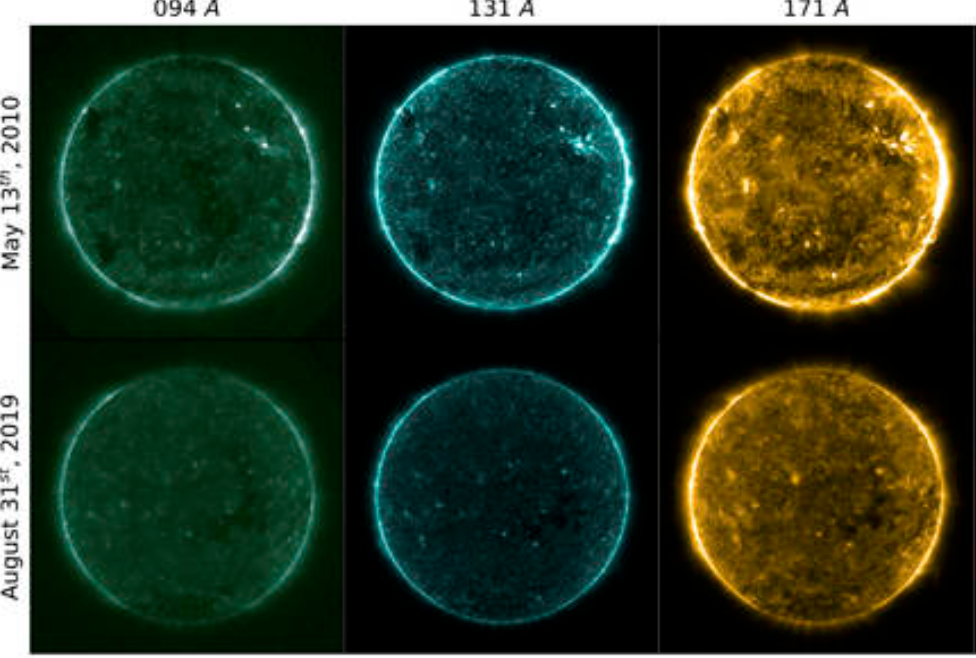
Space telescopes require frequent recalibration due to their degradation associated with intense radiation exposure and particle bombardment. The NASA neural network allows us to solve this problem remotely, opening up the possibility of processing images from telescopes remote from the Earth, direct calibration of which is impossible.
Previously, NASA used meteorological rockets to calibrate telescopes-small rockets that are in space for only about 15 minutes. These rockets are needed in order to register radiation at wavelengths absorbed by the atmosphere and inaccessible to observation from Earth. By comparing images from meteorological rockets and telescopes, it is possible to determine the level of degradation of the latter. However, rocket flights do not occur often enough, they are complex, and the viewing angle in the images is fixed. In addition, such a calibration method is not possible when telescopes are located in deep space.
The NASA neural network is trained to recognize certain events-solar flares-in images from telescopes. The training dataset consisted of images obtained from meteorological rockets. After training, the model began to identify solar flares regardless of the degree of deterioration in the quality of the telescope lens and determine the calibration parameters required for accurate image restoration. An important result obtained at NASA is the ability of the neural network to recognize events at several wavelengths. The effectiveness of the model was sufficient to be used as an alternative to meteorological rockets.