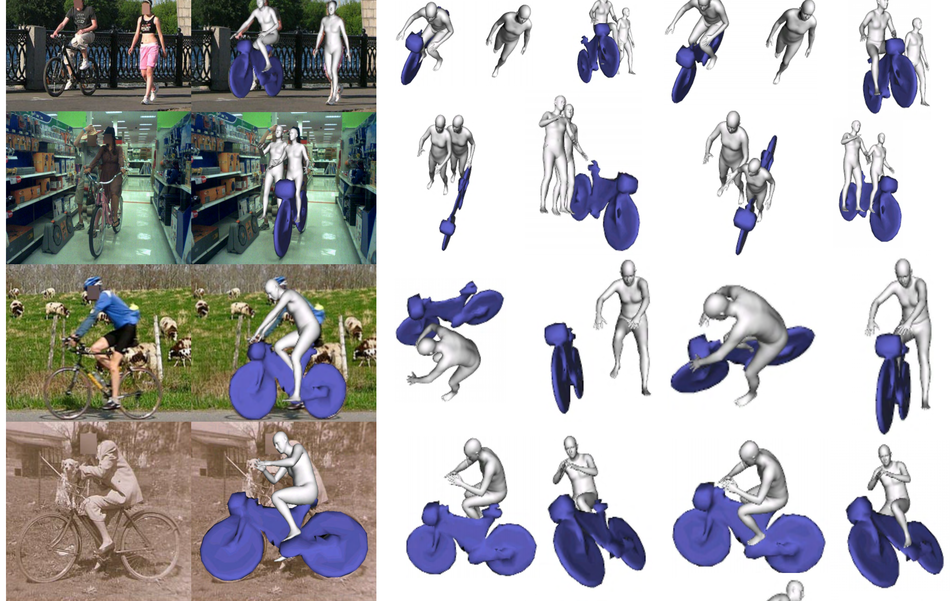
Researchers from the Carnegie Mellon University, Facebook AI Research, Argo AI, and the University of California have developed a method that successfully extracts 3D humans and objects along with their spatial relationship from a single in-the-wild input image.
The method abbreviated PHOSA (Perceiving 3D Human-Object Spatial Arrangements) works without any scene- or object-level supervision and it is able to extract plausible arrangements of humans and objects in the 3D space. In fact, researchers tried to leverage the so-called “3D common sense” by incorporating prior knowledge as constraints into the learning process which are used to resolve ambiguities. In this regard, they employ several loss terms such as scale loss (for object’s size), silhouette re-projection loss (for optimizing for human pose), and interaction loss (for optimizing for human-object spatial arrangements).
The proposed framework for learning 3D objects and spatial arrangements uses 3D human pose estimation models, instance segmentation models, 3D differentiable renderer together with the proposed constraints in the learning process in order to generate plausible reconstructions and configurations. The image below shows the diagram of PHOSA.
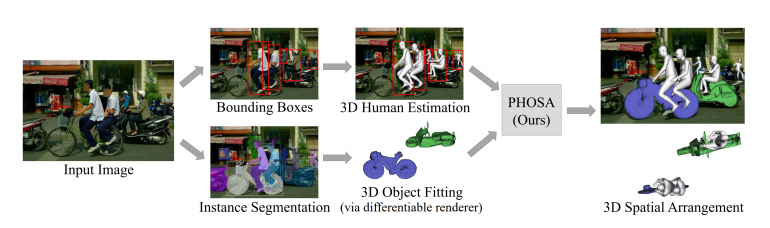
Through extensive experiments, researchers showed the effectiveness of the new method in reducing the space of possible configurations. They conducted quantitative and qualitative evaluations using the COCO-2017 dataset and explored in-the-wild cases where humans intract with big objects (surfboards, bikes, etc.) and also small handheld objects (laptops, tennis rackets, etc.). PHOSA performs well on a variety of everyday objects as part of a range of human-object interactions.
More details about the method, the experiments, and challenges can be read in the paper. The implementation of the method will be open-sourced and available on Github.