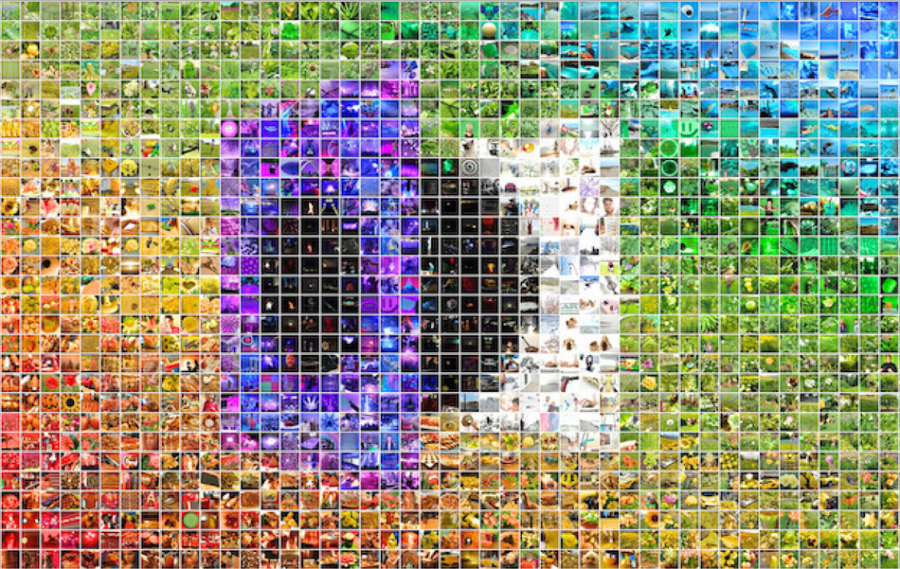
Researchers and engineers from Menlo Park-based startup Leia Inc., have collected and released a large-scale stereo image dataset with in-the-wild images.
The dataset called Holopix50k, was collected with the main objective of having variable and large-scale collection of stereo images. According to the researchers, existing datasets are limited both in size and variability and that heavily influences the performance of state-of-the-art methods, especially the ones based on deep neural networks and learning from data. Taking this into account and the availability of dual-camera phones nowadays, they collected more than 49 thousand in-the-wild stereo images from the mobile social media platform Holopix.
The data in Holopix50k is coming mainly from RED Hydrogen One mobile phone. Initially, researchers collected around 70 thousand stereo image pairs and after several post-processing steps, the final number of images left in the dataset was around 50 thousand. Mismatching images in terms of the resolution were downscaled, then stereo pairs with vertical disparity were removed and finally, disparity-based filtering was applied to filter the remaining images and remove the pairs with disparity failures.
The contribution of such a dataset was measured by experimentally testing state-of-the-art models trained using Holopix50k data in tasks such as stereo image super-resolution and monocular depth estimation. Researchers designed the experiments so that the same method was evaluated using several datasets. Quantitative and qualitative evaluations show that the novel dataset significantly improves results on both of these tasks and models trained with Holopix50k outperform models trained with popular datasets such as KITTI, ETH 3D, Flickr 1024, WSVD, etc.
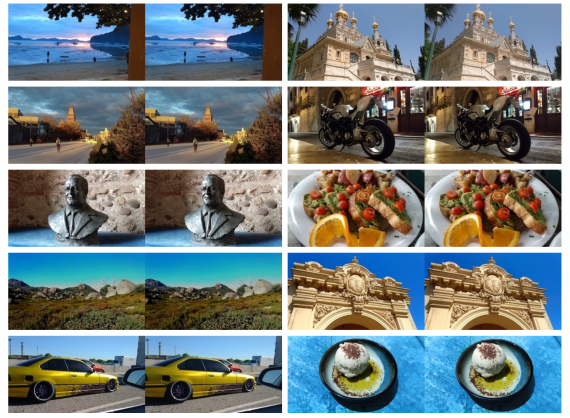
Researchers mention that the new dataset is an order of magnitude larger than any of the existing stereo datasets of a similar kind. The dataset was open-sourced and is available for academic usage and non-commercial purposes.