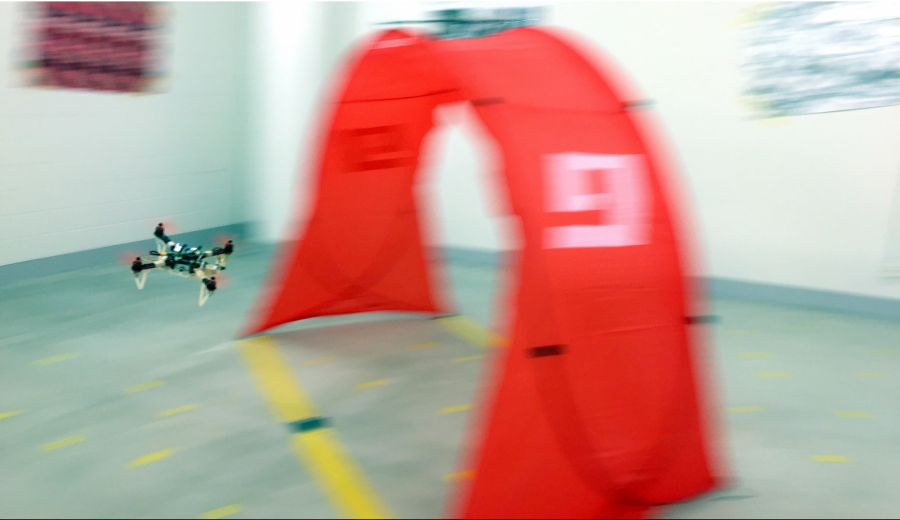
Researchers from the University of Zurich, ETH Zurich and Intel propose a vision-based method for drone racing using a path-planning system and a convolutional neural network.
Arguing that robot (and/or drone) navigation in complex and dynamic environments represents a fundamental challenge, they combine the perceptual capabilities of CNNs with state-of-the-art path-planning methods to tackle the problem.
In their proposed approach, the convolutional neural network directly maps raw image data to waypoints and speed values. The path planner takes those values and tries to generate a minimum-jerk trajectory. As part of the modular drone-racing system, the drone controller receives the generated trajectory and moves the drone accordingly.
Researchers mention that a big advantage of their modular design that divides perception and control is that it allows training of perception exclusively in simulation and testing the system in real-life without the control part changed. They show that simulation provides enough and diverse training data that makes the method more robust.
The experiments conducted showed that the method outperforms current state-of-the-art drone control systems in both simulated and real-world driving.
Researchers released a video showing the performance of the proposed method. The paper was published and is available on arxiv.