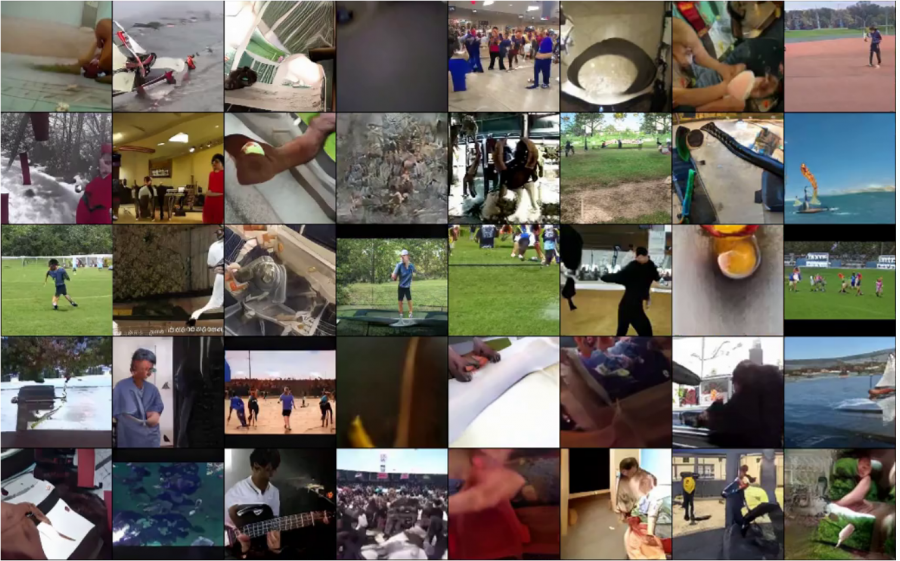
Researchers from Google Deepmind have proposed a novel method for efficiently generating videos using a deep neural network.
In their paper, named “Efficient Video Generation on Complex Datasets”, researchers led by Aidan Clark, describe their method based on Generative Adversarial Networks. The proposed method – DVD-GAN (Dual Video Discriminator) GAN is able to synthesize realistic new videos with a length of up to 48 frames and a resolution of 256×256.
The idea behind DVD-GAN is to employ a large- image generation model such as BigGAN for generating video frames. In order to do this, researchers proposed several techniques for accelerating training of BigGAN model and scaling it to video generation.
Since video generation differs greatly from image generation in the sense of the requirement of temporal consistency, researchers designed a specific architecture that will be able to take care of this. The proposed DVD-GAN employs self-attention and Recurrent Neural Network (RNN) units as well as a dual discriminator architecture to deal with the temporal dimension. The two discriminators are a spatial discriminator, which critiques the frame content and a temporal discriminator which critiques the motion i.e. forces the generator to generate movements of objects within several frames.
This kind of architecture helped researchers obtain a method that can generate realistic videos. The method was evaluated on the tasks of video synthesis and class-conditional video synthesis. Researchers showed that the method achieves state-of-the-art results on the Kinetics-600 dataset (for Frechet Inception score) and also state-of-the-art results UCF-101 (for Inception score).
