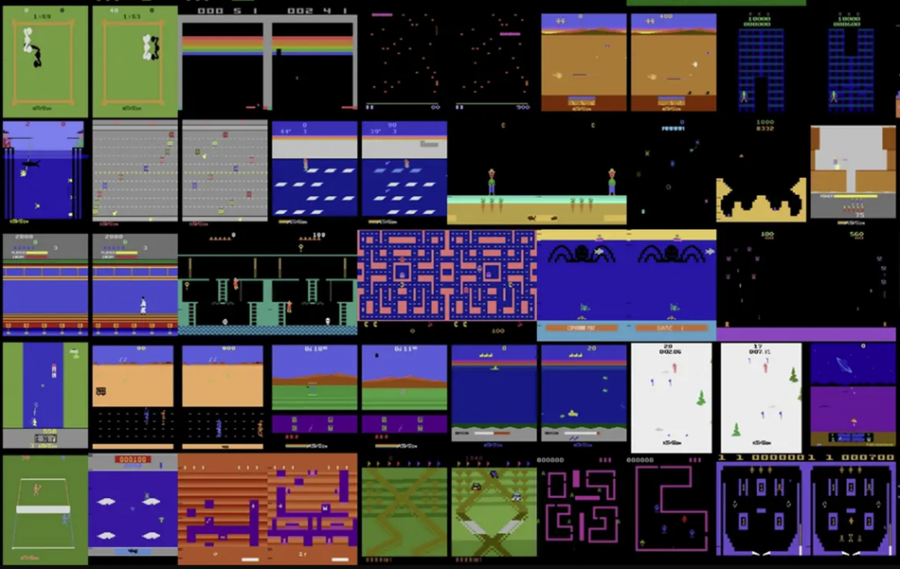
DeepMind has announced that they have developed a Reinforcement Learning (RL) agent that outperforms human baselines in all 57 Atari games, as part of the Atari57 suite.
Back in 2012, the so-called Arcade Learning Environment or Atari57 benchmark was introduced as a collection of games that pose a number of challenges for reinforcement learning agents. Since then, the benchmark was heavily used by the research community but no agent was able to achieve better-than-human performance on all of the tasks. The latter was true especially for 4 of the games in the suite: Montezuma’s revenge, Pitfall, Solaris and Skiing.
The novel method called Agent57 is able to achieve above-human performance on all of the 57 games present in the suite. The agent is based on DQN or Deep Q-network Agents, method which was also developed by researchers from DeepMind. This agent, abbreviated as DQN was to some extent successful in tackling numerous challenges in reinforcement learning. Many of the other RL methods proposed later were based on those kinds of DQN networks. Agent57 also builds upon some of these works and in particular upon improvements of DQN in terms of short-term memory, episodic memory, exploration, etc. One important improvement over existing methods was the introduction of a so-called meta-controller in Agent57, which allows the agent to learn how to balance exploration and exploitation – one of the key problems in Reinforcement Learning.
The new agent is built on top of the idea of learning when it’s better to exploit and when it is better to explore. It computes a mixture of long and short term intrinsic motivation to both explore and learn a family of policies, and the choice of the policy is done by its meta-controller. The controller acts as a teacher and determines what data will the agent learn from and it allows the agent to choose between the exploration-exploitation tradeoff.
According to DeepMind researchers, Agent57 is one step closer to artificial general intelligence, as it is a more generally intelligent agent that achieves above-human performance on the given tasks. They also mention that the agent is able to scale with increasing amounts of computation and data: longer training and more data imply higher scores.
More about Agent57 can be read in DeepMind’s official blog post or in the paper published on arxiv.