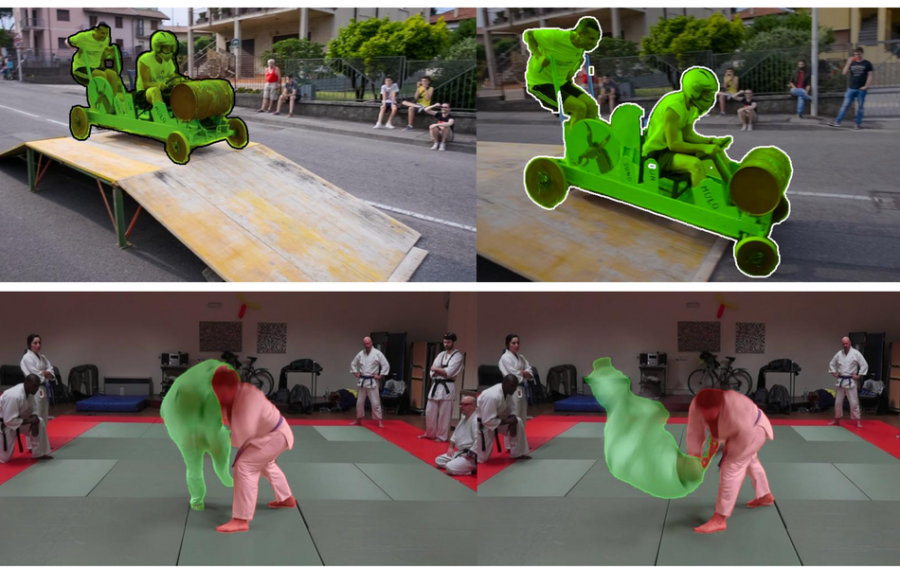
Researchers from the Inception Institute of Artificial Intelligence, UAE and Indiana University have proposed a novel state-of-the-art method for video object segmentation based on graph neural networks.
In their work, they propose to frame the problem of video object segmentation as a process of iterative information fusion over video graphs. The new method called AGNN or attentive graph neural network constructs a so-called video graph where frames are represented as nodes and relations between them as edges.
The relations between a pair of frames are actually described by an attention mechanism. According to researchers, the correlation between frames can be efficiently captured by employing attention and therefore the time-consuming optical flow estimation can be avoided. The method works by using recursive message passing that allows information to be propagated in the video graph. It also preserves the spatial information making the AGNN significantly different than previous graph neural networks.
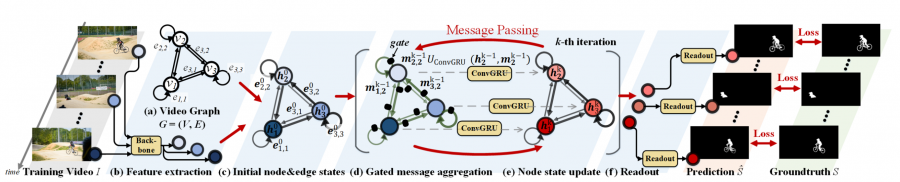
Researchers evaluated the proposed method on three popular benchmark datasets: DAVIS16, Youtube Objects, and DAVIS17. They showed that the method is superior over current state-of-the-art methods. Additionally, the generalization capabilities of AGNN were demonstrated by extending the method to another task – Image Object Co-segmentation (IOCS).
More about the novel method can be read in the paper. The implementation was open-sourced and it is available on Github.