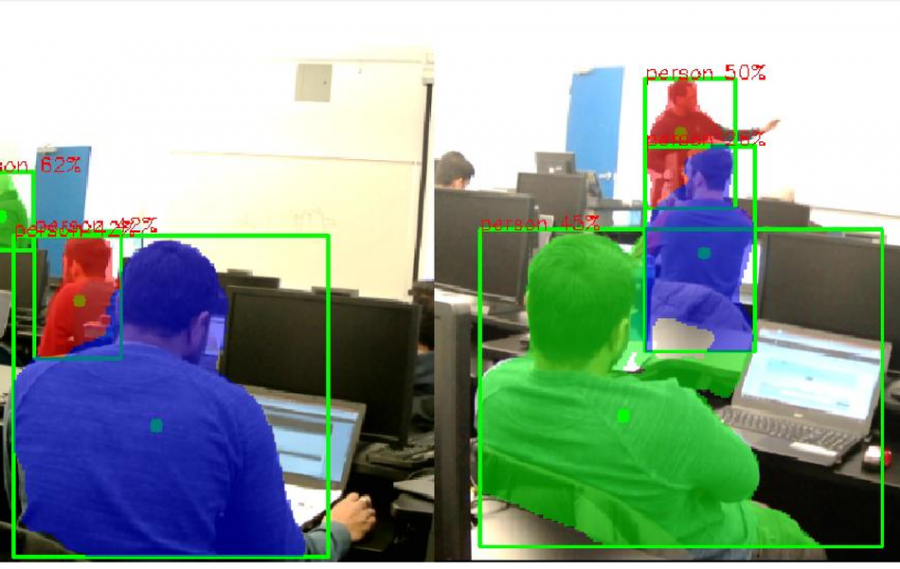
A group of researchers from Lakehead University in Canada has proposed a new single-shot instance segmentation method called EOLO.
The name of the method – EOLO, stands for “Embedded Object Segmentation only Look Once”. It is an anchor-free object segmentation method that relies on the idea of reformulating the instance segmentation problem as predicting semantic segmentation and then distinguishing overlapping objects problem.
Within the framework of single-shot instance segmentation, researchers designed and trained a network that does instance center classification and 4D distance regression to each pixel of an object. In other words, they redefine the instance segmentation with a 4D vector and semantic segmentation results.
The architecture of the proposed network is rather simple and it consists of a Mobilenetv3 backbone network, a feature pyramid network and three heads for each of the defined tasks: center of gravity prediction, 4D size prediction, and segmentation. The method first detects objects and does the semantic segmentation and then it combines the results to get instance segmentation.
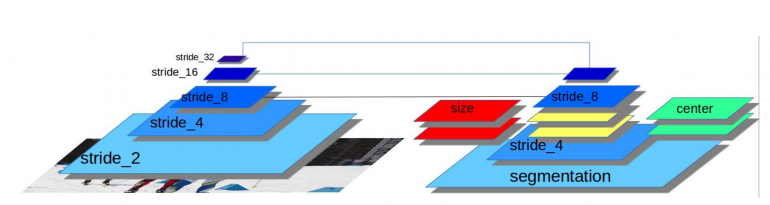
It was trained on the popular MS COCO 2017 instance segmentation dataset and it was compared to state-of-the-art methods in instance segmentation. As researchers reported, the proposed EOLO method does not outperform existing methods in instance segmentation, however, it achieves much faster inference with an average segmentation precision. Using Mobilenetv3 backbone, the method achieves an AP of 11.7 at 30 FPS processing on a single 1080Ti GPU.
Researchers mention that EOLO can be useful and can serve as a cornerstone for other instance-level recognition tasks due to its simplicity, flexibility and acceptable performance.