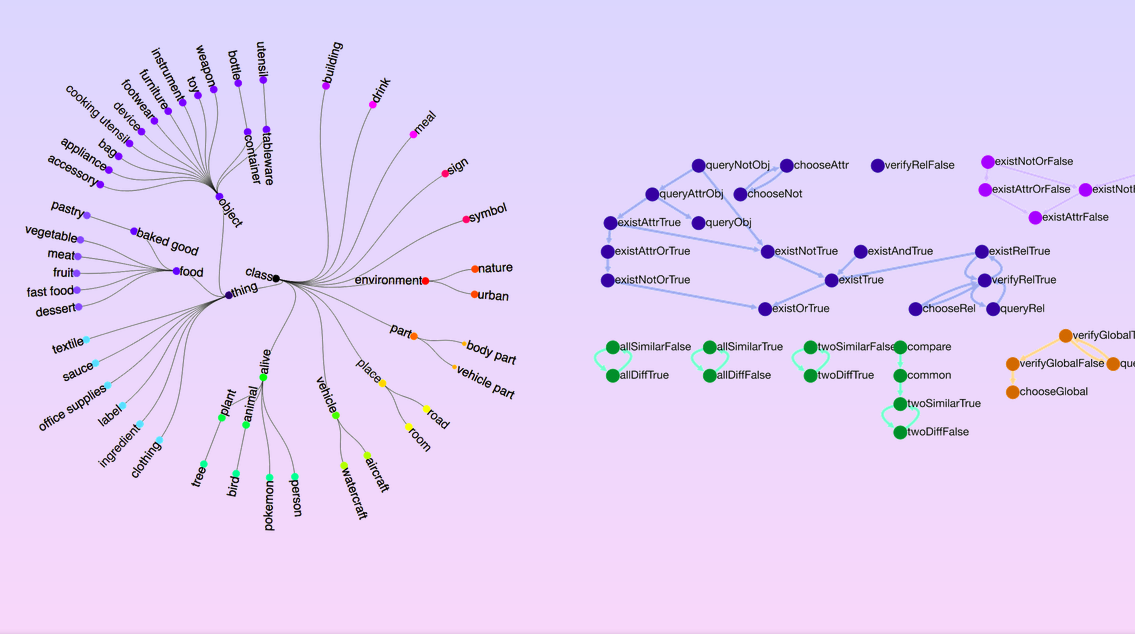
Researchers from Stanford University have released a new large-scale dataset for compositional question answering called CQA.
The new dataset, created by Drew Hudson and Christopher Manning, consists of 22 million questions about various day-to-day images. Questions emerge from natural relations between concepts in 113K of images covered in this work.
Each question in the dataset is associated with a structured representation of its semantics and a functional program that gives the reasoning steps necessary to answer the questions.
On the other hand, each of the images is associated with a scene graph of the objects present in the image. This setting provided researchers with a strong and robust question engine that allows extracting a large number of natural reasoning questions.
The dataset construction is a five-stage pipeline that includes: Graph normalization (ontology construction), question generation, sampling and distribution balancing, entailment relations (functional programs) and metrics (validation and plausibility). This pipeline generates a standard natural language form for each question together with and a functional program representing its semantics.
Additionally to the dataset, researchers contribute with a new set of evaluation metrics that express qualities such as consistency, grounding, and plausibility of natural questions.
Researchers report that LSTM obtains mere 42.1%, strong VQA models achieve 54.1% while human performance is as high as 89.3%. This indicates that the dataset will challenge researchers to further push the capabilities of language models. The dataset is available at visualreasoning.net.