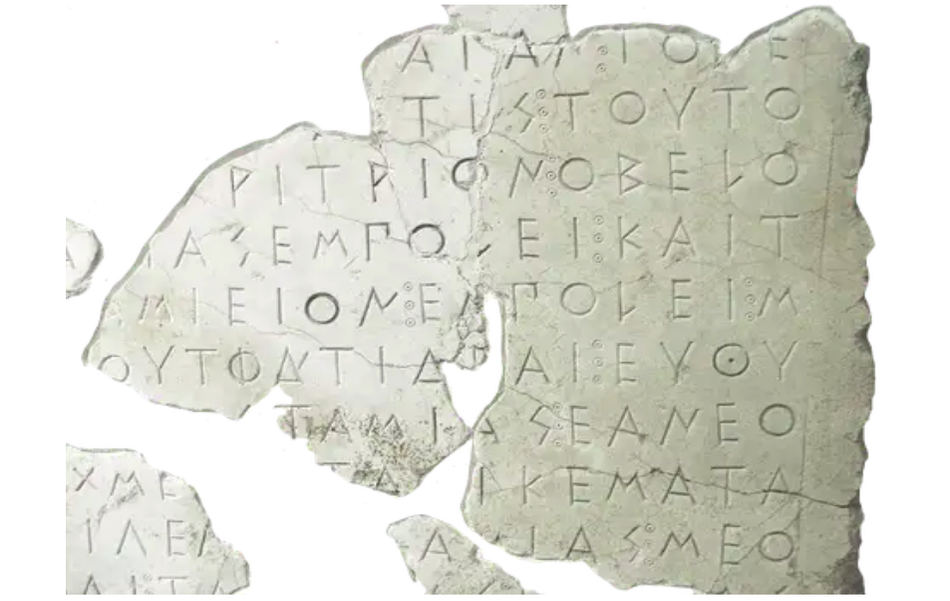
A group of researchers from Google’s Deepmind has published their most recent work on restoring ancient text using deep learning. In their novel paper named, “Restoring ancient text using deep learning: a case study on Greek epigraphy“ researchers present the first ancient text restoration model that is able to recover missing pieces from text fragments.
The method called Pythia uses character-level deep neural networks that can recover characters in damaged texts written in Greek. Researchers designed the method in a way that it takes sequences of damaged text and predicts the missing characters using long-term context information. In their architecture, they employed Long-Short Term Memory networks(LSTMs) (both an encoder and a decoder) which in the past have proven to be able to memorize longer sequences and provide context for making more precise predictions. Each input to the model is first encoded using a lookup table of learnable vector embeddings before it is passed to the recurrent neural network model for predicting the missing characters.
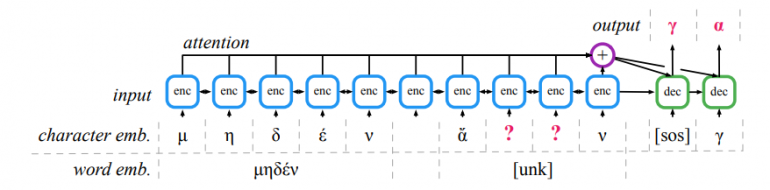
For evaluation of the proposed method, researchers built a data processing pipeline in order to convert the largest digital corpus of ancient Greek inscriptions to machine-readable text. The corpus was named PHI-ML after the name of the original corpus – PHI Greek Inscriptions. This corpus was used to compare the predicted results of Pythia with the results of human ancient historians. Researchers report that Pythia achieves a 30.1% error rate on character level, while human experts (historians from Oxford) achieve 57.3%. The results achieved by Pythia are set to be the new state-of-the-art scores in ancient text restoration.
In detail explanation of the method and the architecture of Pythia as well as the evaluations can be found in the official paper published on arxiv. Additionally, more details about the method and its development can be read in the official blog post.