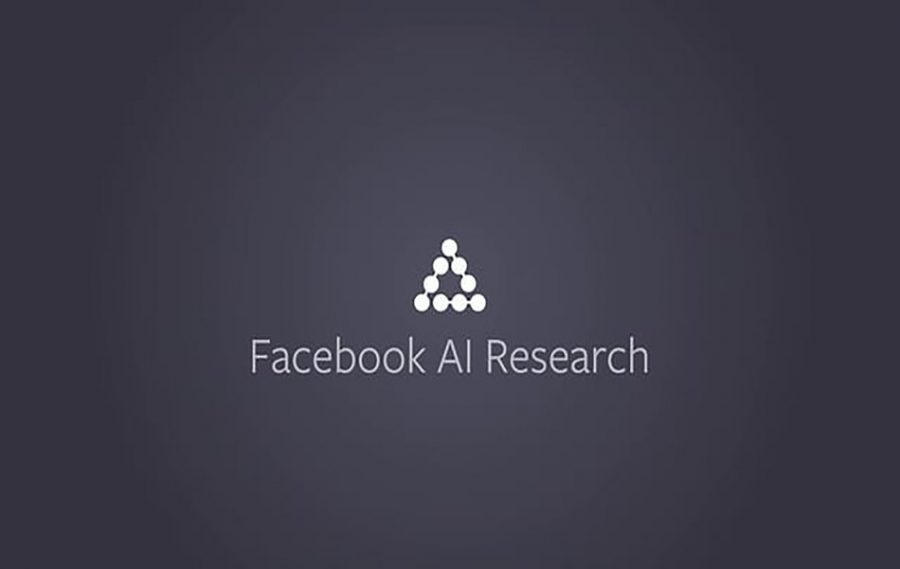
Facebook AI has announced the release of Polygames – a new open-source environment for training AI agents to master strategy games through self-play.
The goal of Polygames is to provide a common ground for development and benchmarking of zero-learning (ZL) techniques that do not require training data. Researchers and engineers from Facebook AI Research designed the framework to work with more kinds of games including popular games such as Hex, Havannah, Minishogi, Minesweeper, Othello, etc. It is meant to be extendible, therefore users can implement their own games using the provided single-file API. The framework can also be used to develop and evaluate transfer learning methods by training and applying models across different games.
Polygames has a flexible architecture and it supports a much wider range of games as compared to other frameworks. It also implements neuroplasticity meaning models are incremental and can grow as they are trained. Researchers from FAIR mention that they have already demonstrated the effectiveness of the environment as a training tool with strong model performances in different competitions.
The framework was open-sourced and it is available on Github. More details about what can Polygames do can be read in the official blog post.