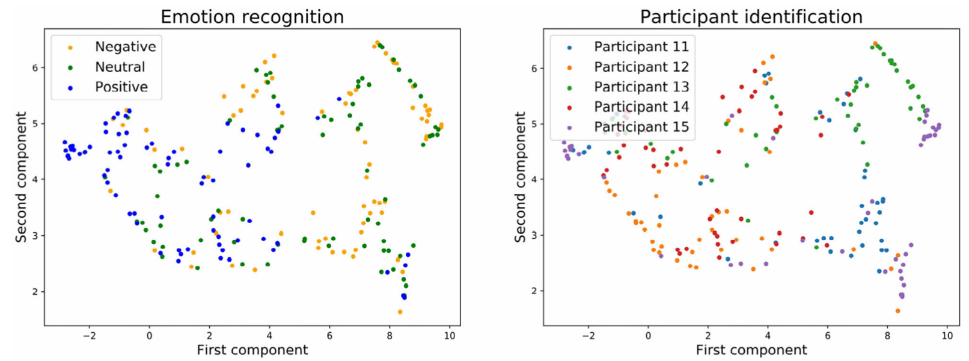
The new stratified normalization algorithm significantly increases the accuracy of emotion recognition based on the electroencephalogram (EEG) signal. Feature normalization per participant and session – the key idea of the method – allows to reduce the correlations between the recognized emotions and individual participants.
The effectiveness of machine learning models associated with emotion recognition based on EEG signals is significantly limited by the inhomogeneity of data obtained from different patients, and even data from the same patient participating in several sessions. To solve this problem, methods based on long-term and complex calibration of EEG signals from individual patients are currently used. The new study suggests an alternative approach – the stratified normalization algorithm. The accuracy of emotion recognition by neural networks trained using stratifies normalization is significantly higher compared to networks trained using batch normalization.
The new method, whose scheme is shown in Figure 1, consists in normalizing the features for individual participants and sessions. To train the model, the researchers used a dataset containing videos of 15 patients, with each patient participating in three sessions. During one experiment, 15 videos were recorded with one patient. All videos have pre-assigned participant emotion (positive, negative, or neutral).
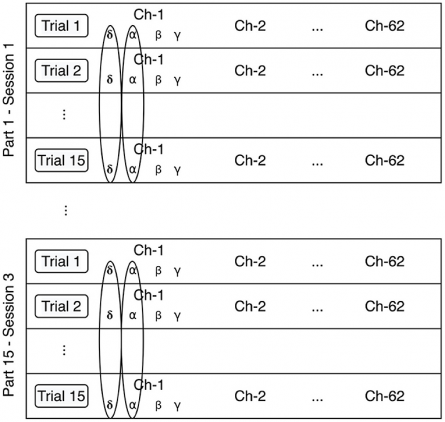
The output of the neural network without the use and with the use of stratified normalization are shown in Fig. 2 and 3, respectively. As can be seen from the figures, the new method leads to strong localization of each of the emotions in the distribution. There is also a significant decrease in the correlations between the recognized emotions and individual participants in the experiments. These results indicate the high efficiency of the method for solving various problems of emotion recognition. In addition, stratified normalization can be used for voice and object recognition.
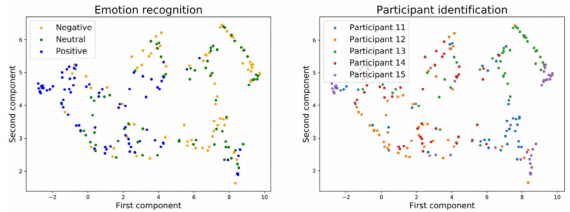
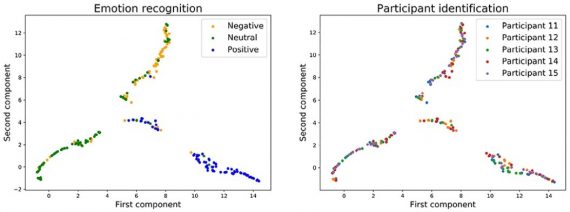
EEG, due to its low cost and noninvasiveness, is the most promising tool for detecting emotions. The stratified normalization method opens new routes towards emotion recognition, used in a vast variety of applications, including the development of brain-computer interfaces, interactive storytelling algorithms, and mood disorder diagnosis.