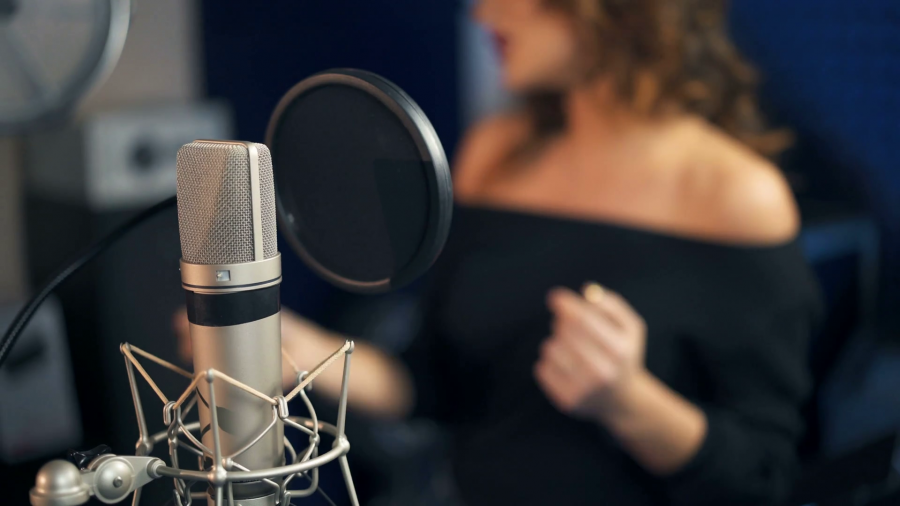
Researchers from FAIR (Facebook AI Research) and Tel Aviv University presented an AI system that can convert one singer’s voice into another. In a pre-print paper published on arxiv, Eliya Nachmani and Lior Wolf propose a deep learning method for singing voice conversion.
The method is based on representation learning and the idea is to learn a singer-agostic representation of the singers’ voice. The system learns to perform this task in a completely unsupervised manner, meaning that it does not need any labeled or classified data.
The proposed innovative training scheme and method allows learning a conversion between singers’ voice by encoding their voice in a latent space. The architecture consists of a single CNN encoder for all singers, a single WaveNet decoder, and a classifier that makes the learned latent representation singer-agnostic. Each of the singers is represented with one vector embedding and the decoder learns a conditional distribution using the singers’ vector embeddings.
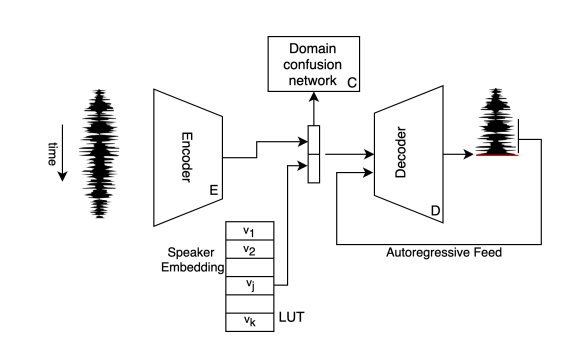
According to the researchers, the whole method is based on the idea of WaveNet (an autoencoder network designed for generating raw audio). The encoder network uses dilated convolutions to encode the signal to a latent representation that is used by the decoder together with the singer’s embeddings. The decoder network, on the other hand, is an autoregressive model which during training is fed the target output from the previous time-step.
The evaluations of the method show that it is able to produce high-quality audio, where the voice is recognizable by humans as the target singer’s voice. For the evaluation, researchers used both automatic metrics as well as user-study based metrics. They show that the proposed model was able to learn to convert audio between singers from just 5-30 minutes of their singing voices.
This work presents the first public implementation of a voice conversion system. Researchers released samples from their method and they are accessible here. More about the method and the evaluation can be read in the pre-print paper.