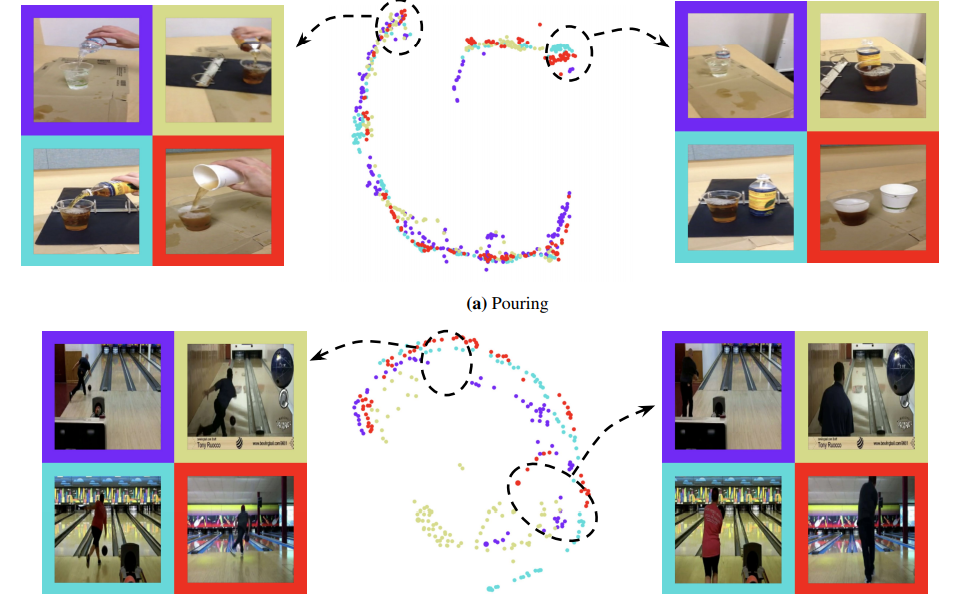
A group of researchers from Google Research and Deepmind has proposed a novel, self-supervised method for video understanding. The new method, called Temporal Cycle-Consistency Learning (TCC) is able to align videos which share similar sequential processes in order to learn video representations suitable for video understanding.
Video understanding is a topic that was well explored in the past but still many challenges persist. Researchers from Google, tackle this problem from the perspective of machine learning (or more precisely deep learning) and employ a deep neural network to learn latent embeddings for better content understanding. Their algorithm is trying to find frame correspondences across videos containing the same action by leveraging the principle of cycle-consistency.
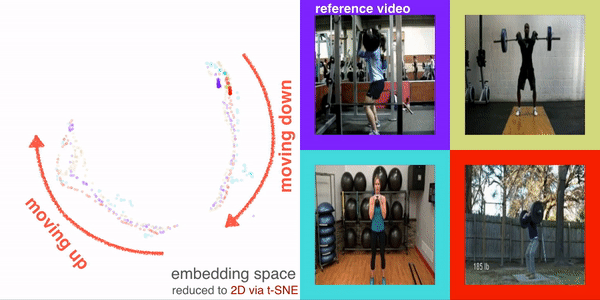
In order to learn such correspondences, researchers designed and trained a “frame encoder” network, which takes a video frame as input and generates latent space embeddings for that video. The TCC algorithm then selects two videos from the same action, it defines a reference frame from the first video and finds its nearest neighbor frame from the second video. The distance between the frames is computed in the latent space (not in the original pixel space). This allows the method to find frame correspondences which actually contain the same moment (or part of an action).
Researchers mention that a method for video understanding such as TCC has a number of applications. Some of them include few-shot action classification (the method can learn to classify videos without large amounts of supervision data), unsupervised video alignment, modality transfer between videos (such as sound or semantic labels transfer from one video to another), per-frame video retrieval, etc.
More about the method can be read in the official blog post. Researchers also released the implementation and it is available on Github.