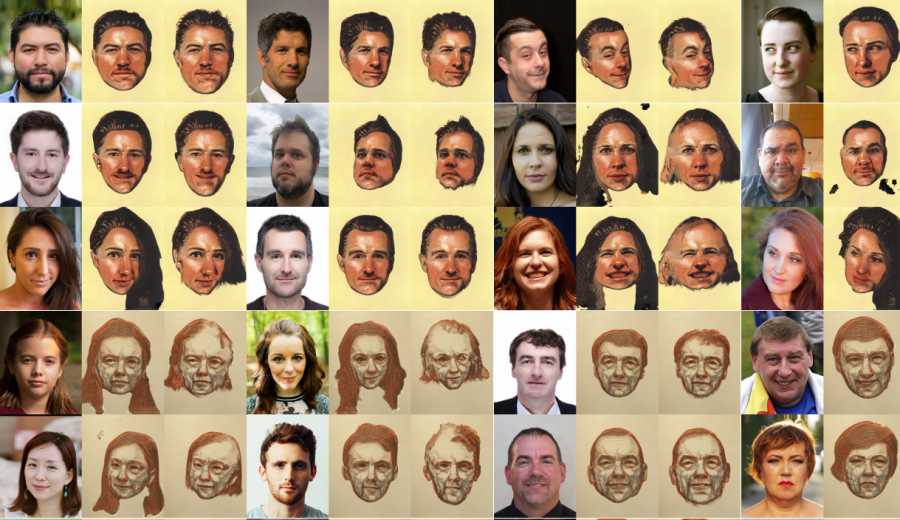
Researchers from the Czech Technical University and Snap Inc. have proposed a novel method that given a portrait image and a style exemplar generates a portrait of a desired style. The method is based on Generative Adversarial Networks and it outperforms current state-of-the-art method in computational overhead with similar visual quality in the results.
FaceStyleGAN Network
In fact, researchers tried to reproduce the output of an existing portrait synthesis algorithm called FaceStyle by employing a conditional Generative Adversarial Network.
The proposed neural network model outperforms the FaceStyle algorithm in terms of computational cost. It also enables real-time high-quality style transfer for portrait videos due to the sufficiently fast inference of the network on GPU. As the researchers mention in their paper, the conditional GAN network provides more visually pleasing results than the original algorithm in some cases due to its generalization power. Modeling such a mapping between the input and the output of an algorithm introduces more robustness and increases generalization capabilities.
The conditional Generative Adversarial Network uses a custom built generator which researchers designed upon the perceptual loss model by Johnson et al. The discriminator network, on the other hand, was taken from the PatchGAN architecture.
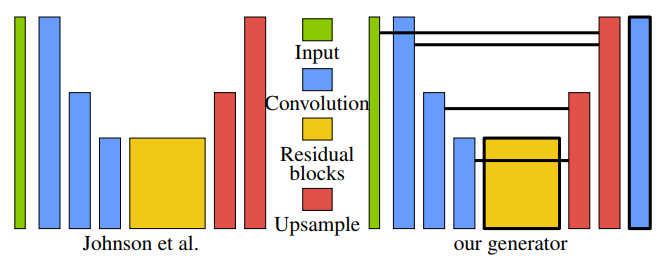
Evaluation and Results
The model was trained on seven different style exemplars and applied to 24 portraits. Researchers evaluated the performance by conducting a perceptual study where they asked 194 participants to rate the visual quality of the generated images.
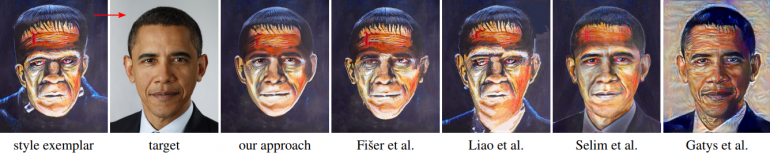
From the results obtained, researchers report that the visual quality of the proposed method is almost at the same level as FaceStyle generated images (with few percents of difference in the score). Therefore, they conclude that the method preserves the visual quality while significantly improving the generation time i.e. reducing the computational cost.
More about FaceStyleGAN method can be read in the paper published on arxiv. Researchers released a video showing the real-time style transfer using a web camera.
[…] الكامنة وراء التزييف العميق والأشخاص المصطنعين و العديد من فلاتر سناب شات. في هذه الحالة ، استخدم الباحثون شبكتهم التوليدية […]
[…] be remarkably realistic. Generative networks are the tech behind deepfakes, artificial people, and many Snapchat filters. In this case, the researchers used their generative network to normalize images read… Read more »