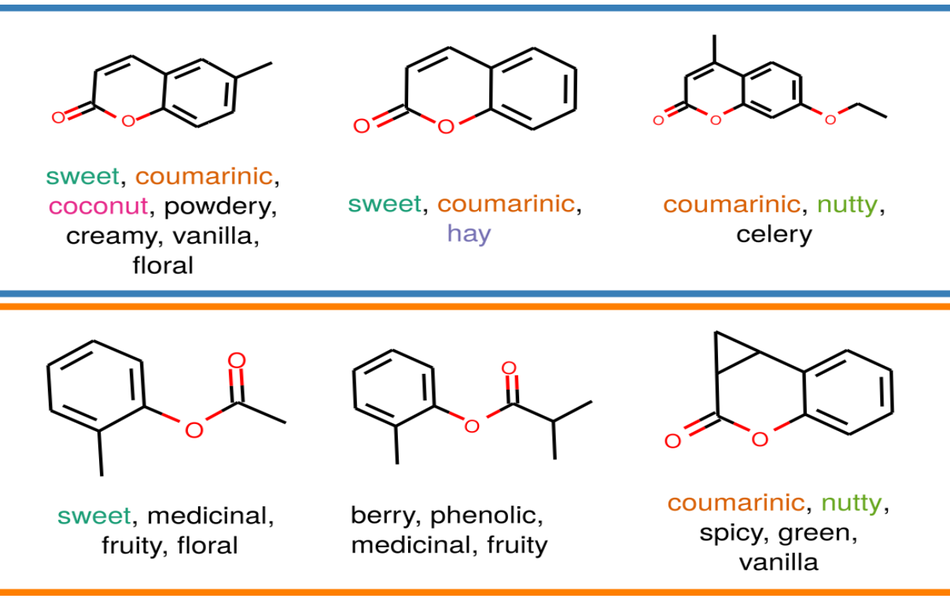
A group of researchers from Google AI has published their work in learning odor descriptors as perceptual representations of small molecules. In the latest Google AI blog post, Alexander B. Wiltschko, a Senior Research Scientist at Google Research, explains their novel method that uses deep learning to learn smell.
He is arguing that in the past, researchers in the field of machine learning have focused mostly, if not only at vision and hearing, and smell has received not even nearly as much attention as visual and acoustic perception.
“For humans, our sense of smell is tied to our ability to enjoy food and can also trigger vivid memories. Smell allows us to appreciate all of the fragrances that abound in our everyday lives, be they the proverbial roses, a batch of freshly baked cookies, or a favorite perfume. Yet despite its importance, smell has not received the same level of attention from machine learning researchers as have vision and hearing.”, says Alexander B. Wiltschko.
In their paper, named “Machine Learning for Scent: Learning Generalizable Perceptual Representations of Small Molecules”, Alexander and his research group present a novel method that leverages the power of graph neural networks for predicting the odor descriptors of individual molecules. They propose a GNN model that takes a molecule graph representation as input and classifies it using pre-defined odor classes. Additionally, the predictions of the network are embedded in a common embedding space using graph embeddings. This allows to group molecules according to their odor properties and ultimately define “meaningful” groups by the predicted smell.
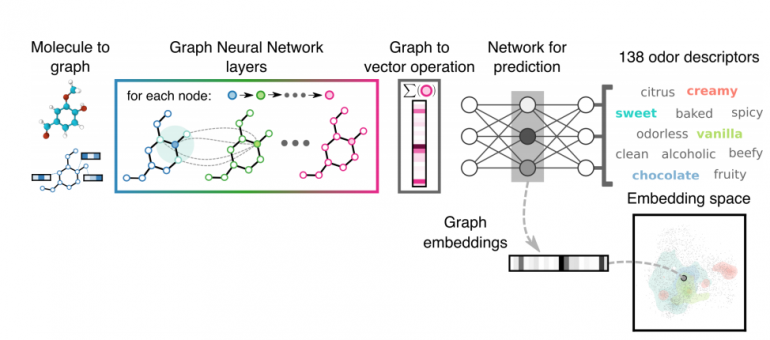
Researchers demonstrate that their method significantly outperforms current state-of-the-art methods in odor prediction. They also showed that it is possible to capture meaningful structure regarding percepted smell and therefore extract clusters of groups of odorants.
More details about the method can be found in the paper or in the official blog post.