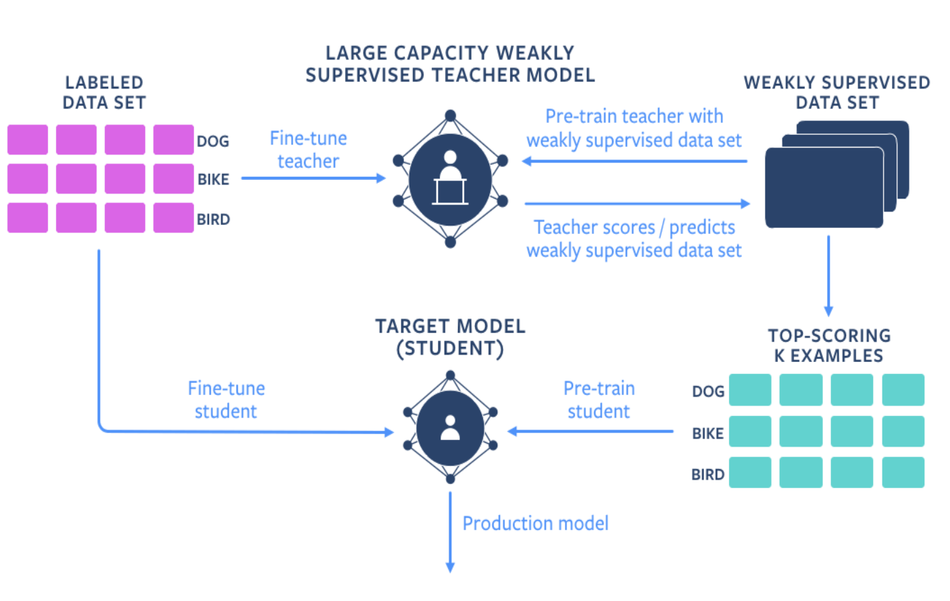
Researchers from Facebook AI Research (FAIR) have announced their work on a new concept in deep learning called Semi-weakly Supervised Learning.
The new approach that the researchers propose, in fact, combines the merits of both well-known training methods: semi-supervised and weakly supervised learning. According to them, both weakly and semi-supervised learning have their limitations and on the other hand, a large amount of publicly available data (especially visual content such as photos and videos) is raw and unlabeled.
In order to make use of such vast amounts of data, considering the fact that labeling of training data cannot scale due to several reasons, researchers designed and proposed a new framework where a student-teacher network setting allows to combine both weakly- and semi-supervised learning.
The framework suggests that a highly-accurate teacher model is first pre-trained with weakly supervised data sets, and then subsequently fine-tuned with all available labeled data. This teacher network is then used to assign “more accurate” scores or class predictions for the weakly supervised data that was previously pre-trained with. Next, the target student model is pre-trained with the weakly supervised data with improved labels from the teacher. Finally, the labeled data is used to fine-tune the student model.

FAIR researchers report that they achieved new state-of-the-art results on academic benchmarks for lightweight image and video classification models. In fact, the proposed approach introduces large improvements in smaller models therefore beating the current highest-scoring models. As Facebook researchers mention, the novel semi-weak supervision allows decreasing the gap between state-of-the-art performance models and production-ready (usually lightweight) models in terms of accuracy.
The new method achieved 81.2 percent top-1 accuracy on ImageNet using the ResNet-50 model for our benchmarking tests. In the case of Kinetics video action classification, it achieved 74.2 percent top-1 accuracy on the validation set with a low-capacity R(2+1)D-18 model. Researchers note that this is a 2.7 percent improvement over the previous state of the art results obtained by the same capacity weakly supervised R(2+1)D-18 model using the same input data sets and compute resources.
