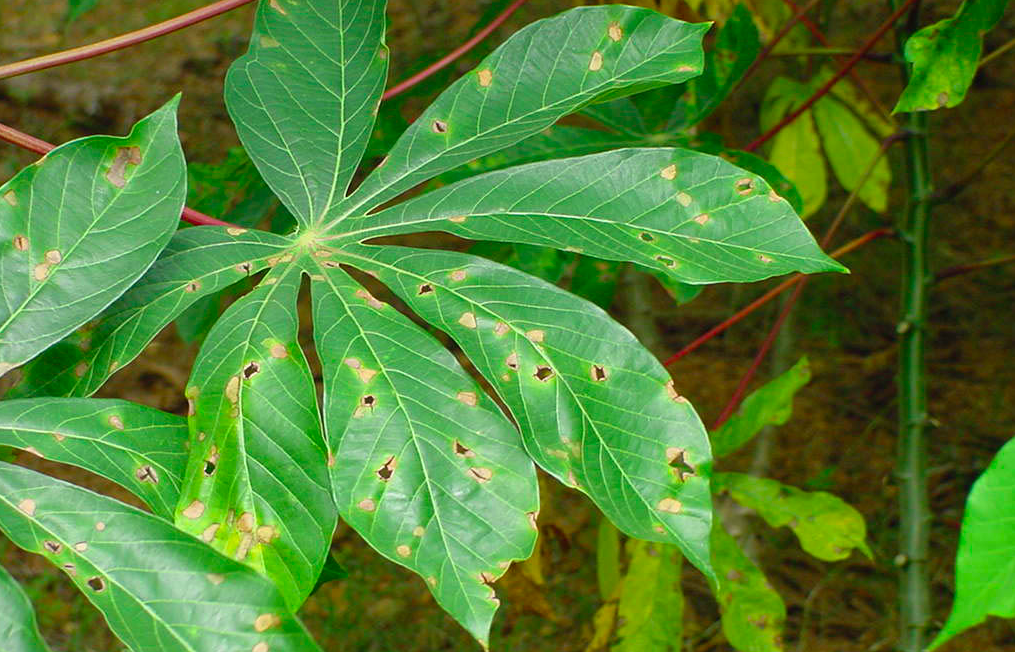
A group of researchers from Google Research and the Makerere University has released a new dataset of labeled and unlabeled cassava leaves along with a Kaggle challenge for fine-grained visual categorization.
In the past decades or so, we have witnessed the use of computer vision techniques in the agriculture field. Researchers have developed methods that assist farmers in a number of ways. One very important application of computer vision techniques in agriculture is disease recognition in crops.
However, in order to employ machine learning, large amounts of (labeled) data are needed. And in the case of disease recognition in crops’ leaves, the complexity of the problem increases as it becomes more difficult to collect annotated (or labeled) data. One particular problem that can be addressed using computer vision is the detection of viral diseases in cassava crops – one of the most commonly cultivated crops in Africa.
For this reason, the researchers collected a dataset of 9 436 labeled and 12 595 unlabeled images of cassava plant leaves. The dataset was collected within a crowdsourcing project by the Artificial Intelligence Lab in the Makerere University. They annotated the images using 5 classes: healthy plant leaves, and 4 types of diseased plant leaves. All the annotations were done manually by experts who scored each image not only with the disease but also the level of severity.
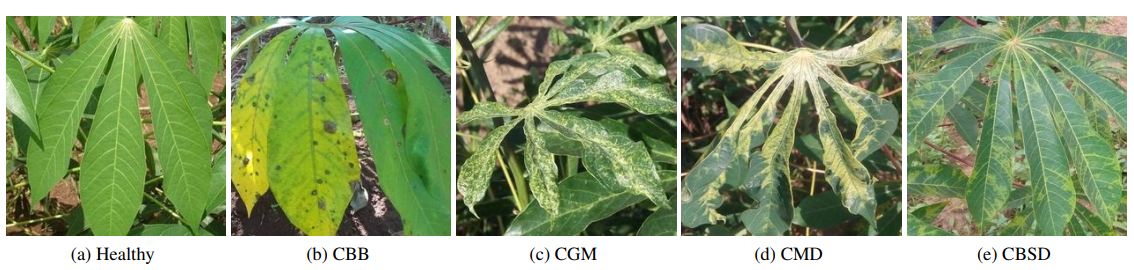
According to the researchers, the dataset is varied and it should be a challenge for machine learning algorithms due to several reasons. Some of them include different image backgrounds, different time, multiple diseases and poor image quality.
Earlier this year, researchers opened a Kaggle challenge for disease classification in images of cassava leaves. The top entries in the challenge achieved around 93% of classification accuracy using ResNet as a base model.