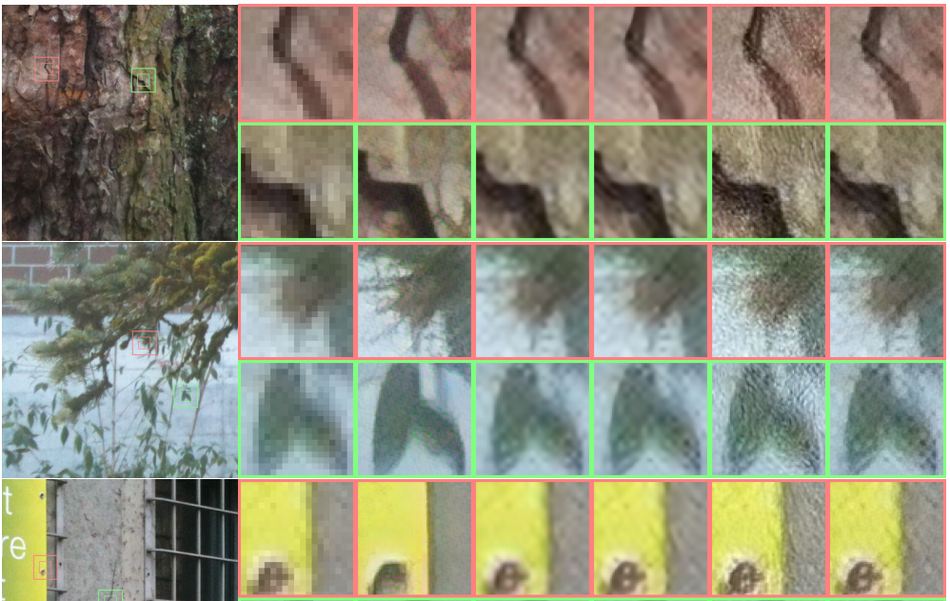
Researchers from ETH Zurich’s Computer Vision Lab have proposed a new, fully unsupervised method for image super-resolution. According to the evaluation, the novel method outperforms current state-of-the-art methods in this task.
In the past few years, a large number of super-resolution methods have been proposed, most of which rely on deep neural networks and supervised learning. A large number of unsupervised learning-based approaches rely on a technique called bicubic downsampling to generate low- and high-resolution image pairs again for supervised training of a deep network.
In their novel paper, researchers from ETH led by Andreas Lugmayr, argue that bicubic downsampling as such introduces significant artifacts in the images thus removing natural sensor noise and other real-world characteristics of the image itself. As stated in the paper, those artificially introduced artifacts will result in poor generalization capabilities of the model.
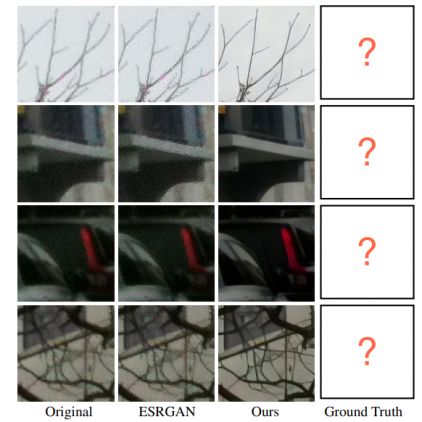
Instead, researchers propose an approach that learns to invert the effects of bicubic downsampling and generate more realistic image pairs for training. The approach leverages the power of Generative Adversarial Networks in a framework that allows training a generator network that can generate a pair of low- and high-resolution images from bicubic images. In this way, researchers tried to inverse the effects of bicubic downsampling and restore the natural image distribution in the generated set. They used cycle consistency loss function to learn to perform that operation as domain correction.
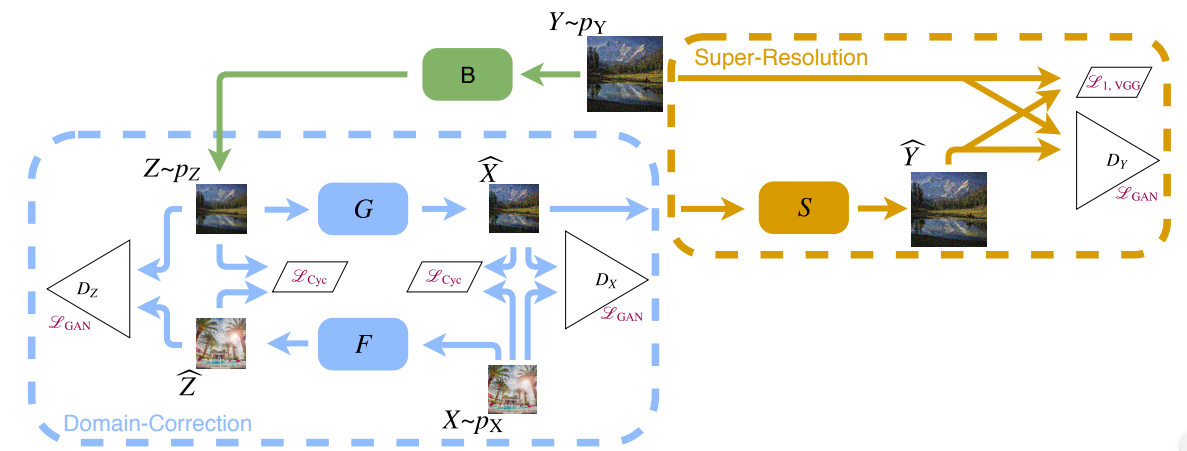
The quantitative and qualitative evaluation showed that the method achieves better or as good performance as other existing methods for image super-resolution.
More about the approach and the architecture of the neural network models can be read in the paper published on arxiv.