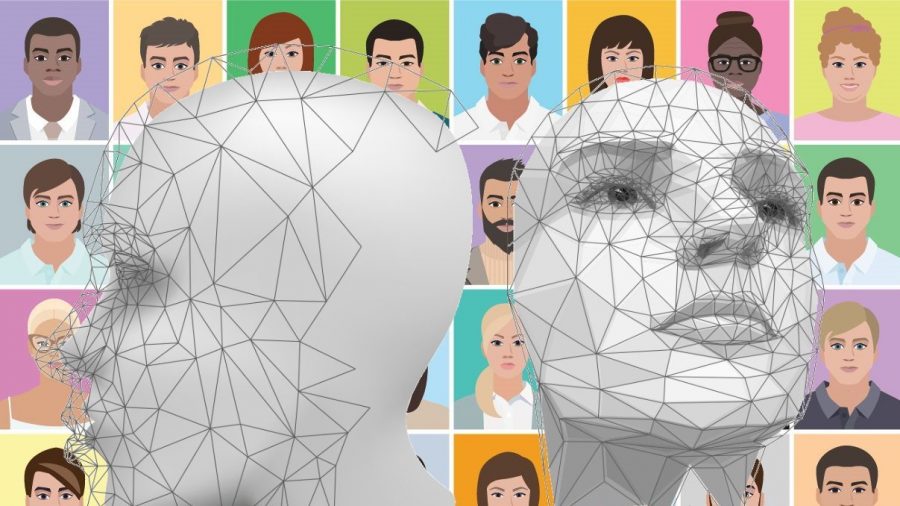
IBM Research has released a new large dataset called Diversity in Faces (DiF) to advance the study of fairness and accuracy in facial recognition technology.
Researchers from IBM Research used publicly available images from the YFCC-100M Creative Commons data set and annotated the faces to create a diverse and balanced dataset. Their goal was to build a dataset where the distribution of features in faces will be uniform and the data will offer coverage and accuracy.
To do so, they annotated the faces using 10 well-established and independent coding schemes including craniofacial (e.g., head length, nose length, forehead height), facial ratios (symmetry), visual attributes (age, gender), and pose and resolution. Previously, in facial recognition research, people were focusing on more “subjective” annotations such as age, gender, and skin-tone. The novel approach that IBM researchers took is based on relevant scientific literature that helped identify different coding schemes.
In the paper, researchers describe the process of generation of the DiF dataset annotations. They also provide a statistical analysis of 1 million of the total 100 million instances in the dataset.
According to them their approach and findings from this project will “further the community’s understanding” about the characterization of human faces and will enable IBM to find new methods for improving its facial recognition technology.
The dataset was open-sourced and made available to the global research community working in the area of facial recognition. Access to the dataset can be requested on the official page.