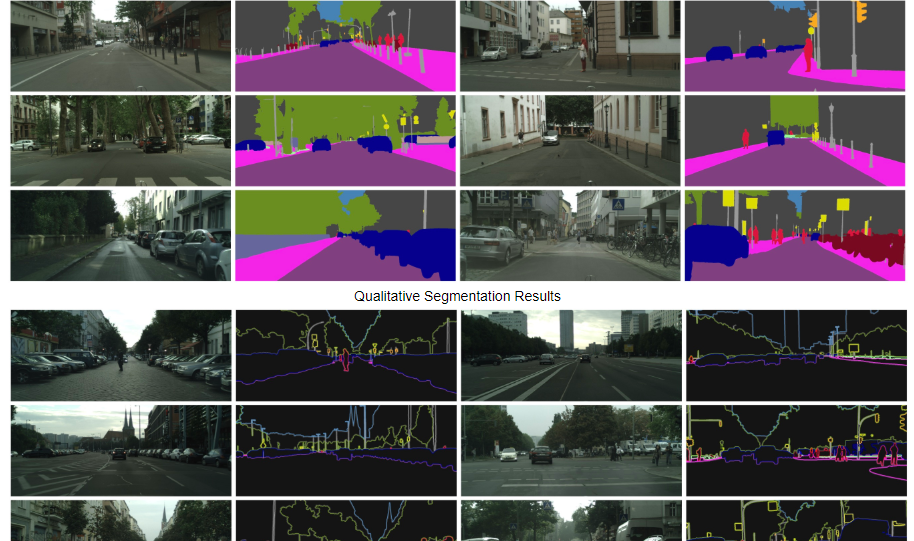
A group of researchers from NVIDIA, the University of Waterloo, the University of Toronto and the Vector Institute have published a new state-of-the-art method for semantic segmentation.
The novel method outperforms current state-of-the-art methods on the Cityscapes benchmark dataset by large margins (2% for mIoU, mean intersection-over-union and 4% on boundary F-score). Researchers propose an interesting architecture composed of two streams – a segmentation stream and a shape stream. The key idea here is to divide the task of image segmentation into semantic segmentation prediction and boundaries prediction. In order to exploit this kind of architecture, researchers introduce a type of gates between the two streams (or branches) that allow the shape stream to learn more robust features using the higher-level activations from the classical (segmentation) stream. They call this architecture a “Gated Shape Convolutional Neural Network” (Gated-SCNN). The last module of the architecture is fusing the output of both streams to produce the final output. The network was trained using segmentation as well as “dual-task” loss.

Researchers showed that this kind of architecture is able to learn more precise boundaries, especially when segmenting smaller objects. The evaluations show that it outperforms strong baseline models such as DeepLabV3, PSP-Net on the Cityscapes dataset.
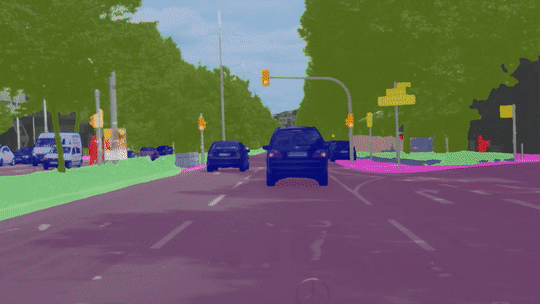
Outputs from the model as well as more details about the method can be found on the official project website. The paper is available on arxiv, while the code will be open-sourced soon, according to the information on the website.