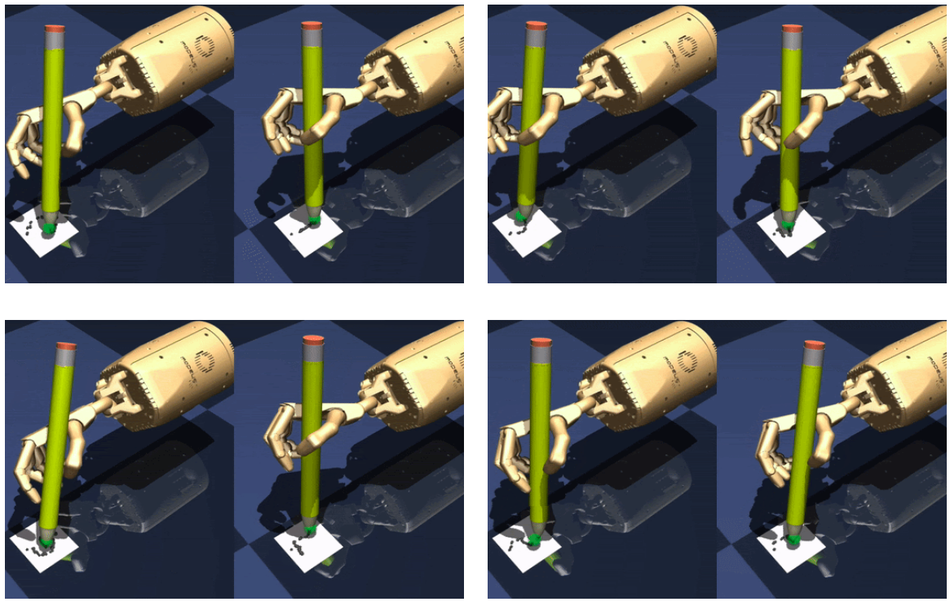
A group of researchers from Google Brain has developed a new method that allows robots to learn how to perform in-hand object manipulation. In their novel paper, researchers describe their work on Deep Dynamics Models for dextrous object manipulation.
They propose a new method called online planning with deep dynamics models (PDDM) which addresses two major challenges: the need for large amounts of data for training dynamics models and the inability to scale properly to complex and realistic tasks for object manipulation. The method is based on uncertainty-aware neural network models coupled with state-of-the-art gradient-free trajectory optimization techniques.
Researchers used both a virtual environment (a simulation) as well as a real robot to perform various experiments with the proposed deep learning method. They started with 9 degrees-of-freedom, three-fingered hand and scaled the approach all the way to 24 degrees-of-freedom, five-finger hand which can perform handwriting and manipulating free-floating objects such as balls.
In the paper, researchers argue that model-based reinforcement learning is not inferior compared to model-free RL, as it is generally known within the machine learning community. They showed that their model-based approach is able to learn and deliver control results that are impressive, using as little as 4 hours of real-world data for training.
A number of videos, showing the performance of the novel method are available on the project’s website, along with the paper and additional documentation. According to the website, the code will also be open-sourced soon.