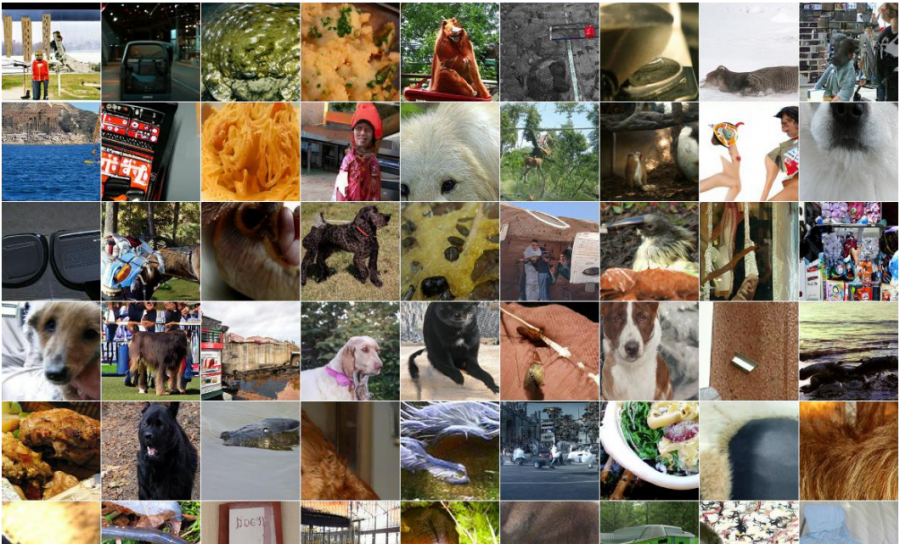
In the past years, Generative Adversarial Networks (or GANs) have shown unprecedented performance on tasks such as image generation. In a novel paper, researchers from Google Deepmind proposed a novel approach where GANs are used for large-scale adversarial representation learning.
The new approach named BigBiGAN, inspired by the state-of-the-art BigGAN model, extends the BigGAN model to representation learning. Researchers proposed a model that is, in fact, a bi-directional GAN model with BigGAN as a generator. They discovered that the DCGAN architecture used in BiGAN is not capable of modeling high-quality images. For this reason, they decided to use the state-of-the-art model (BigGAN) for improving the representation learning capacity. In this way, they wanted to leverage the generative capabilities of the BigGAN model in the framework of representation learning as defined with bi-directional GAN models.
Researchers designed a specific architecture that will provide the best of both worlds and they refer to their model as BigBiGAN – bi-directional BigGAN. They showed that their approach works for improving the representation learning performance of bi-directional GAN models (or GAN models in general). The proposed BigBiGAN model achieves state-of-the-art performance in unsupervised representation learning on ImageNet, and in unconditional image generation.
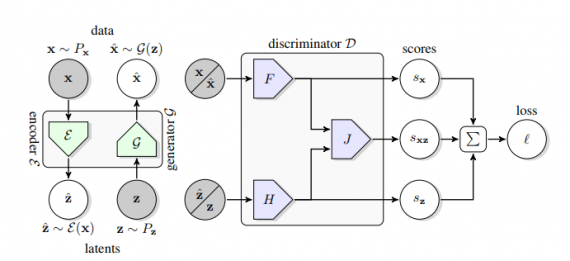
This work showed the potential of powerful generative models towards representation learning and researchers mention that the advances in generative models will be beneficial for representation learning. More details about the proposed BigBiGAN model can be found in the paper available on arxiv.