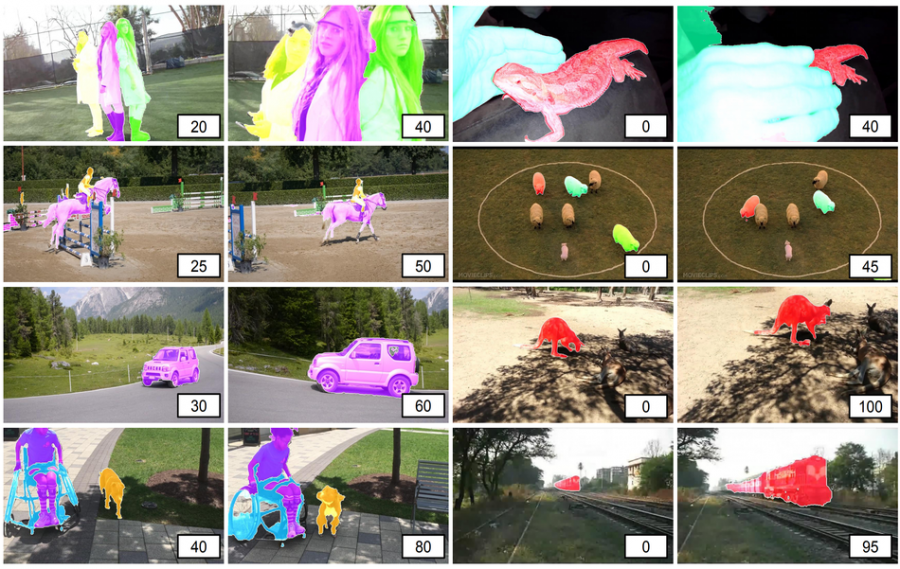
Researchers from the Visual Geometry Group at the University of Oxford have proposed a new self-supervised method for dense tracking which outperforms current state-of-the-art methods in self-supervised visual object tracking.
In their paper, named “MAST: A Memory-Augmented Self-Supervised Tracker”, researchers describe their new model and their findings comparing supervised and self-supervised methods for visual object tracking. In fact, they argue that self-supervised methods are actually superior to the majority of the supervised methods in terms of generalization capabilities. The new method that they proposed it’s called MAST and it solves the problem of semi-supervised video object segmentation which is a more fine-grained object tracking problem since pixel-level segmentation masks are being tracked instead of shapes, bounding boxes or similar.
MAST works by using a so-called memory bank where for any new frame as input, a query is computed for the present frame and keys for all frames in the bank. This procedure is repeated for all the frames in the training set until the encoder network is trained. After that, the supervision signal comes from the instance mask, and not from the image itself.
In order to tackle long temporal dependencies, researchers propose to use a two-step attention mechanism. In the first step, images are matched with the frames in the memory bank to obtain regions of interest (regions that potentially contain good matches). During the second step, these regions of interest are extracted and pixel matching is performed.
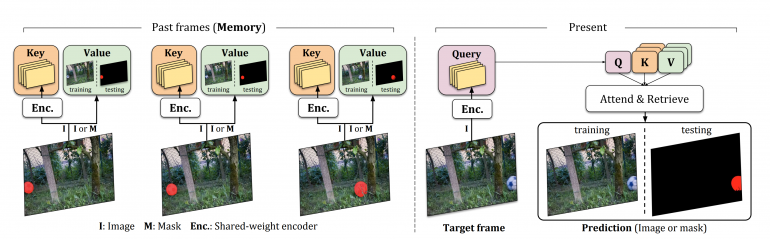
The model was trained using two large datasets: the YouTube VOS dataset, and OxUvA. The evaluations of the method were performed on two public benchmarks: DAVIS-2017 and YouTube-VOS and results showed that MAST outperforms all existing self-supervised methods.
The implementation of the new method was open sourced and it is available here. More in detail about MAST and the evaluation performed, can be read in the paper.