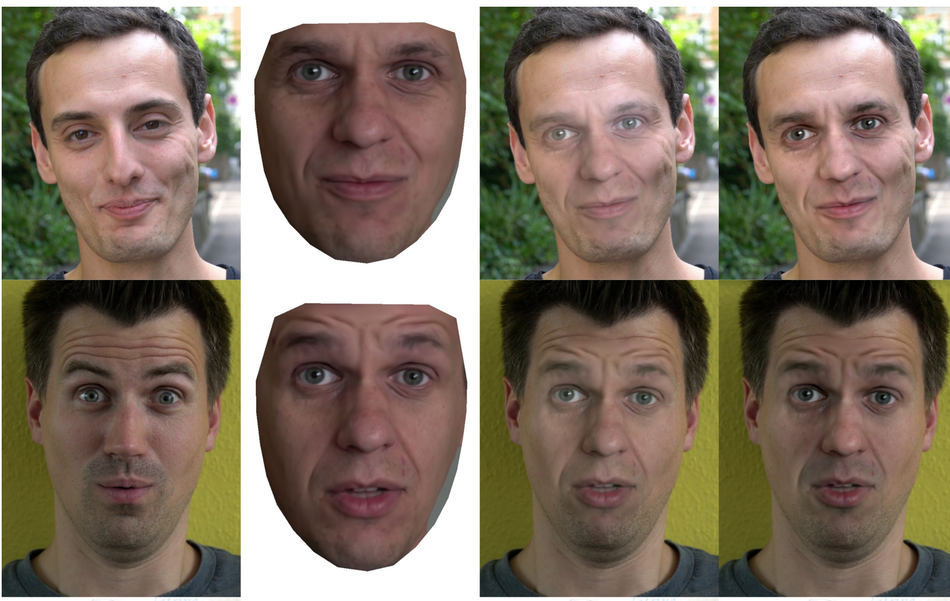
A group of researchers from the Disney Research Studios and ETH University have proposed a new deep learning-based method for face swapping that is able to produce highly realistic high-resolution images of swapped faces.
In their research paper, “High-Resolution Neural Face Swapping for Visual Effects”, they describe their novel approach which yields superior results over existing state-of-the-art methods. The proposed face-swapping method leverages the power of progressive training in order to train a multi-way comb network together with a blending method.
Researchers propose to use an encoder-decoder deep neural network which will provide an output image of a face that is suited for swapping on a given particular image. In fact, the network consists of a common encoder that takes the input image and encodes it into a latent vector which can later be decoded by a number of different decoders trained independently. The output face reconstruction from this decoder is finally merged to the input image using the proposed multi-band blending method.
Defined in this way, the method achieves identity transformation through a domain-transfer approach. Having a single shared encoder and multiple decoders makes it possible to generate a number of decoding paths to different domains (person identities). This kind of model was named a “comb” model in the paper.
To train and evaluate the proposed neural network model, researchers collected their own high-resolution dataset of human faces. The comparison with existing and open-source face-swapping methods showed that the proposed method outperforms all other methods.
More details about the new deep neural network for face swapping can be read in the paper or in the official blog post.