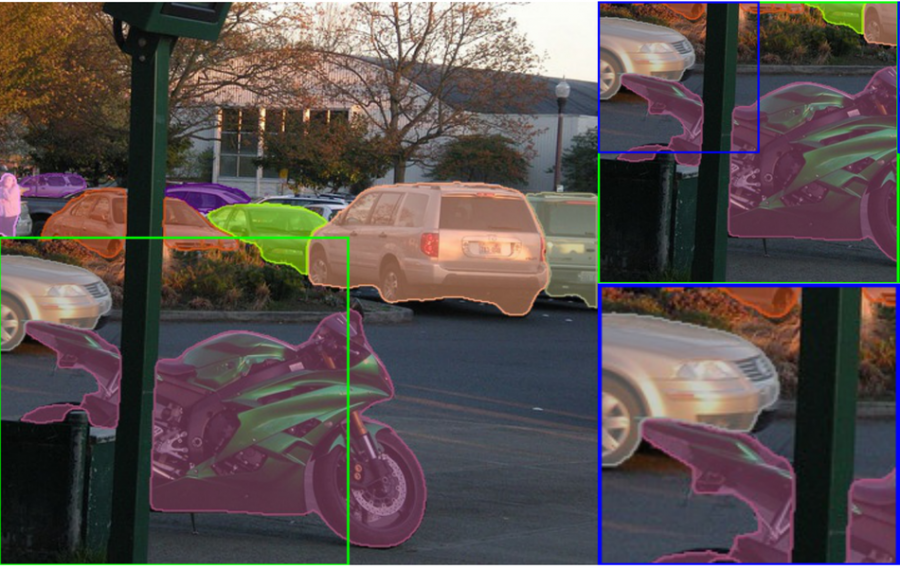
Researchers from the University of Adelaide in Australia have proposed a novel state-of-the-art method for instance segmentation.
In their latest paper, “Conditional Convolutions for Instance Segmentation”, they propose a new perspective for solving the problem of instance segmentation where the goal is to identify and segment every unique object present in an image. According to them, the new approach allows for improved performance in both accuracy and inference speed compared to previous approaches.
The main differences compared to current well-performing instance segmentation methods such as Mask R-CNN is that the proposed method does not need ROI (region-of-interest) cropping and feature alignment, as the task of segmenting instances is solved by a fully convolutional network. This comes as a consequence of the employment of conditional convolutions in the proposed architecture. Researchers mention that due to the improved capacity of these convolutions, the size of the mask head can be decreased significantly, leading to large performance gains. The complete architecture of the method can be seen in the image below.
The proposed method was evaluated on the popular, large-scale benchmark MS-COCO. Researchers report that CondInst outperforms current state-of-the-art methods on the COCO dataset such as Mask R-CNN, TensorMask, YOLACT, etc. The method outperforms the best existing method which is Mask R-CNN by 0.8%, and according to researchers is the first simpler method to outperform Mask R-CNN in both accuracy and speed.
The implementation of the method was open-sourced and can be found here. The pre-print paper can be found on arxiv.