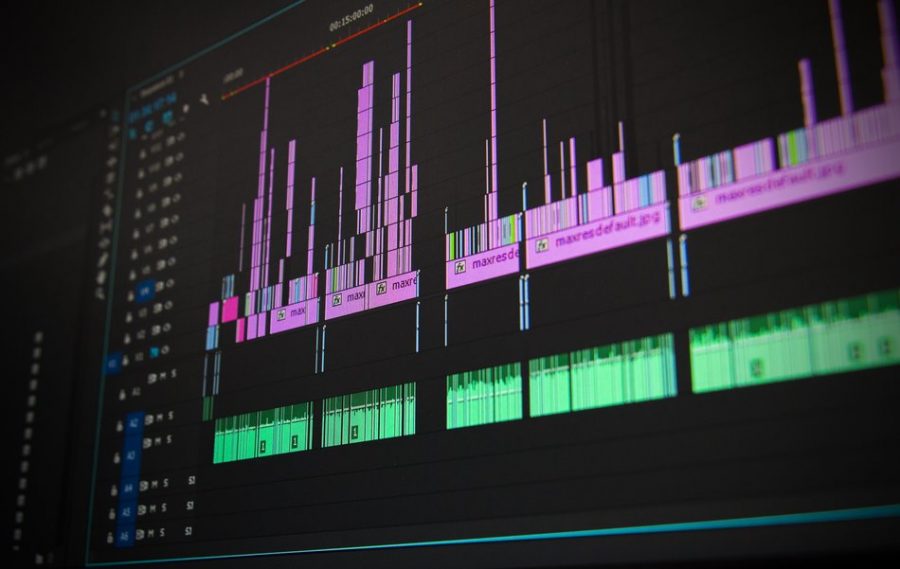
Researchers from Facebook AI have developed a new system that can do sound source separation to a very high level of accuracy.
The problem of sound source separation has existed for decades and it represents a big challenge for researchers. Previous works have had some advances in solving this problem but only to some extent and some level of performance and accuracy. The new method created by Alexandre Defossez, a research scientist at Facebook AI, is able to detect complex patterns in sound waves and therefore perform much better sound source separation using those patterns.
The method was called Demucs and it uses a U-Net-like network architecture to learn to extract waveform patterns. The proposed network is convolutional, meaning it takes Mel-spectrograms as image inputs. Demucs is ane encoder-decoder architecture that uses bi-directional LSTMs to take into account the temporal dimension of the input. The output of the network is a stereo estimate for each sound source.
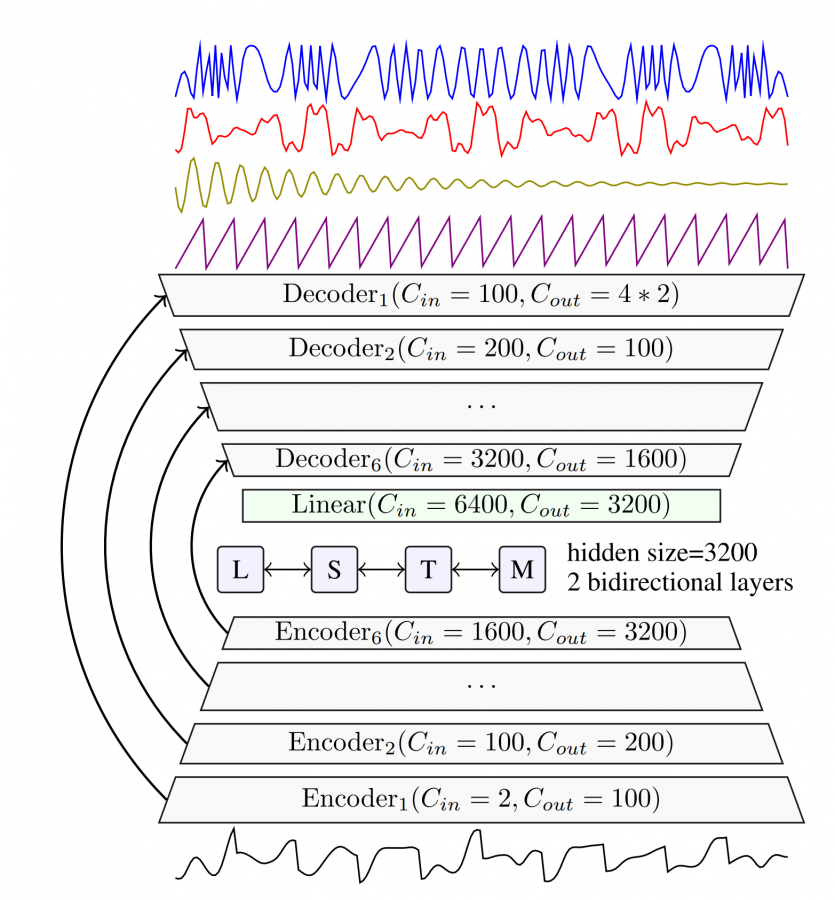
According to the researchers, Demucs significantly outperforms current state-of-the-art methods on the MusDB dataset – which is a standard benchmark for multi-instrument sound source separation. Also, human evaluations of Demucs show the higher perceived quality in the outputs as compared to existing methods.
Defossez says that Demucs has great potential and can be used in a number of ways from breaking down a song to its constituents all the way to improving the natural language understanding of virtual assistants.