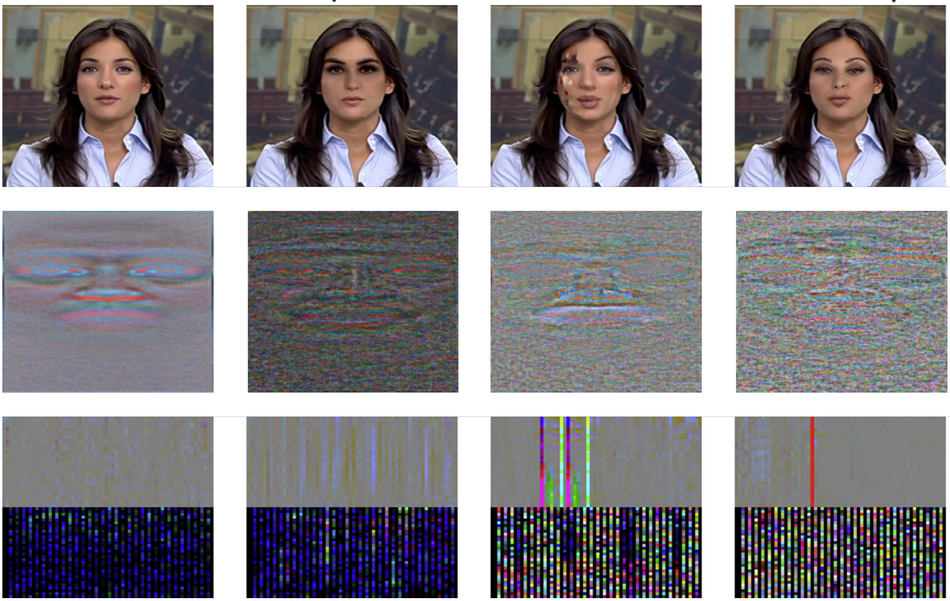
Deepfakes have been a popular topic in the past few years. The fake portrait videos generated using generative models have been used widely for many different use cases from celebrity imitation to Instagram filters and political propaganda. As a consequence, research in this direction has been growing over the years.
In a novel paper, researchers from Binghampton University and Intel Corporation have analyzed the “heartbeat” of deep fakes. They tried to design a model that can detect the deepfake video source meaning the model that was used to generate it.
Researchers analyzed the residuals from a generator model from a GAN (Generative Adversarial Network) and tried to make a connection with biological signals. They proposed a deepfake detection framework under which they can classify a deepfake video into the class corresponding to a generative model that potentially generated this sample.
The proposed framework starts by having several generator networks to which a single input real video is fed. The pair of the realistic video and the many generated (deepfake) videos are passed to a so-called registration module. Here the system extracts facial regions of interest (ROIs) and from those, it extracts biological signals in order to create PPG cells. This representation is in fact intended to provide residuals projected in the spatial and frequency domains. The last module is the classification module which is responsible for determining both: if a video is deepfake or not and in the case of deepfake it should predict the most plausible generative model.
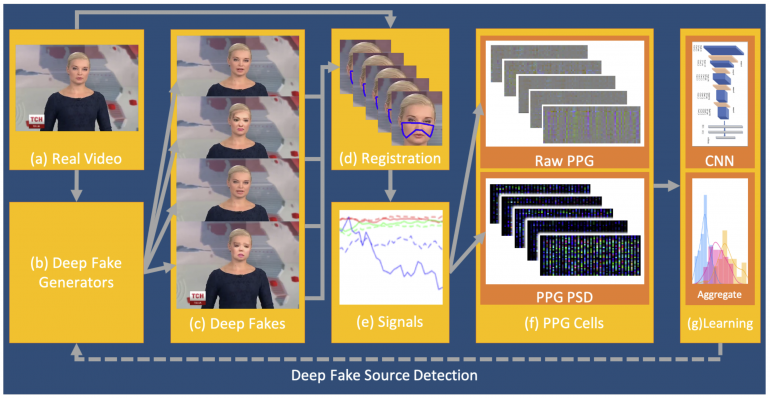
Researchers used several publicly available deepfake datasets which they categorized into single-source and multiple-source datasets depending on how many generative models were used. Results showed that the proposed framework is able to guess deepfakes vs. real-videos with an accuracy of 97.3% and it is able to guess the generative model out of 5 models with an accuracy of 81.9%.
More details about the proposed deepfake source detection framework can be read in the paper.