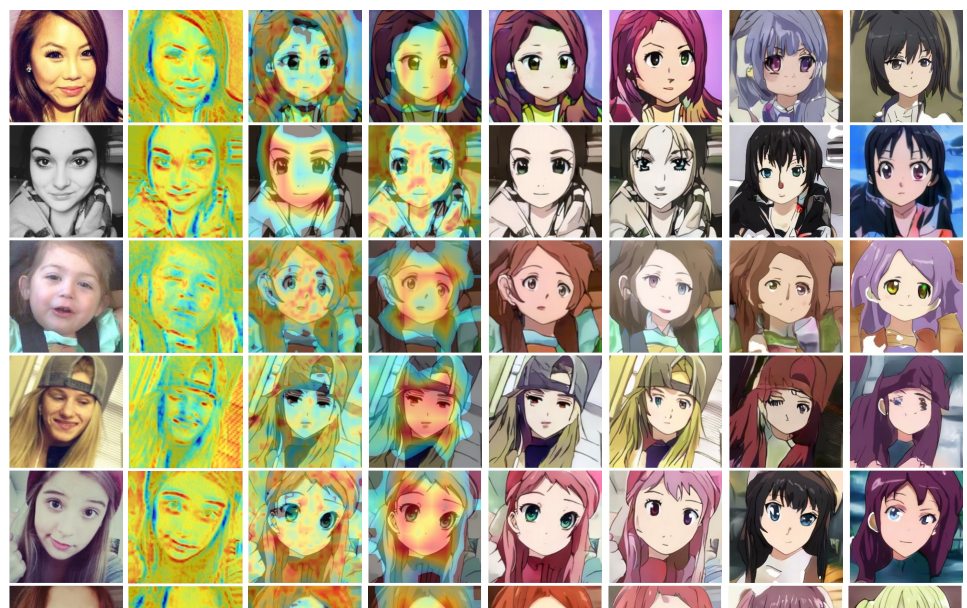
A group of researchers from NCSOFT and Boeing Korea Engineering and Technology Center has proposed a novel state-of-the-art method for image-to-image translation.
By introducing several novelties, researchers managed to build a generative model which outperforms current state-of-the-art models in the task of image to image translation. Their model, named U-GAT-IT is based on the paradigm of Generative Adversarial Networks (GANs) and learns to perform the task in a completely unsupervised manner.
The problem that “image-to-image” translation is solving is the transformation of images from one domain so they have the style (or characteristics) of images from another domain. In this work, researchers tried to tackle this problem by learning a mapping between the two domains in an unsupervised fashion and leveraging the power of generative adversarial networks.
Within their framework, the neural network model is trying to learn a cross-domain transformation by only taking unpaired samples from each of the domains. Researchers designed an architecture comprising of two generators and two discriminators. They also proposed a new attention module and a new normalization function called AdaLIN. According to them, the attention module is guiding the model to focus on important regions in the image which contain distinguishing features between the source and the target domain.
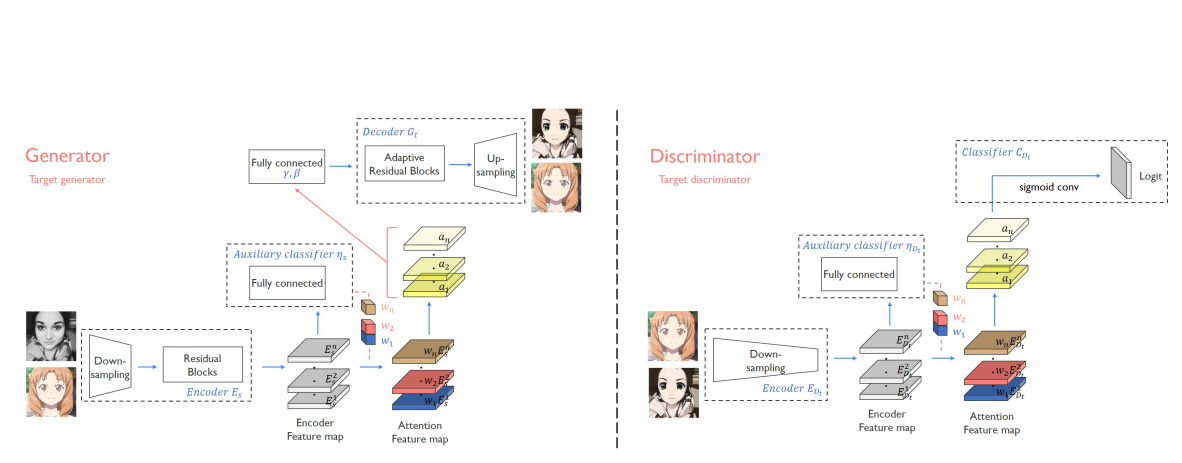
The experiments and evaluations showed that the method is able to successfully learn how to perform image-to-image translation. Also, the results show that the method is superior to existing state-of-the-art GAN models for unsupervised image-to-image translation.