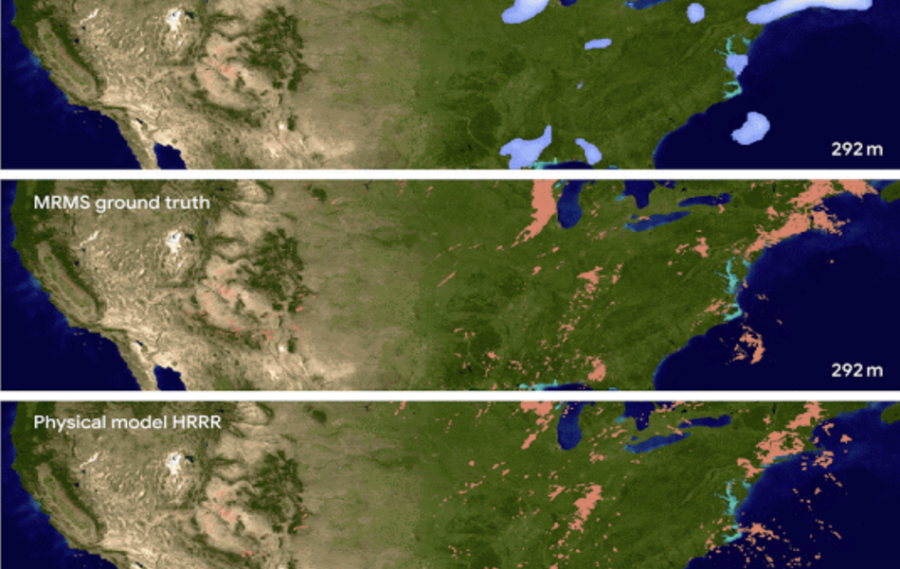
Researchers from Google have developed a new deep neural network that makes weather forecasts on par or better than physical models.
Today, in a new blog post, researchers from Google AI have presented a new method for precipitation forecasting that relies completely on deep learning and has no explicit knowledge of physical laws that describe atmosphere dynamics and govern weather conditions changes. The new Neural Weather Model, called MetNet, builds on previous research done by Google researchers on precipitation nowcasting (which is essentially weather predictions within a relatively short period of time).
According to researchers, MetNet is capable of doing precipitation forecasts for a 1km resolution (1 km x 1km earth spatial cells), over two-minute intervals and it outperforms current state-of-the-art physical models used by United State’s National Oceanic and Atmospheric Administration for predictions up to 8 hours. The new model also outperforms the physics-based methods in terms of inference speed and gives results in a matter of seconds, which is a huge improvement as opposed to physical models that take hours of computational time.
MetNet learns to do precipitation forecasting directly and only from observational data. The input to the model is image-like data coming from two sources: radar stations and satellite networks. The network gives predictions for an area of 64 km x 64 km using 1 km resolution. To achieve up-to-8-hour precision on such area, the model needs to take into account a larger portion of the space, namely around 1024 km x 1024 km area, taking into account the dynamics and movement of clouds over this time period.
The architecture of the network consists of three main parts: a spatial downsampler, a temporal encoder, and a spatial aggregator. The downsampler first reduces the spatial dimensions in the input patches and therefore decreases memory consumption, making the forecasting for larger resolutions possible. The Temporal Encoder, on the other hand, uses Convolutional LSTMs to capture long term temporal dependencies and it provides it’s output to the Spatial Aggregator which uses self-attention to capture long-range spatial dependencies, instead. Finally, the output of the network is a probability distribution as an estimate of precipitation for each of the 1 km x 1km blocks.
The model was compared to two physics-based models – NOA’s HRRR (High-Resolution Rapid Refresh) system and an optical flow method for precipitation forecasting. MetNet outperforms both of the baseline methods for predictions up to 8 hours, in terms of the measured F1 score. Researchers mention that MetNet is several orders of magnitude faster since it can give predictions in matter of seconds on TPU hardware, whereas the baseline physical models take hours on powerful supercomputers.
More about the new deep learning-based weather forecasting model can be read in the official blog post. The paper was published on arxiv.