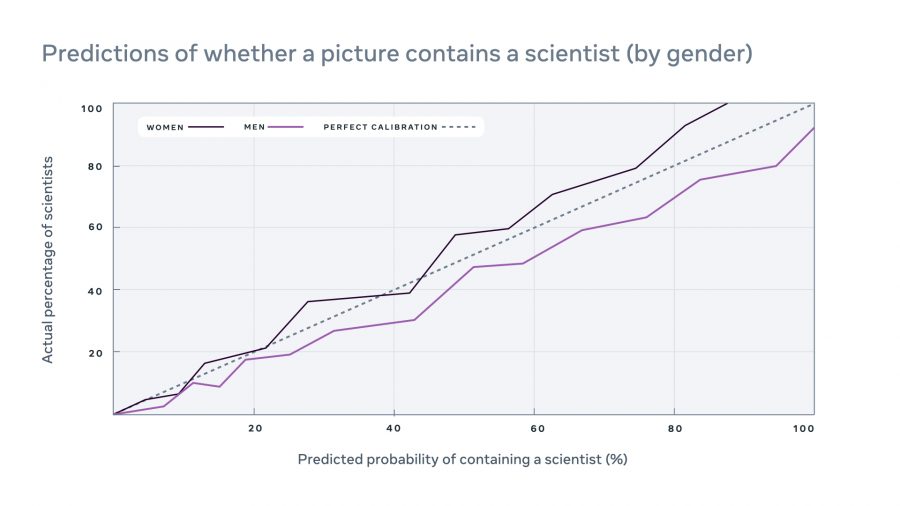
Fairness Flow is a set of diagnostic tools that helps you compare how fair models and label markers perform for specific user groups.
Why is it needed
Facebook AI specialists are convinced that cases of AI bias can lead to undesirable consequences, and even harm users of the services. As an example of model bias, the same content from different users can either be flagged as spam or not. Labelers may also rate the same content differently by different users. Fairness Flow detects cases of models and labels bias concerning user groups (by gender, age, etc.).
How it works
In assessing model fairness, Fairness Flow divides the dataset into relevant user groups and evaluates metrics for each group separately. Finally, it:
- verifies whether the model’s result is regularly overestimated or underestimated for one or more groups compared to others;
- evaluates how well the diversity of data is represented for each user group.
It is important to note that the difference in metrics does not always indicate model bias: in such cases, a deeper analysis of the problem is required.
Labels given by people may reflect their prejudices, which are not considered when assessing model fairness. In assessing labels fairness, Fairness Flow derives new metrics using a methodology based on signal detection theory. The resulting metrics are used to estimate how well labelers are doing.
Future expectations
Fairness Flow is not context-sensitive. However, at the moment, the best practices with its use have been successfully adapted for certain tasks. These tasks use specific types of models (for example, binary classifiers) and labels with reliable data available in sufficient volume. The goal of the Facebook AI team is to develop processes that will enable AI teams to systematically identify potential problems while creating products. To do this, it is necessary to identify common patterns and expand the range of problems and models for which Fairness Flow is applicable.
Great Article , Thanks for sharing keep posting