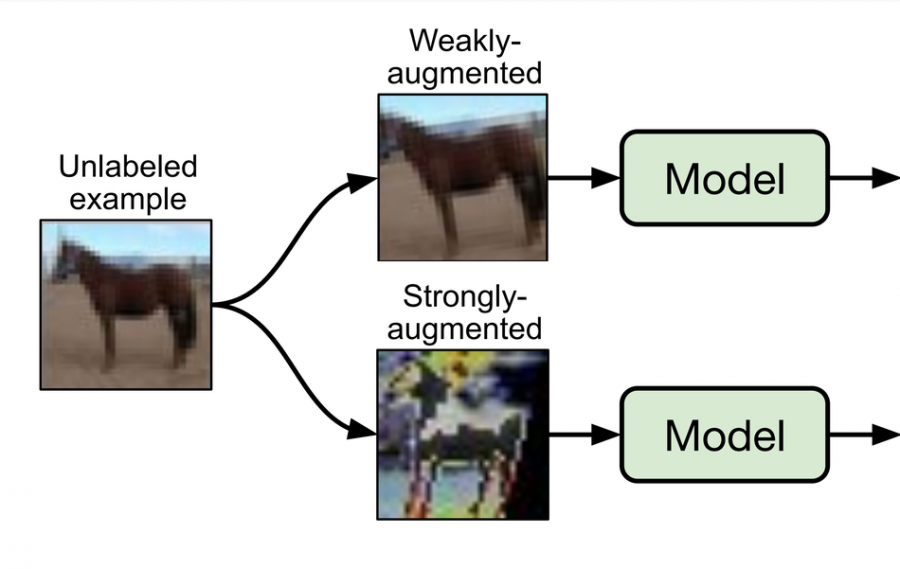
Researchers from Google Research have proposed a new state-of-the-art method in semi-supervised image classification.
The problem of image classification has been successfully tackled in the past several years thanks to the development and success of deep convolutional neural networks. However, going beyond some accuracy threshold requires large amounts of labeled data which is often expensive to retrieve. Researchers in the last couple of years have developed a large number of methods that overcome this limitation by using semi-supervised and weakly supervised learning.
In their new paper, researchers from Google propose a novel semi-supervised approach that outperforms existing methods on the popular CIFAR-10 benchmark achieving 94.93% accuracy. The method, called FixMatch follows a simple idea and combines two common semi-supervised learning methods: consistency regularization and pseudo-labels.
In fact, within the proposed framework there are two branches: the first one that accepts a weakly-augmented image from the original image and predicts pseudo-labels, and the second one that gets a strongly-augmented image and tries to predict the true label. The second model is trained by backpropagating the error obtained comparing the prediction with the predicted pseudo-label from the
first model.
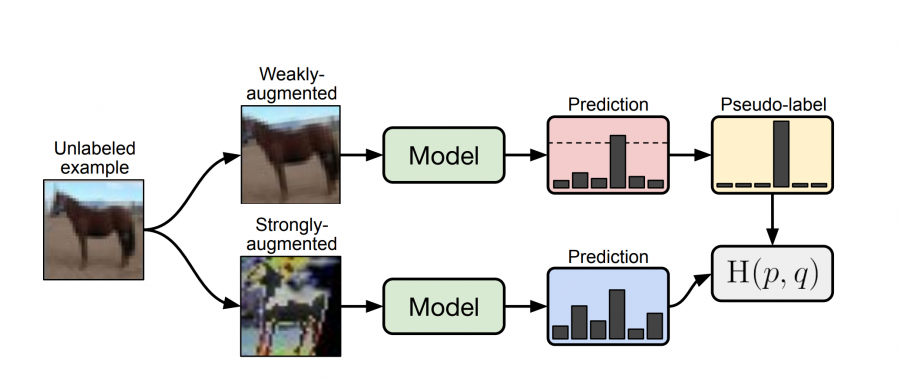
The proposed method was evaluated using several datasets and benchmarks including CIFAR-10, CIFAR-100, ImageNet, and STL-10. Researchers report that FixMatch achieves state-of-the-art results on several datasets despite its simplicity. According to them, the method achieves 94.93% accuracy on CIFAR-10 with 250 labels.
More in detail about the new method can be read in the paper. The implementation was open-sourced and the code is available on Github.