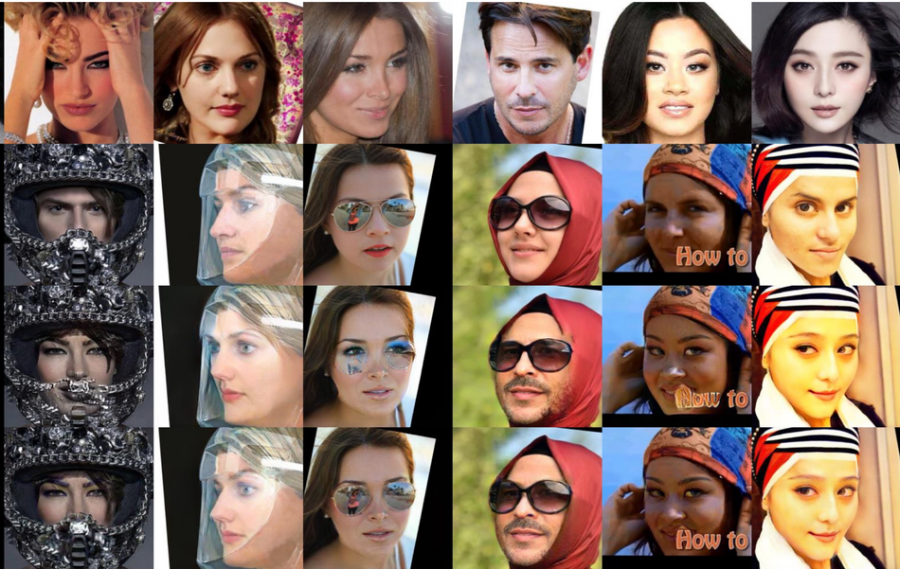
Researchers from Microsoft and Peking University have proposed a new method for face swapping in images called FaceShifter.
In their two published papers, FaceShifter and Face X-Ray, researchers propose a new framework that produces highly realistic face-swapping even under occlusions and while preserving the person’s identity. The novel method is based on a multi-stage architecture where in the initial stage it extracts target face attributes that are later adaptively fused during the following stages to generate a realistic output image.
Researchers introduced a number of improvements as part of the FaceShifter method. They proposed a new attributes encoder, a new generator network with so-called Adaptive Attentional Denormalization layers and a new network that is able to recover anomaly regions called HEAR-Net or Heuristic Error Acknowledging Refinement Network. The proposed architecture combines these modules in a way that the method provides occlusion aware face-swapping.
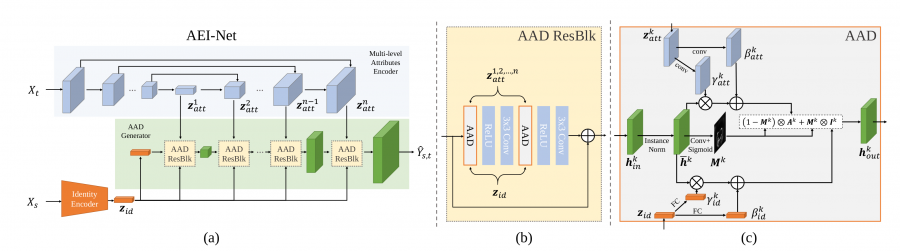
Researchers designed and performed several experiments to show the effectiveness of the proposed method. The first network named Adaptive Embedding Integration network (AEI) was trained using the CELEBA-HQ, FFHQ, and VGGFace datasets. The HEAR-Net network was subsequently trained using a portion of the samples that have a top-10% error in the datasets that the encoder was trained on.
The evaluations arising from several ablation studies showed that the proposed method outperforms existing methods for face swapping. FaceShifter shows superior performance in generating realistic and perceptually appealing face-swapped images. The experiments also showed that it performs better than state-of-the-art methods in identity preservation.
More about the new method for face swapping can be read in the paper published on arxiv.