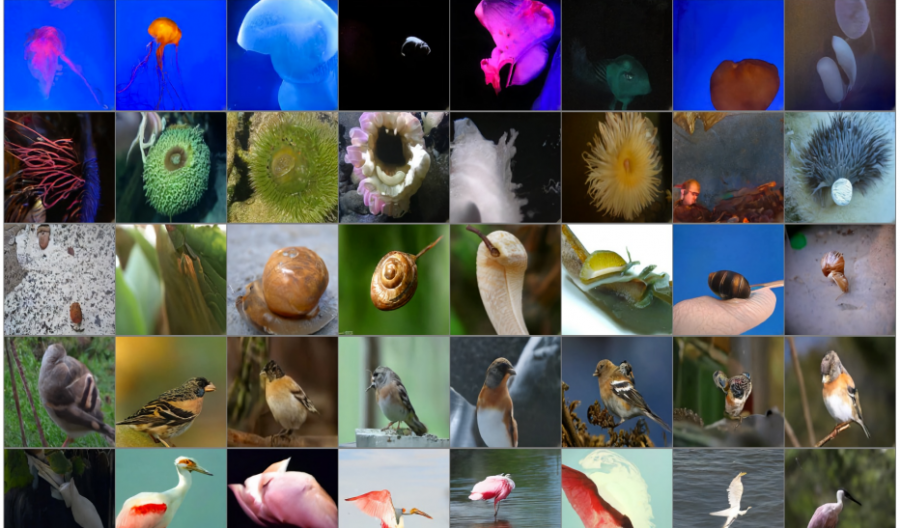
In a recent paper, researchers from DeepMind propose a variation of VQ-VAE (Vector Quantized Variational Autoencoder) model that rivals Generative Adversarial Networks in high-fidelity image generation.
The new model, called VQ-VAE-2 introduces several improvements over the original vector quantized VAE model. Researchers explored the capabilities of the new model and prove that it is able to generate realistic and coherent results.
In the past few years, Generative Adversarial Networks were considered the most powerful generative models for generating high-fidelity images. Despite having several problems such as training instability, mode collapse, etc., GANs have shown superior results compared to other generative models.
Variational Autoencoders, on the other hand, have had a major problem when trained to learn a distribution of realistic, high-fidelity images. In fact, in Variational Autoencoders the approximation of the posterior distribution is usually oversimplified and the model cannot capture the true distribution of the images. GANs were able to solve this at the expense of introducing a mode-collapse and reducing the variational capabilities of the model.
In the new paper, researchers improve Variational Autoencoders or more specifically VQ-Variational Autoencoders to be able to generate high-fidelity images comparable with those generated from GANs.
Researchers proposed two main improvements: a multi-scale hierarchical organization of the autoencoder model and learning powerful priors over the latent codes which are used for sampling.
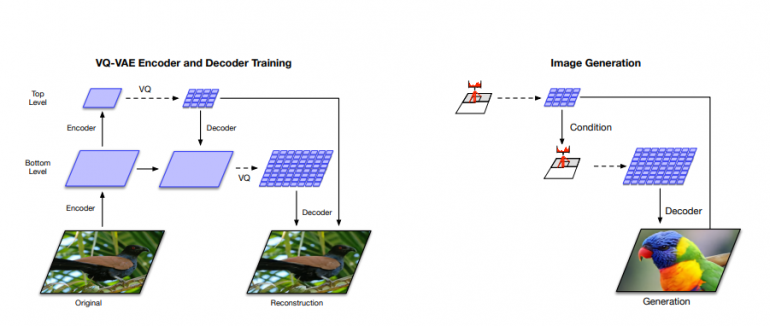
The evaluations show that the proposed method is able to generate realistic images, trained on the popular ImageNet dataset. According to researchers, the results are comparable to the ones that a GAN network can generate and the novel method does not suffer from the mode collapse issue.