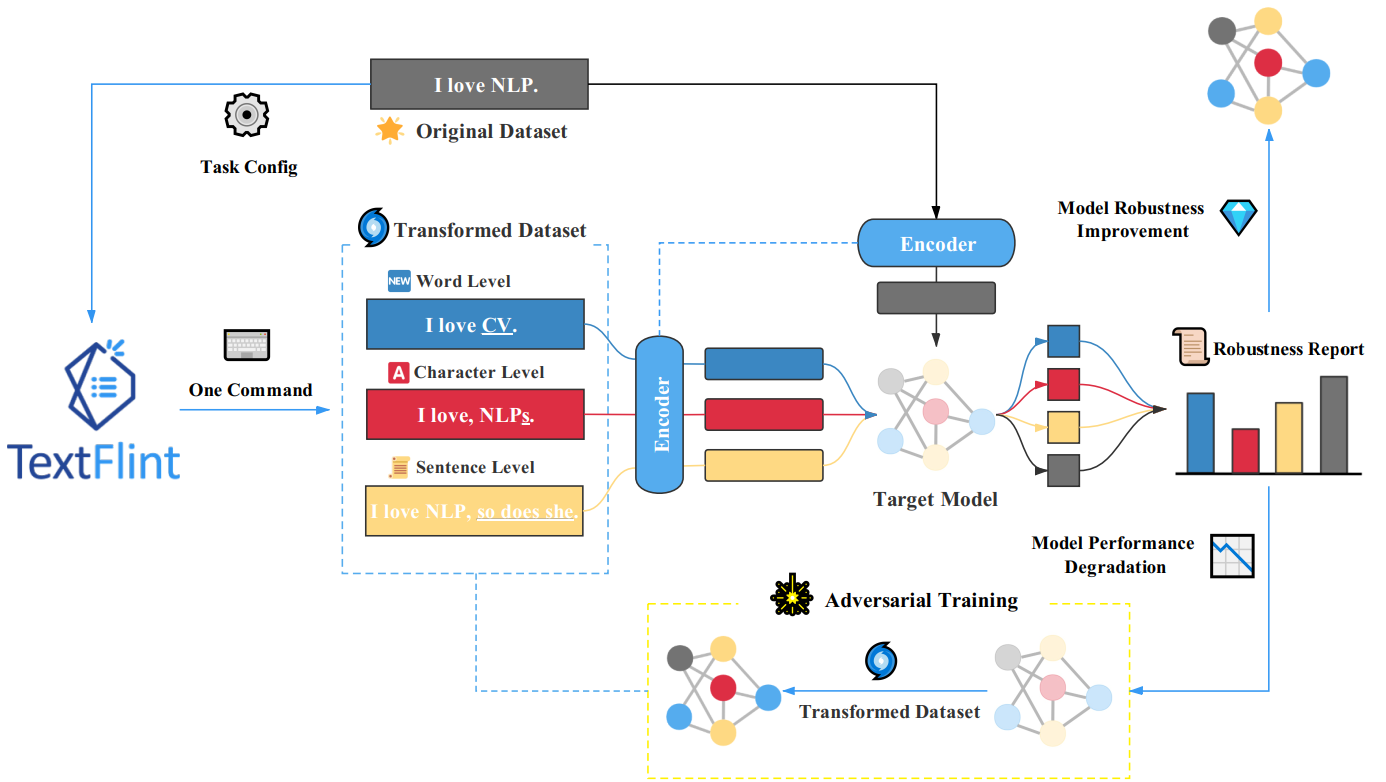
TextFlint is a multilingual, multitasking platform for analyzing NLP models stability. Open source available for English and Chinese, other languages are being developed. Included text processing tools:
- general and specific text transformations;
- subpopulations;
- converting text for adversarial attacks;
- combinations of the above.
Why is it needed
Generalization of NLP models is necessary to ensure stable performance not only on test data but also on arbitrary data. Models trained on insufficient datasets repeat data incompleteness. Furthermore, deep neural networks are vulnerable to adversarial attacks based on carefully picked examples.
To verify the advantage of TextFlint, the authors have tested over 67,000 modern deep learning models, classic supervised learning models, and performing systems. Nearly all models showed significant performance degradation. The accuracy of BERT predictions for text sentiment recognition, named entity recognition, and natural language inference has been reduced by more than 50%. The goal of the authors is to provide developers with a convenient and automated tool for creating robust NLP models.
Features of TextFlint
Full coverage of text transformations. TextFlint applies 20 general transforms, 8 subpopulations, and 60 specific transforms, as well as thousands of their combinations. Supports text transformation for adversarial attacks on a target model.
Generation of labeled augmented data. TextFlint supports the transformation of arbitrary texts with the generation of labels. There are 6903 datasets available for download, generated by transforming 24 known NLP-datasets.
Complete analytical report. TextFlint provides an analysis report on the lexis, syntax, and semantics of the target model, indicating defects. It is assessed how the result of the model is linguistically consistent and acceptable to people. Based on detected defects, TextFlint generates a large amount of data for additional training of the target model.