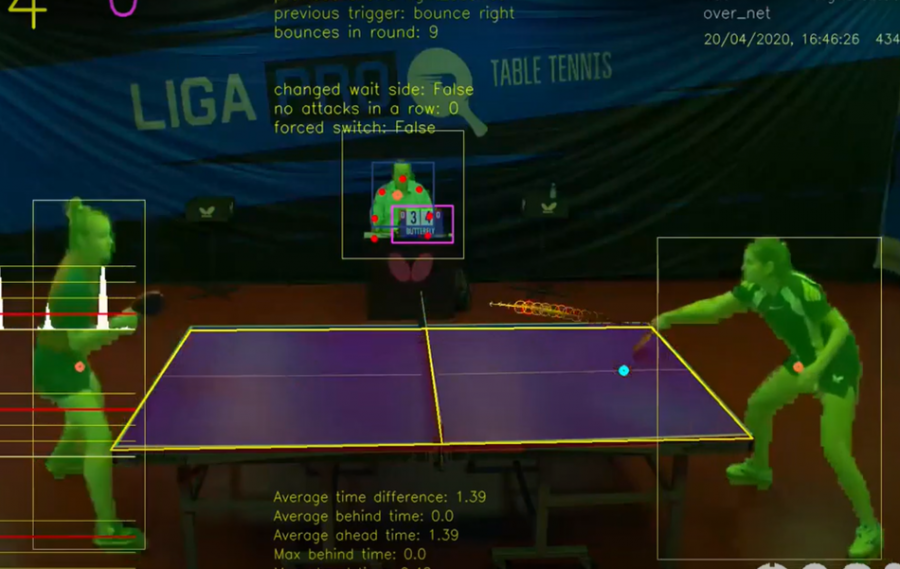
A group of researchers from OSAI (Constanta) has designed a deep neural network for real-time analysis of table-tennis videos. Their model, named TTNet is able to extract temporal and spatial information from table tennis match videos which can be further used by an auto-referee system.
The proposed model detects “special” events during the game such as bounces and net hits of the ball and it also provides spatial information in terms of player and field semantic segmentation, ball detection, etc. The architecture of the model consists of four blocks: a, b, c, and d. These blocks are responsible for: ball detection (a and b), semantic segmentation of field and players (block c), and events spotting (block d). All the components of these blocks are shown in the diagram below.
In order to develop and train such a model, researchers first collected a multi-task dataset, called OpenTTGames, of videos of table tennis, with labels for the events, segmentation masks, ball coordinates, etc. The designed neural network was tested using this dataset and researchers report 97% accuracy in-game events spotting with an error of 2 pixels (RMSE) in ball detection.
Researchers mention that their model achieves real-time inference and thus can be used as part of a real-time auto-referee system for table tennis. More about the method can be read in the paper published on arxiv.