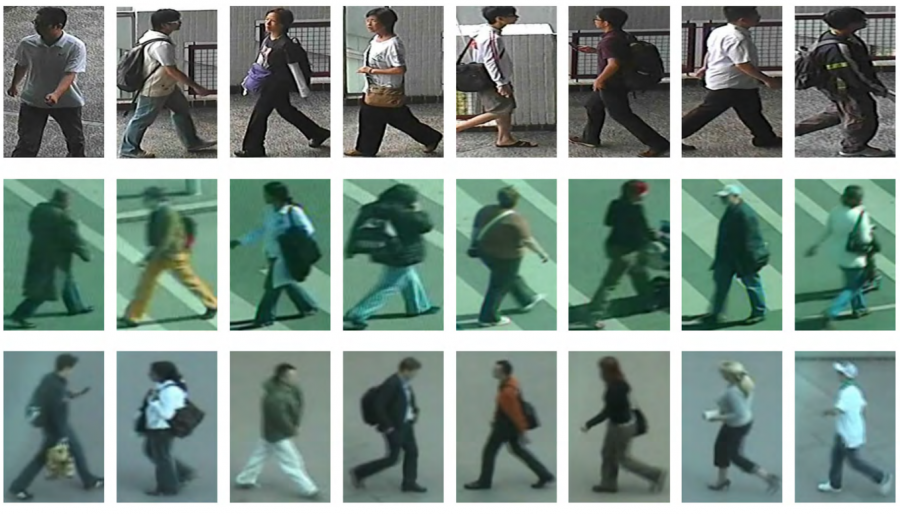
Researchers from the University of Surrey, the Queen Mary University of London and Samsung AI have proposed a novel neural network model that achieves state-of-the-art performance in person re-identification.
Person re-identification (or person reID for short) is defined as the problem of matching people across disjoint camera views in a multi-camera system. Defined in such a way, the problem of person re-identification is non-trivial and presents a number of challenges that need to be addressed.
From a machine learning point of view, large intra-class variations (due to the changes of the camera perspective and conditions) and small inter-class variations (for example different people wearing similar clothes) are two major challenges for person re-identification algorithms.
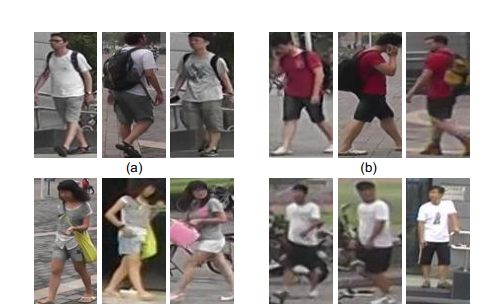
The researchers propose a method that overcomes these challenges by learning discriminative and omni-scale features. In fact, their neural network architecture allows learning features which capture a combination of multiple scales.
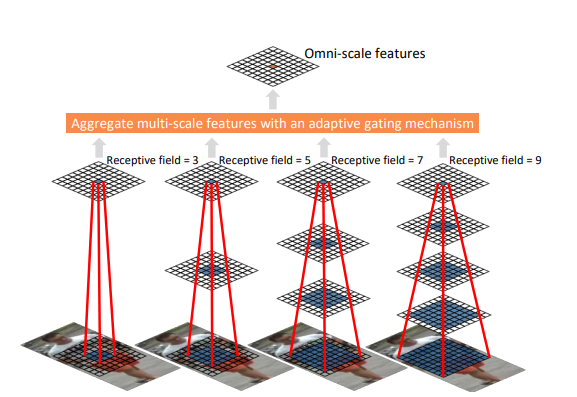
The proposed network contains a specifically designed residual block which is comprised of multiple convolutional feature streams. Each of the streams is learning features at a certain scale and an aggregation gate is dynamically fusing these features of different scale. The neural network model contains both pointwise and depthwise convolutions.
Researchers report that their proposed Omni-scale Network achieves state-of-the-art performance on six-person re-identification datasets. More about the neural network architecture can be read in the official pre-print paper. The implementation of the method was open-sourced and it is available on Github.