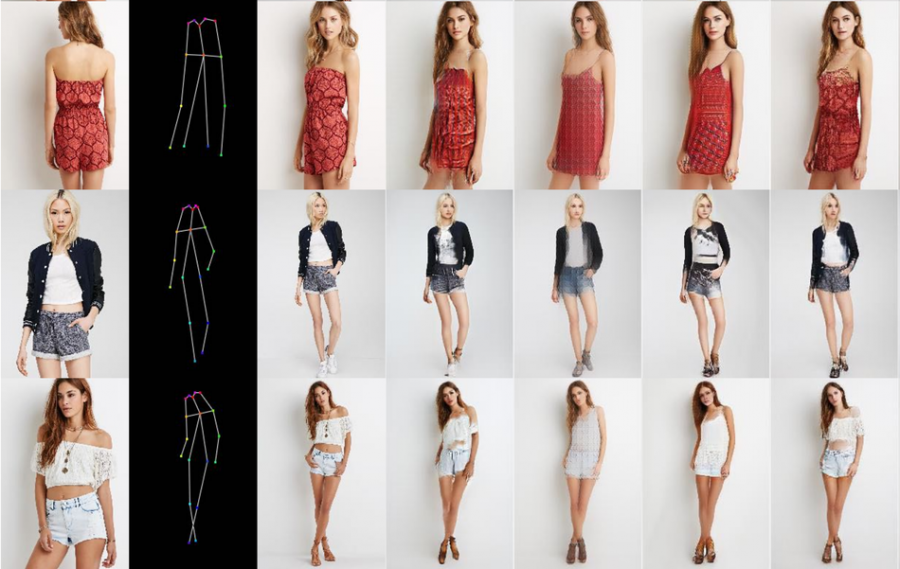
A group of researchers from Peking University and the Peng Cheng Laboratory have proposed a new method for person image generation that achieves superior results in image spatial transformation.
The new method called global-flow local-attention is able to do pose-guided person image generation by employing a differentiable framework which reassembles the inputs at the feature level. The architecture of the proposed method consists of two main parts (or branches): a Flow Field Estimator and a Target Image Generator. Global flow fields are generated by the flow field estimator network and are used by the target image generator to transform source features using local attention. The local attention is applied multiple times at different scales.
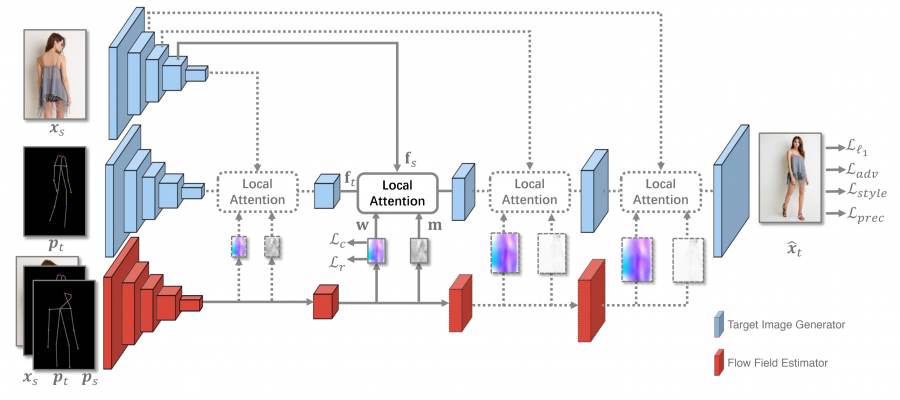
Researchers used two datasets to train and evaluate the proposed model: Market-1501, a person re-identification dataset and DeepFashion, a clothes retrieval benchmark. The method was compared to existing methods such as Def-GAN, VU-Net, Pose-Attn using evaluation metrics such as Frechet Inception Distance (FID) and Learned Perceptual Image Patch Similarity (LPIPS). Some sample outputs of the method are shown in the image below. According to researchers, their method is able to generate target images with correct poses while maintaining a lot of the details from the source images.
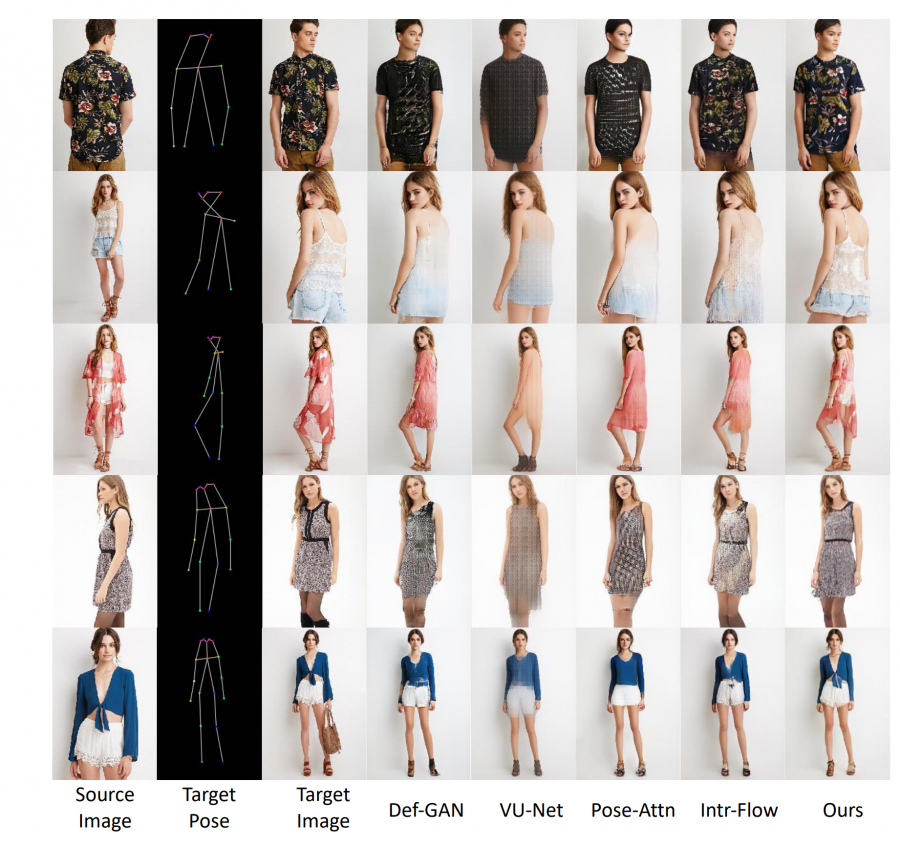
The implementation of the method was open-sourced and can be found in Github. More details about the model can be read in the paper published on arxiv.