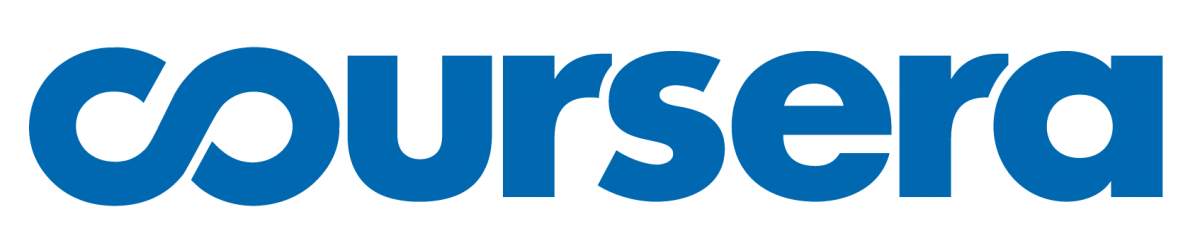
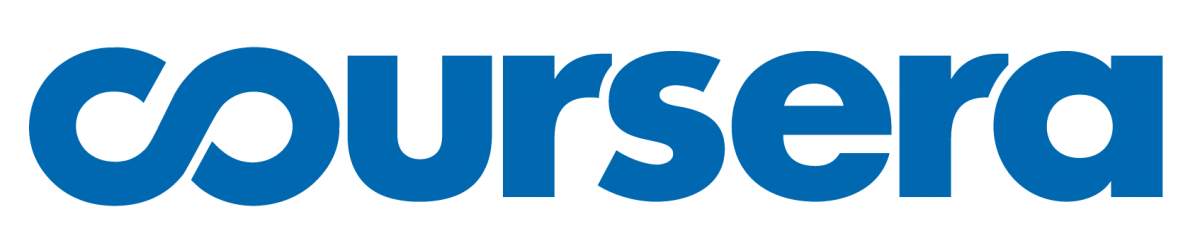
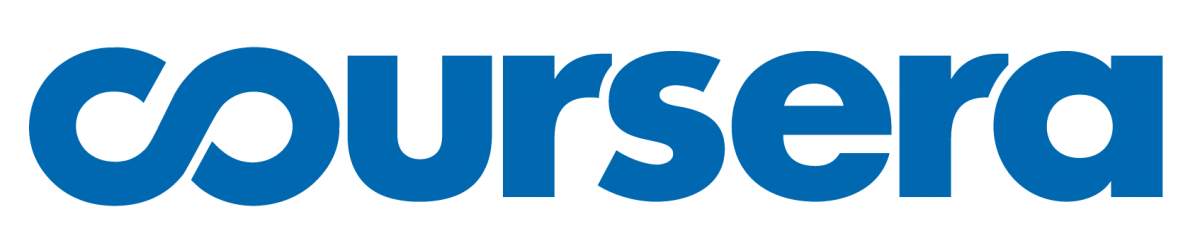
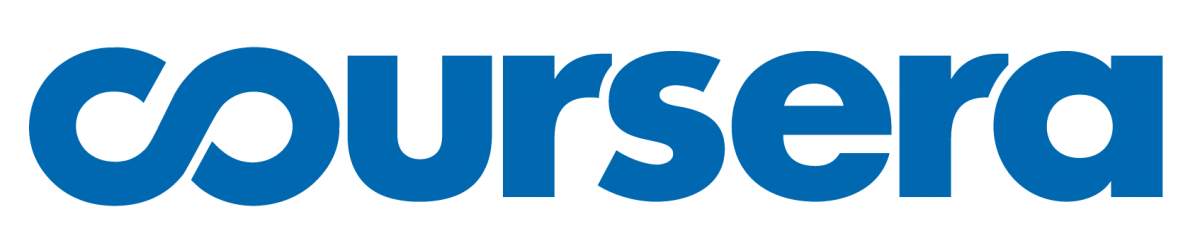
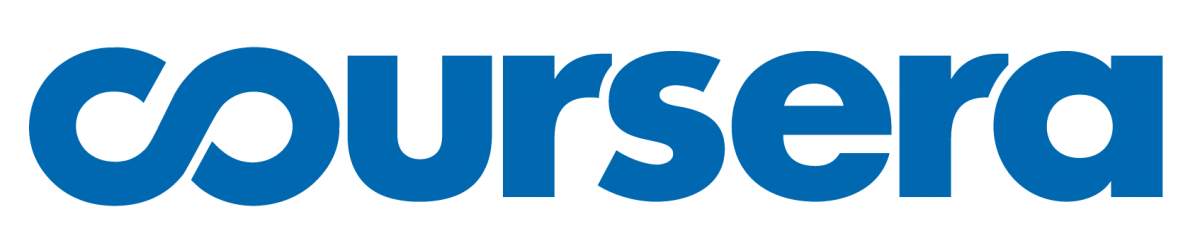
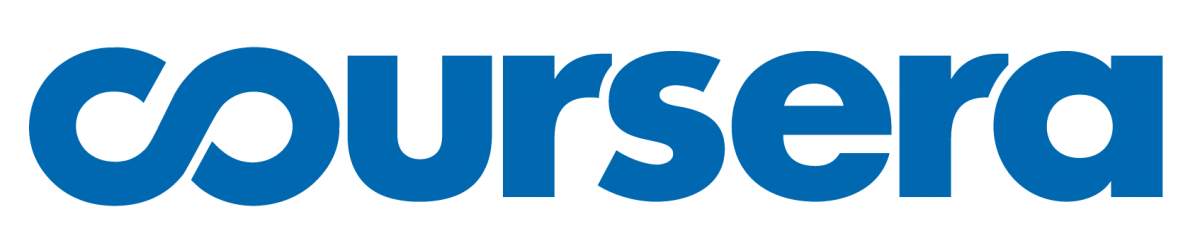
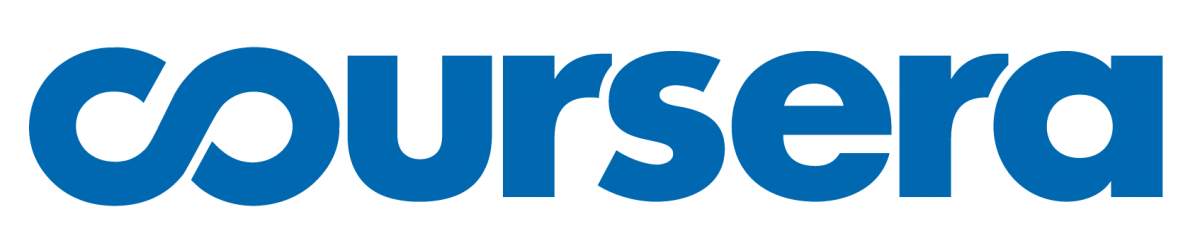
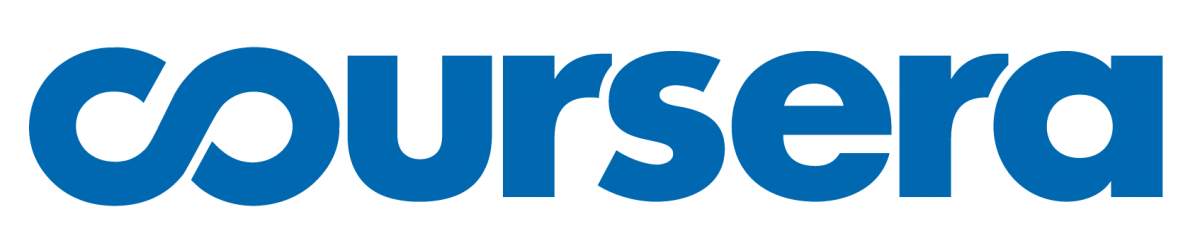
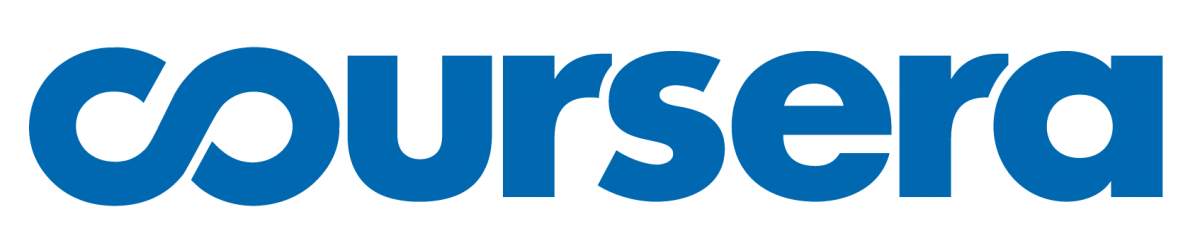
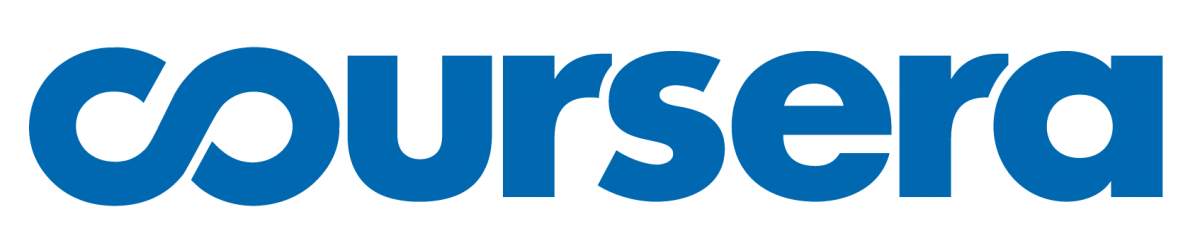
Description
In this intermediate-level specialization students will learn about: pattern discovery, clustering, text retrieval, text mining and analytics, and data visualization. It consists of 6 courses throughout which students learn how to handle both structured and unstructured data in the framework of data mining. The specialization also offers one capstone project where the task is to solve a real-world problem using data mining techniques.
Teachers:
- University of Illionis: John C. Hart, Professor of Computer Science
- University of Illionis: ChengXiang Zhai, Professor
- University of Illionis: Jiawei Han, Professor
- University of Illionis: Abel Bliss, Professor
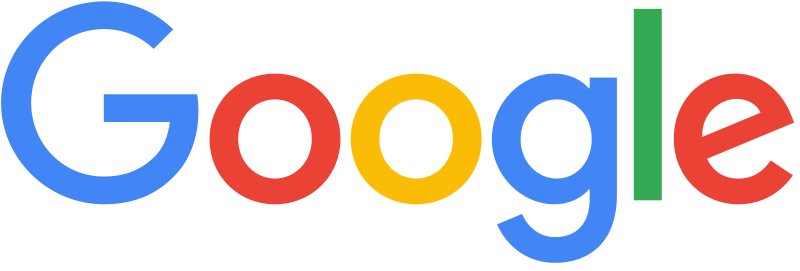
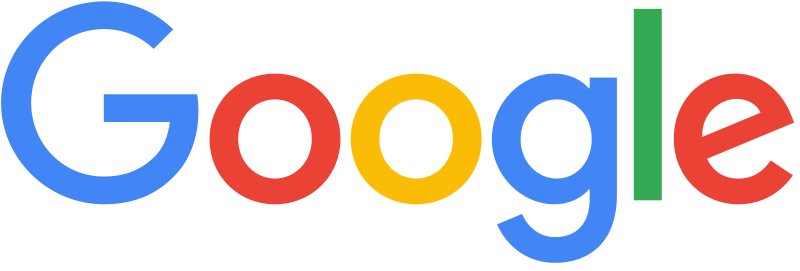
Machine Learning with TensorFlow on Google Cloud Platform
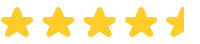
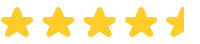
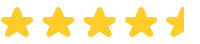
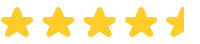
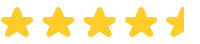
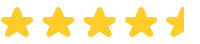
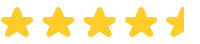
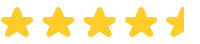
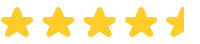
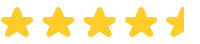
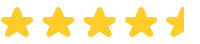
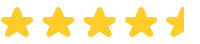
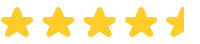
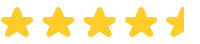
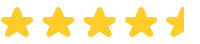
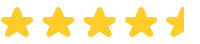
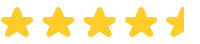
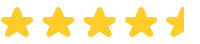
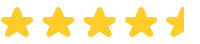
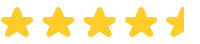
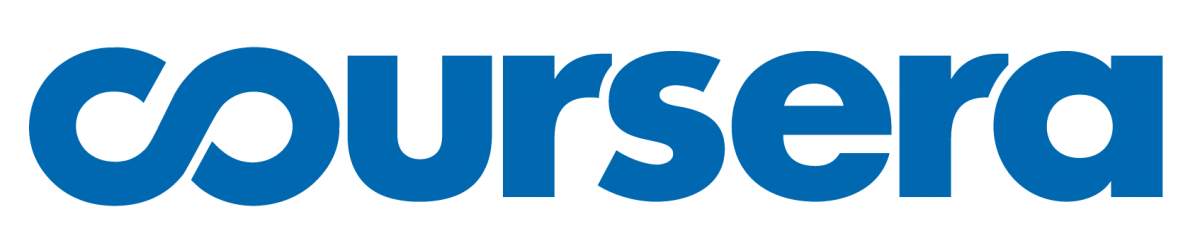
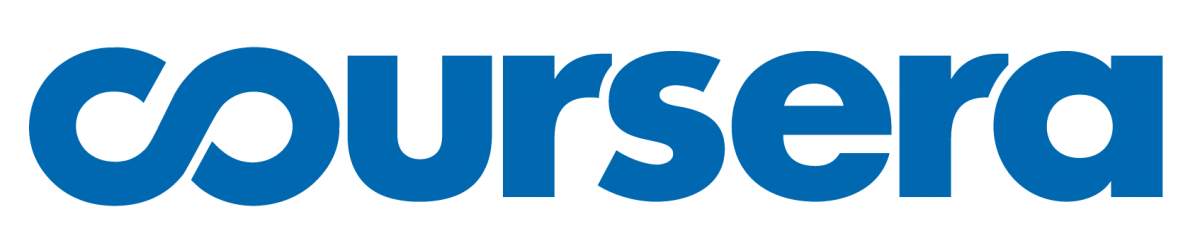
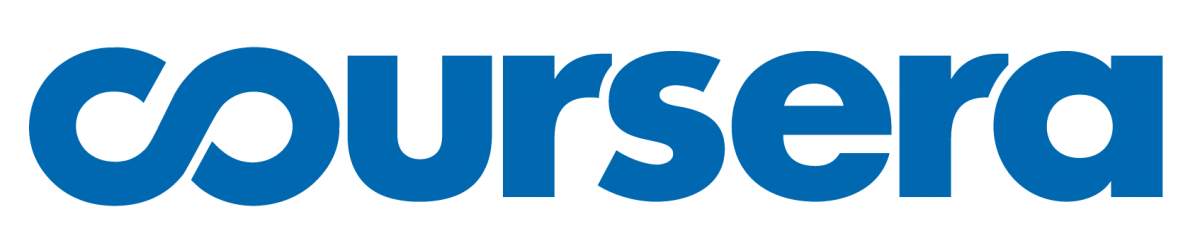
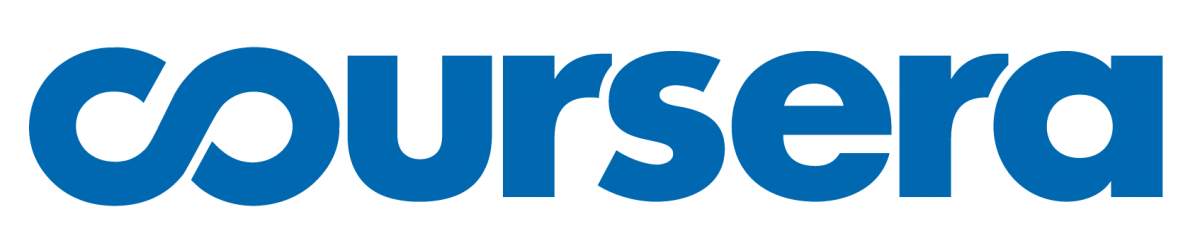
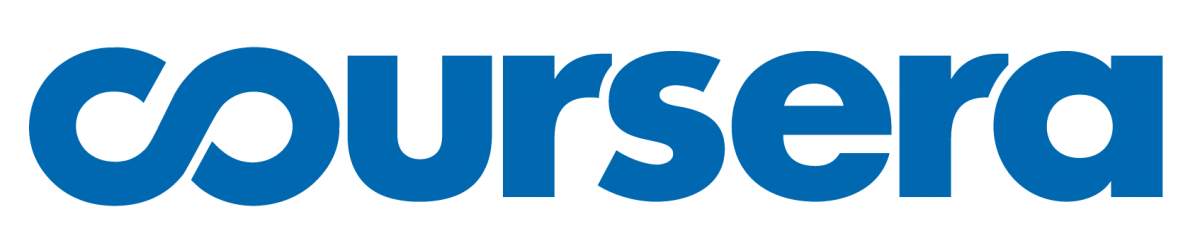
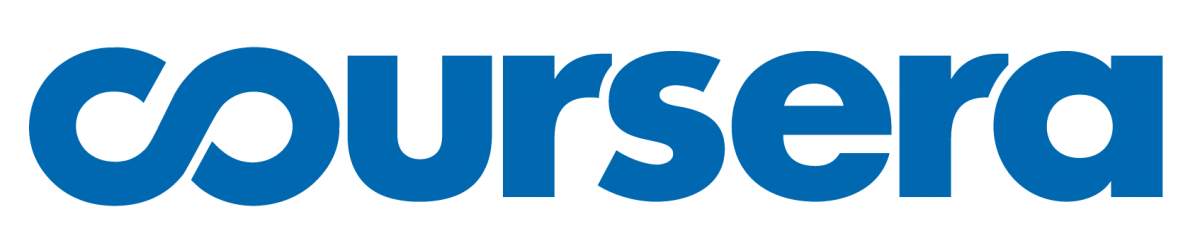
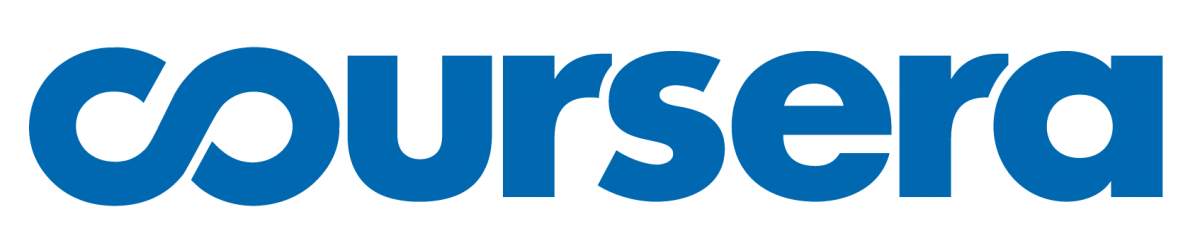
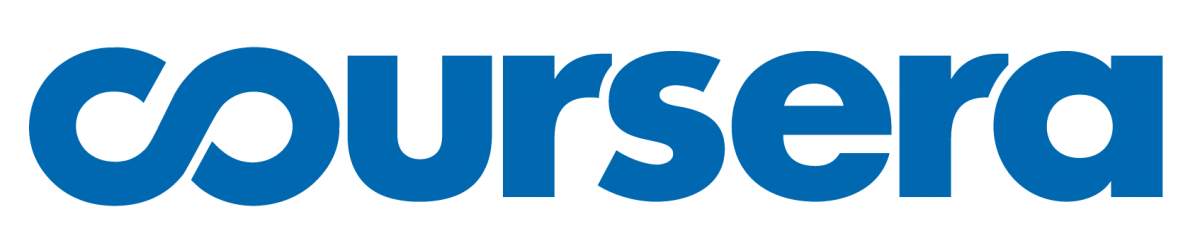
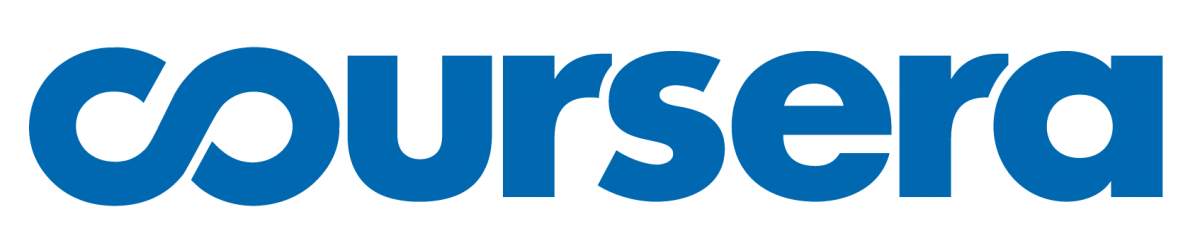
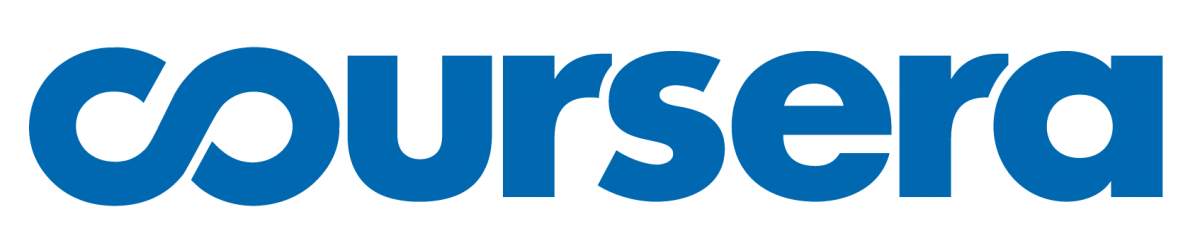
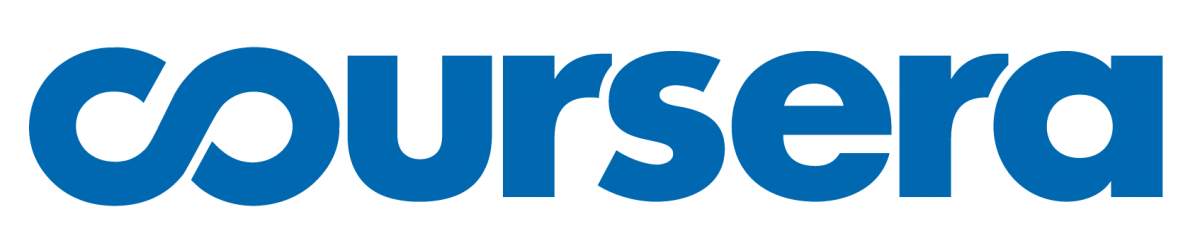
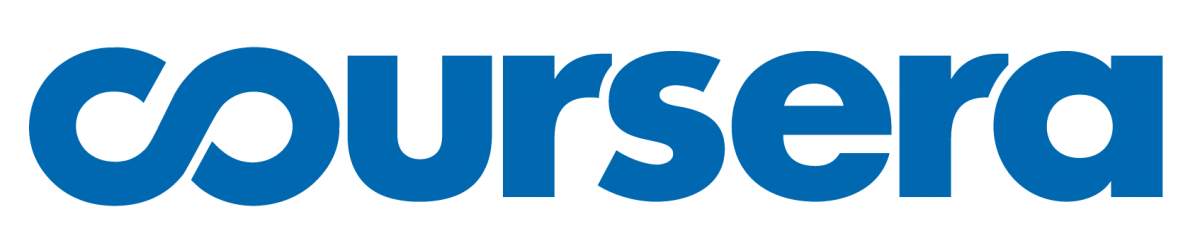
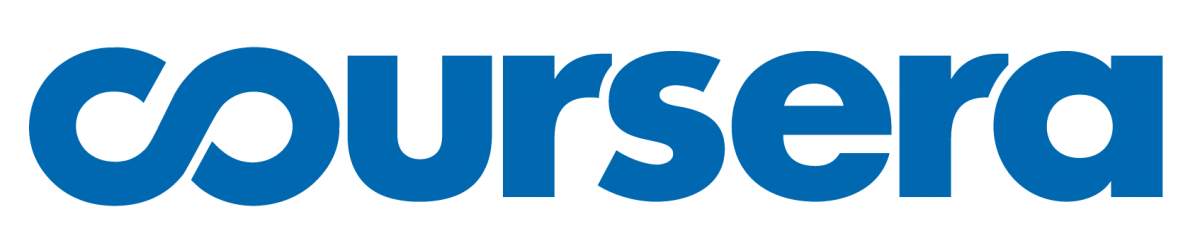
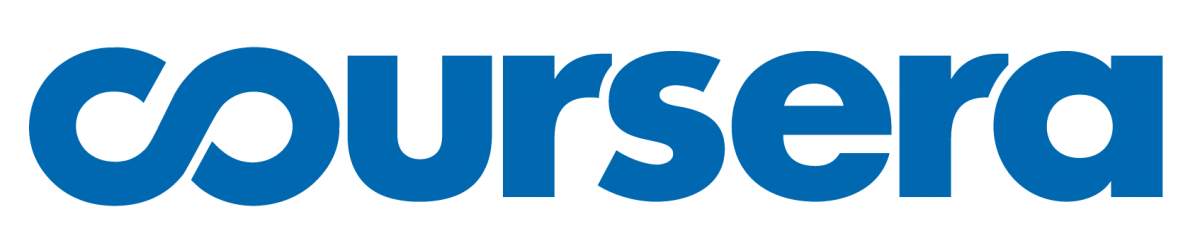
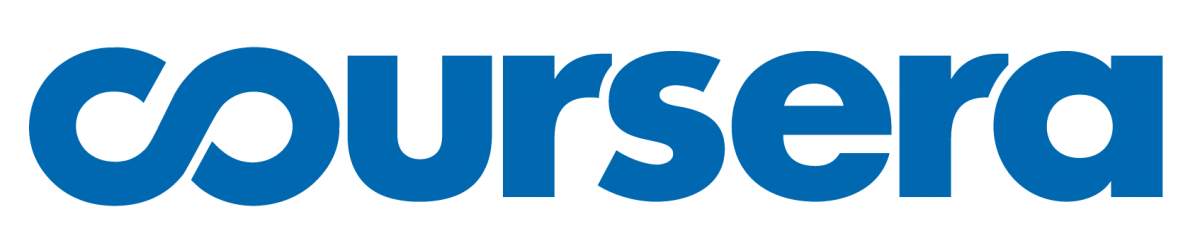
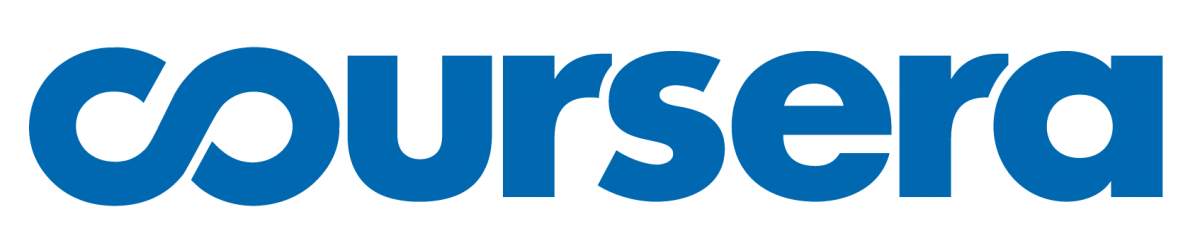
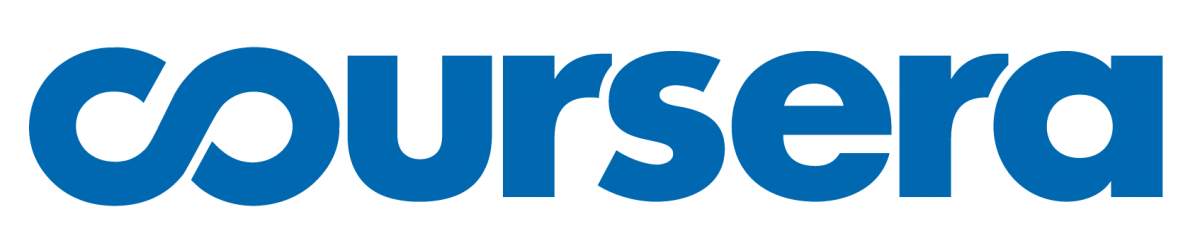
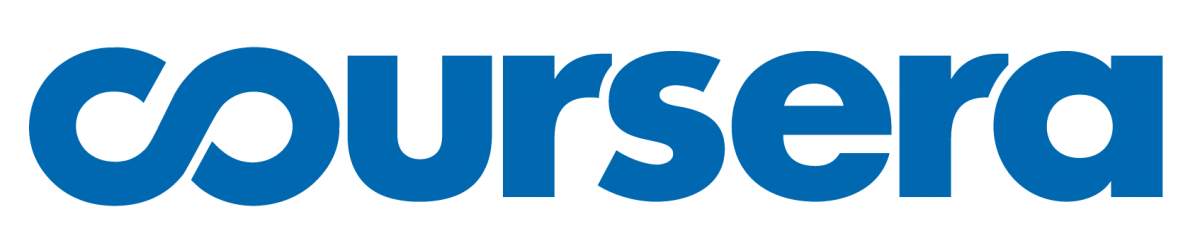
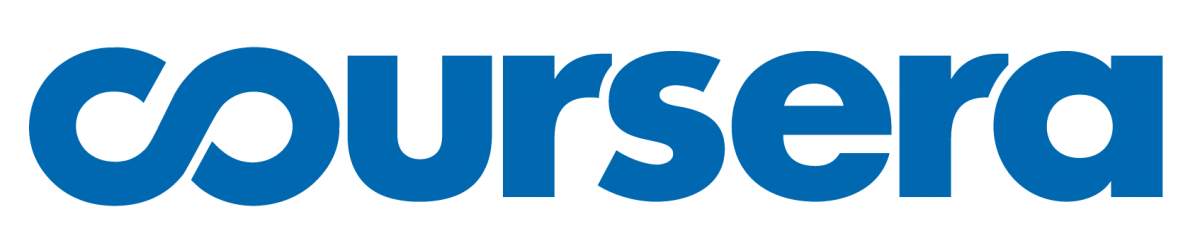
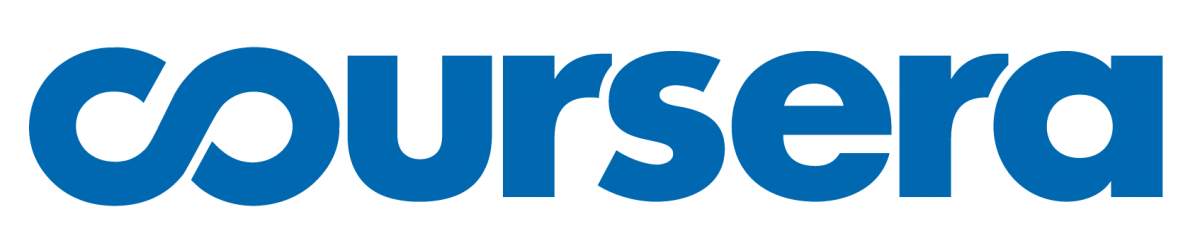
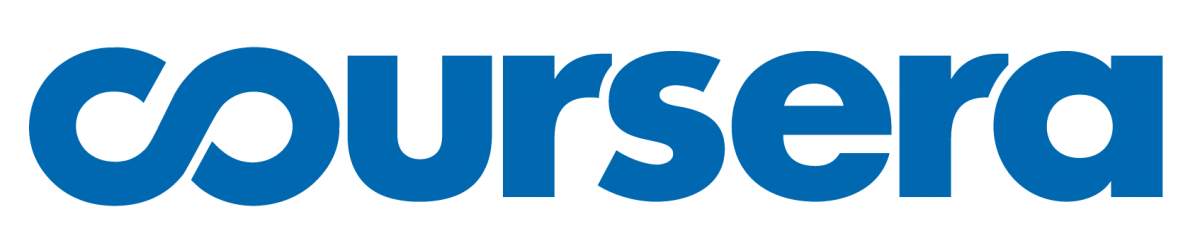
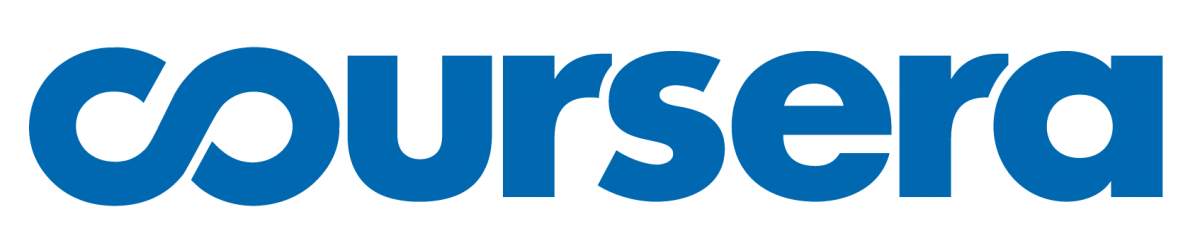
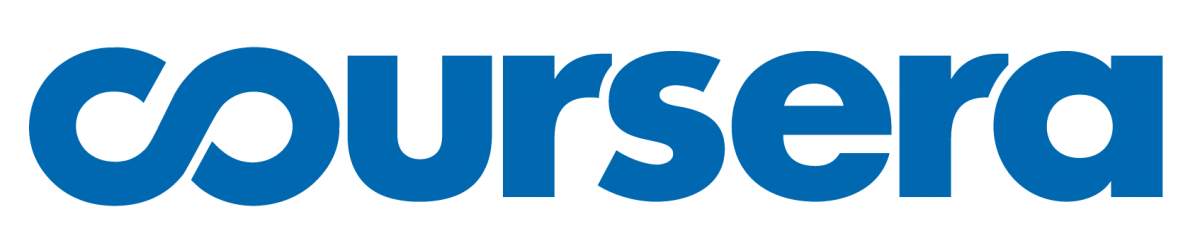
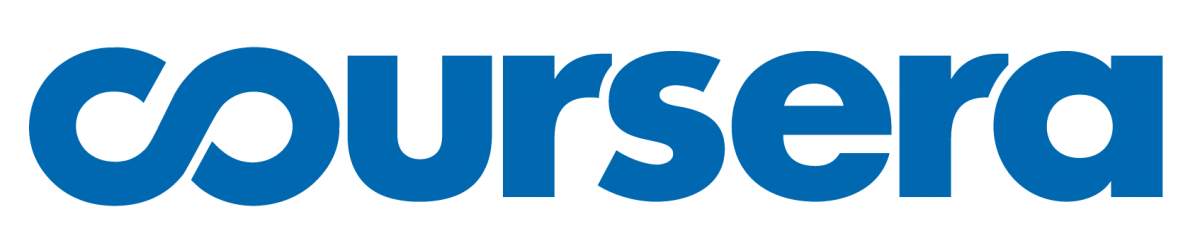
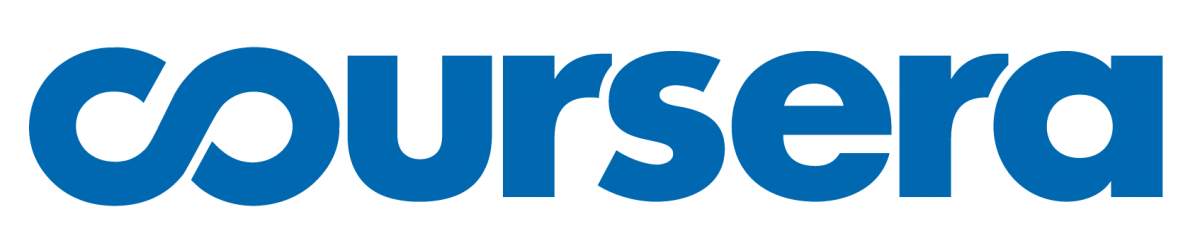
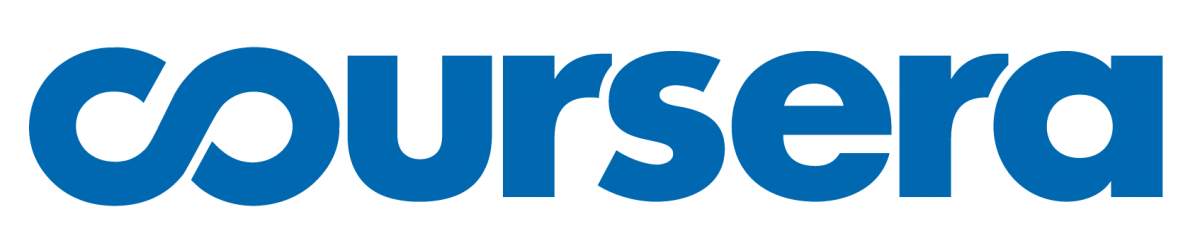
Description
There are 5 courses within this specialization offered by Google. Students will learn how to write distributed machine learning models that scale in Tensorflow, scale out the training of those models. and offer high-performance predictions using the Google Cloud Platform. This is an intermediate-level specialization that also provides an introductory course to Tensorflow.
Teachers:
- Google: Instructors from Google Cloud Training
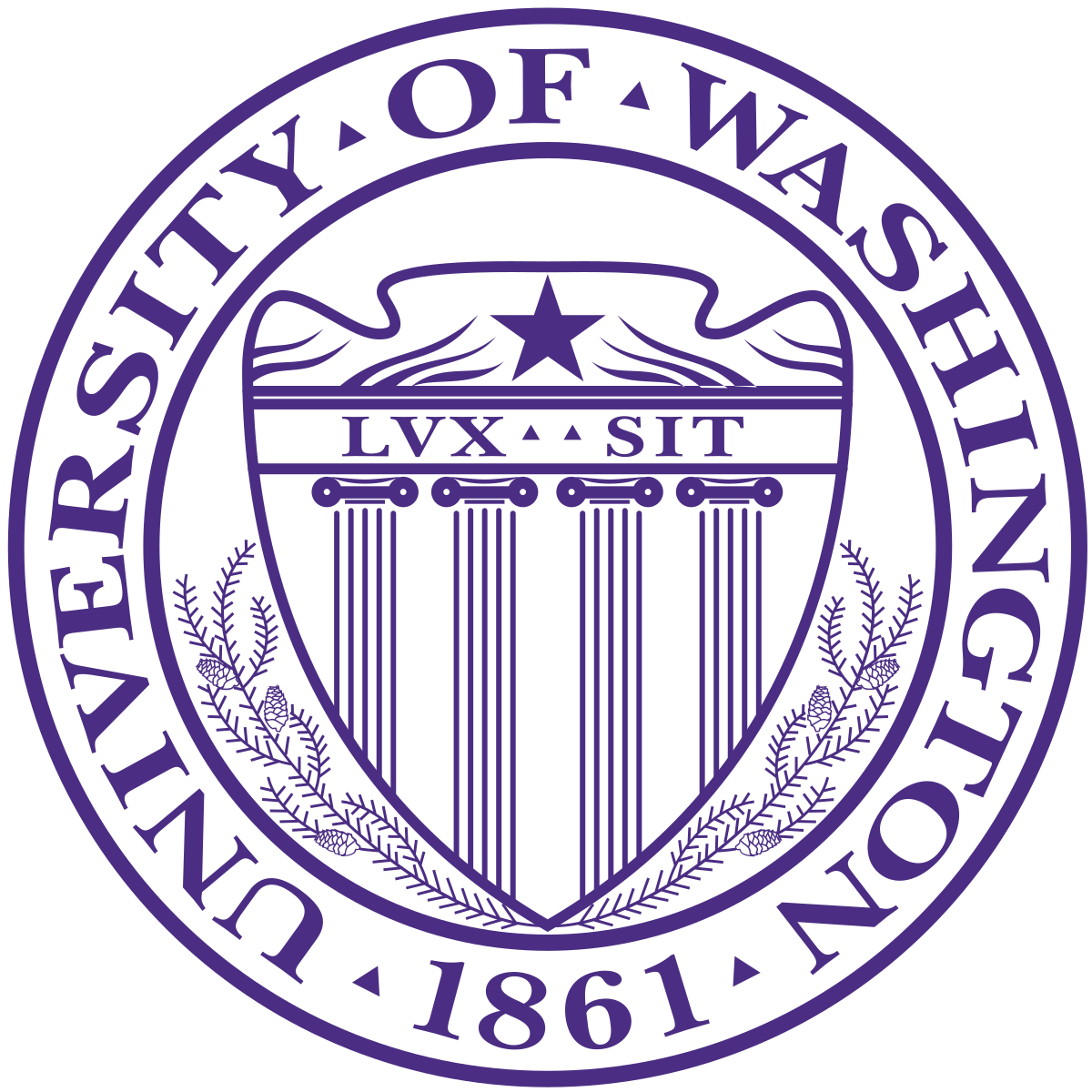
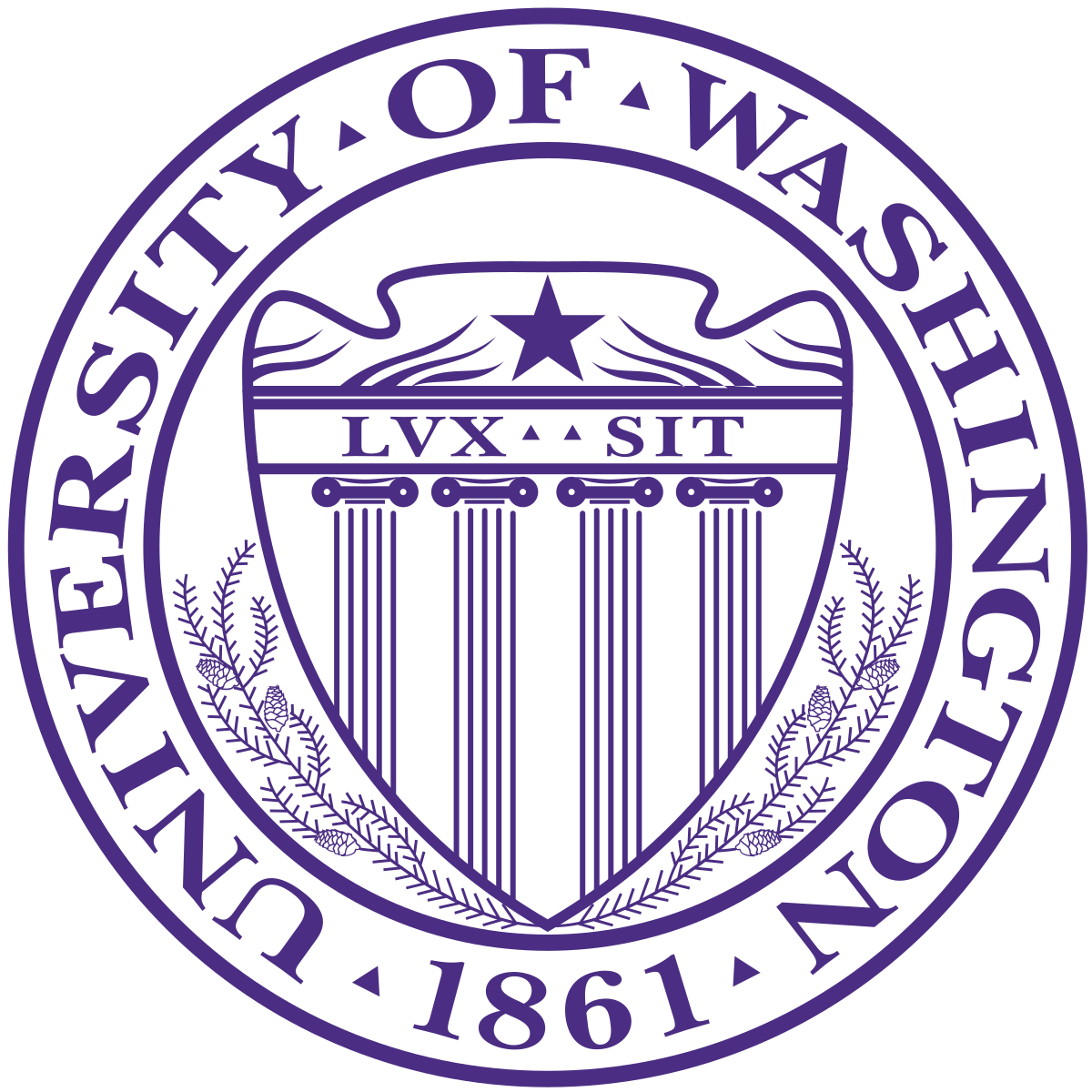
Machine Learning
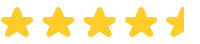
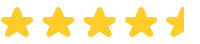
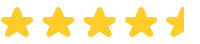
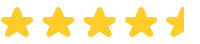
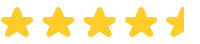
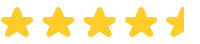
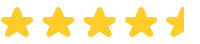
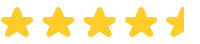
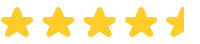
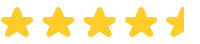
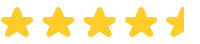
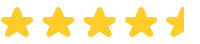
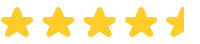
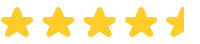
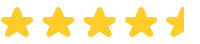
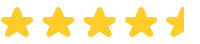
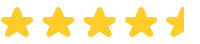
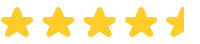
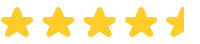
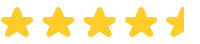
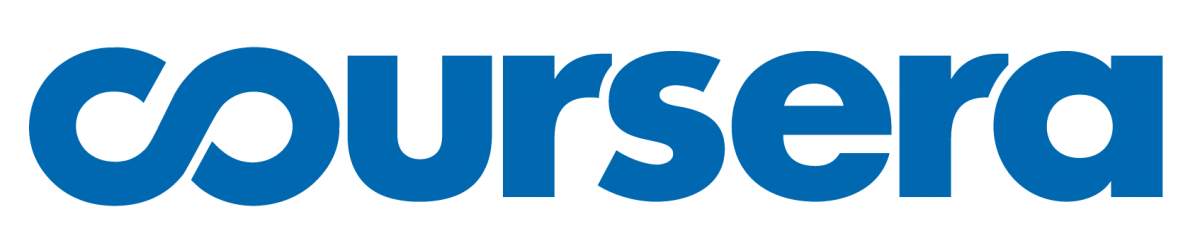
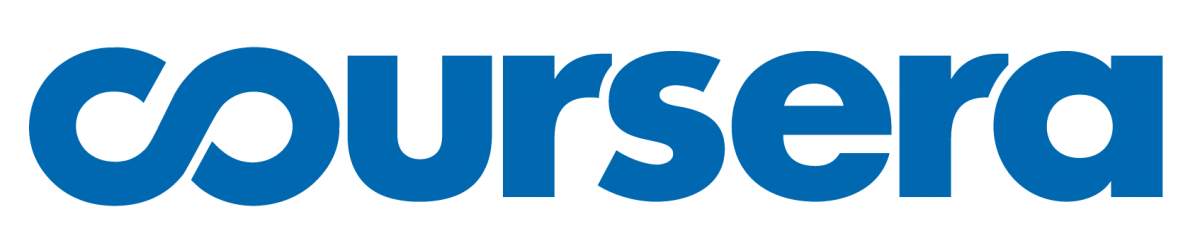
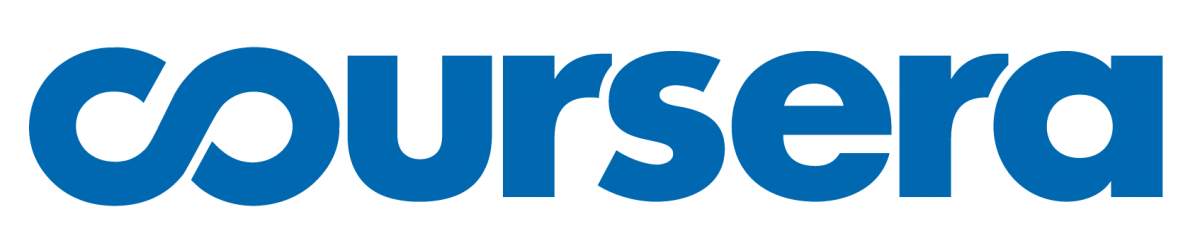
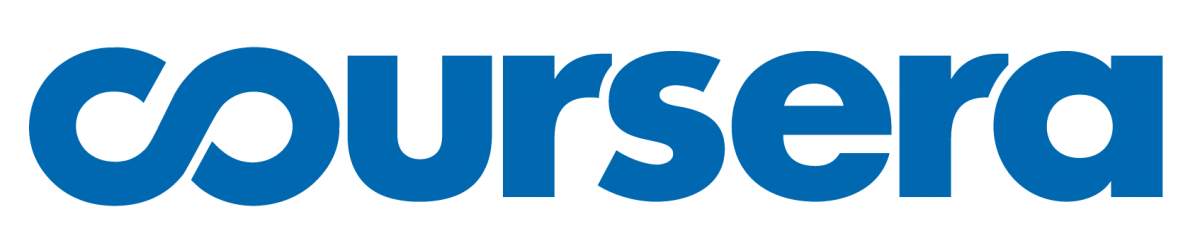
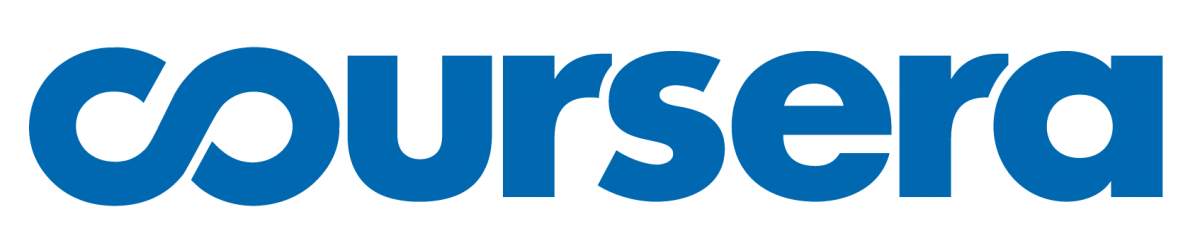
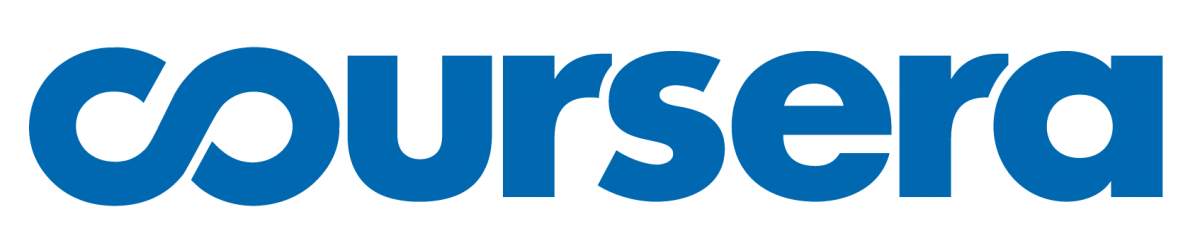
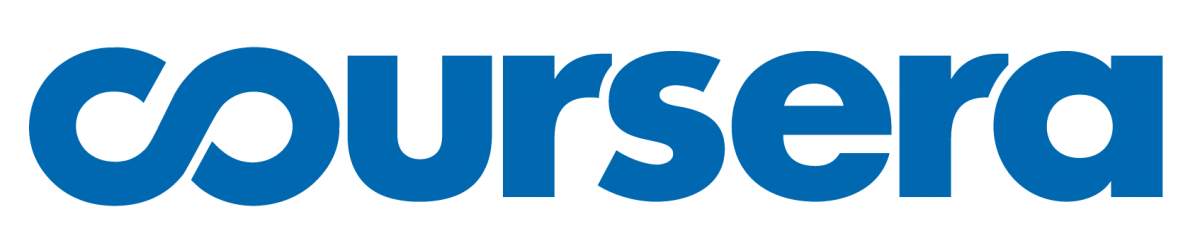
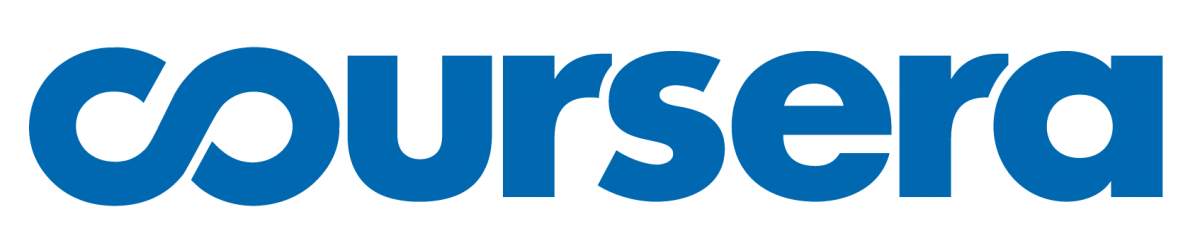
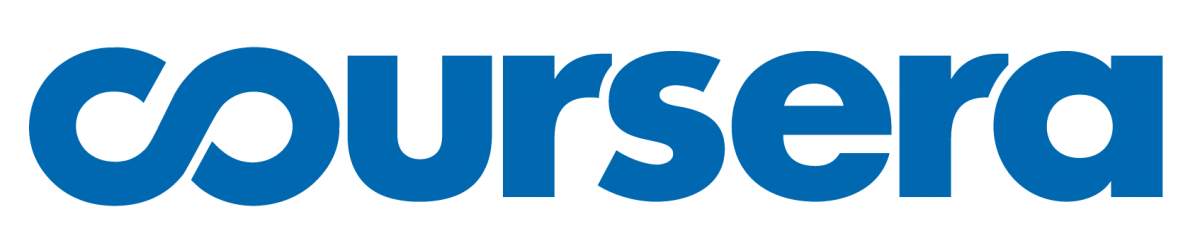
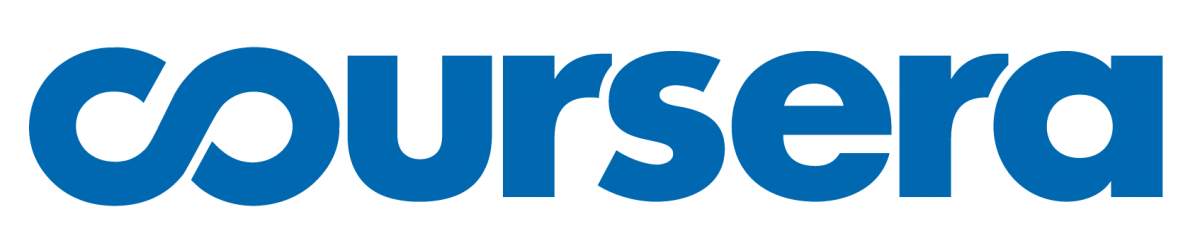
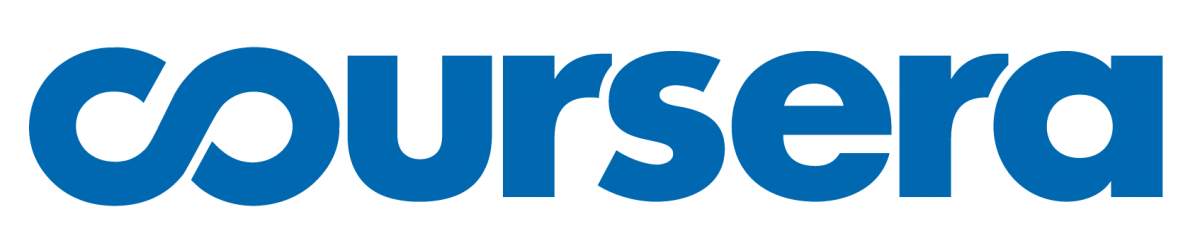
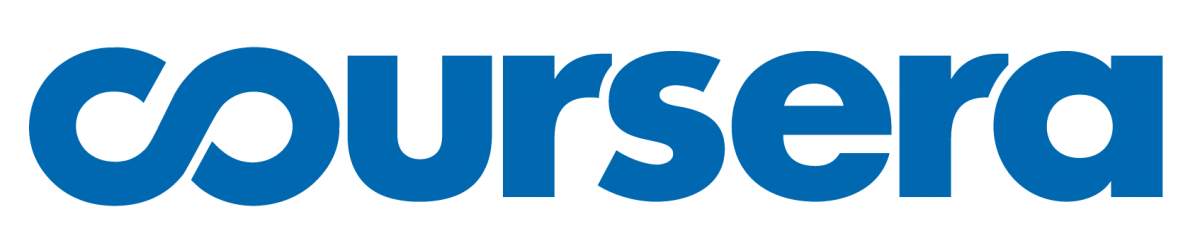
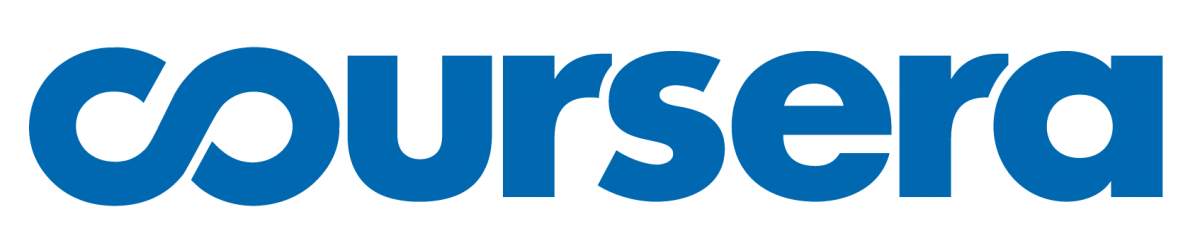
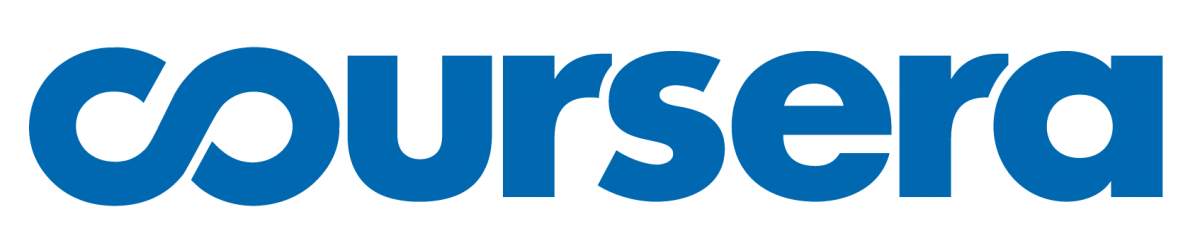
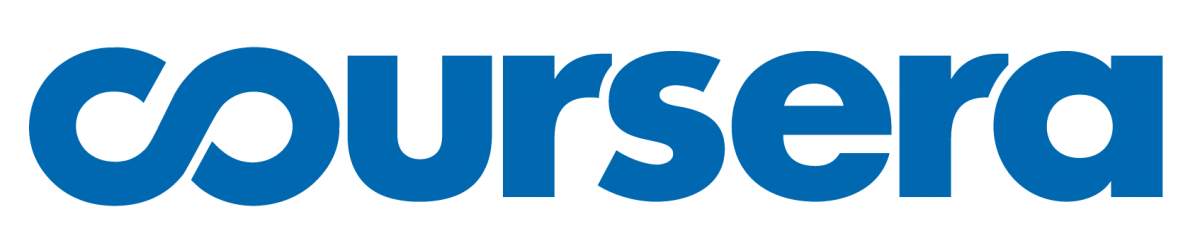
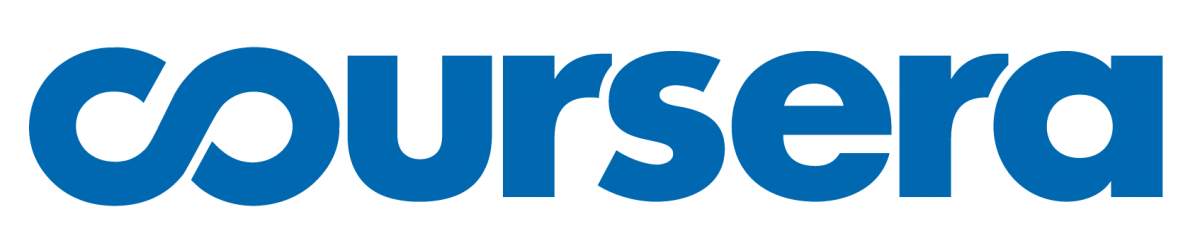
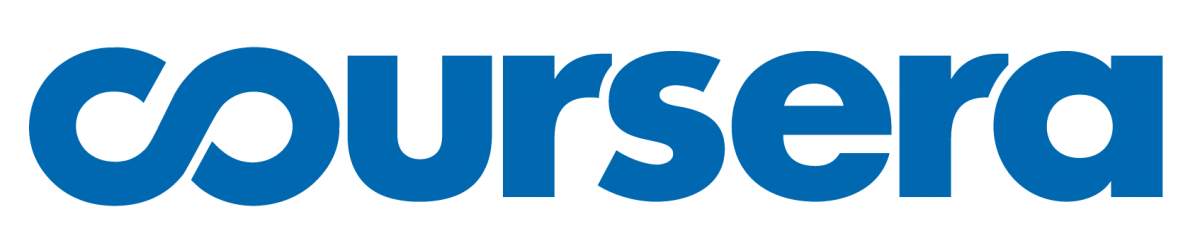
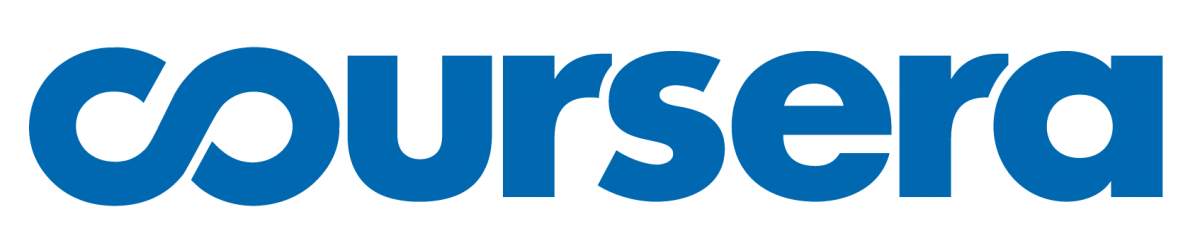
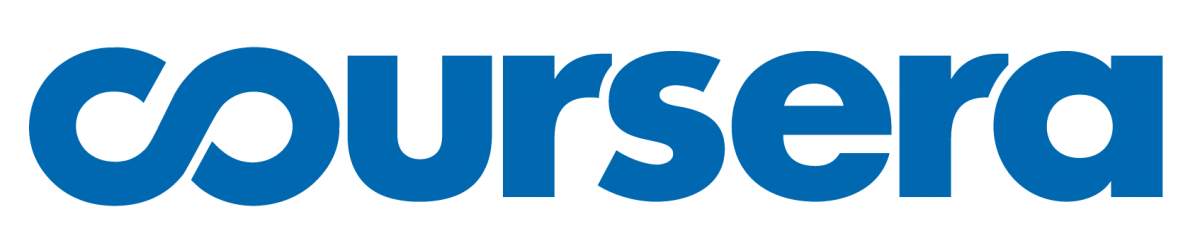
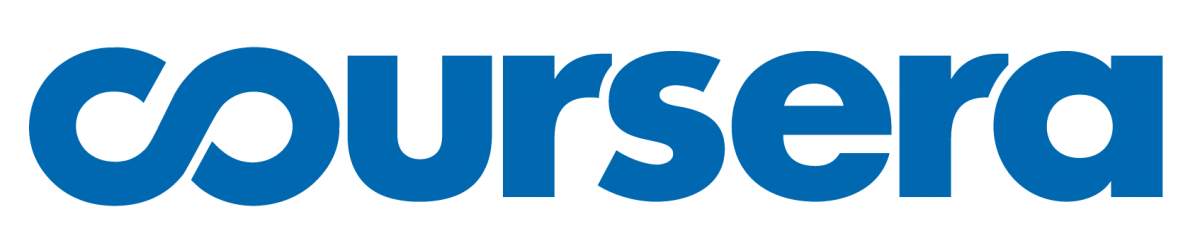
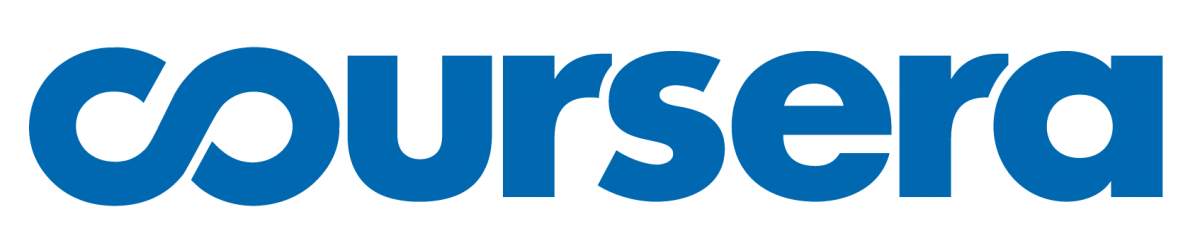
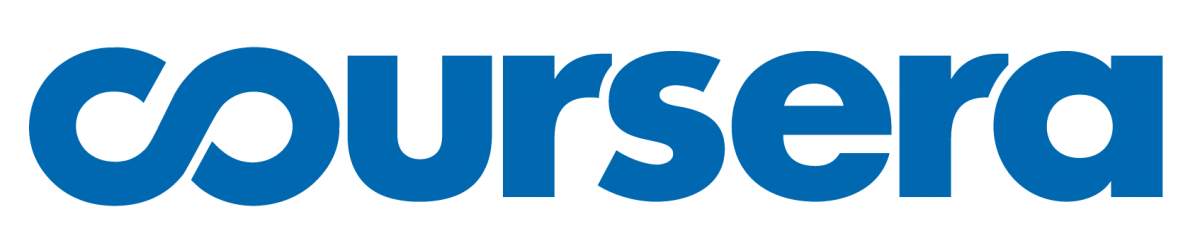
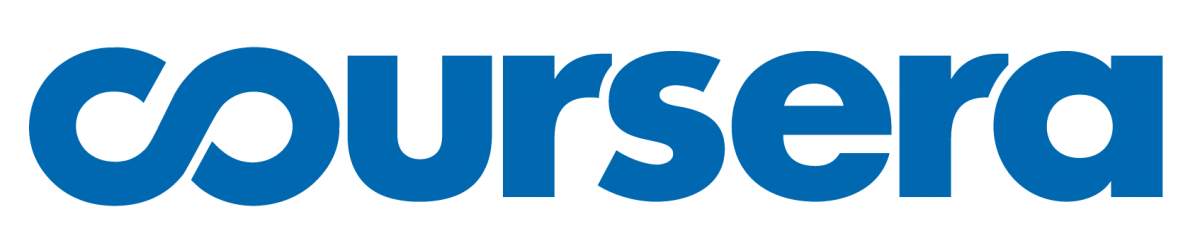
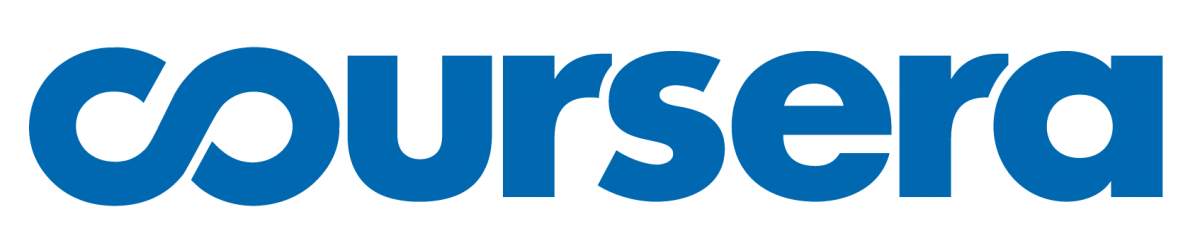
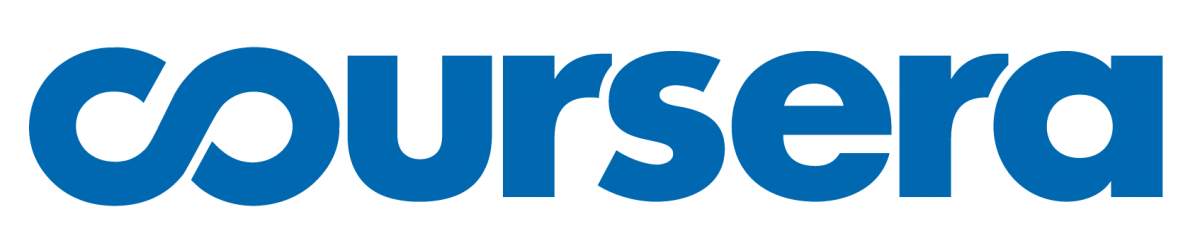
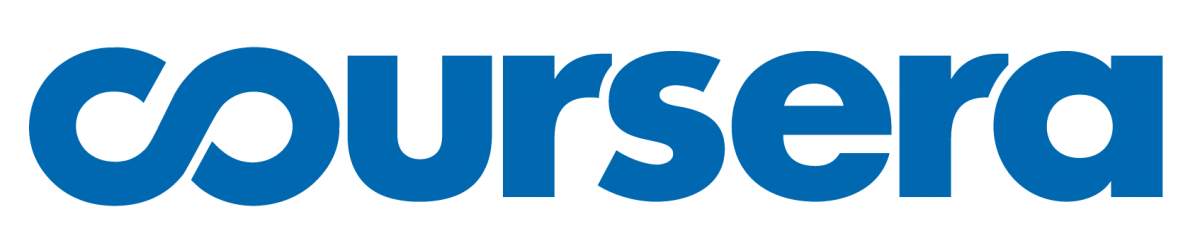
Description
In this specialization, students will learn the fundamentals of machine learning and cover concepts such as regression, clustering, and classification. It is offered by the University of Washington and the instructors are Amazon Professors of Machine Learning. The specialization consists of 4 courses.
Teachers:
- Amazon: Carlos Guestrin, Professor of Machine Learning
- Amazon: Emily Fox, Professor of Machine Learning
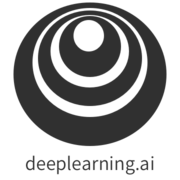
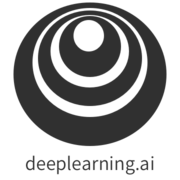
Deep Learning
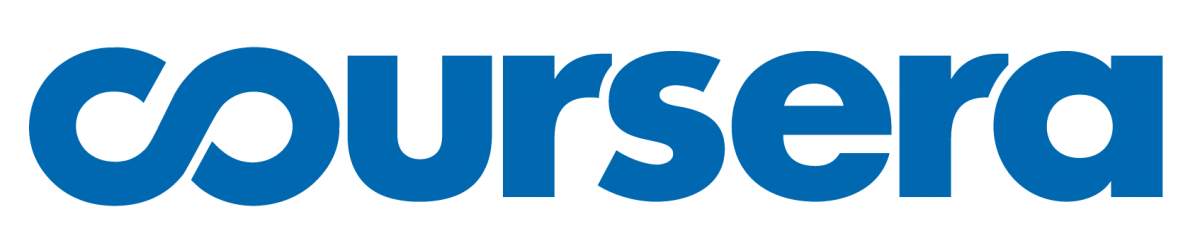
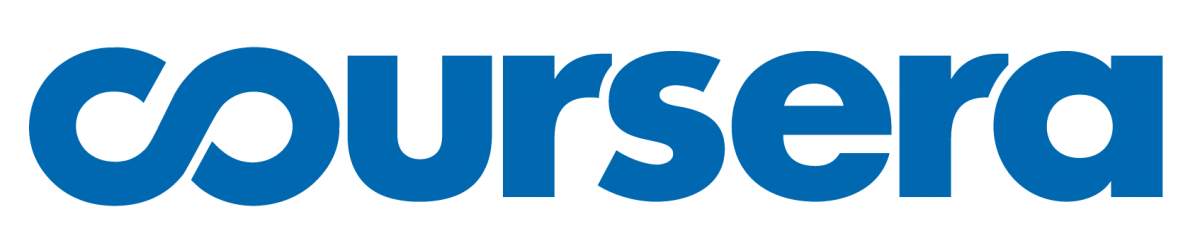
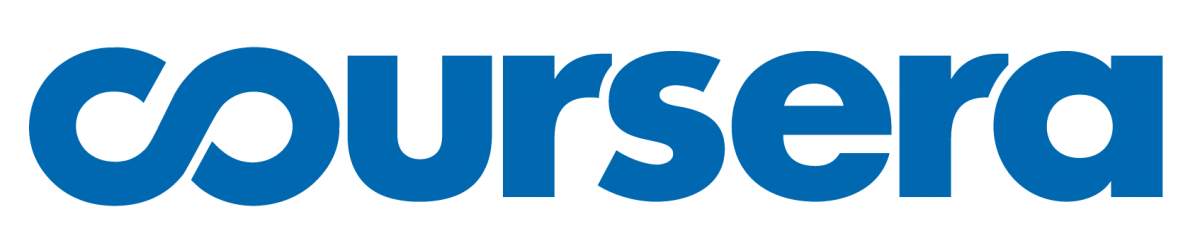
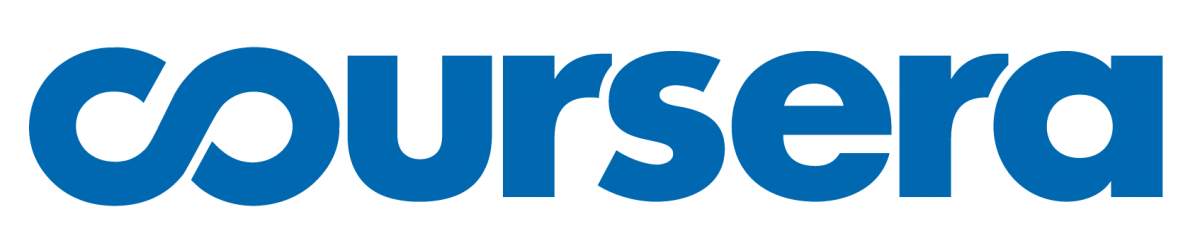
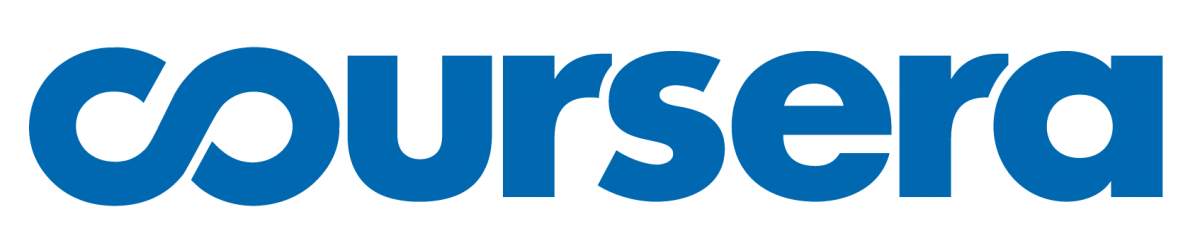
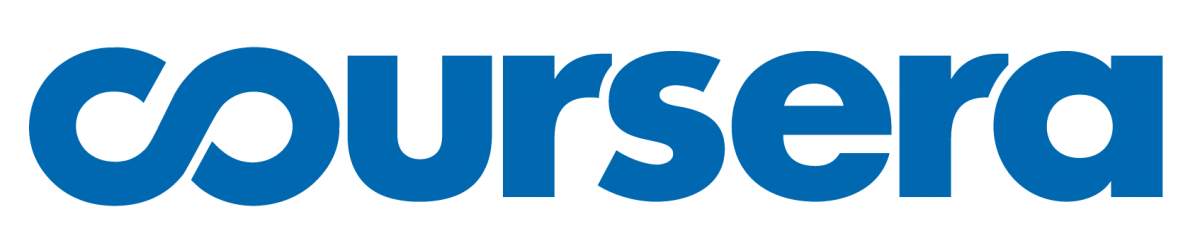
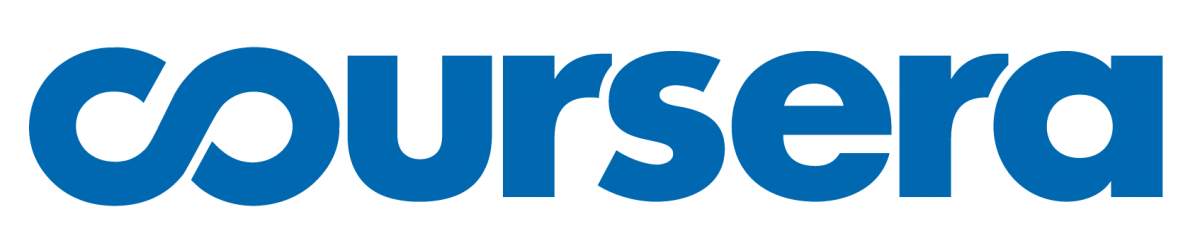
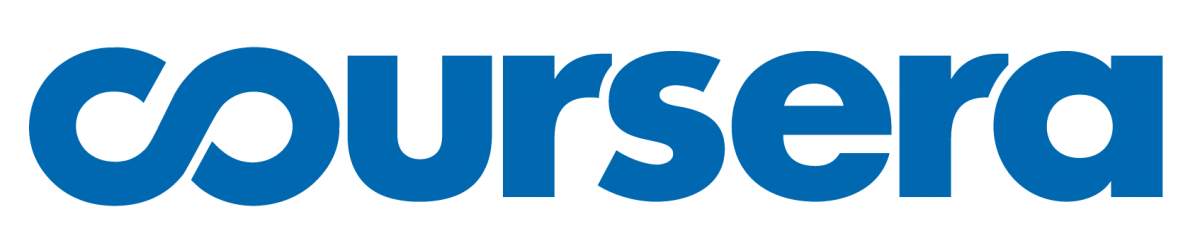
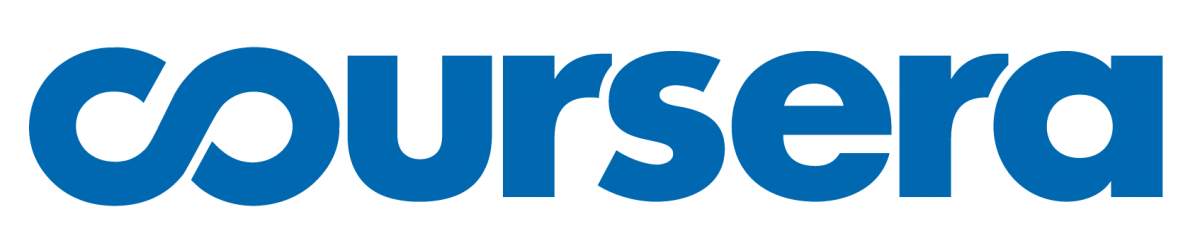
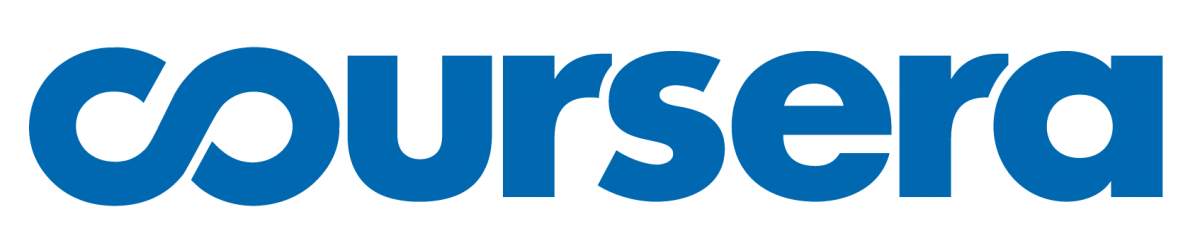
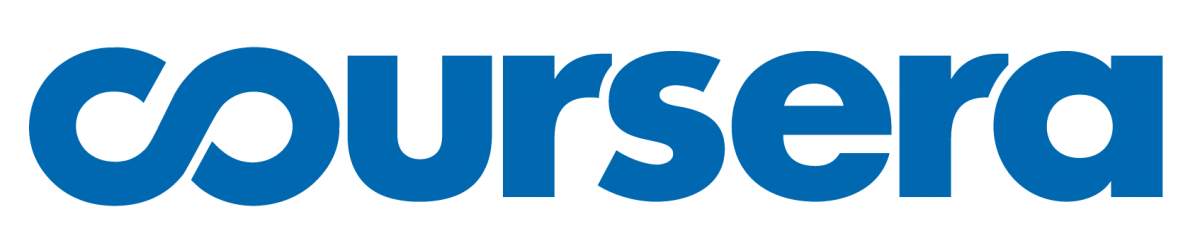
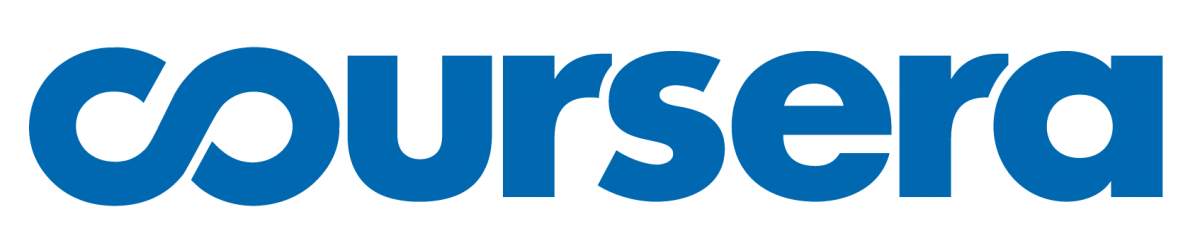
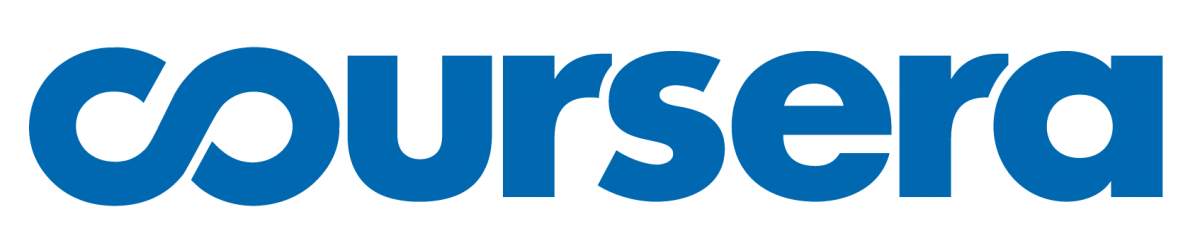
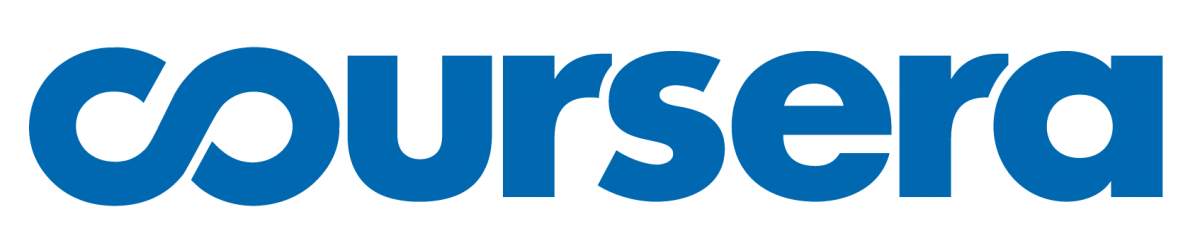
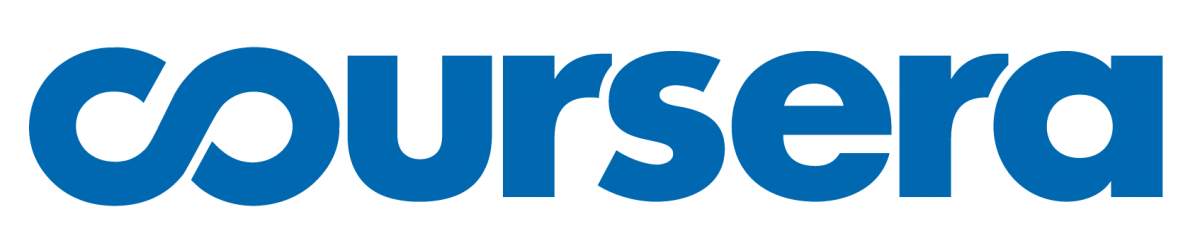
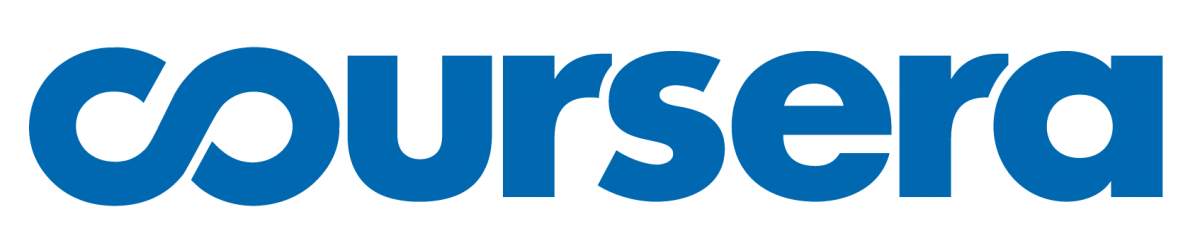
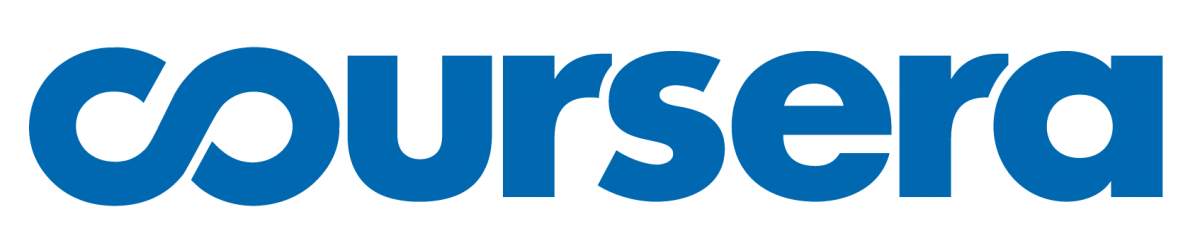
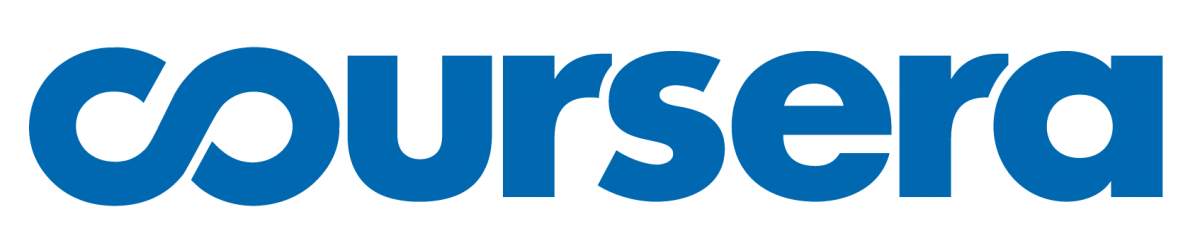
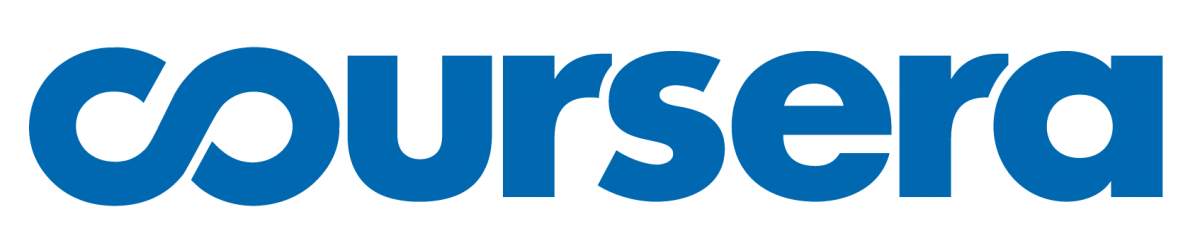
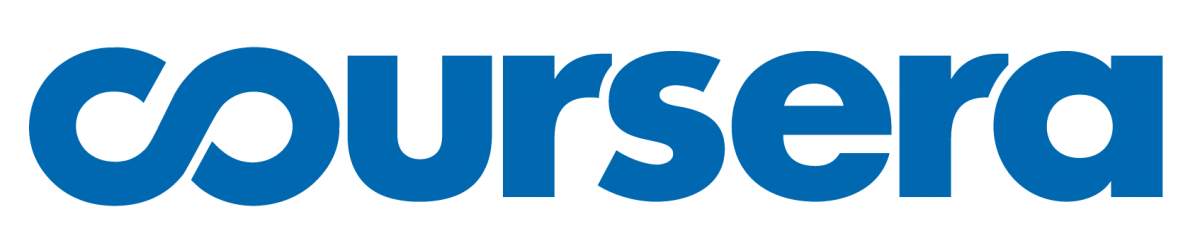
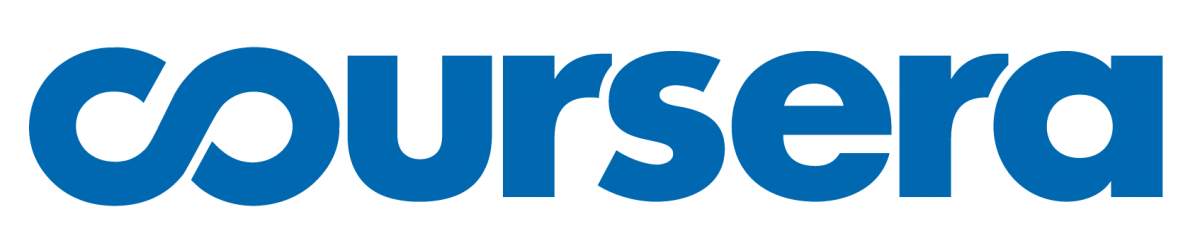
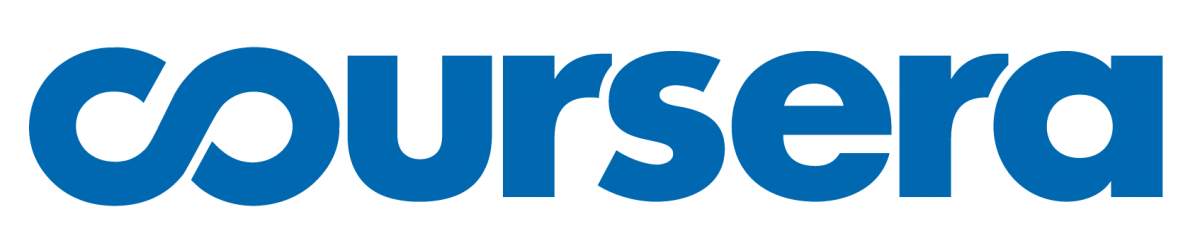
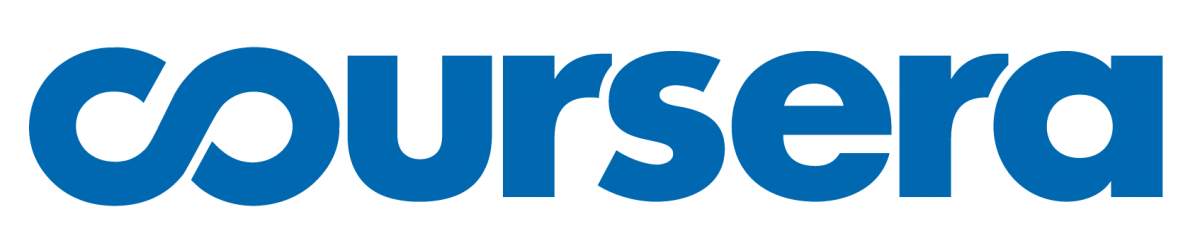
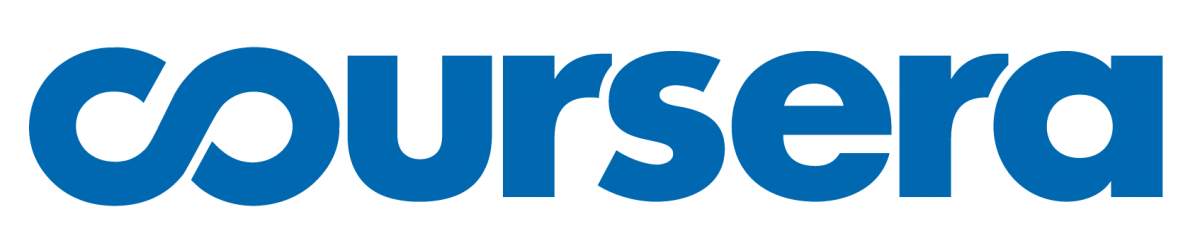
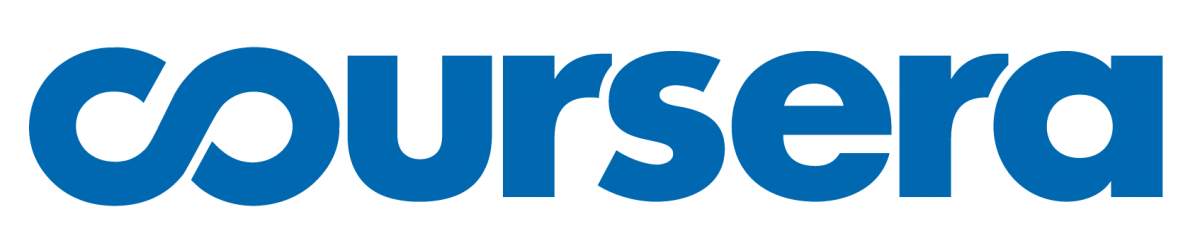
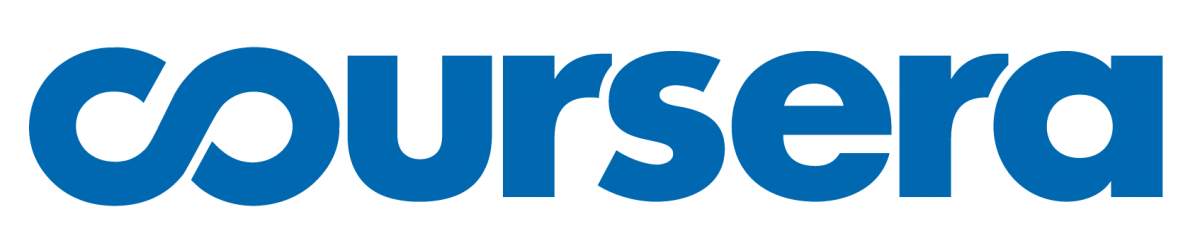
Description
The popular Deep Learning Specialization offered by deeplearning.ai and Andrew Ng. In this specialization students will learn how to build neural networks, and learn how to lead successful machine learning projects. CNN, RNNs, LSTM, Adam, Dropout, BatchNorm, Xavier/He initialization, through 5 courses: Neural Networks and Deep Learning, Improving Deep Neural Networks, Structuring Machine Learning Projects, Convolutional Neural Networks, Sequence Models.
Teachers:
- Landing AI: Andrew Ng
- :
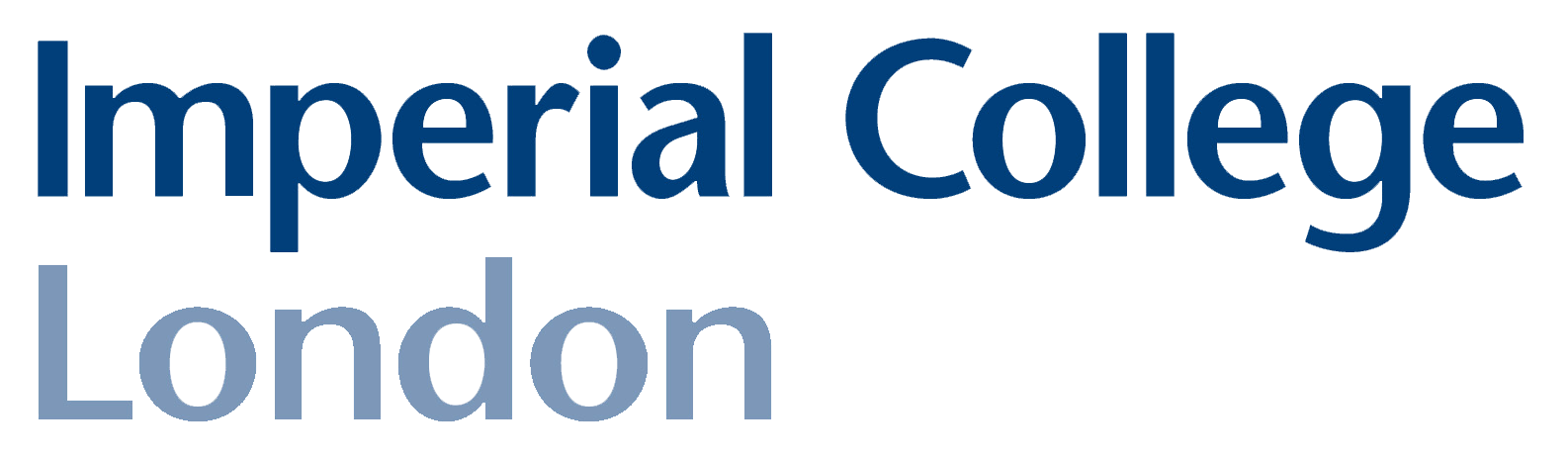
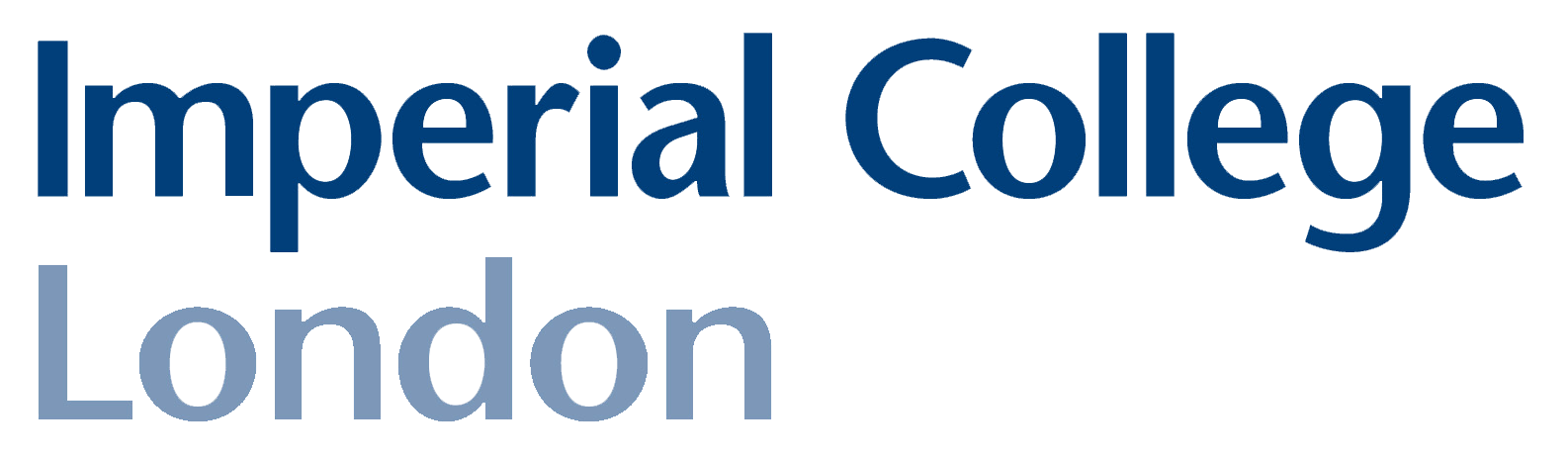
Mathematics for Machine Learning
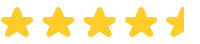
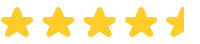
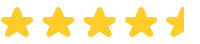
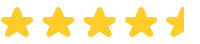
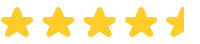
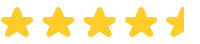
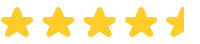
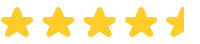
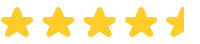
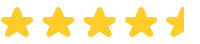
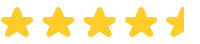
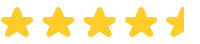
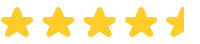
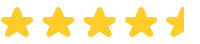
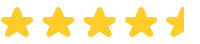
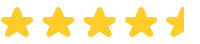
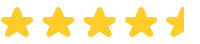
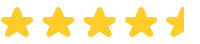
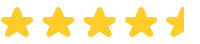
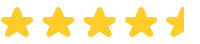
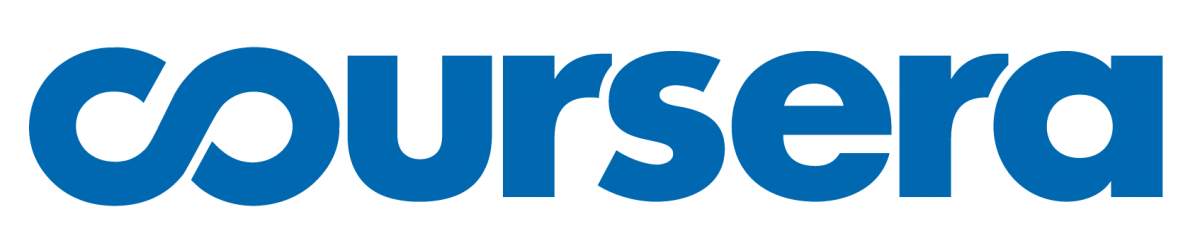
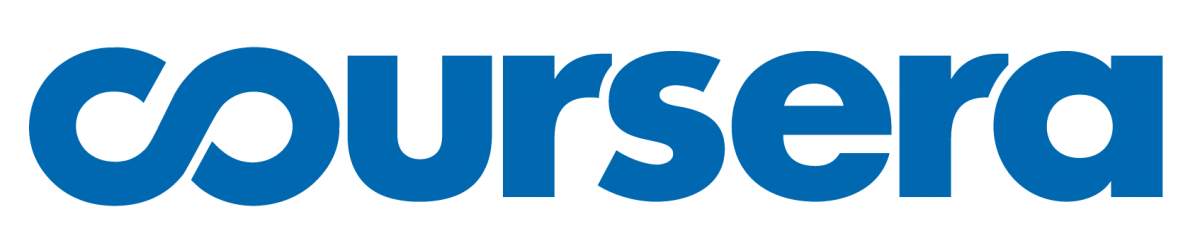
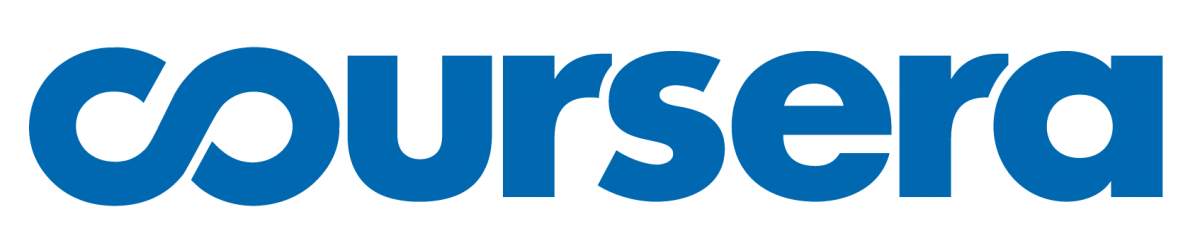
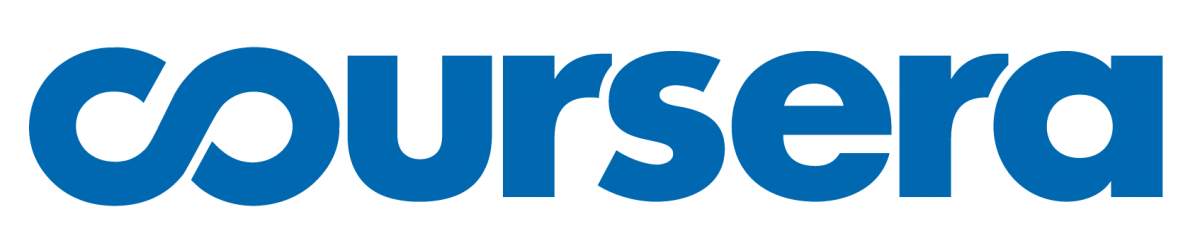
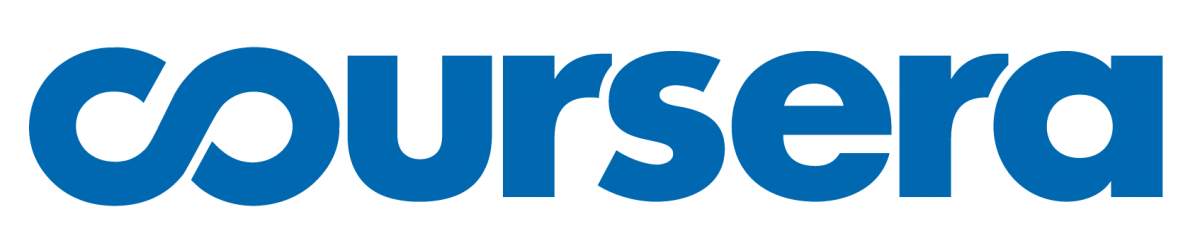
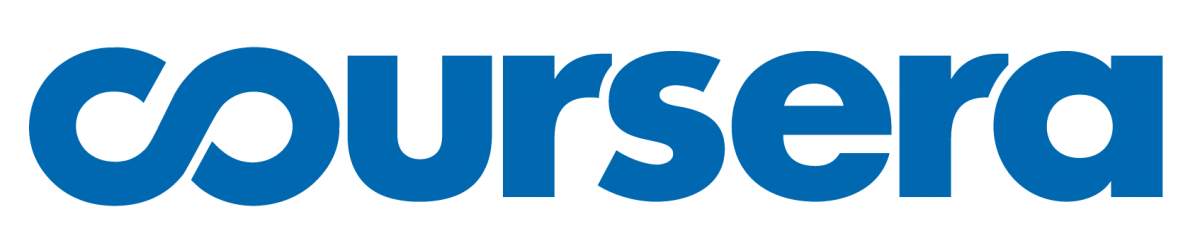
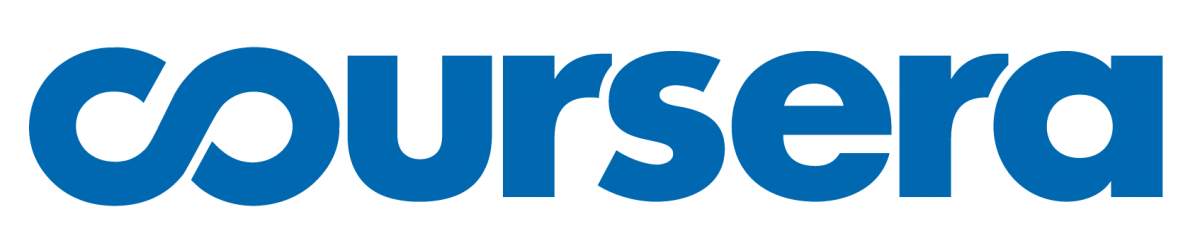
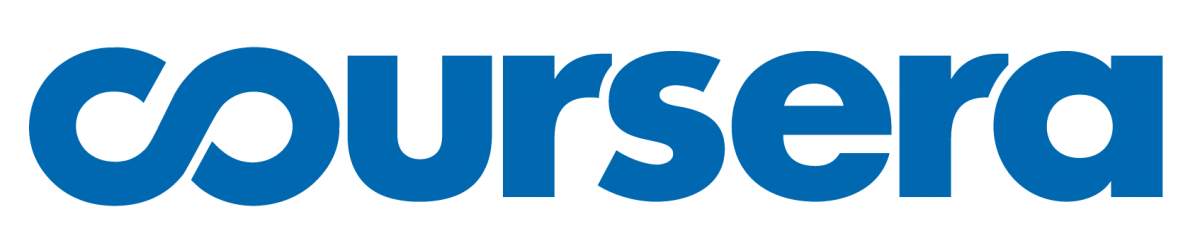
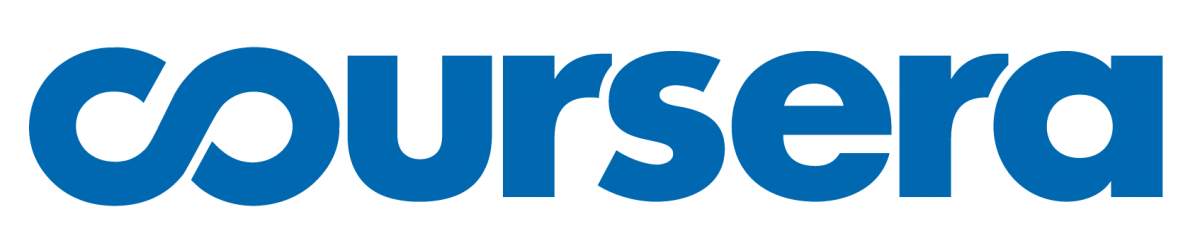
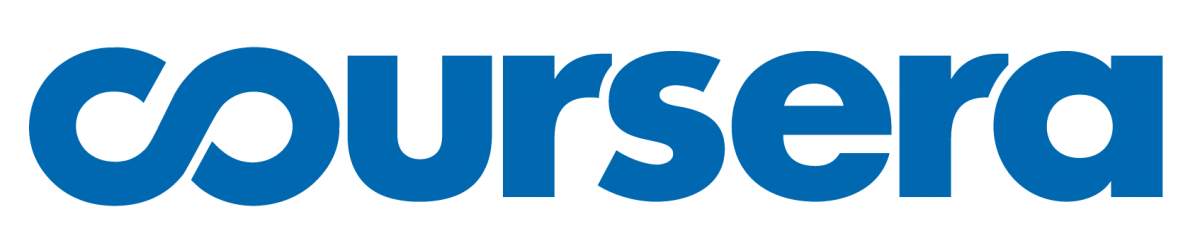
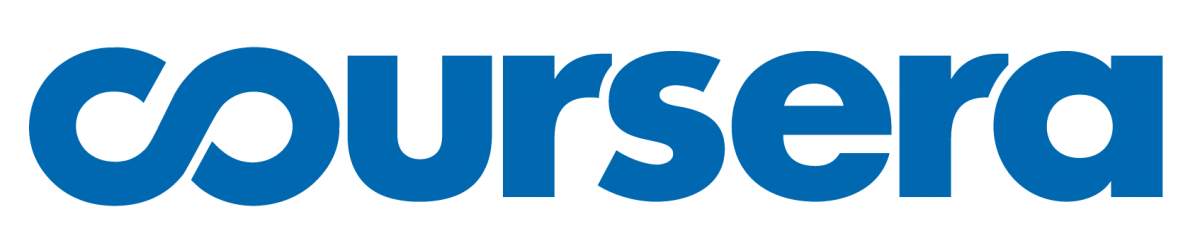
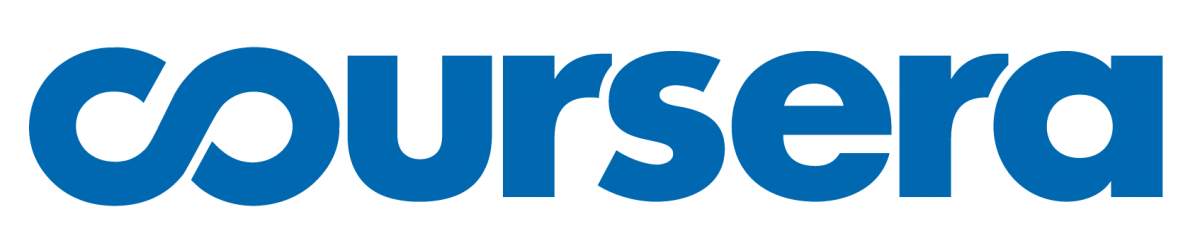
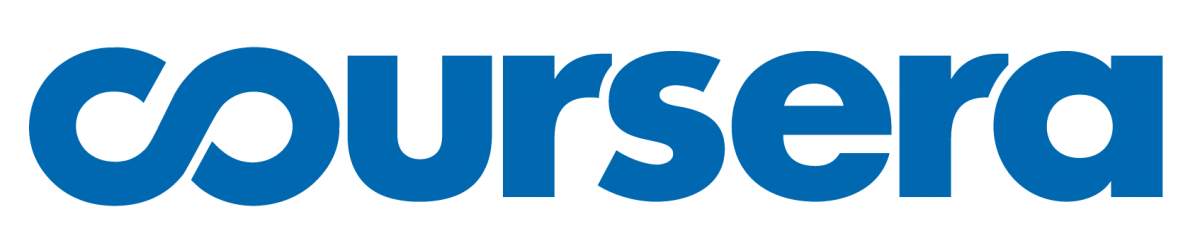
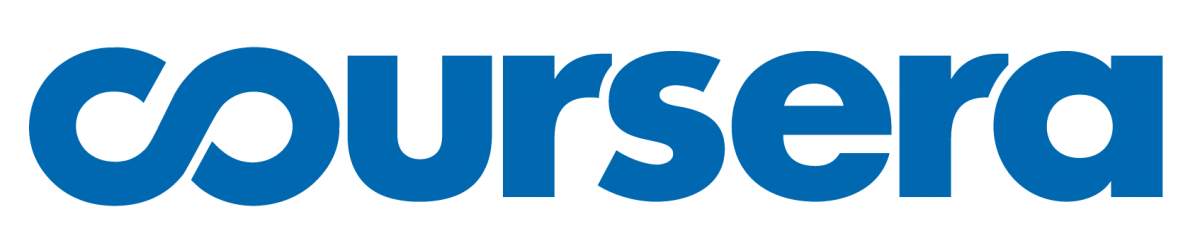
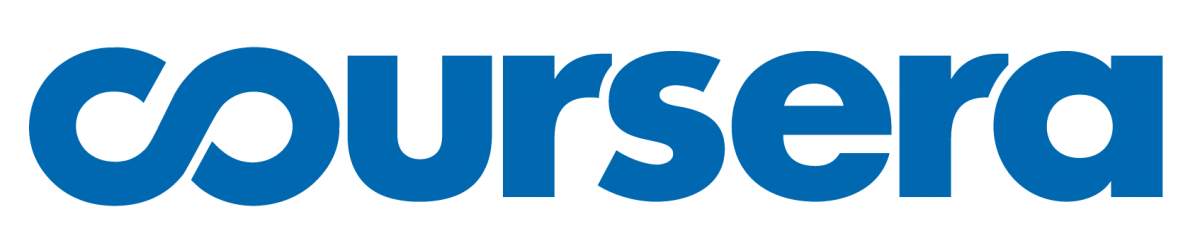
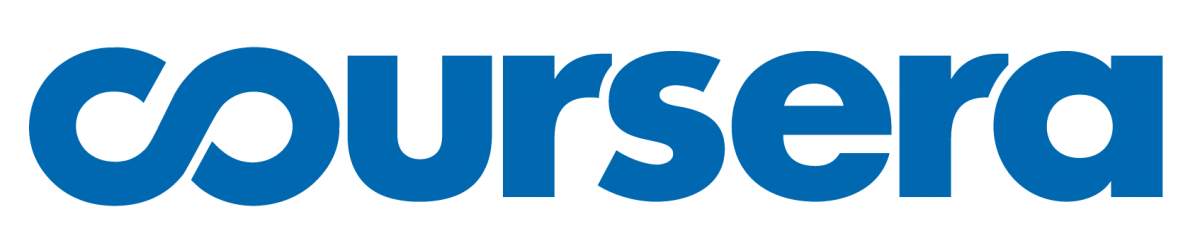
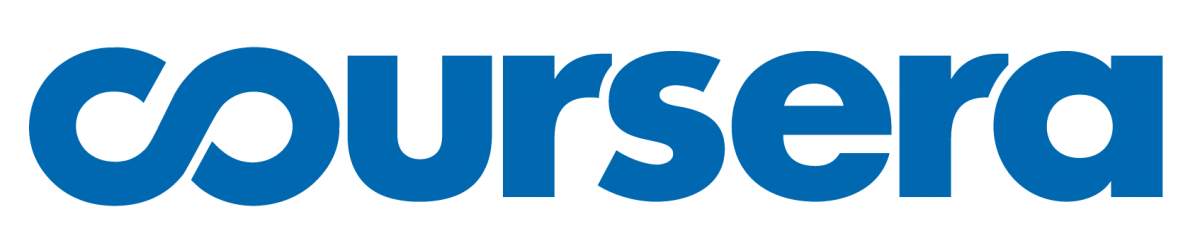
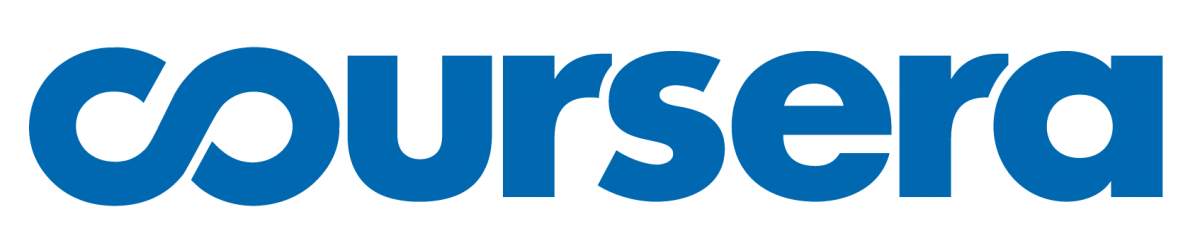
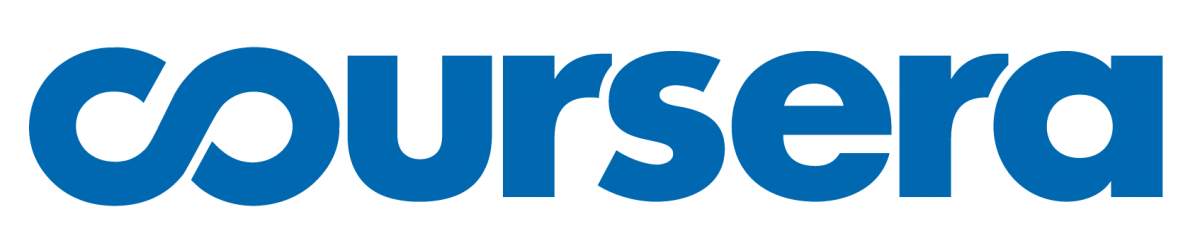
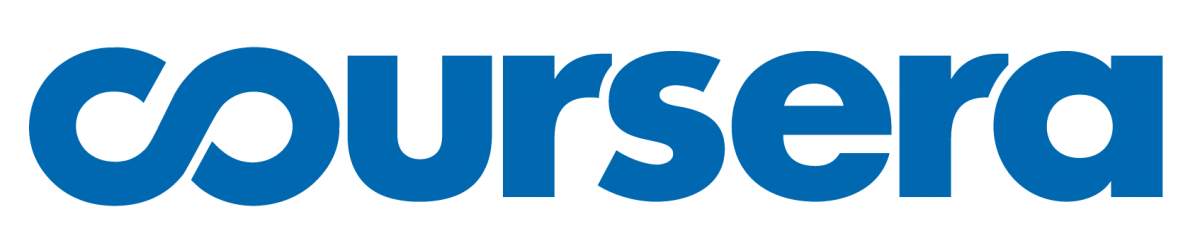
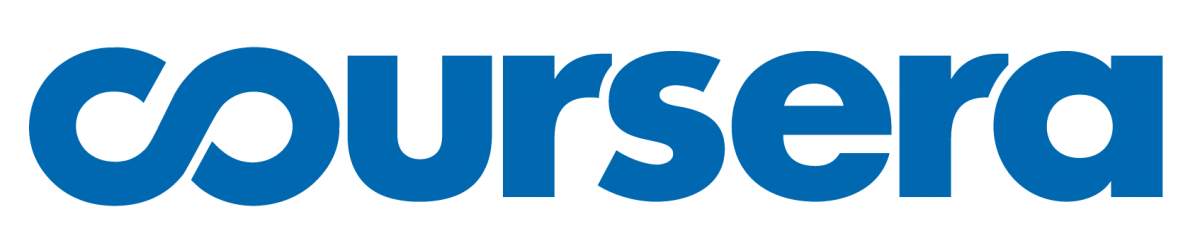
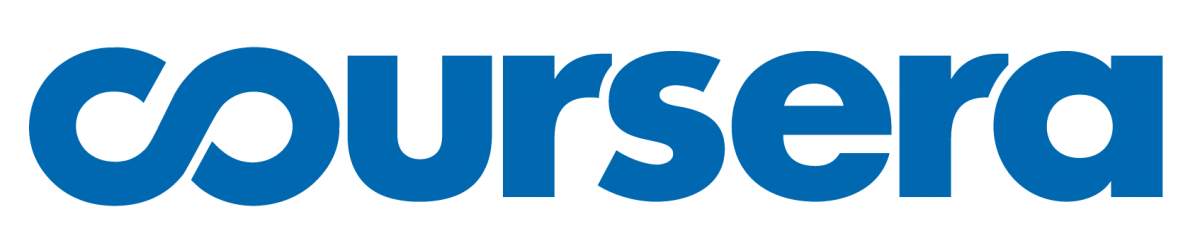
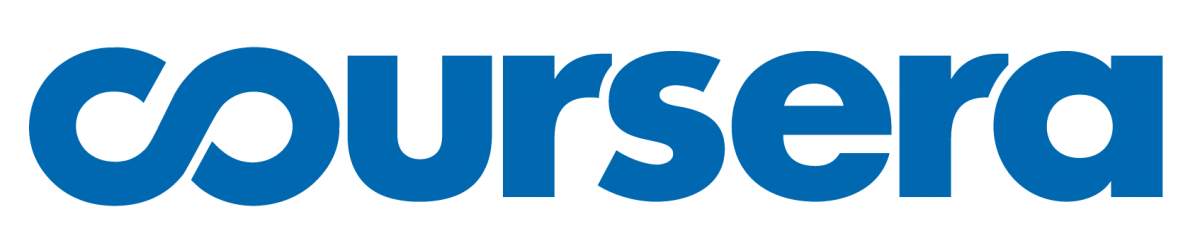
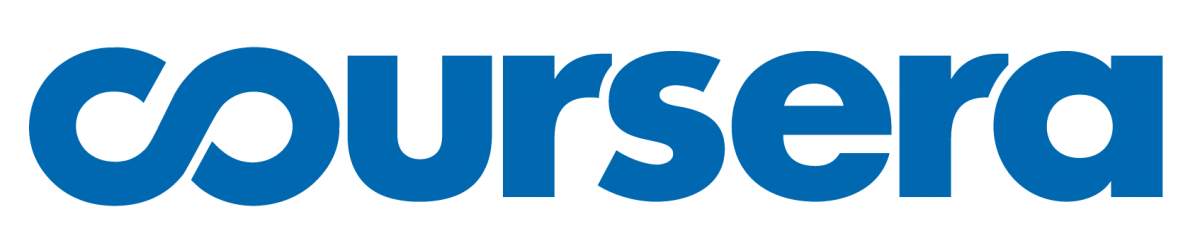
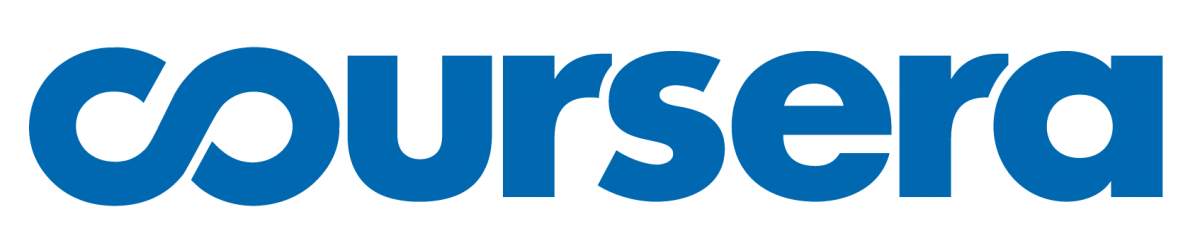
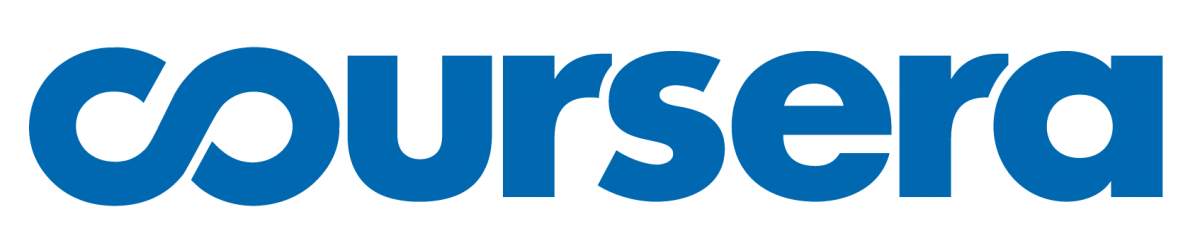
Description
A beginner-level specialization that provides students with the possibility to learn about the underlying machinery behind machine learning. Students will cover how to build an intuitive understanding, and how to relate it to Machine Learning and Data Science.
Teachers:
- Imperial College London: David Dye, Professor of Metallurgy
- Imperial College London: A. Freddie Page, Strategic Teaching Fellow
- Imperial College London: Marc P. Deisenroth, Lecturer in Statistical Machine Learning
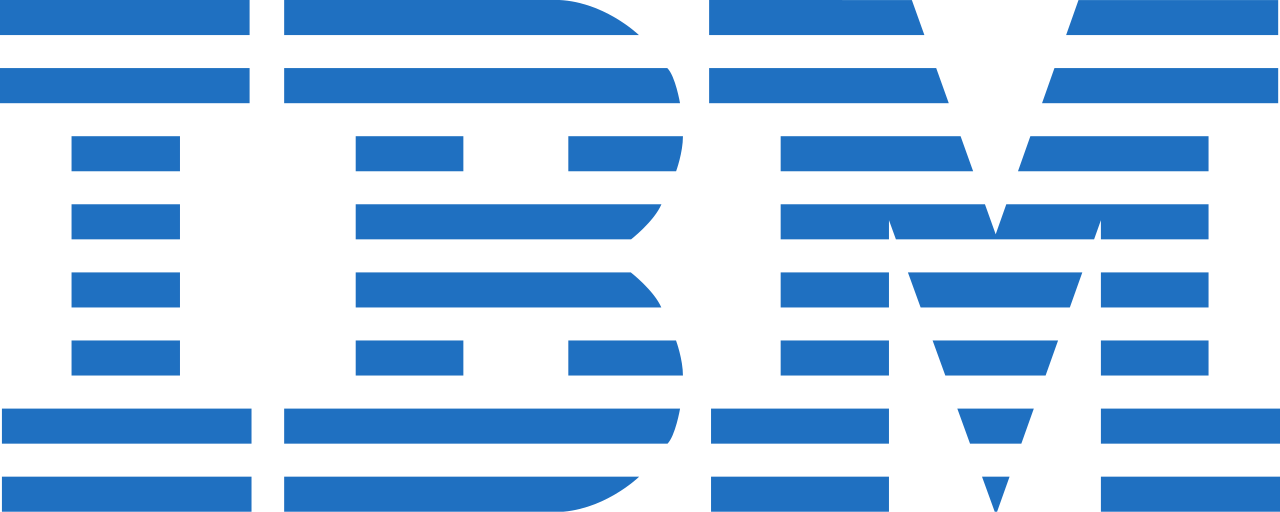
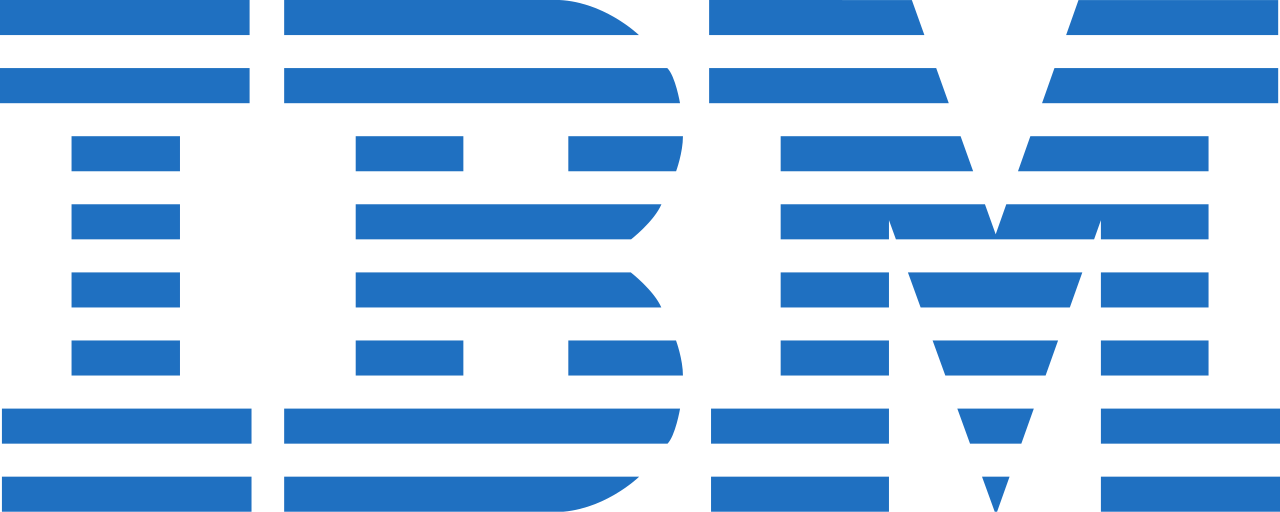
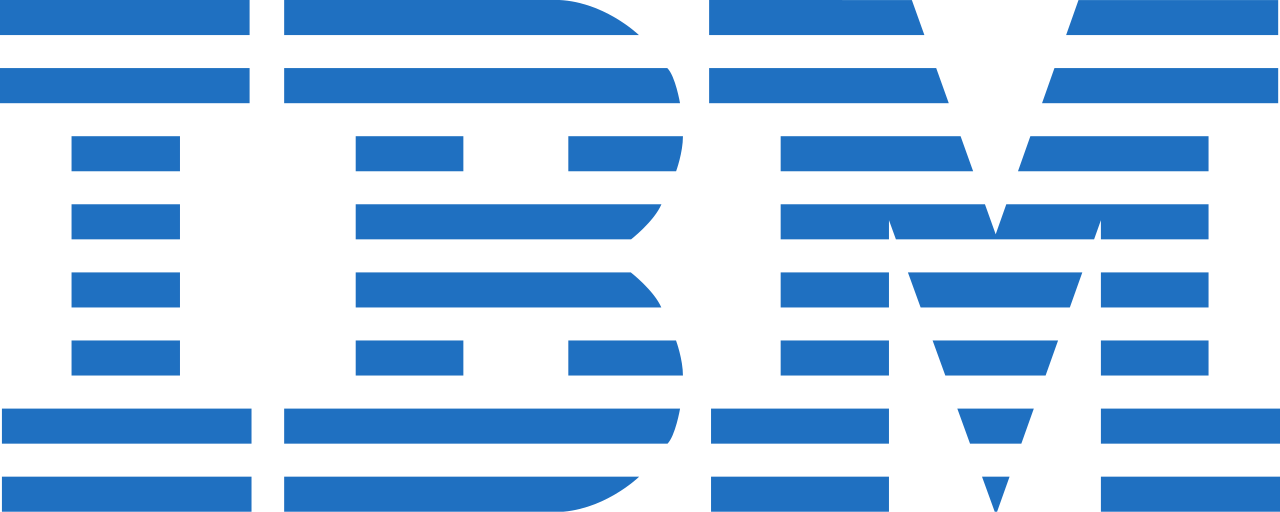
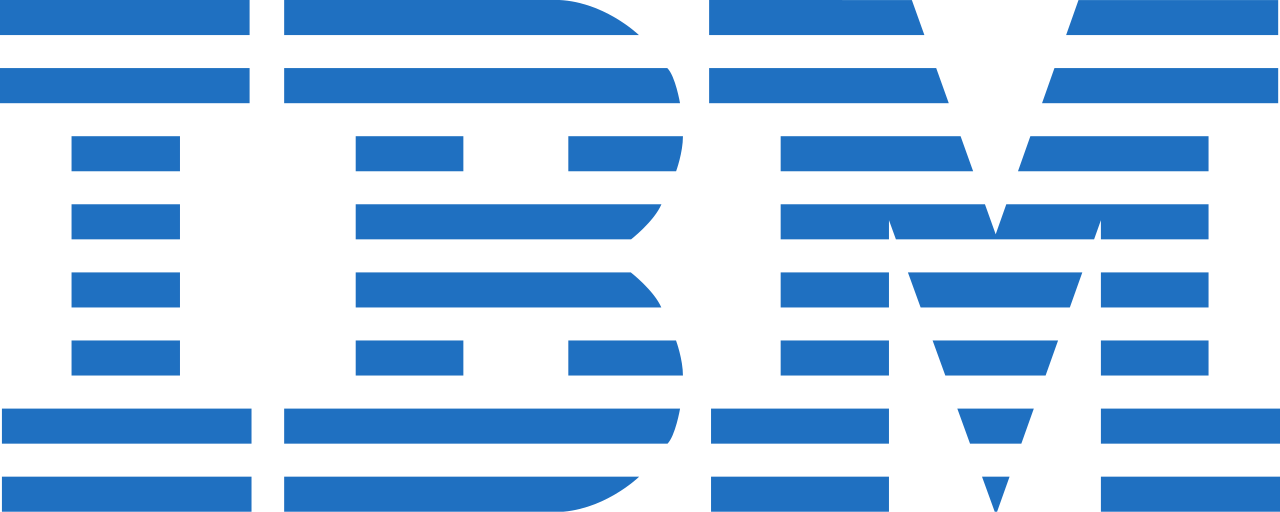
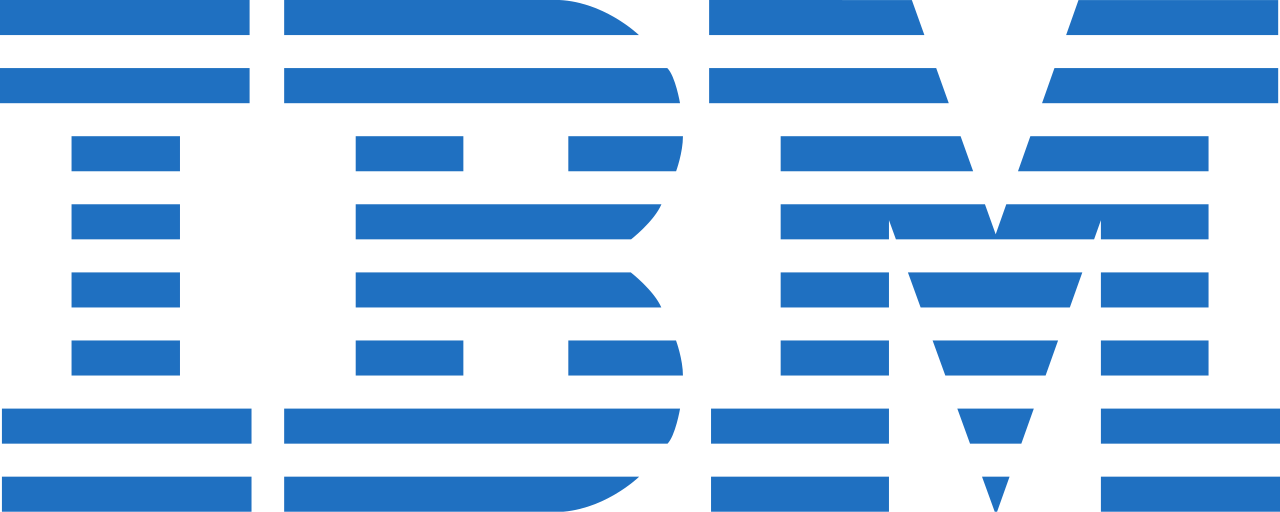
Advanced Data Science with IBM
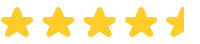
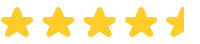
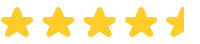
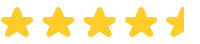
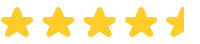
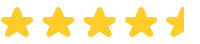
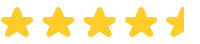
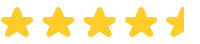
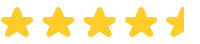
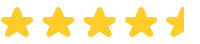
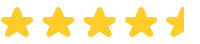
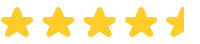
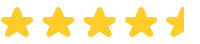
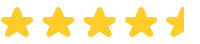
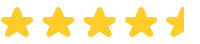
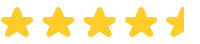
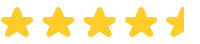
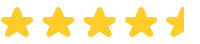
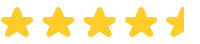
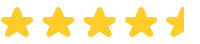
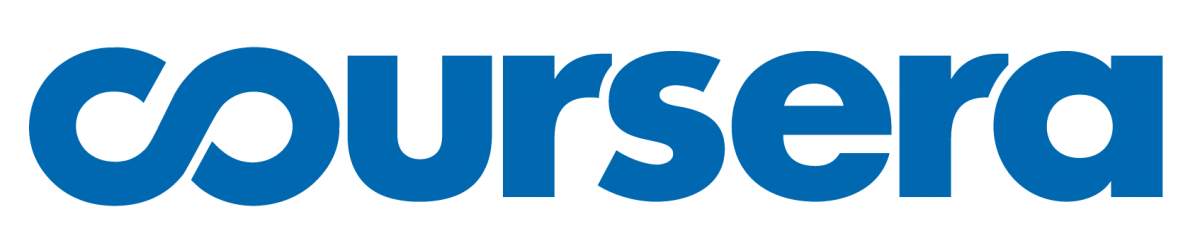
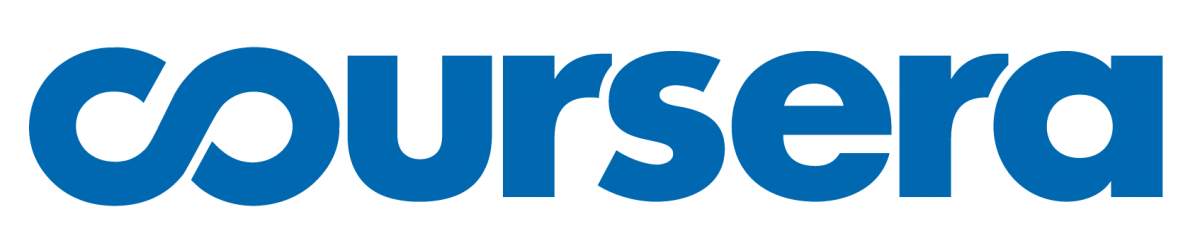
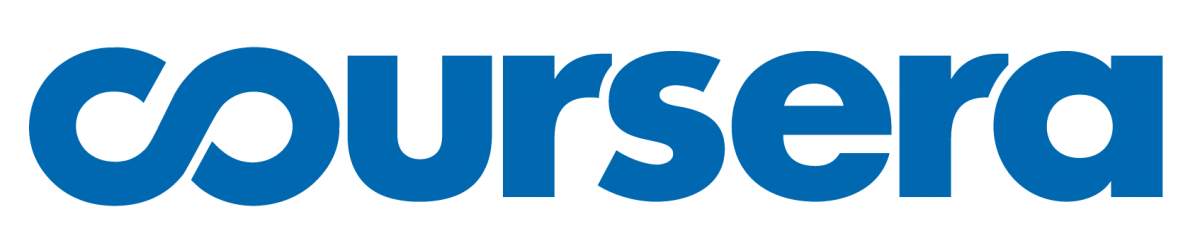
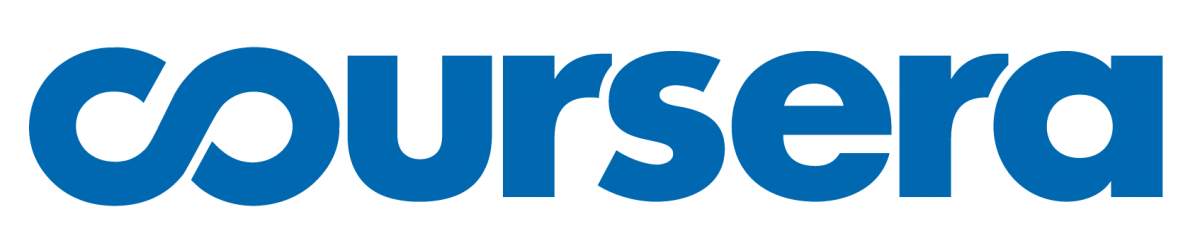
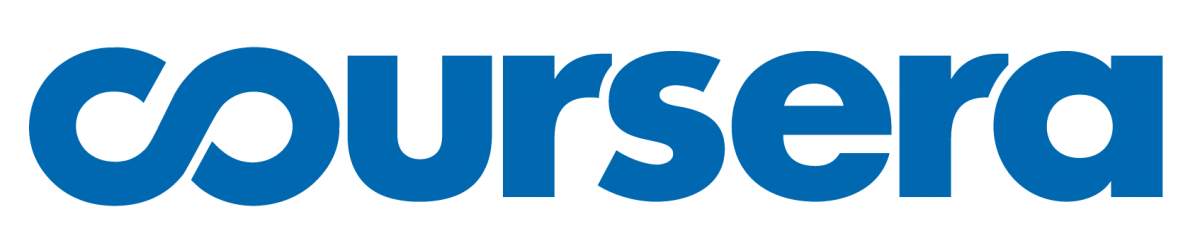
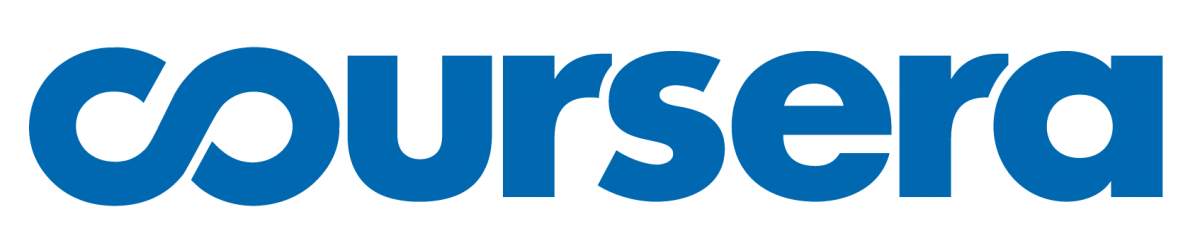
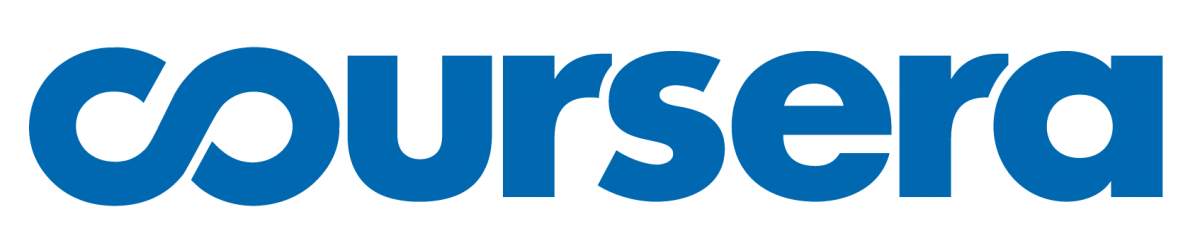
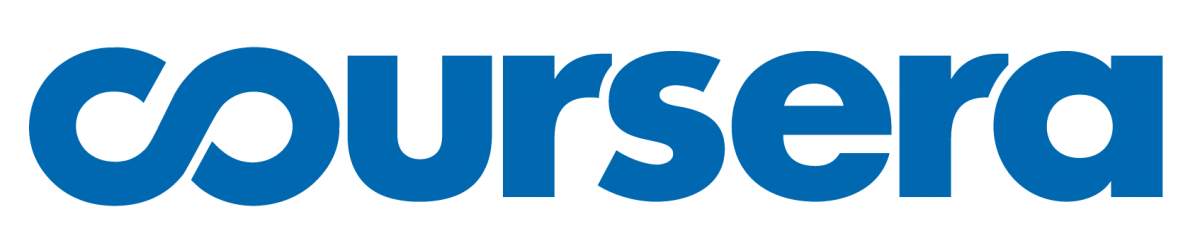
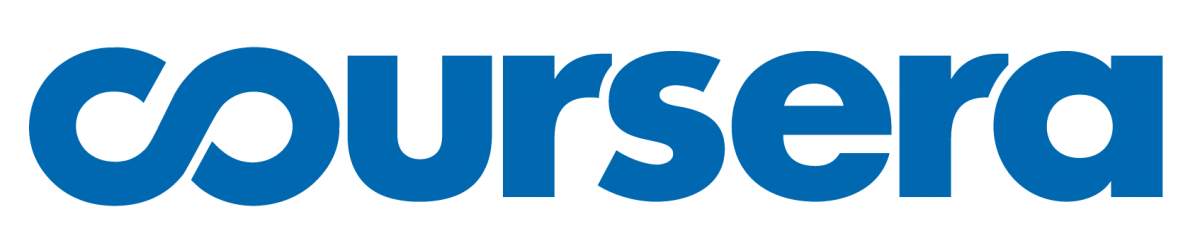
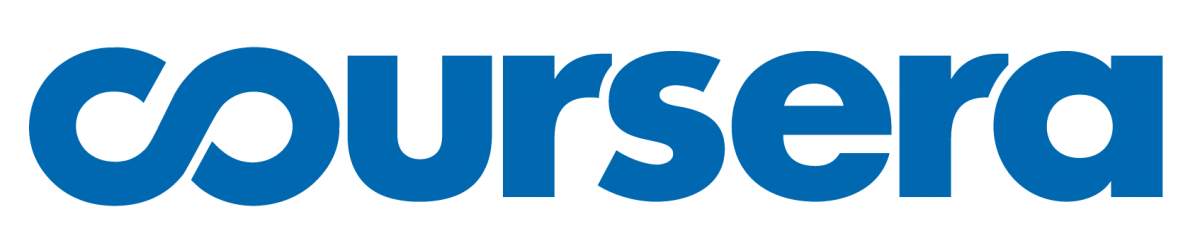
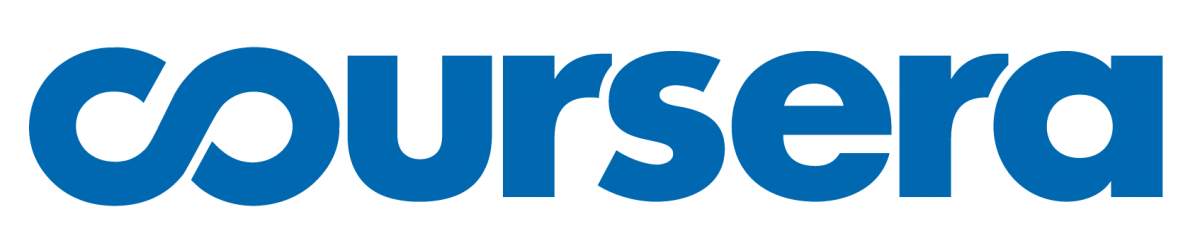
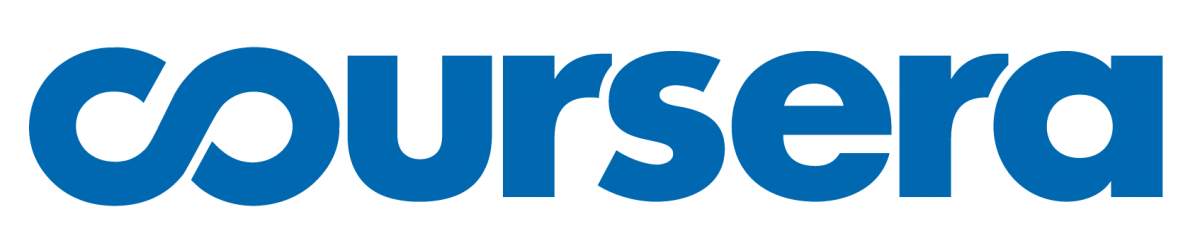
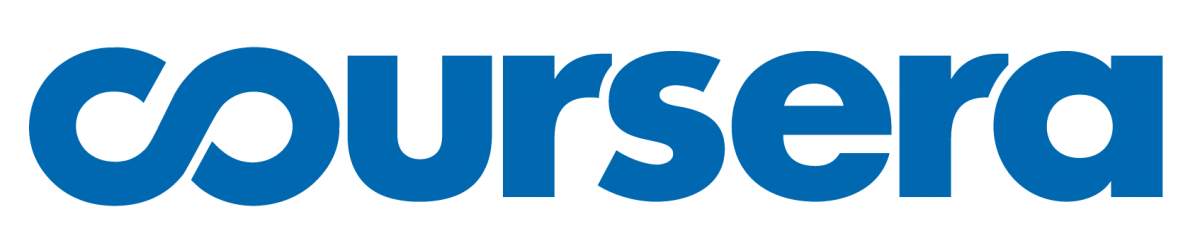
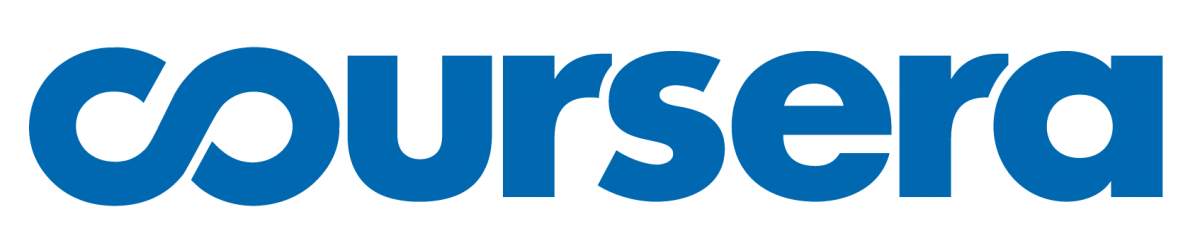
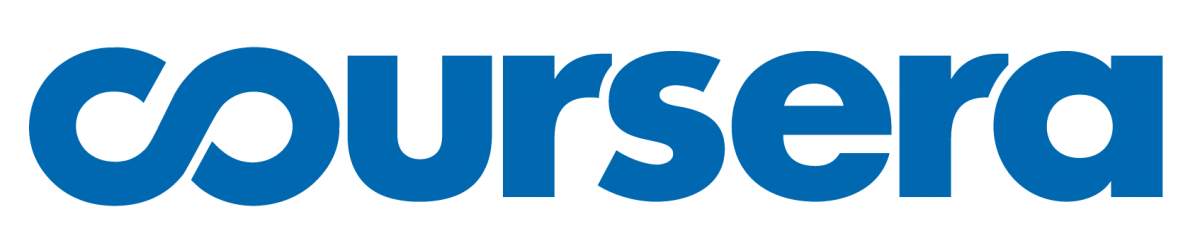
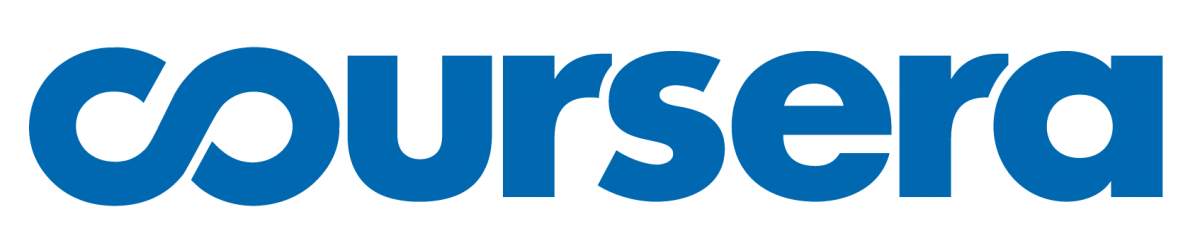
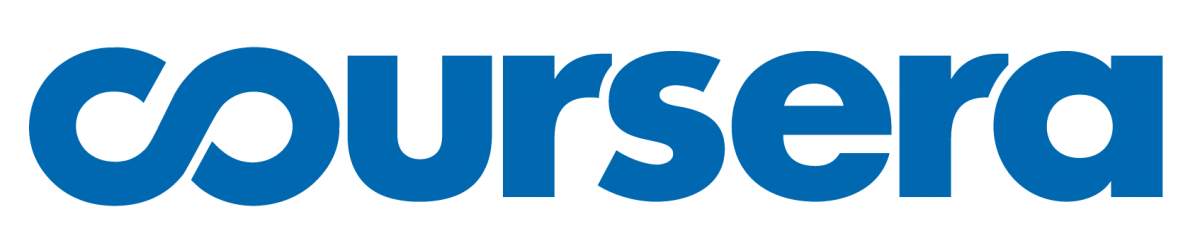
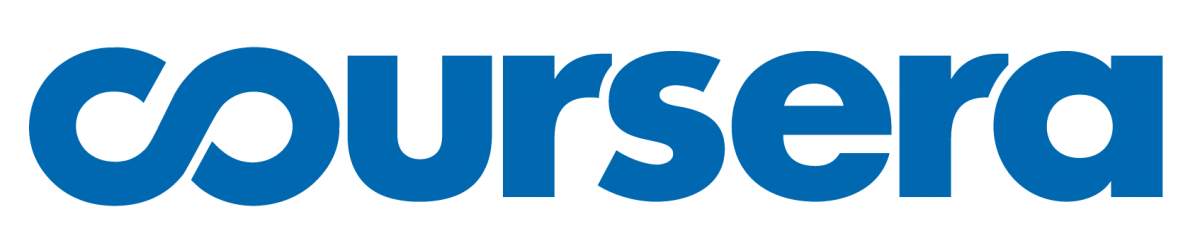
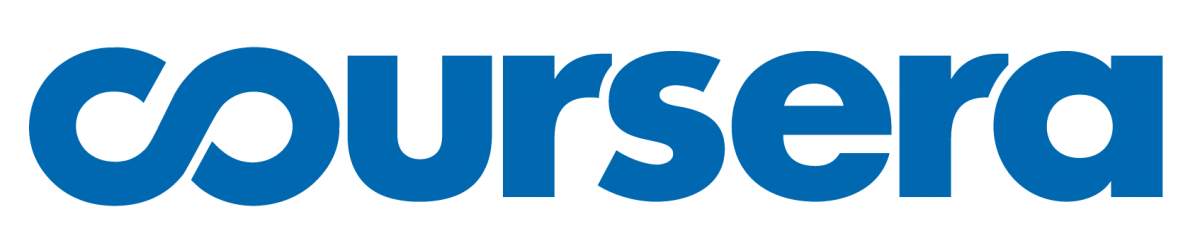
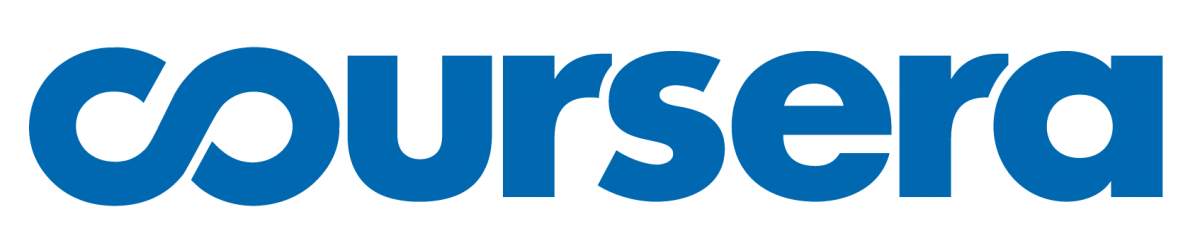
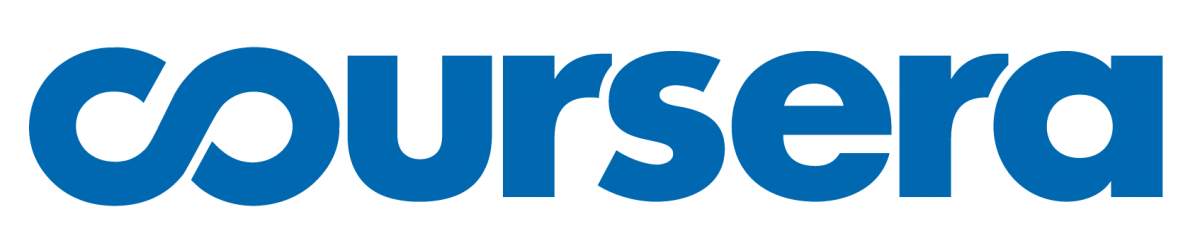
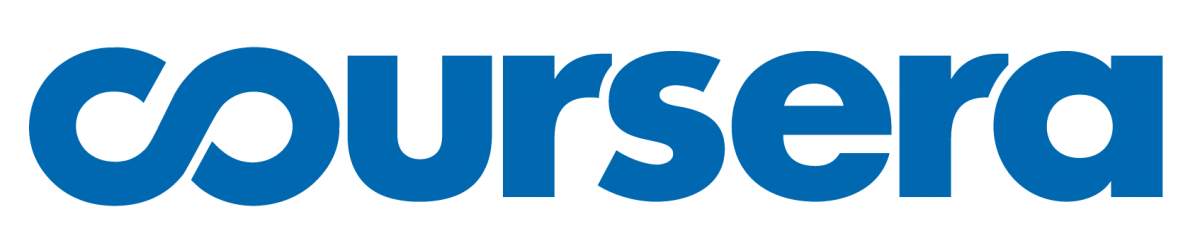
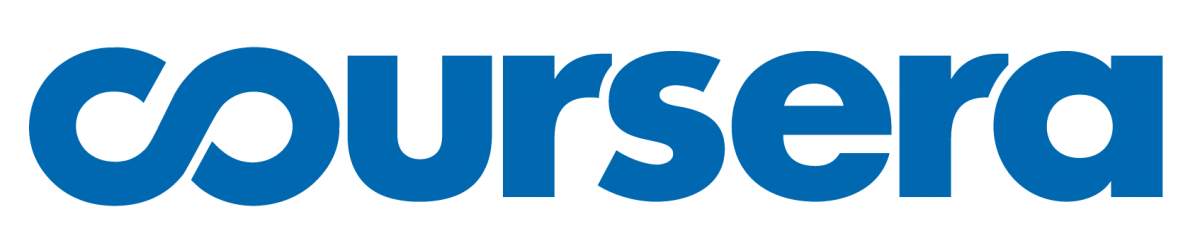
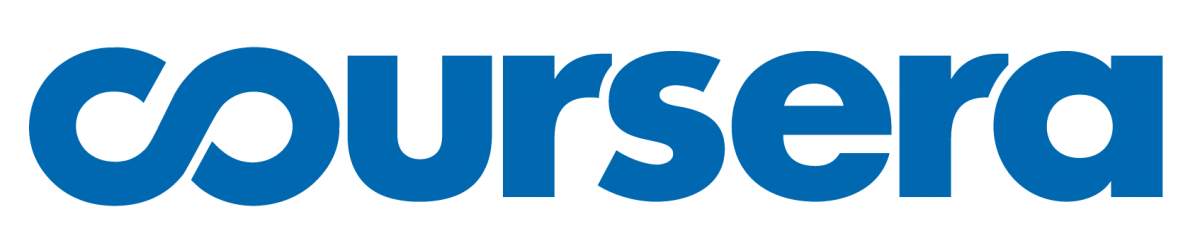
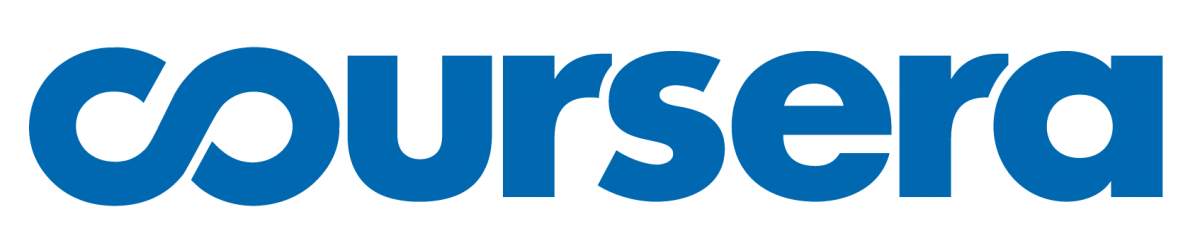
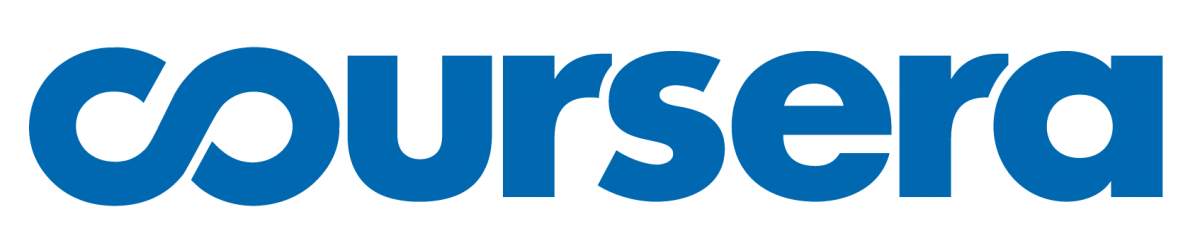
Description
A 4-course IBM specialization that teaches students advanced concepts in Data Science. Students will gain a deep understanding on massive parallel data processing, data exploration and visualization, and advanced machine learning & deep learning. The specialization also features a final applied data science project.
Teachers:
- IBM: Niketan Pansare, Senior Software Engineer
- IBM: Nikolay Manchev, Data Scientist
- IBM: Romeo Kienzler, Chief Data Scientist
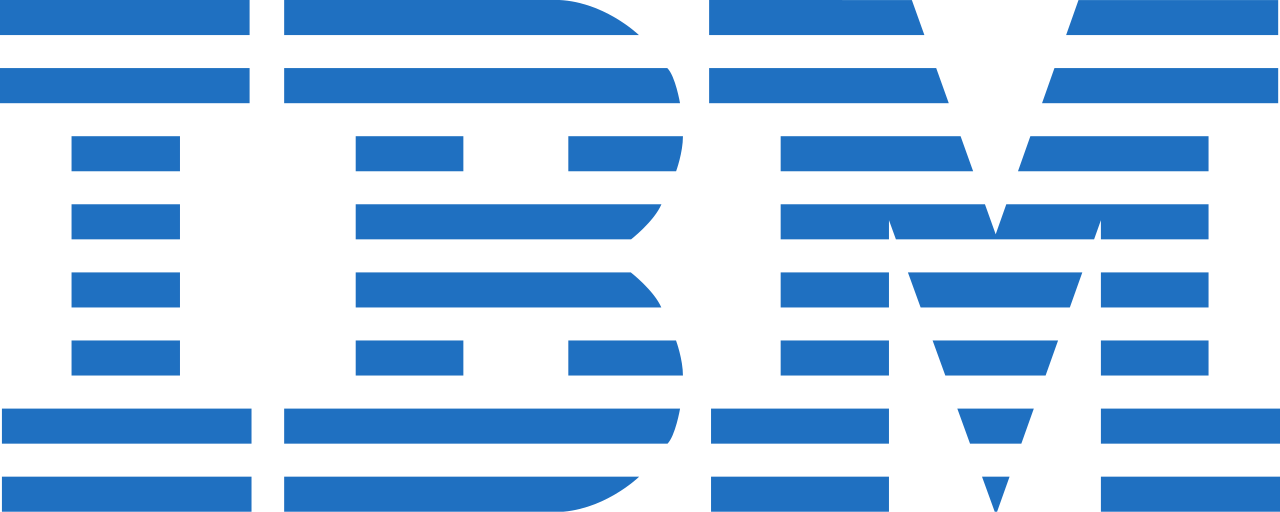
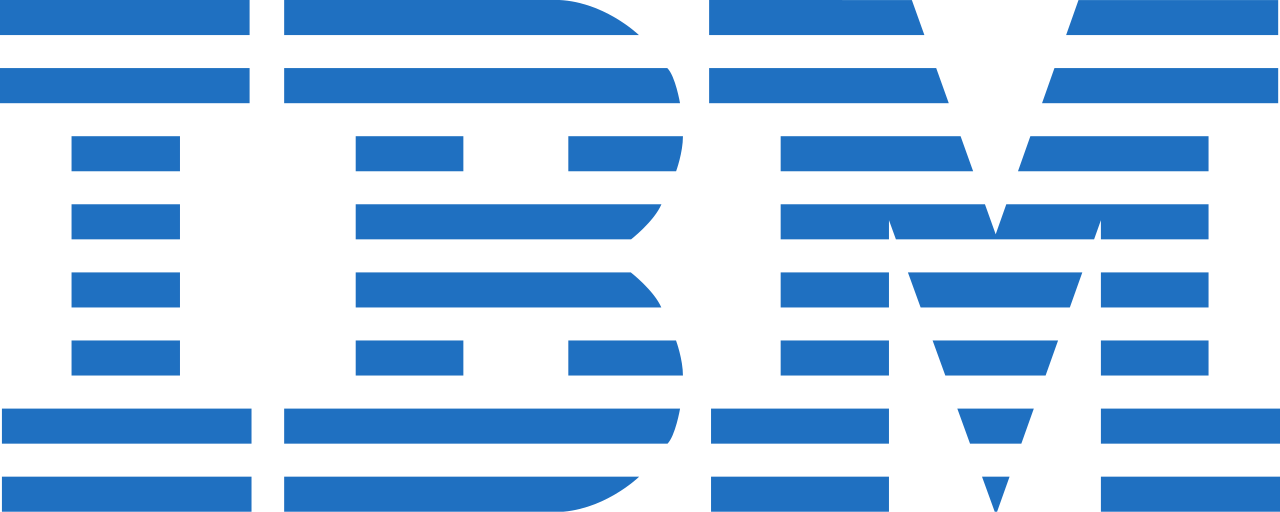
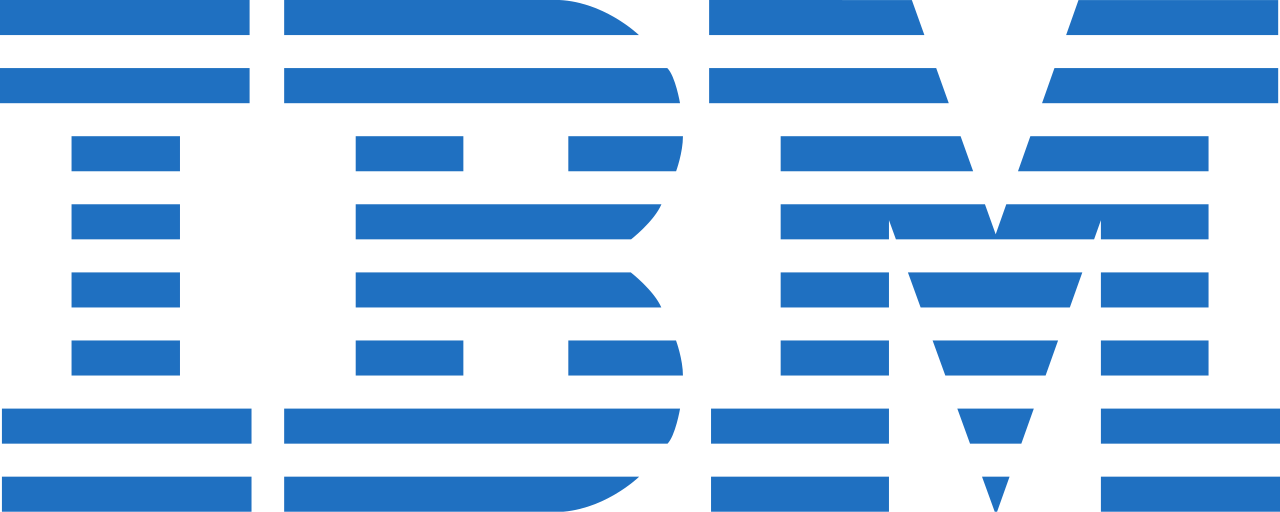
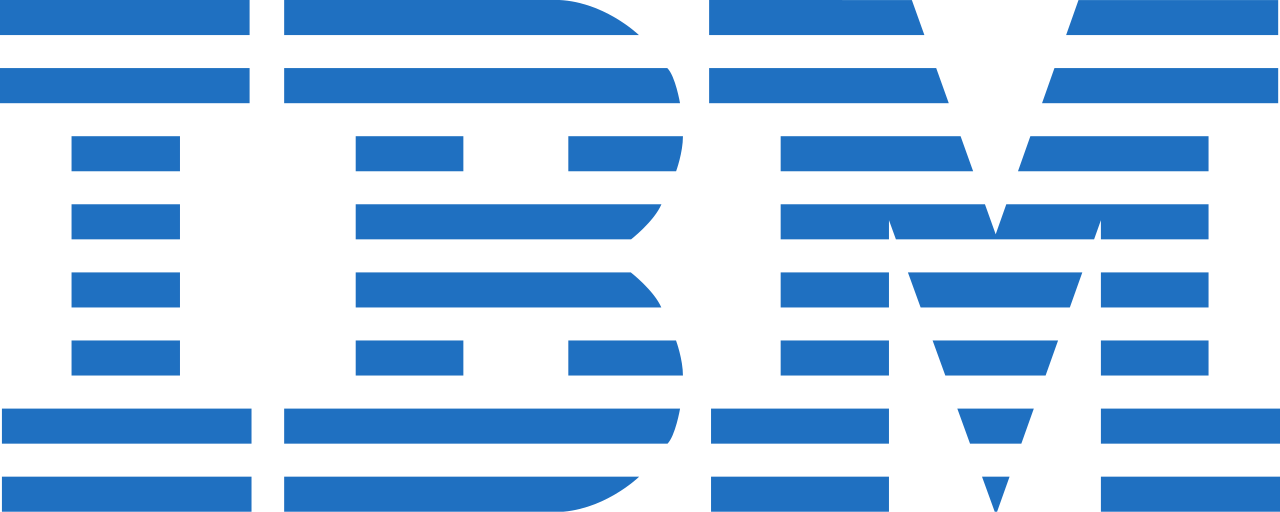
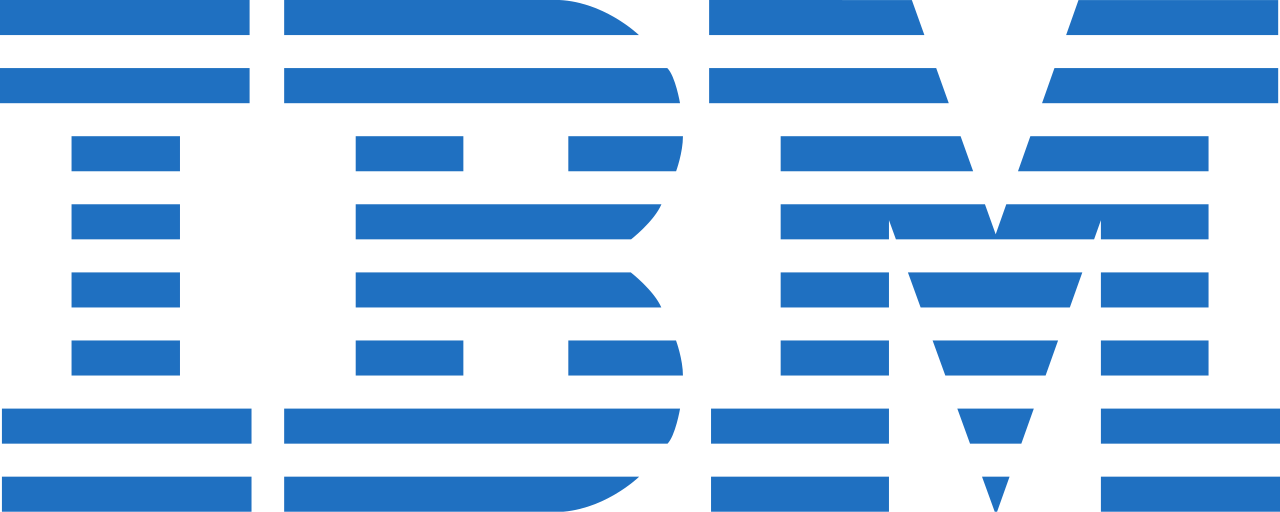
IBM Data Science Professional Certificate
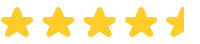
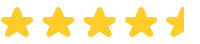
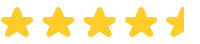
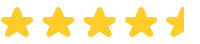
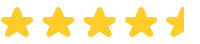
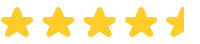
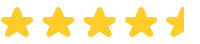
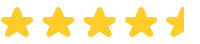
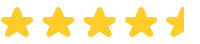
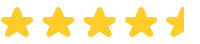
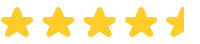
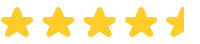
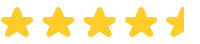
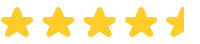
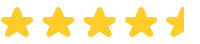
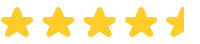
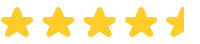
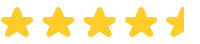
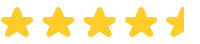
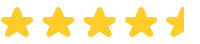
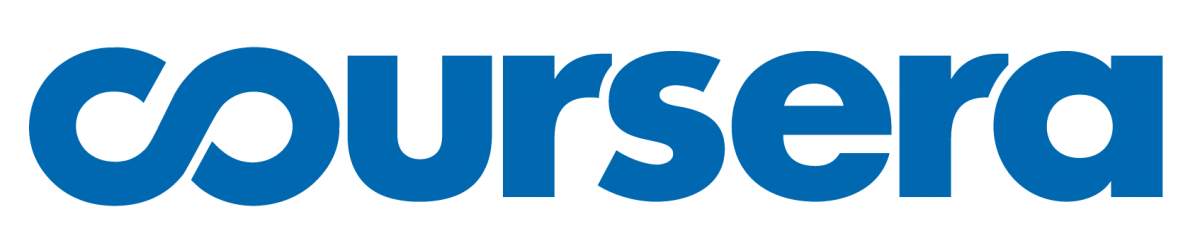
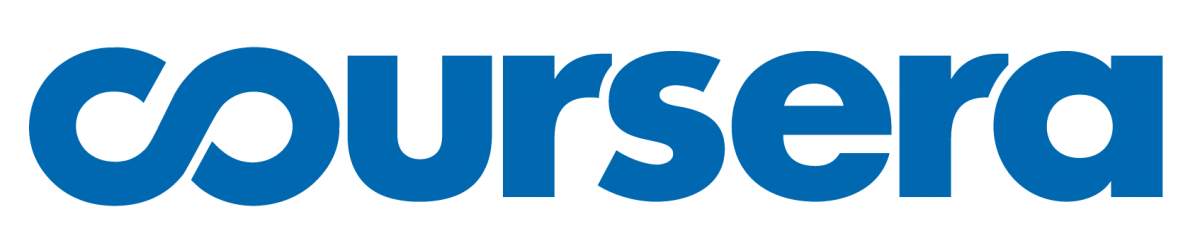
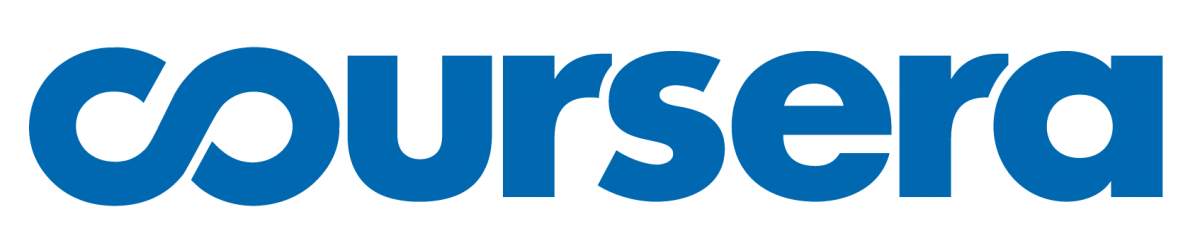
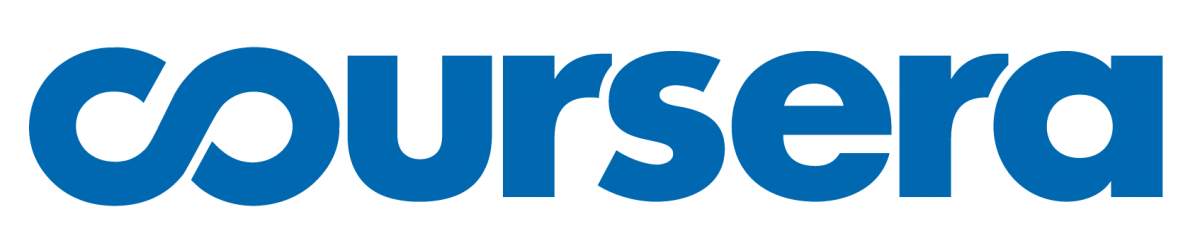
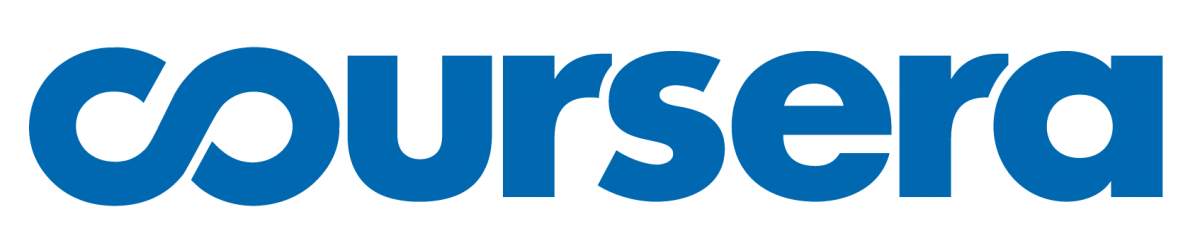
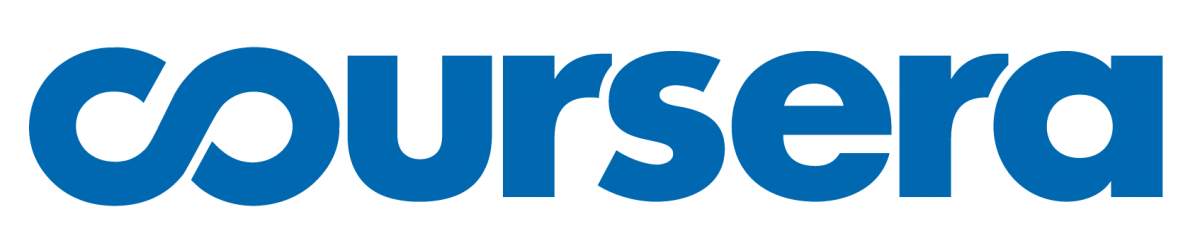
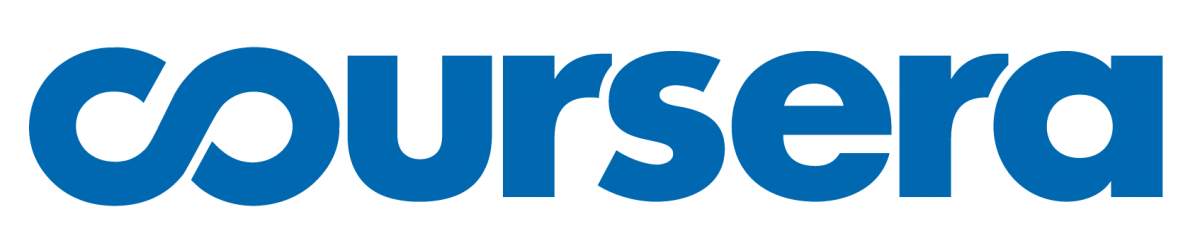
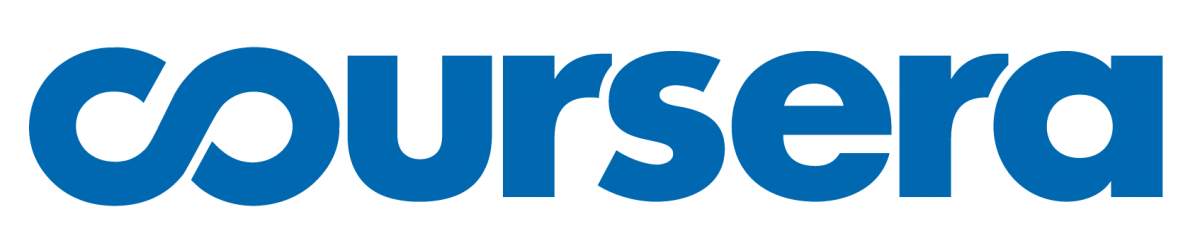
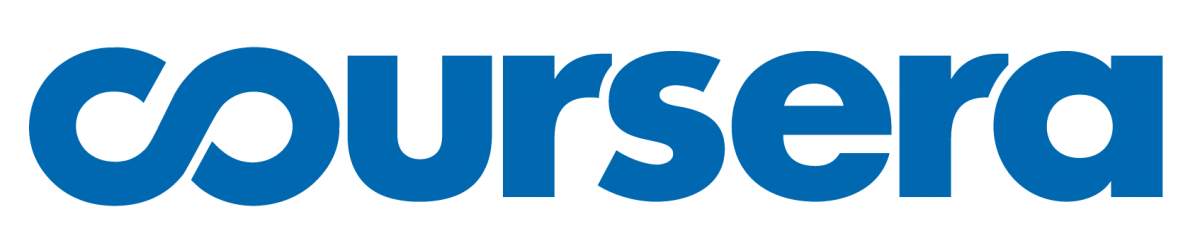
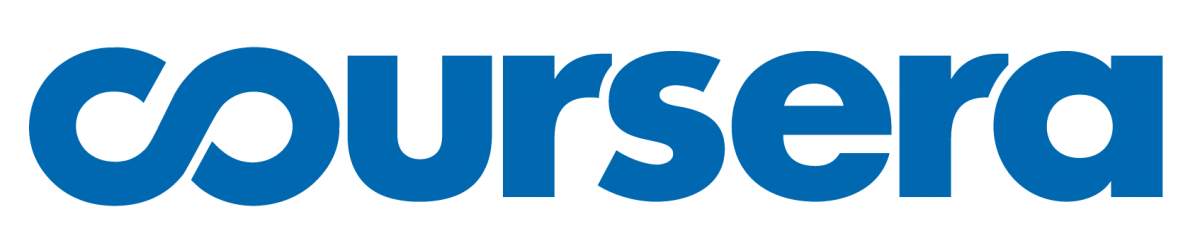
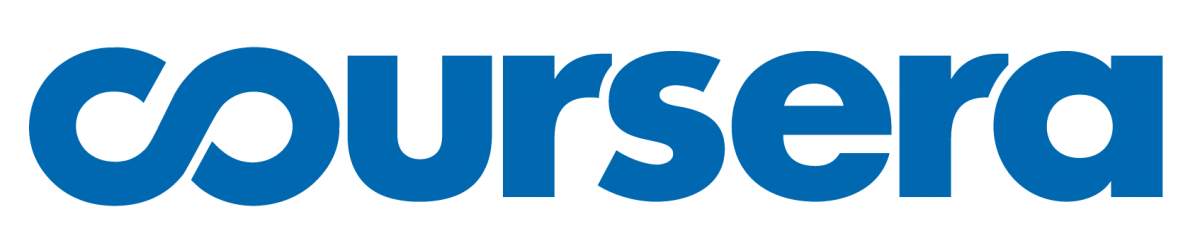
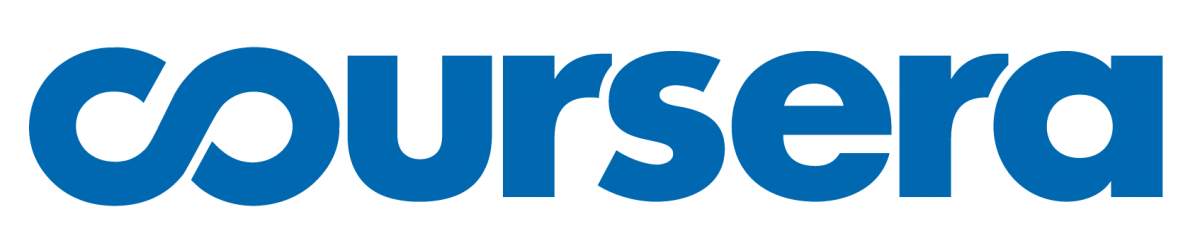
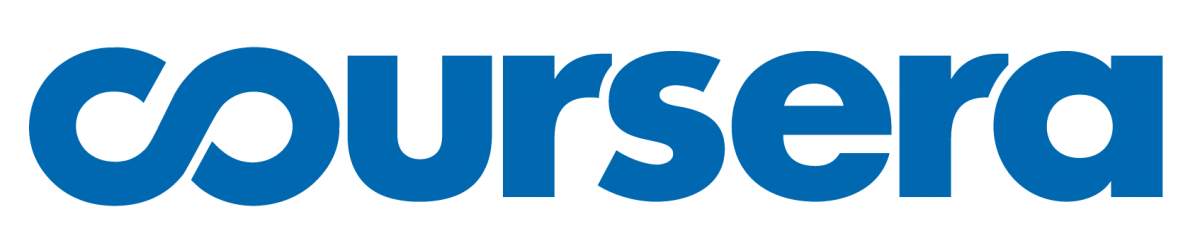
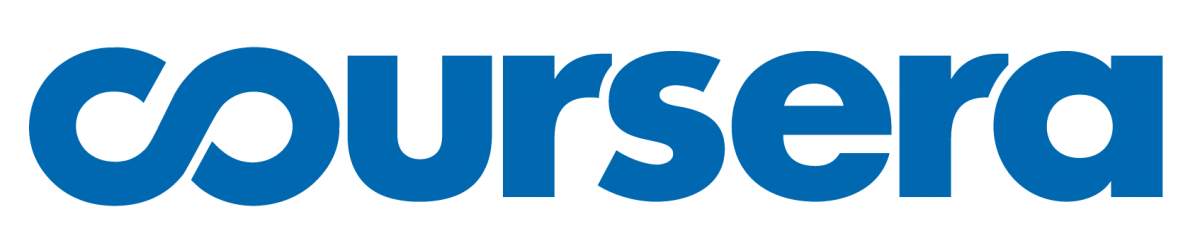
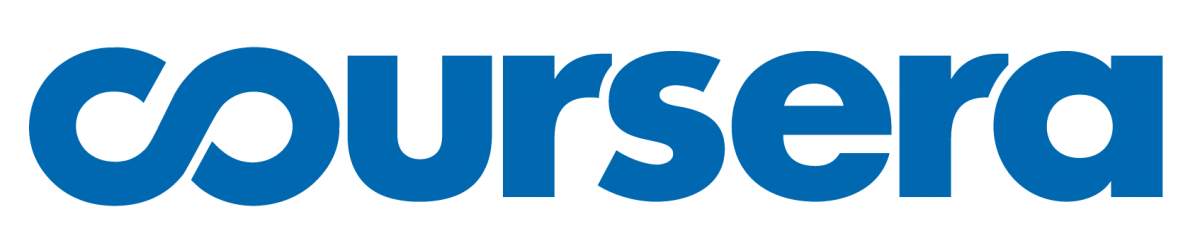
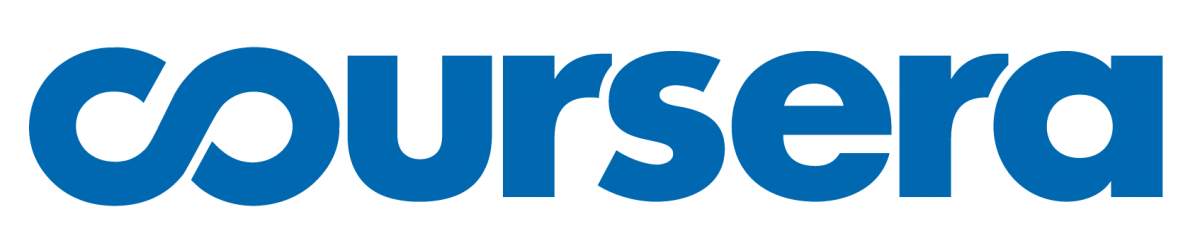
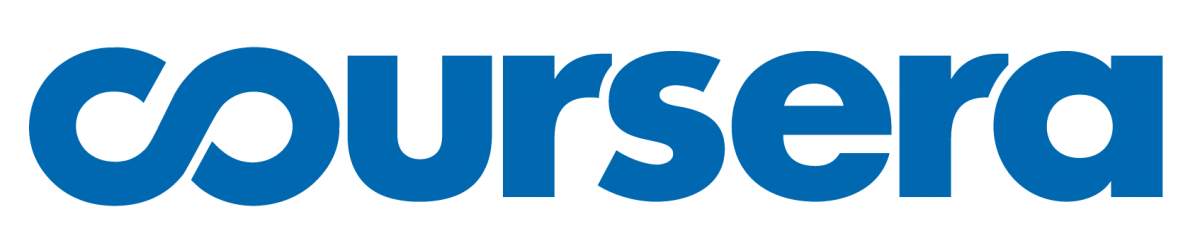
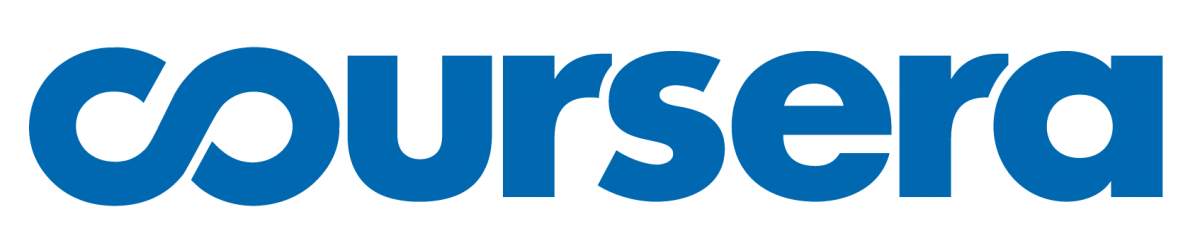
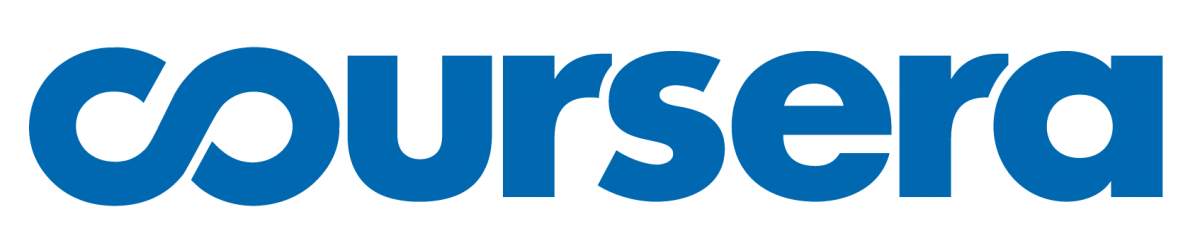
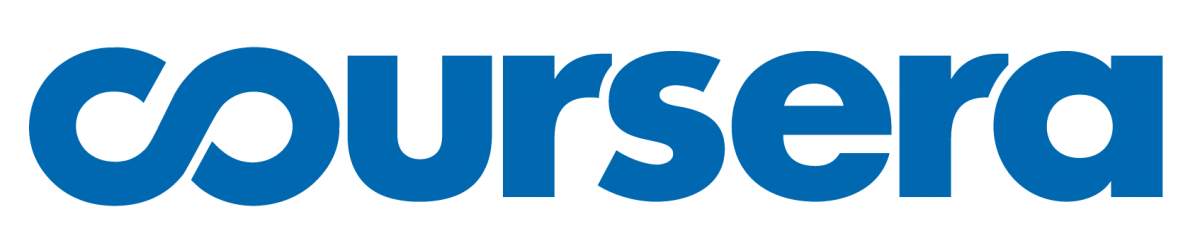
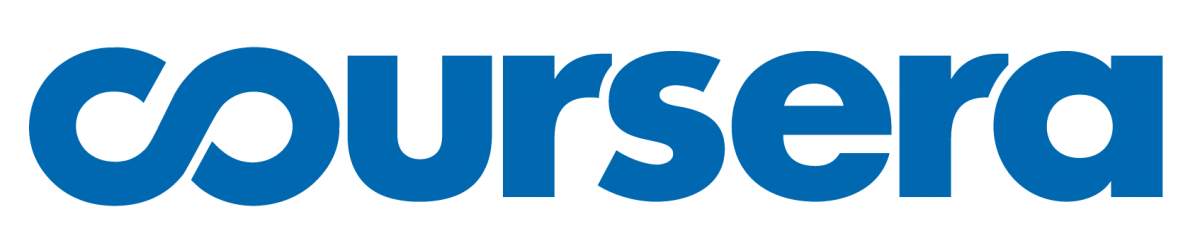
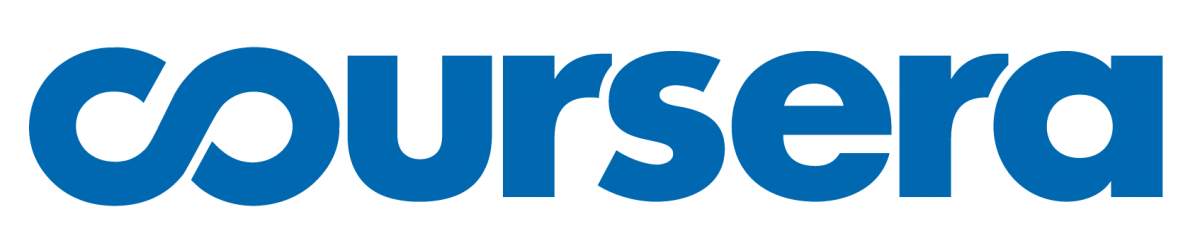
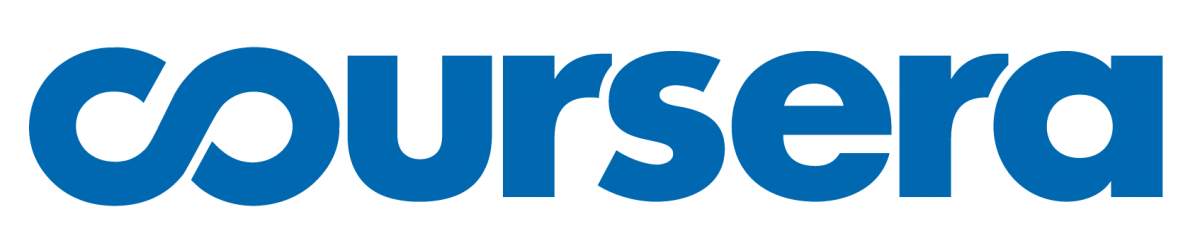
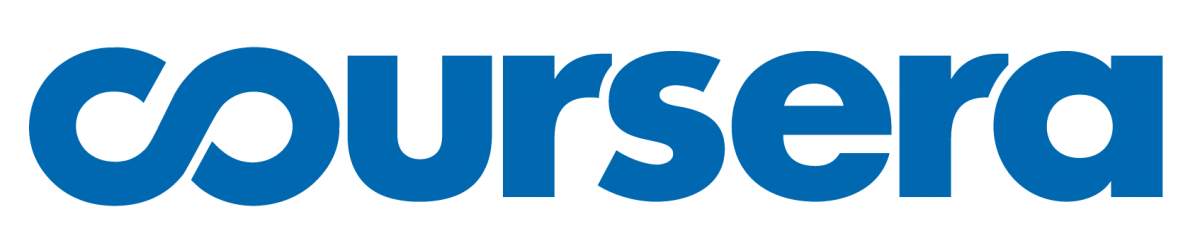
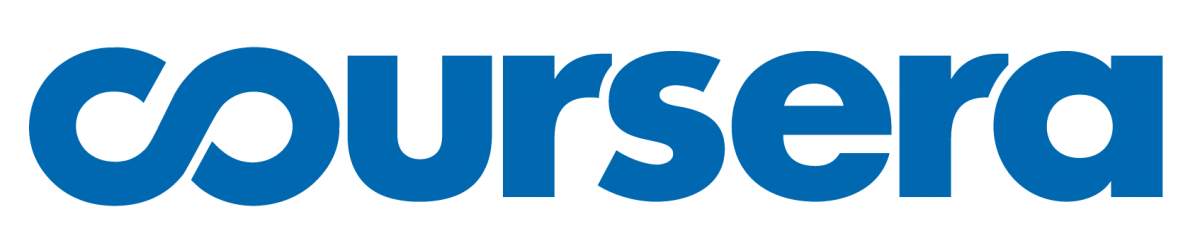
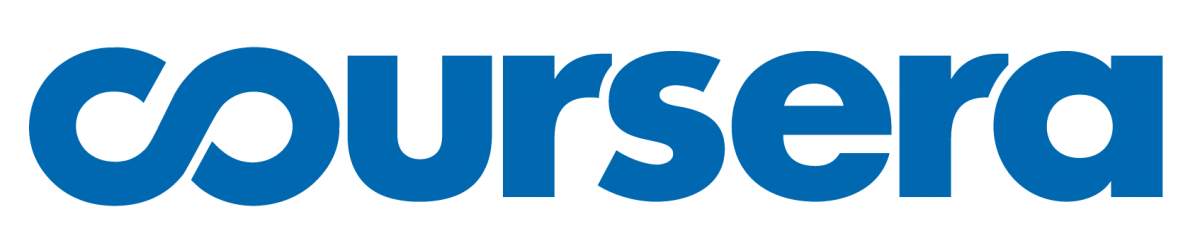
Description
A specialization that allows students to acquire the latest job-ready skills and techniques covering a wide array of data science topics including open source tools and libraries, methodologies, Python, databases, SQL, data visualization, data analysis, and machine learning. It is offered by IBM and consists of 9 courses in total.
Teachers:
- IBM: Joseph Santarcangelo, Ph.D., Data Scientist
- IBM: Alex Aklson, Ph.D., Data Scientist
- IBM: Rav Ahuja, Data Science Program Manager
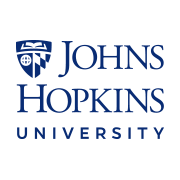
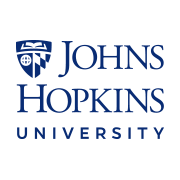
Data Science
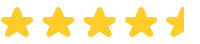
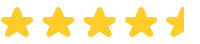
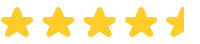
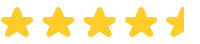
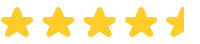
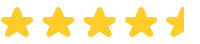
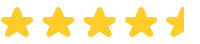
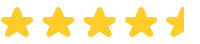
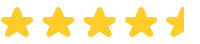
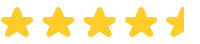
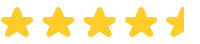
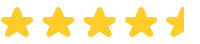
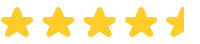
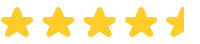
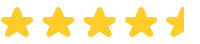
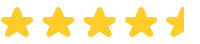
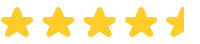
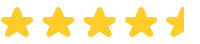
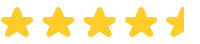
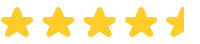
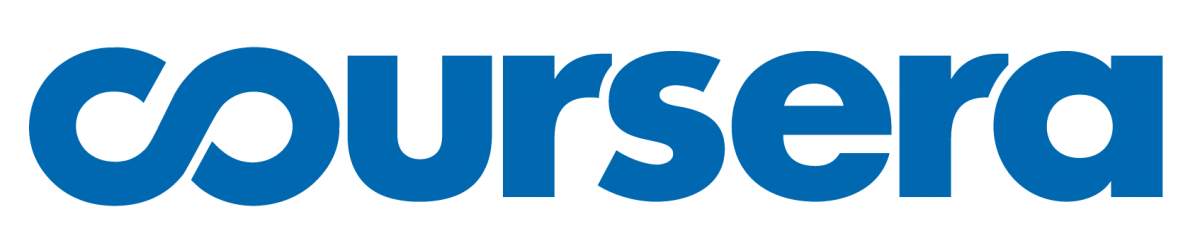
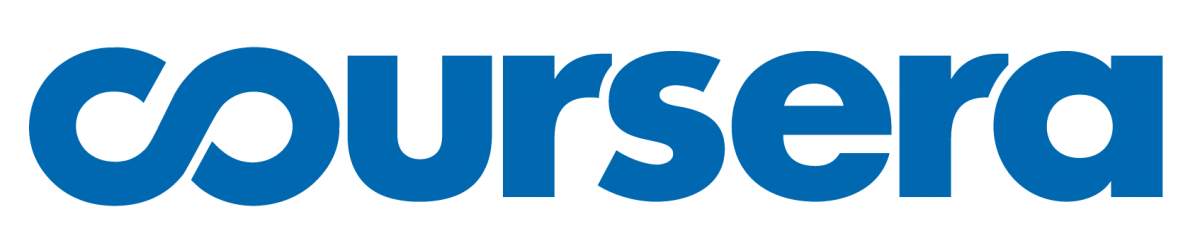
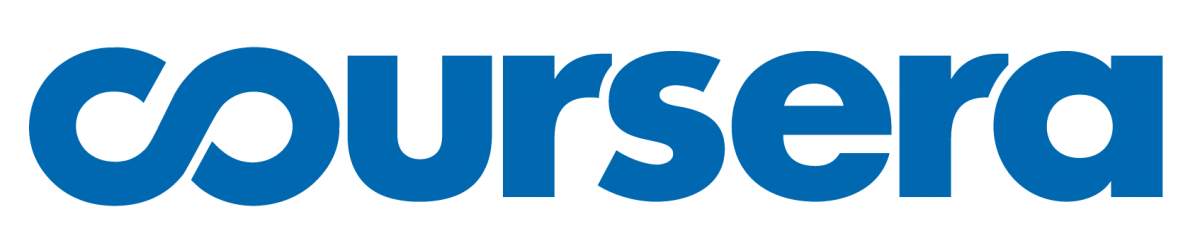
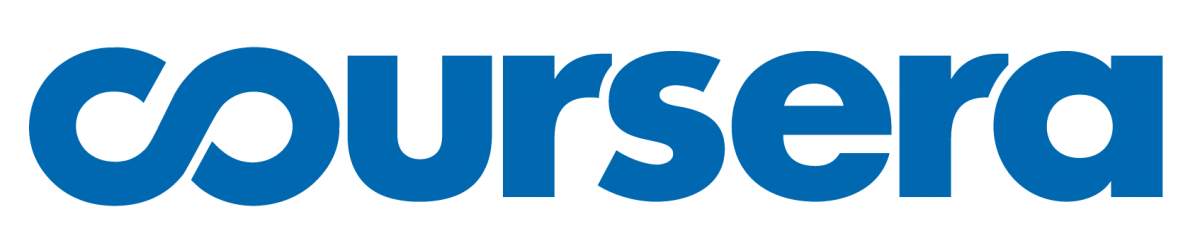
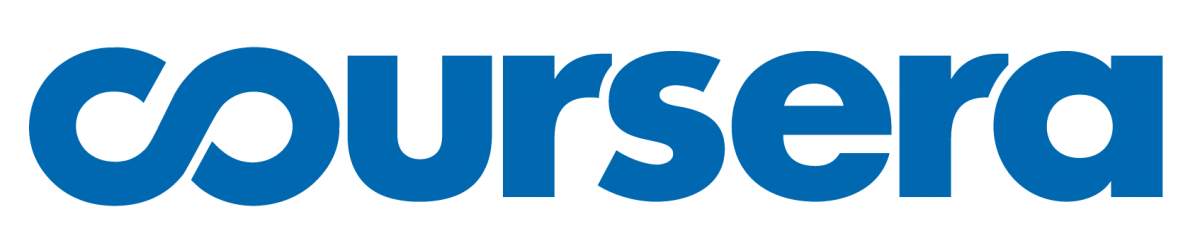
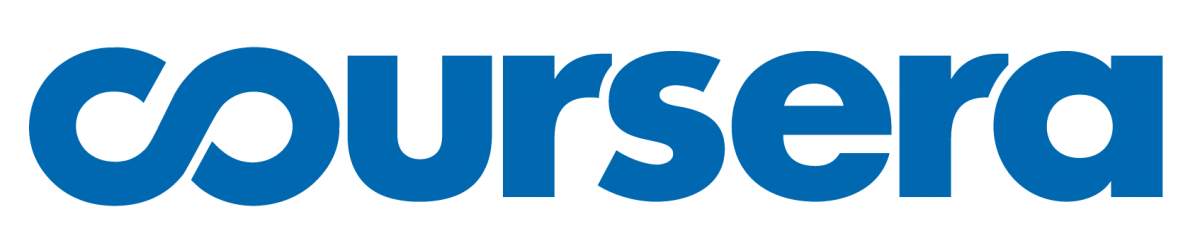
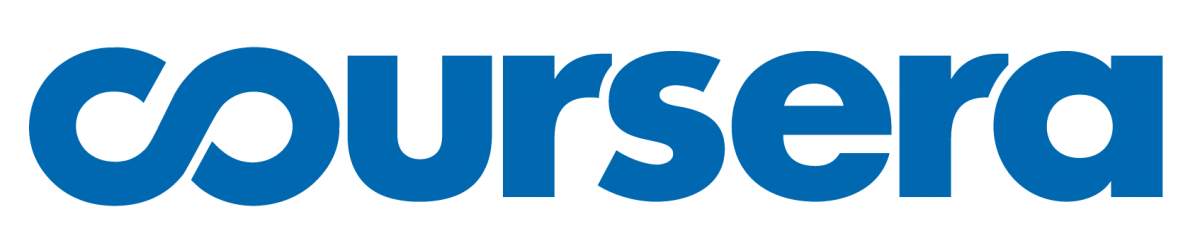
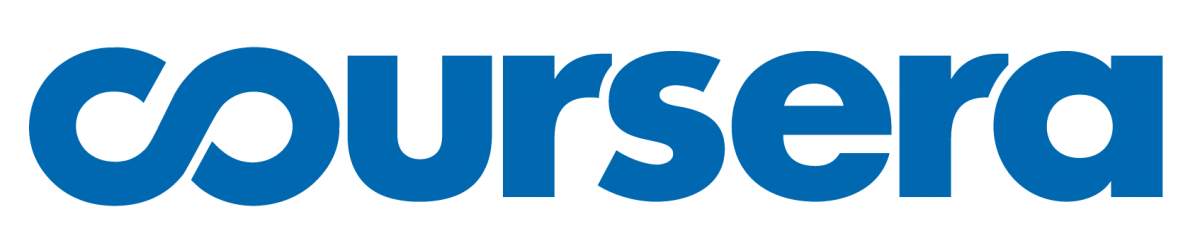
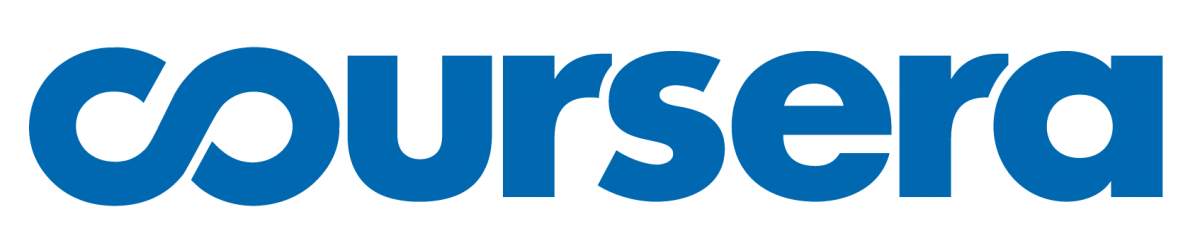
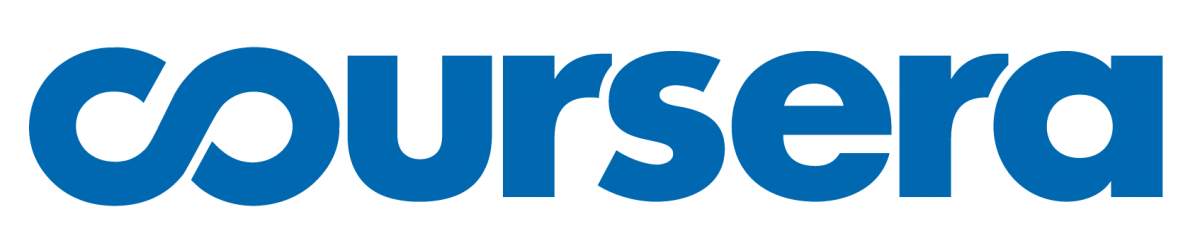
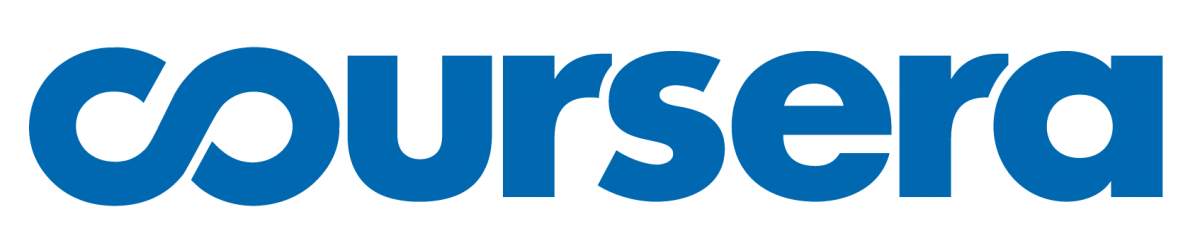
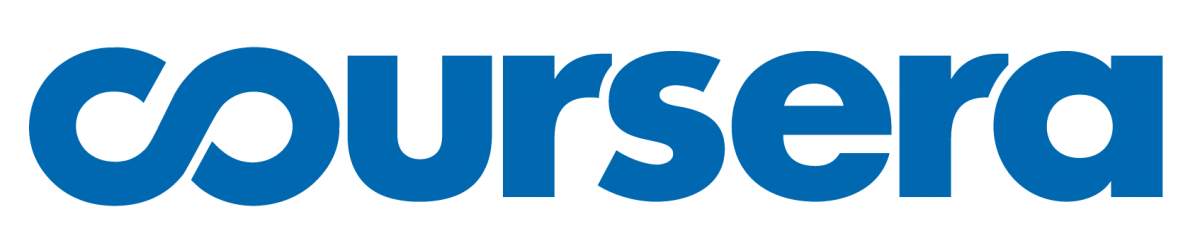
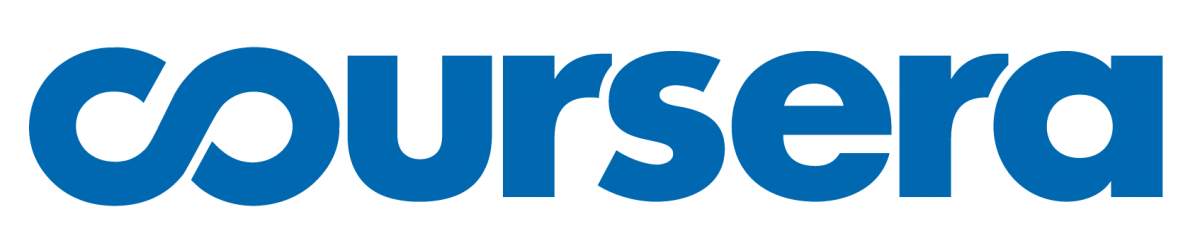
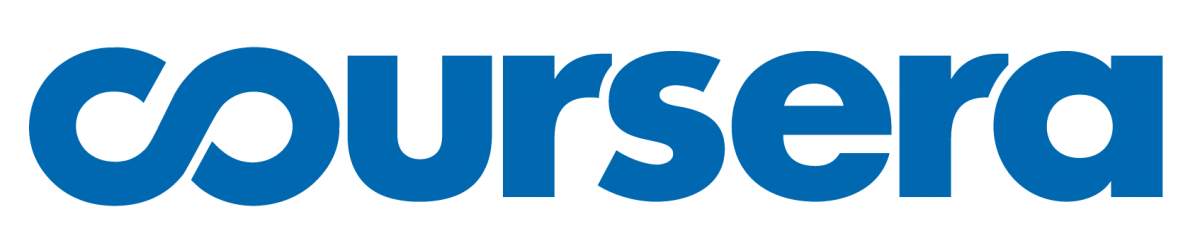
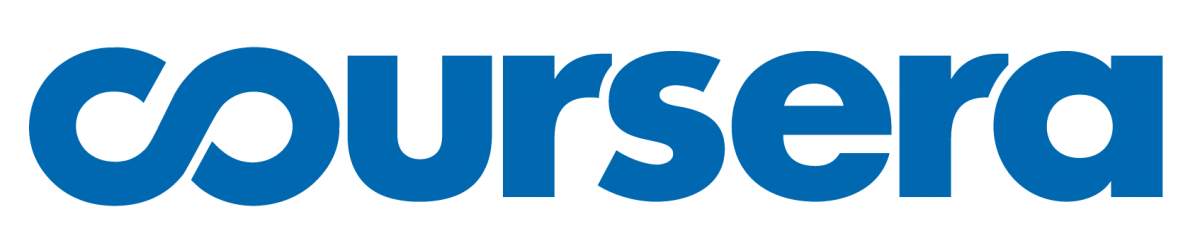
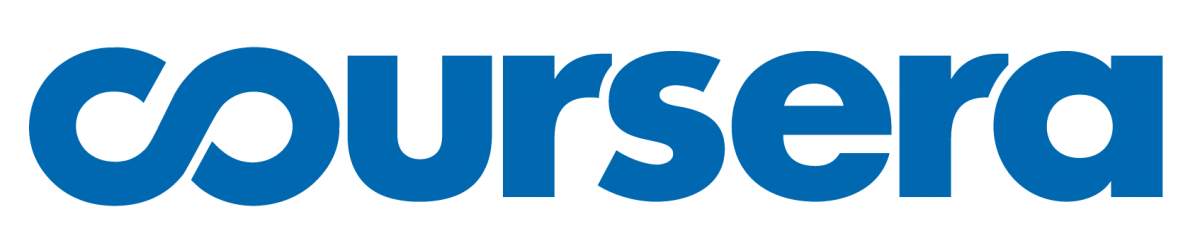
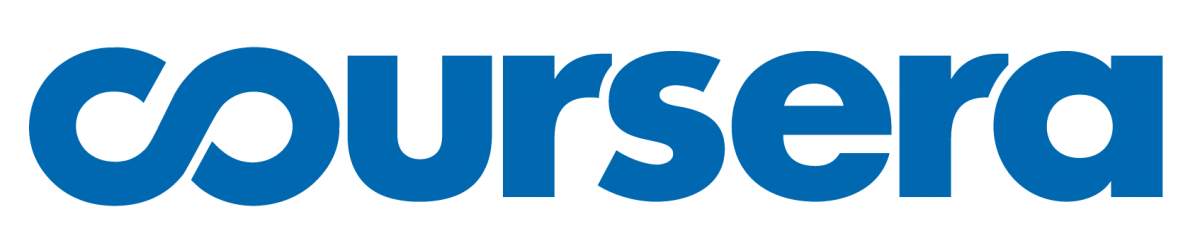
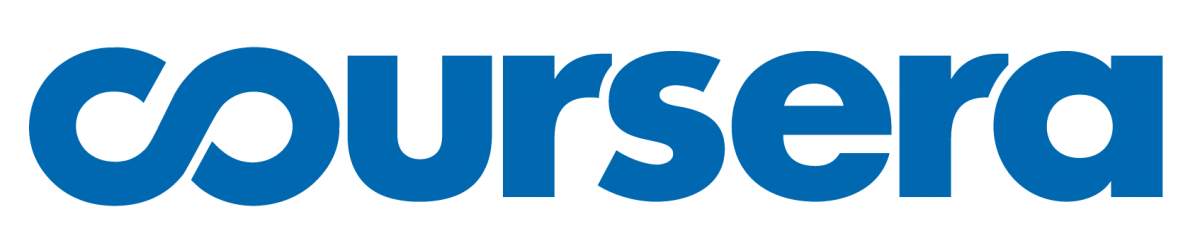
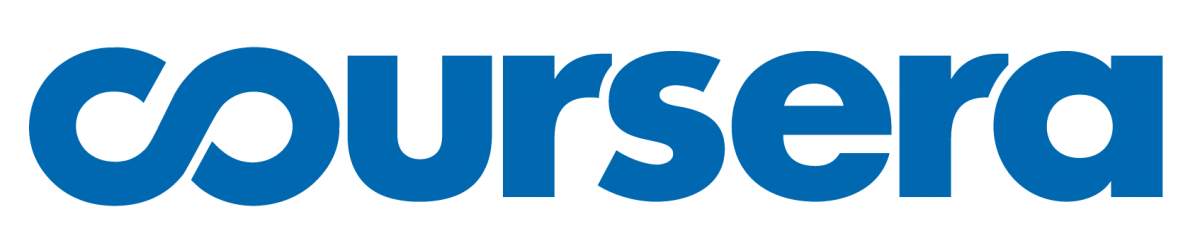
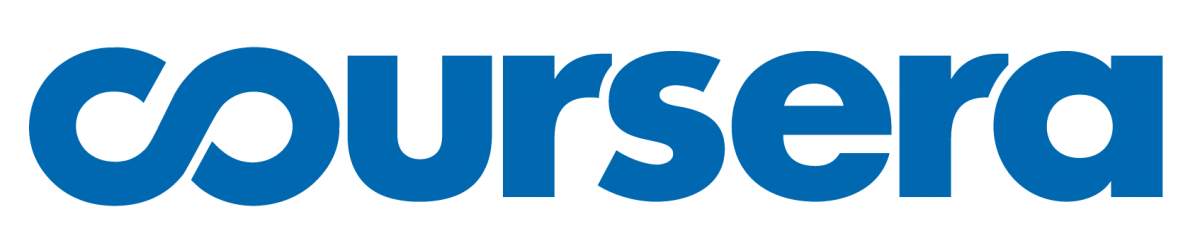
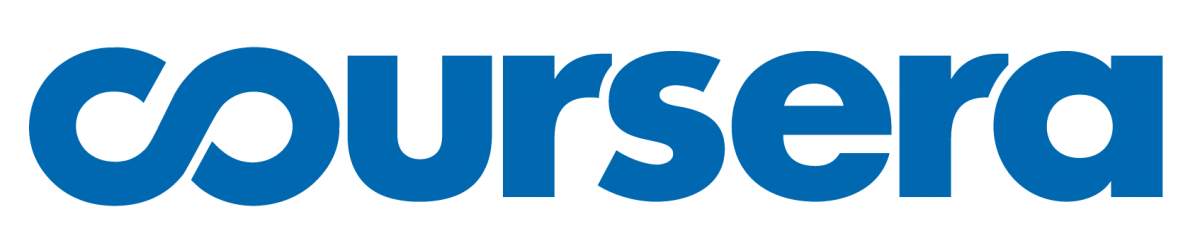
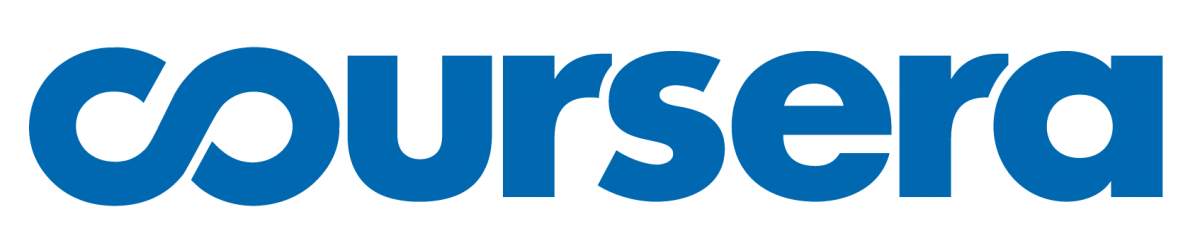
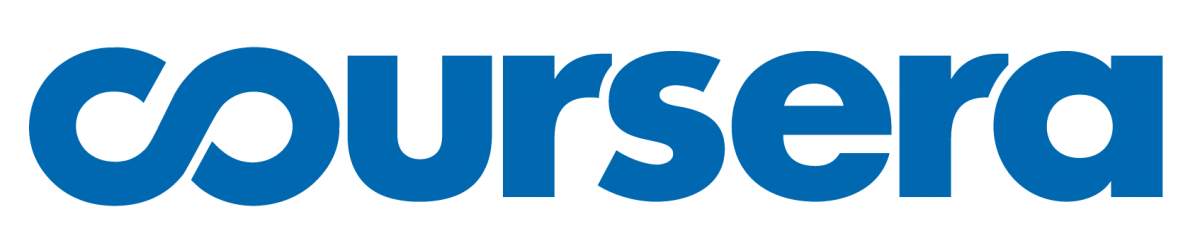
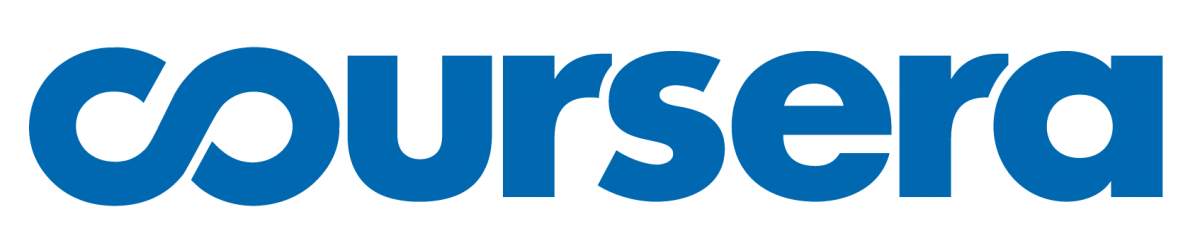
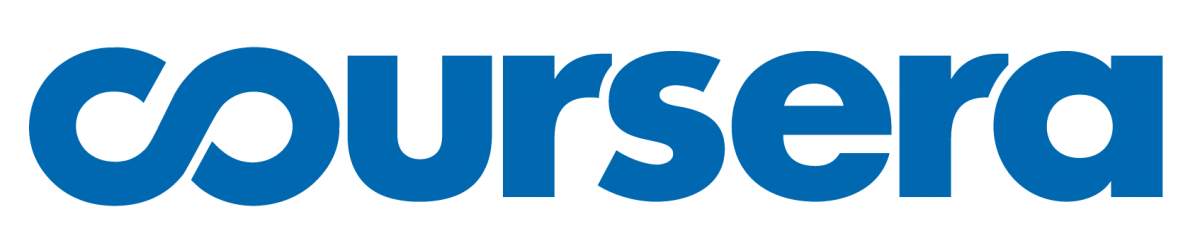
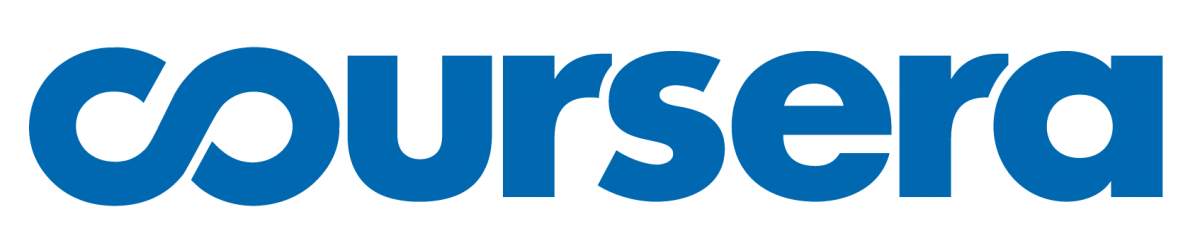
Description
Another Data Science specialization offered in Coursera. This specialization is provided by professors from the John Hopkins University and covers the concepts and tools you’ll need throughout the entire data science pipeline, from asking the right kinds of questions to making inferences and publishing results.
Teachers:
- John Hopkins University: Jeff Leek
- John Hopkins University: Roger D. Peng,
- John Hopkins University: Brian Caffo,
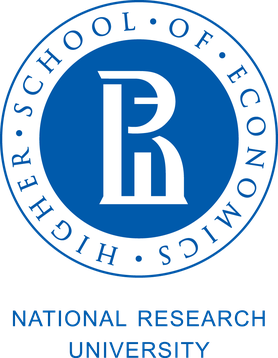
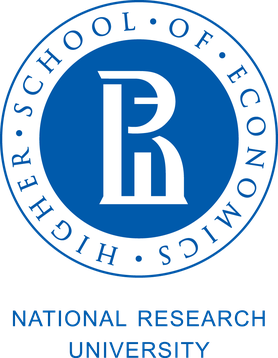
Advanced Machine Learning
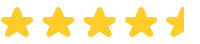
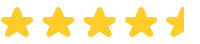
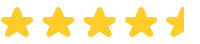
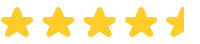
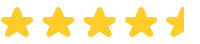
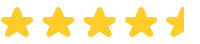
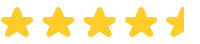
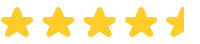
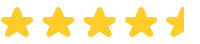
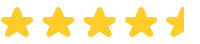
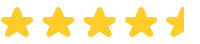
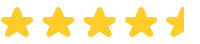
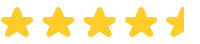
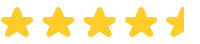
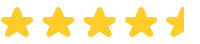
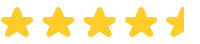
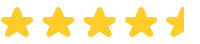
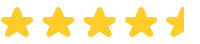
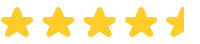
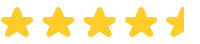
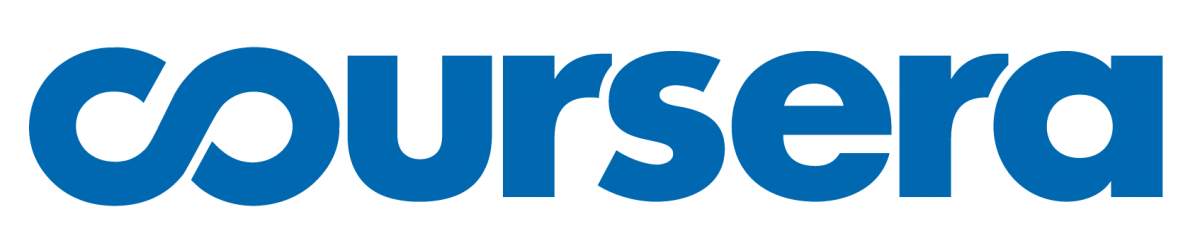
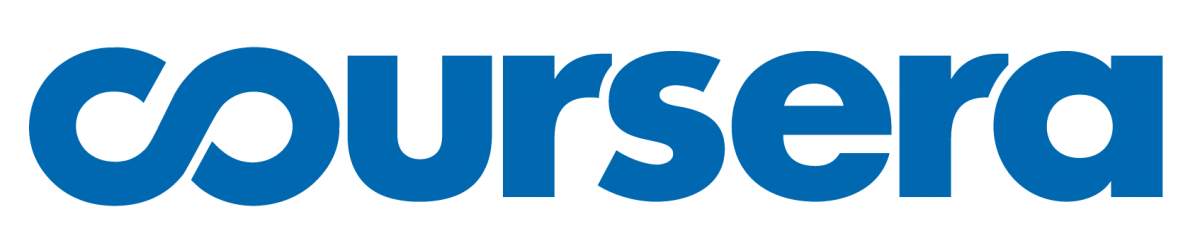
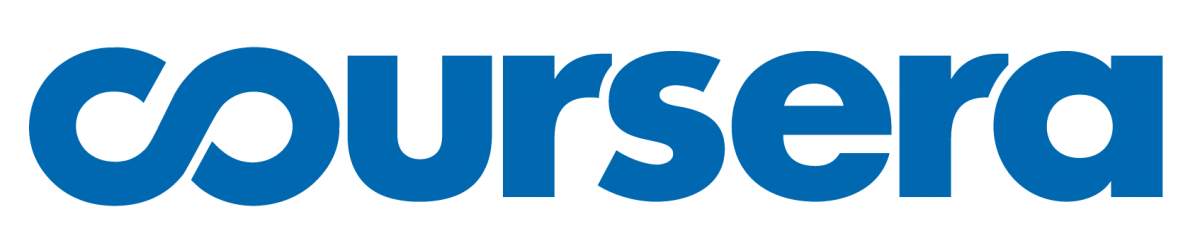
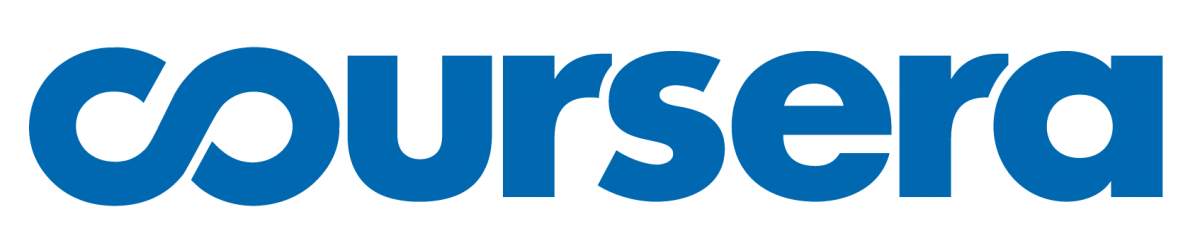
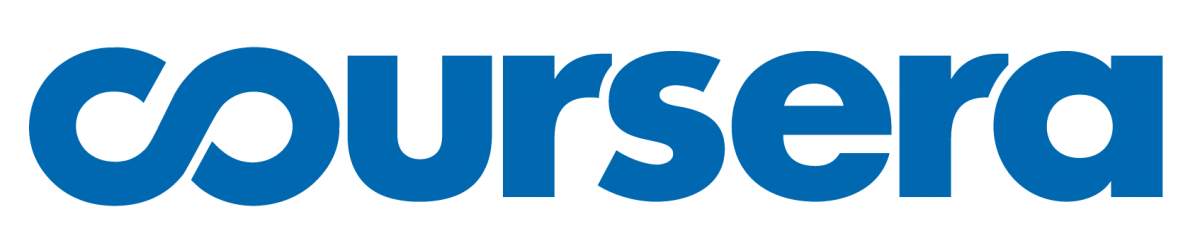
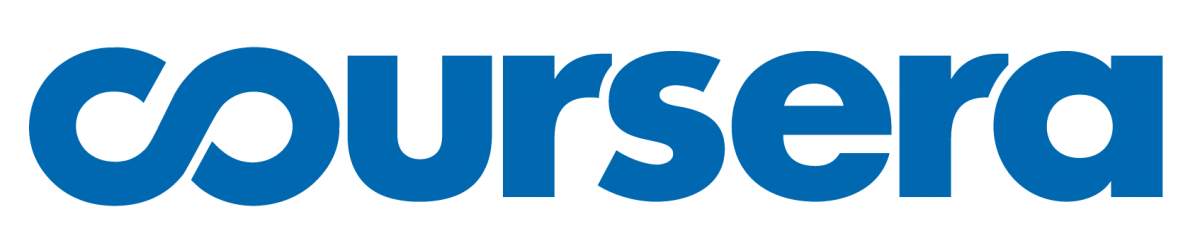
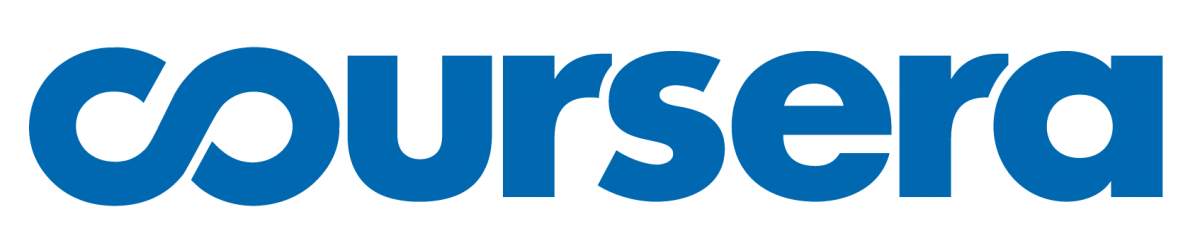
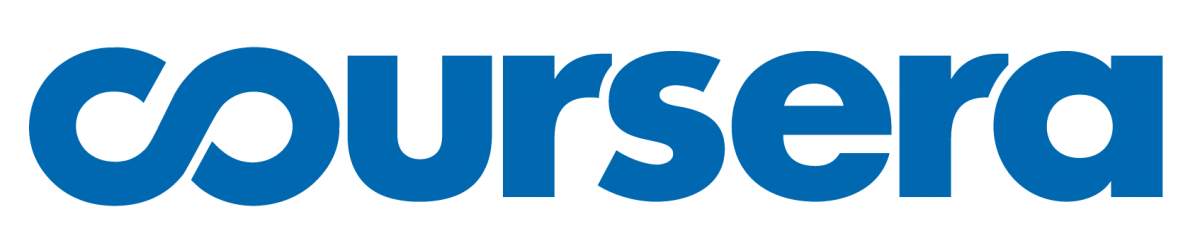
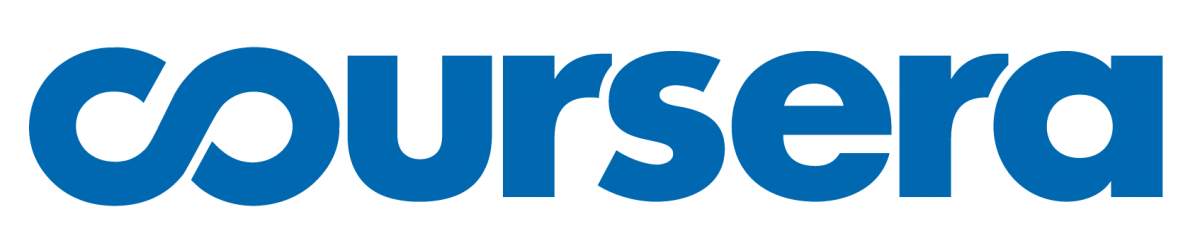
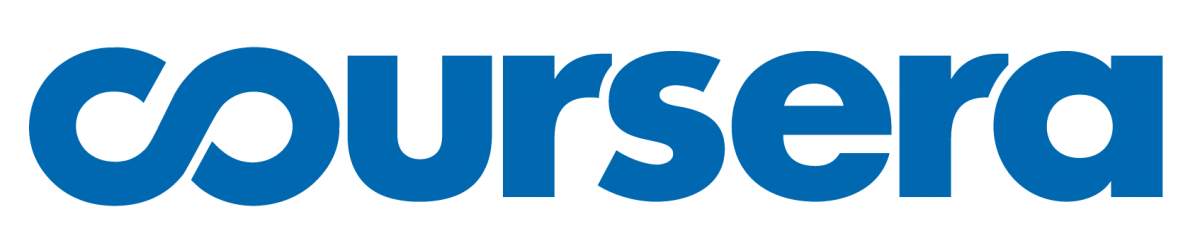
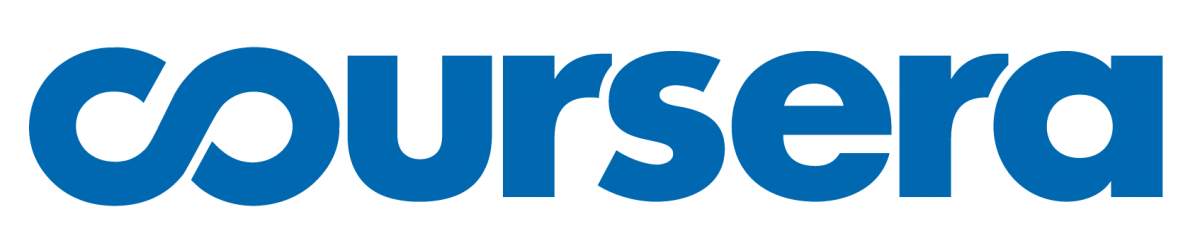
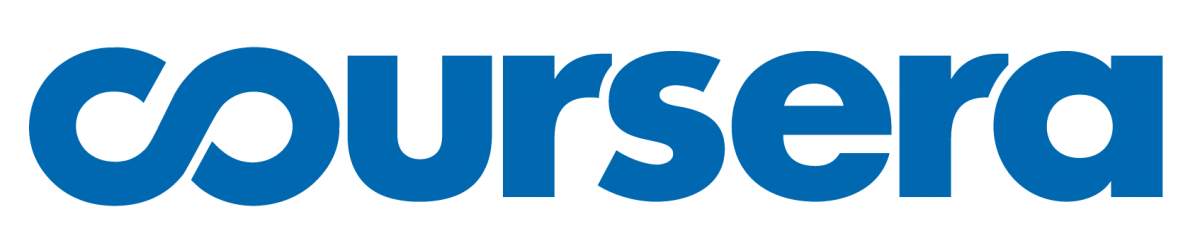
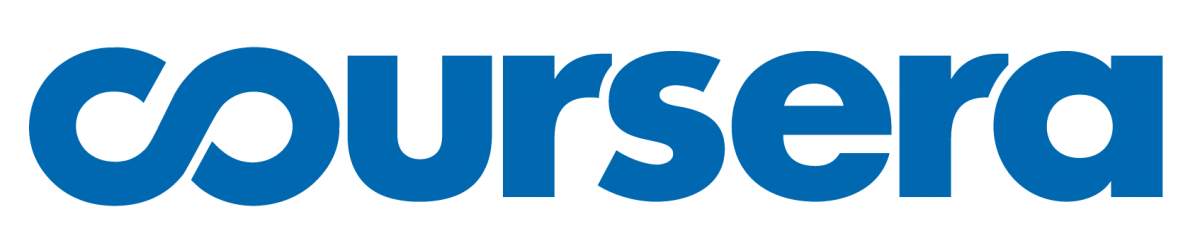
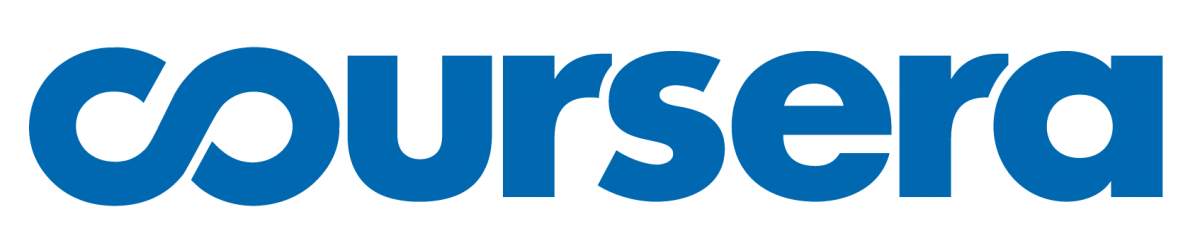
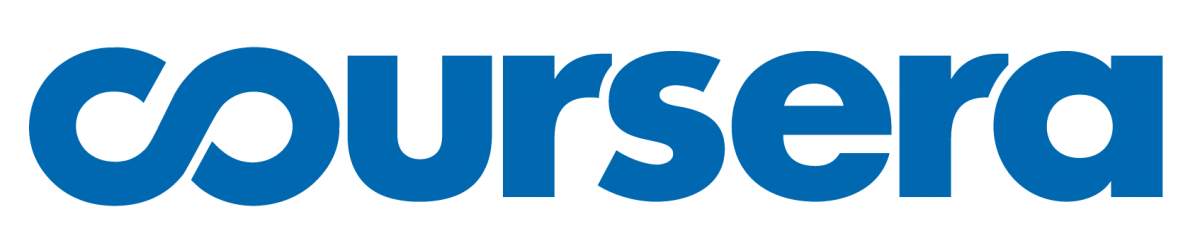
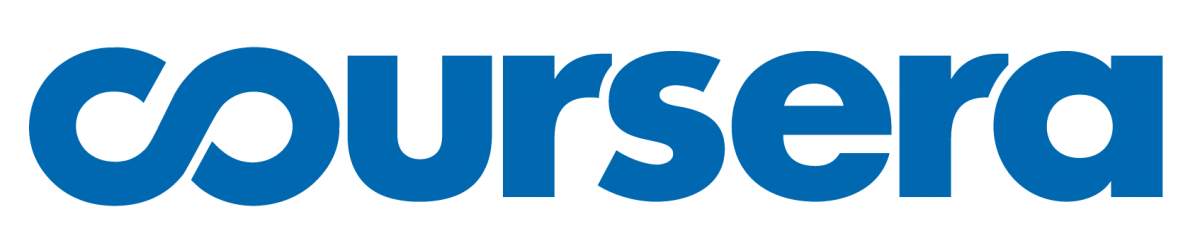
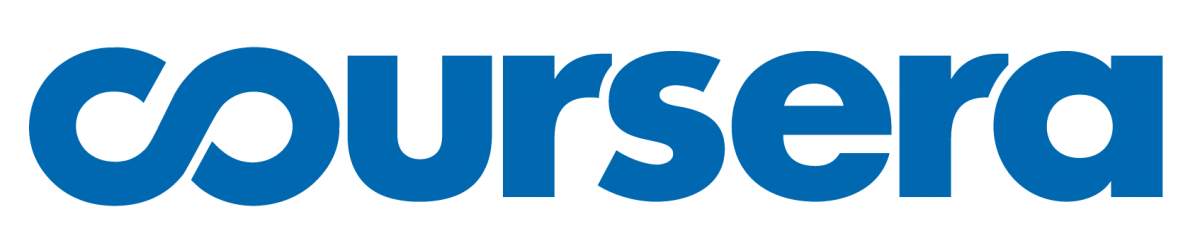
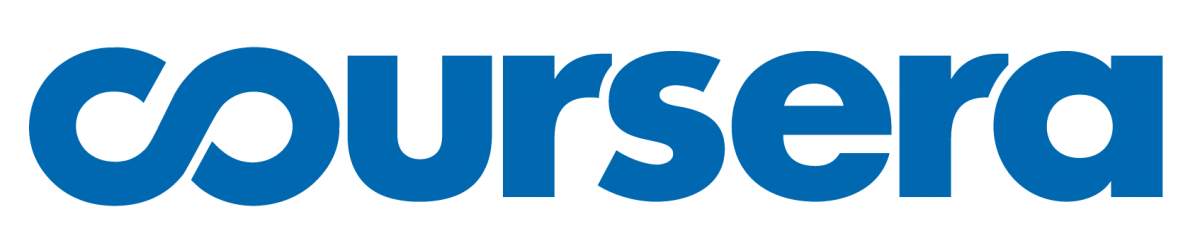
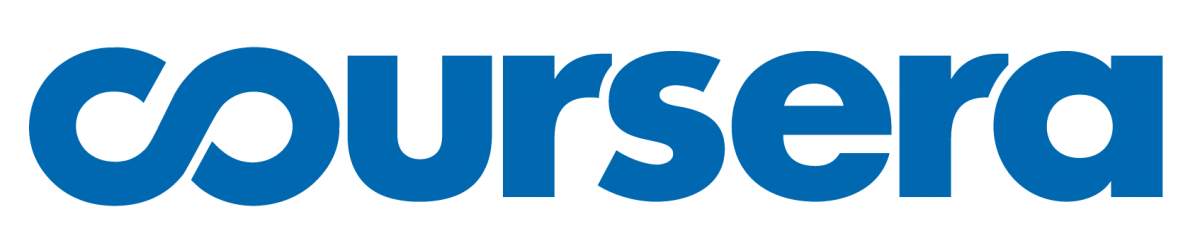
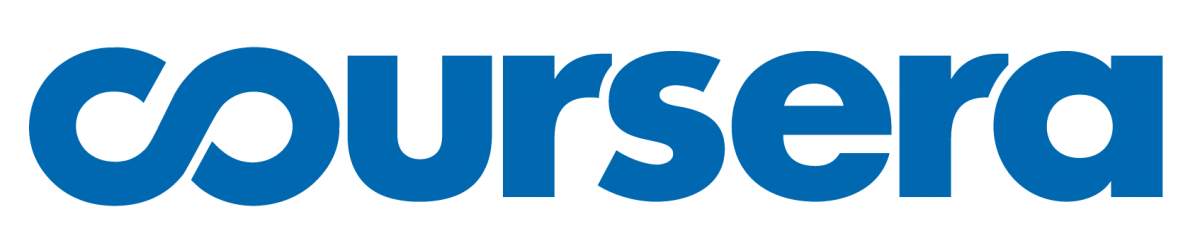
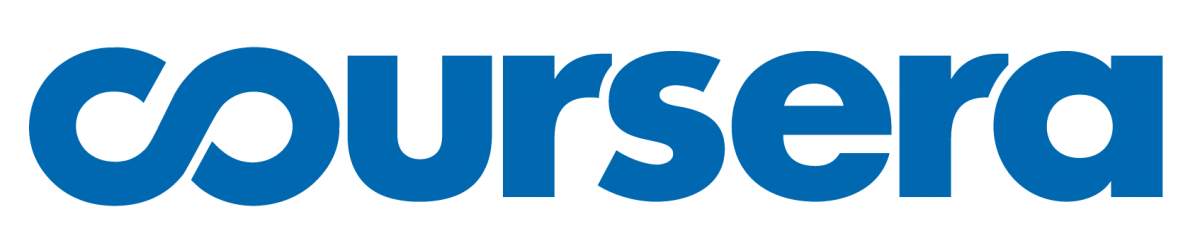
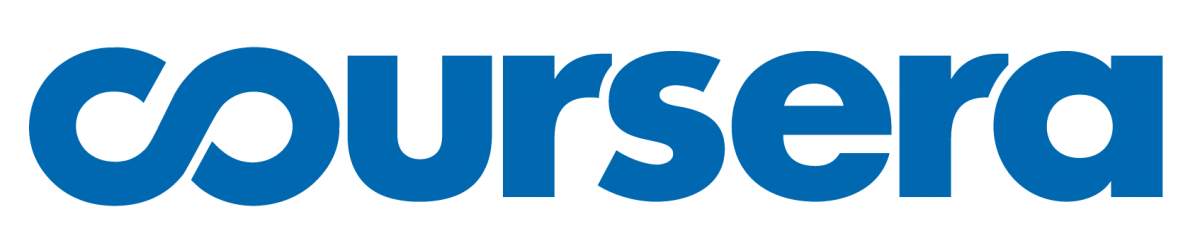
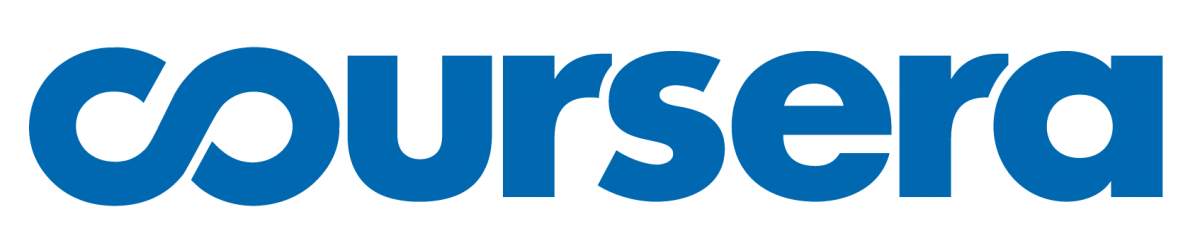
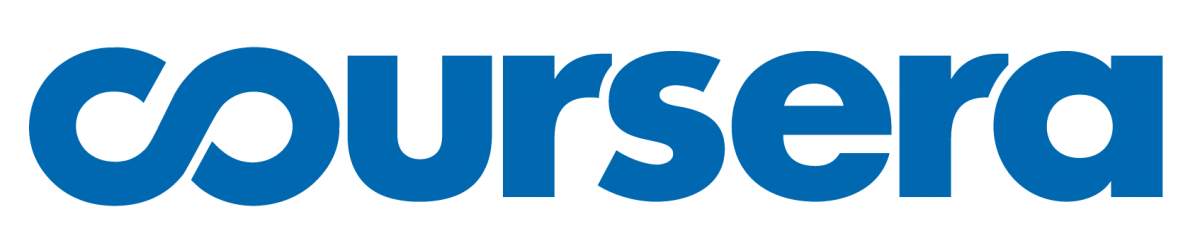
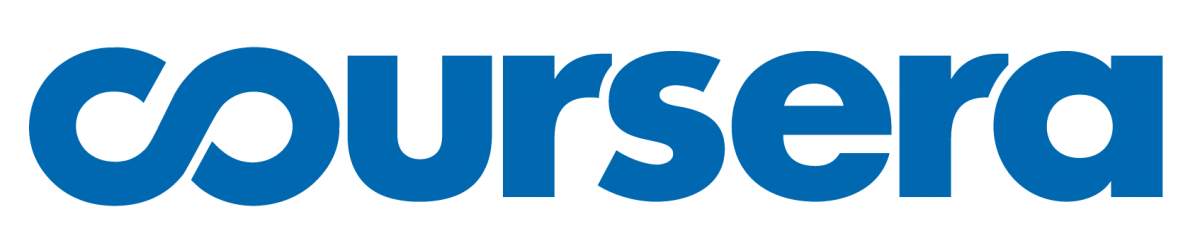
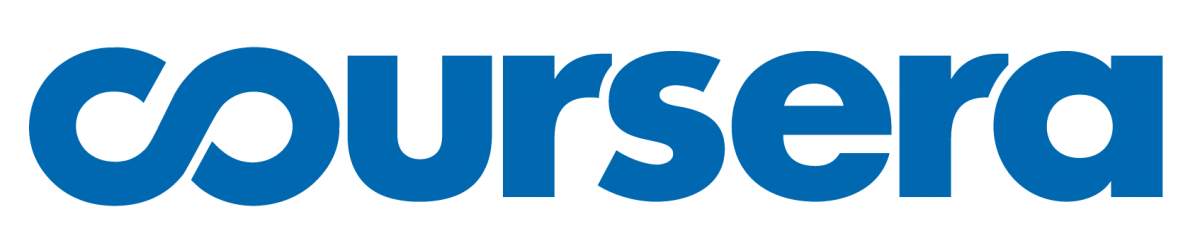
Description
An advanced-level specialization that teaches topics such as NLP, Bayesian methods, Reinforcement Learning etc. The instructors are top Kaggle competitors and CERN scientists which will help students to bridge the gap between theory and practical applications of machine learning techniques.
Teachers:
- National Research University: Alexey Zobnin
- National Research University: Mikhail Hushchyn
- National Research University: Alexey Artemov
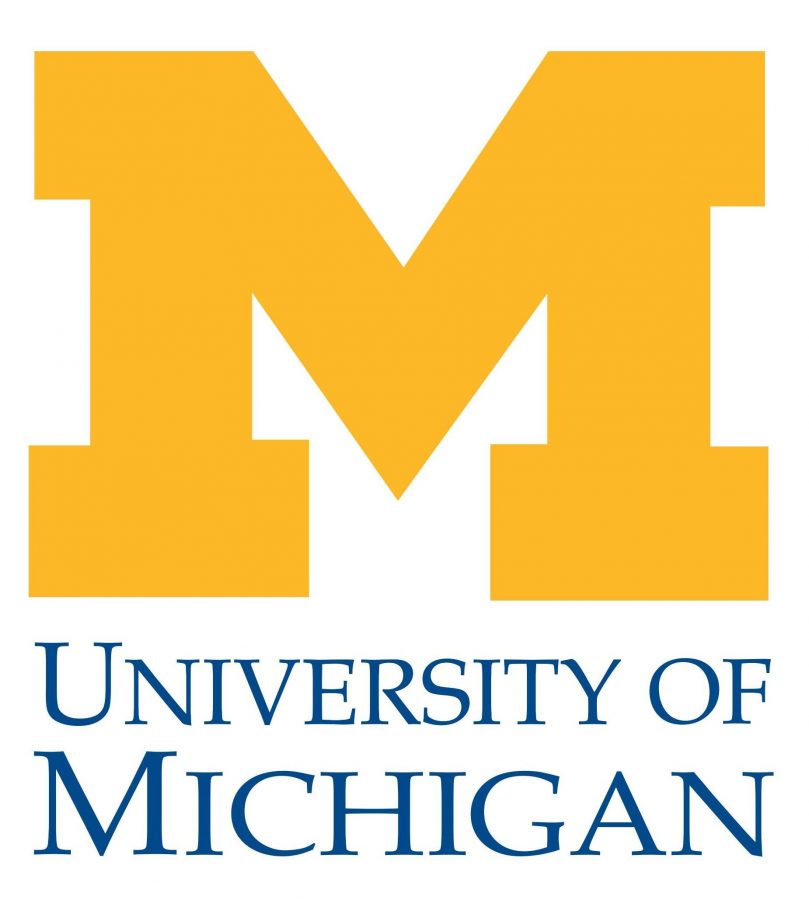
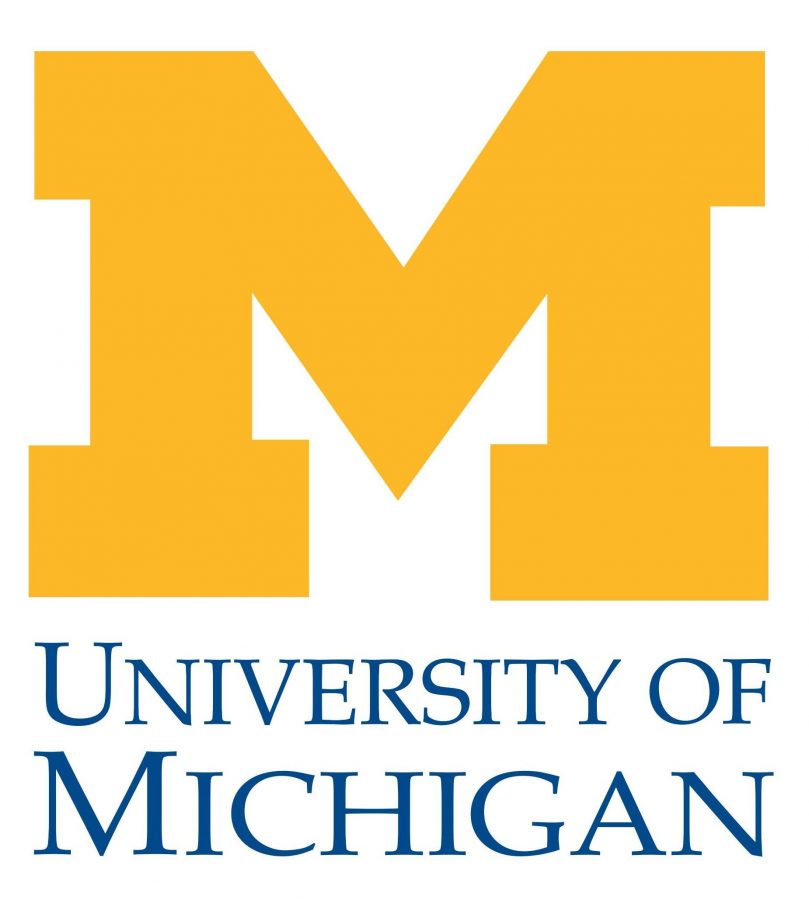
Applied Data Science with Python
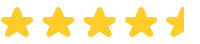
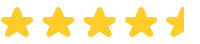
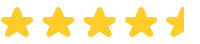
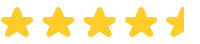
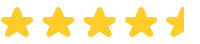
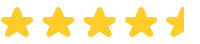
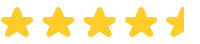
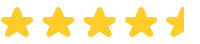
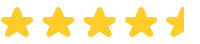
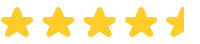
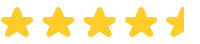
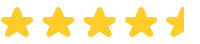
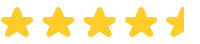
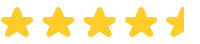
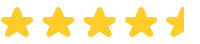
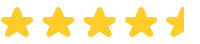
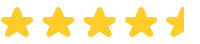
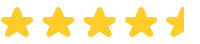
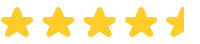
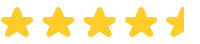
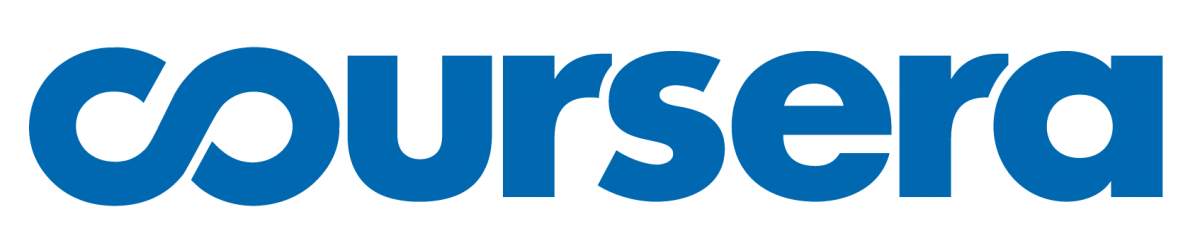
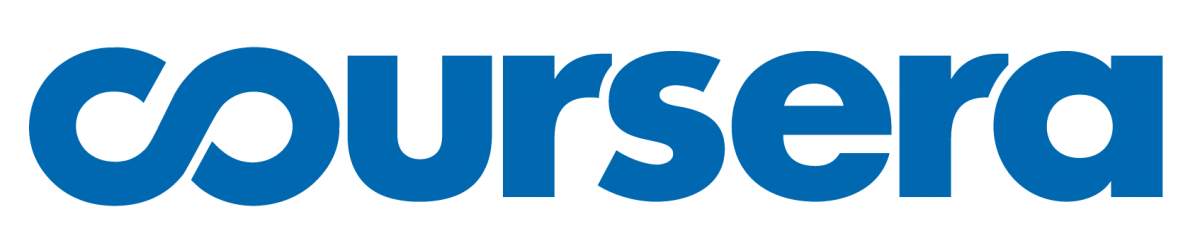
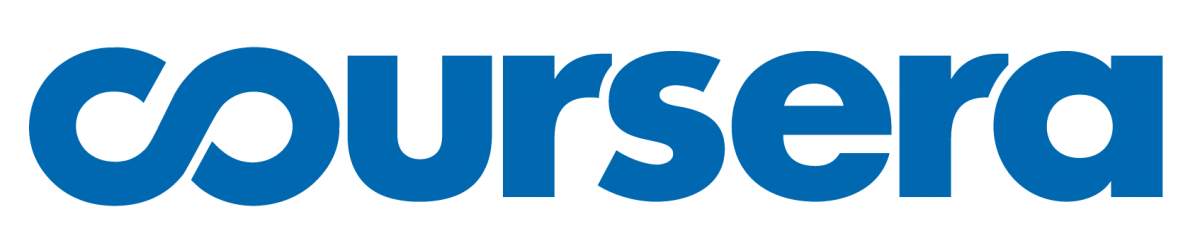
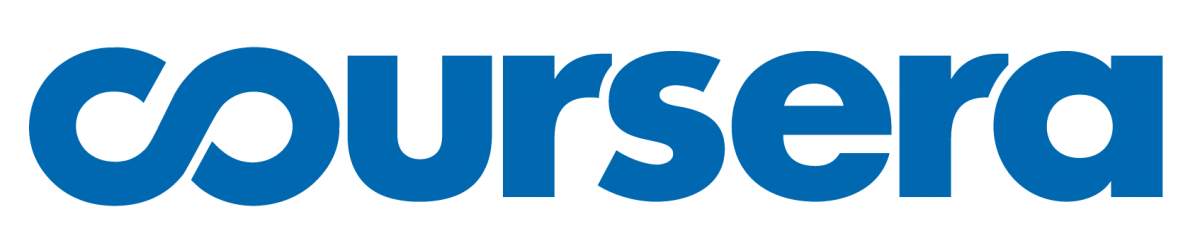
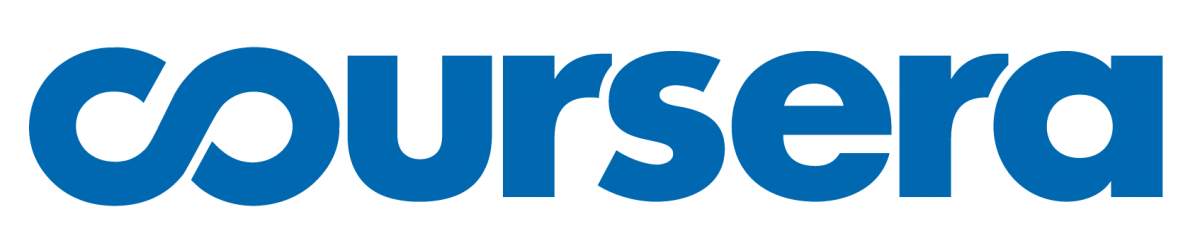
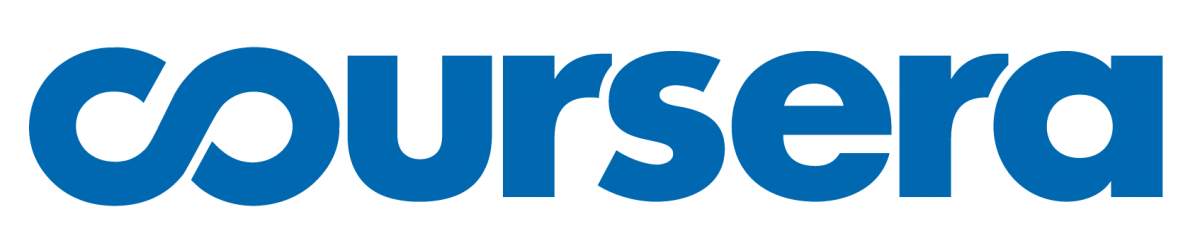
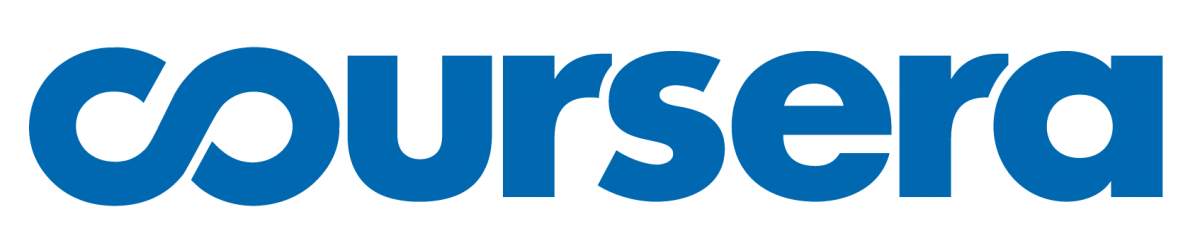
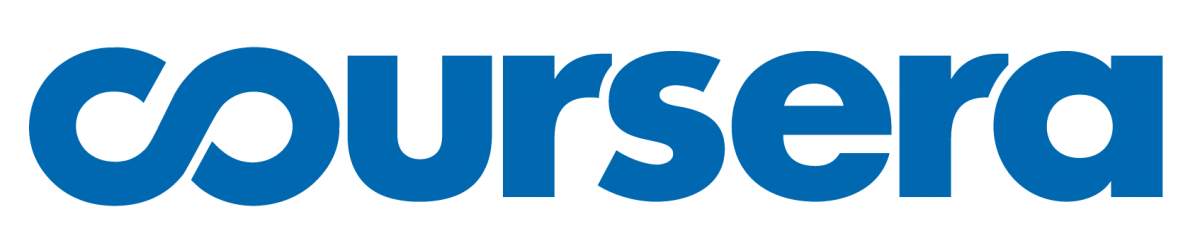
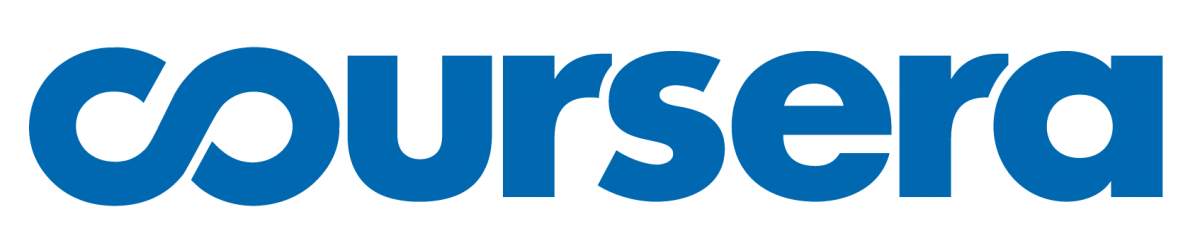
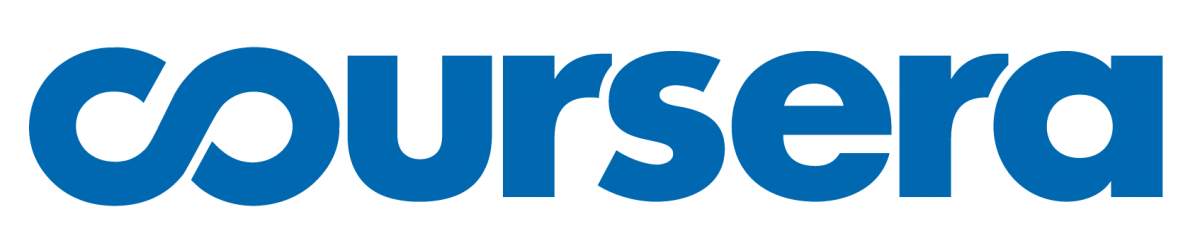
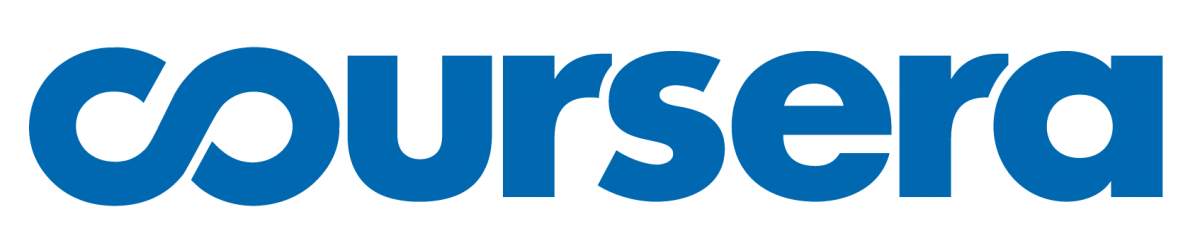
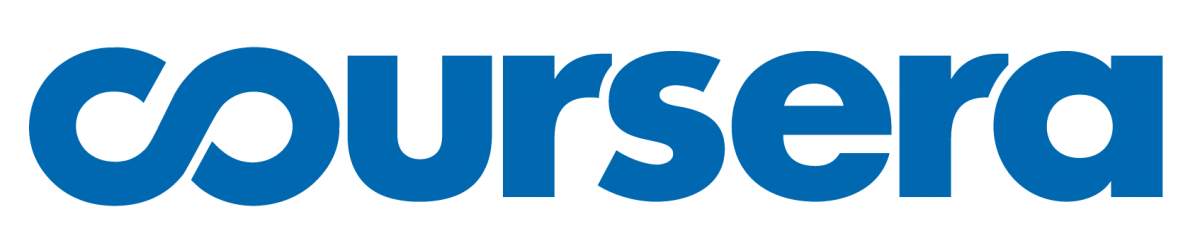
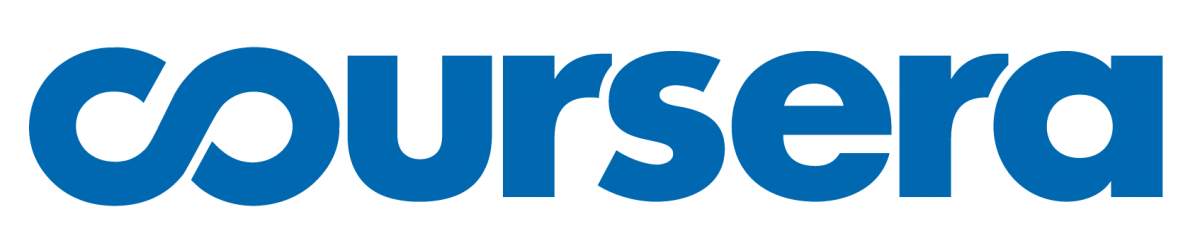
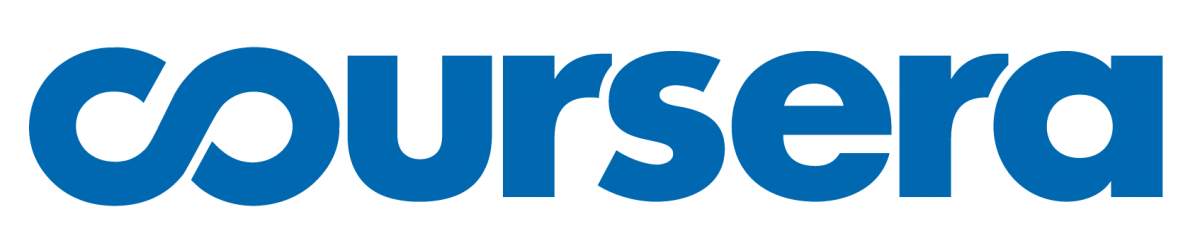
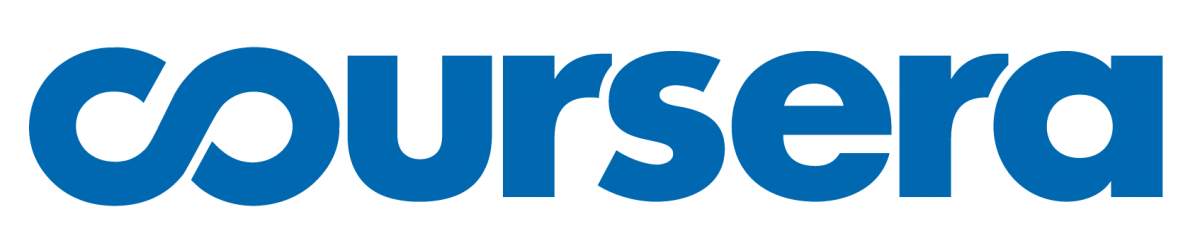
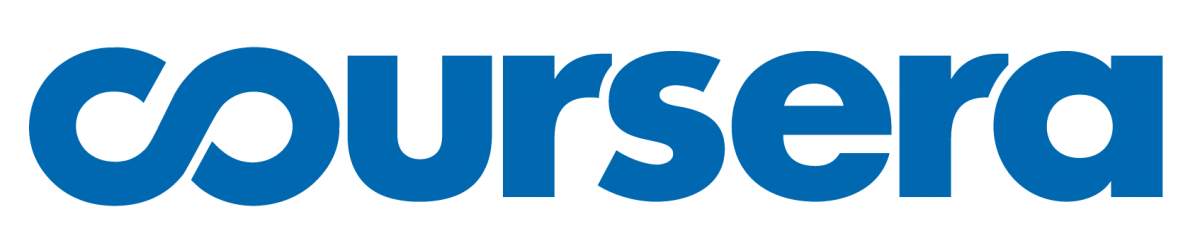
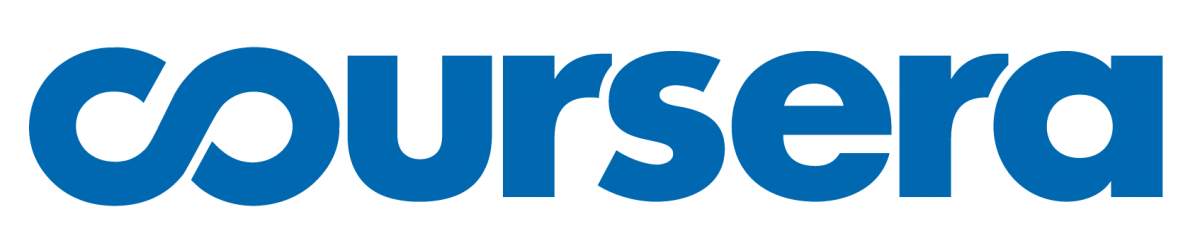
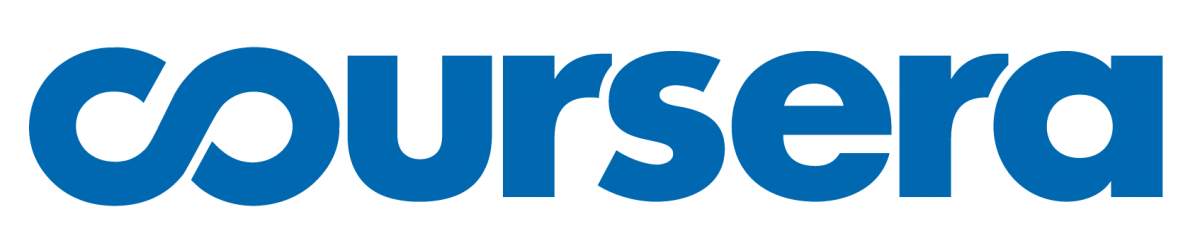
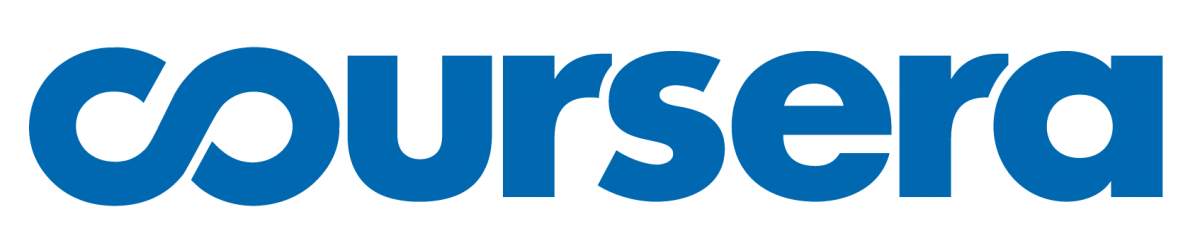
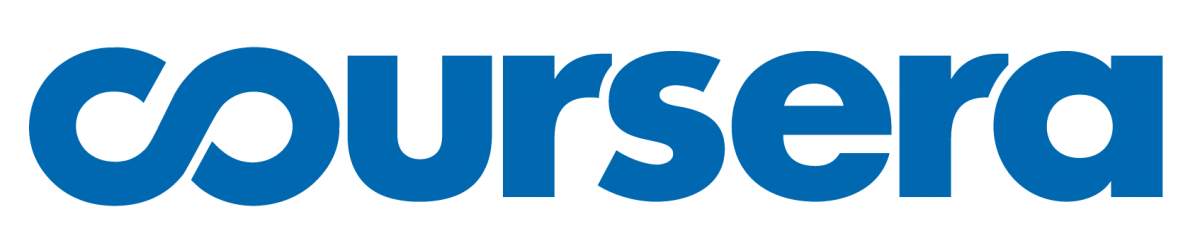
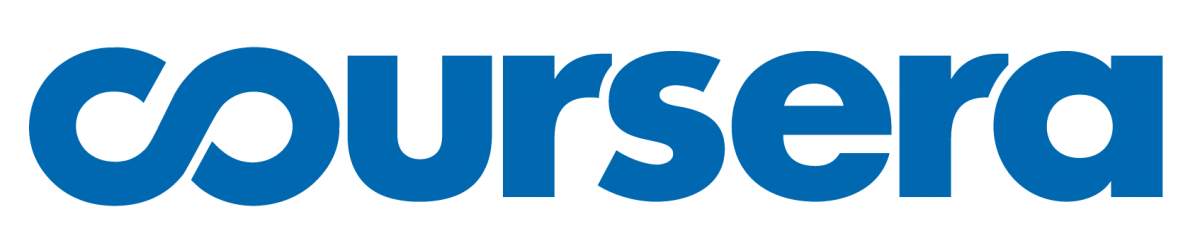
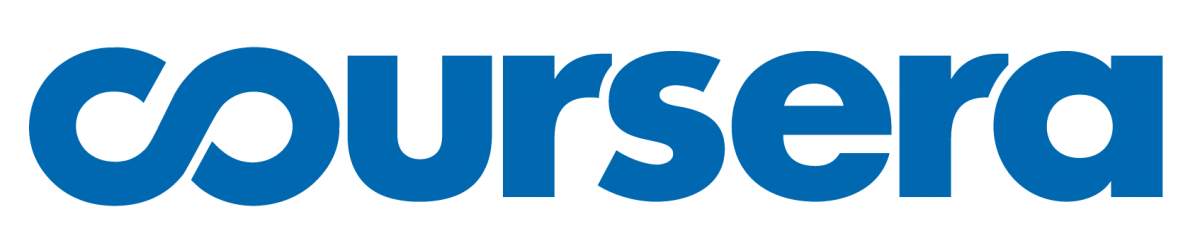
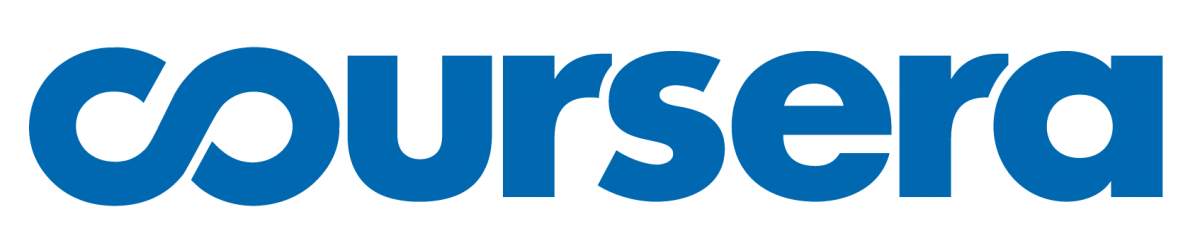
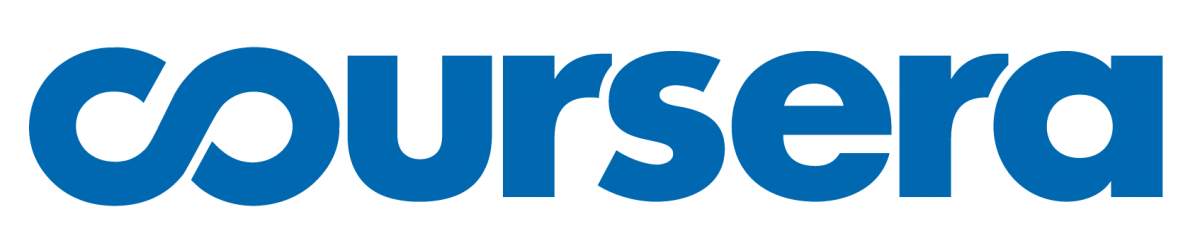
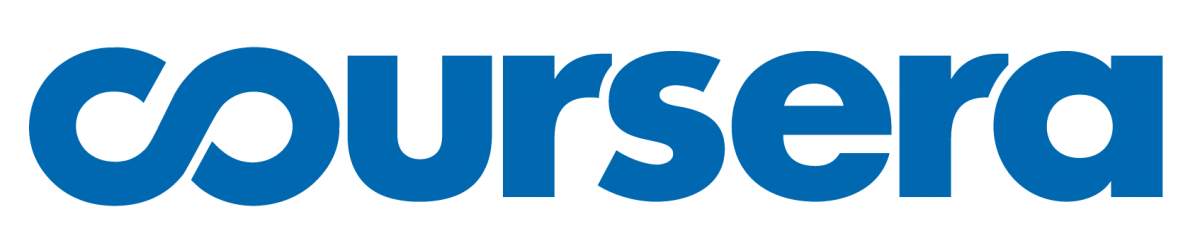
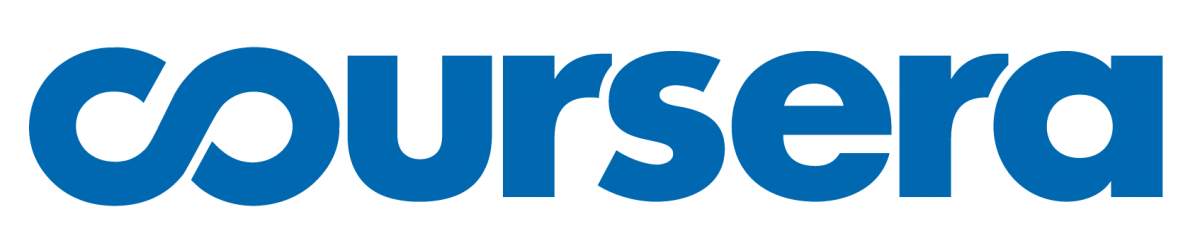
Description
This skills-based specialization is intended for learners who have a basic python or programming background, and want to apply statistical, machine learning, information visualization, text analysis, and social network analysis techniques through popular python toolkits such as pandas, matplotlib, scikit-learn, nltk, and networkx to gain insight into their data.
Teachers:
- University of Michigan: Christopher Brooks
- School of Information: Kevyn Collins-Thompson