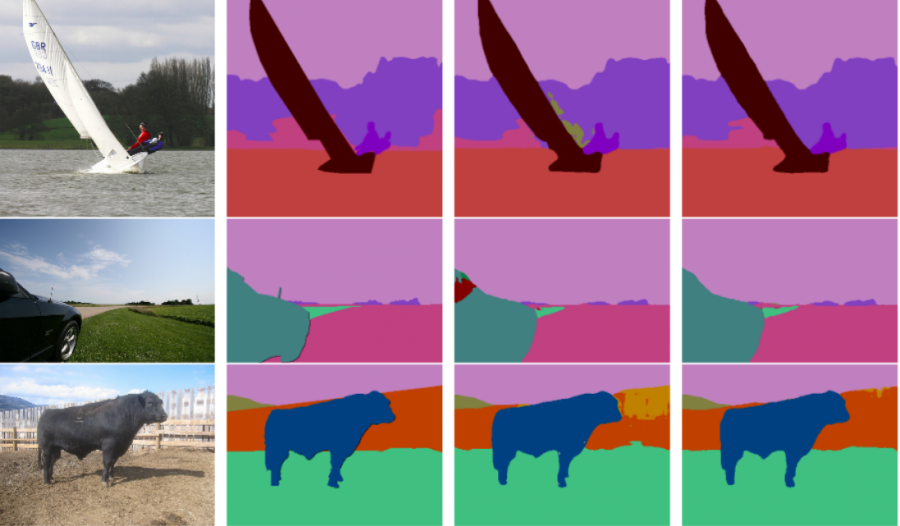
Researchers from the Chinese Academy of Sciences and Deepwise AI Lab, have proposed a new method for semantic segmentation which reduces computational complexity and achieves state-of-the-art results.
Semantic segmentation, as one of the most important tasks in computer vision, has been studied extensively in the past years. Modern approaches relying on deep learning have achieved remarkable success in solving this task and they usually employ Fully Convolutional Neural Networks.
In fact, the best results for semantic segmentation were obtained using downsampling-upsampling, encoder-decoder architectures and dilated convolutions.
In the novel method, called FastFCN, researchers propose to replace the time and memory consuming dilated convolutions with a joint upsampling module named JPU (Joint Pyramid Upsampling).
The Joint Pyramid Upsampling module is designed for generating a feature map that approximates the activations of the final feature map of a DilatedFCN (a fully convolutional network with dilated convolutions). In order to approximate those activations, researchers reformulate the problem into the problem of joint upsampling of different layers. Then they resolve this problem by designing a separate Convolutional Neural Network.
Evaluations show that the proposed upsampling method is superior and reduces the computational complexity by more than three times. Researchers show that the method can be applied to other existing approaches to reduce complexity and improve performance.
The proposed semantic segmentation method achieves state-of-the-art results in the Pascal Context dataset with mIoU of 53.13% and the ADE20K dataset. In both of the cases, the computational complexity is reduced by more than three times.
The implementation of the proposed method was open-sourced and is available here.