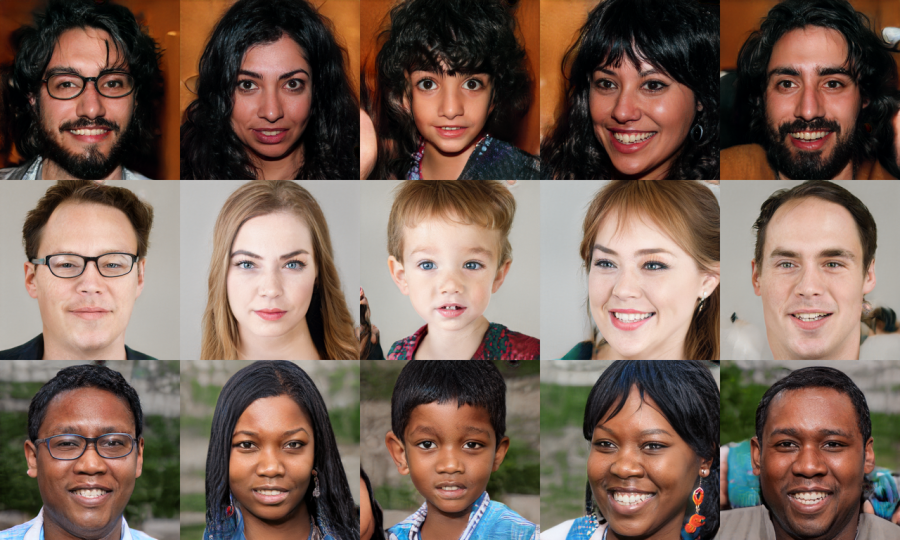
A new method developed by researchers from Google AI is achieving state-of-the-art results in high-fidelity image generation with GANs using 10 times fewer labels.
Generative Adversarial Networks have done huge breakthroughs and one of the most popular application has been image generation. Recently, methods like StyleGAN are able to generate high-fidelity natural images that are almost indistinguishable from real images. In fact, a number of architectural improvements over the past few years have traced the path to high-fidelity image generation.
When it comes to natural images, the state-of-the-art results have been achieved by a family of models called conditional GANs. These models use labels to capture multi-modal data distributions and to leverage the learned representations for more powerful image generation. However, as it is often the case, labeled data is not easy to acquire and this approach requires a large amount of labeled data to be able to generate high-fidelity images.
To overcome this problem, researchers from Google have proposed a method that leverages both conditional and unsupervised generation of high-fidelity images. The method is based on self-and semi-supervised learning and it achieves state-of-the-art results on ImageNet using only 10% of the labels.
The idea is actually to build a semantic feature extractor that is learned from the data via self-supervision and use a small subset of labels from which other labels can be inferred. Then the labels can be used for learning conditional distributions with conditional GANs.
Together with this announcement, Google released a major update of their GAN library called CompareGAN. More about the method and the improvements in the library can be read at the official blog post. The paper can be found here.