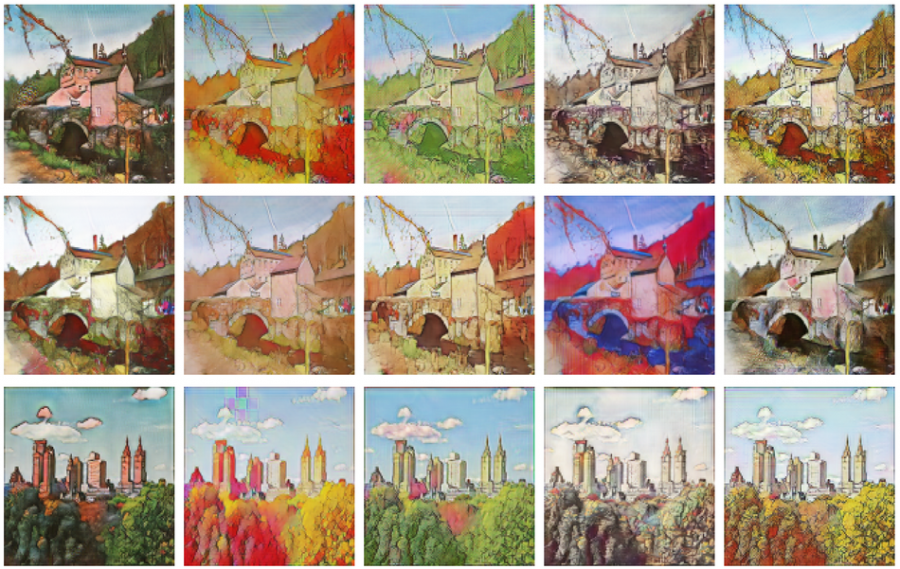
Generative Adversarial Networks have been applied to many domains in image-to-image translation. However, it represents a challenge for GANs to successfully transfer both style and content from one to another domain in image-to-image translation problems.
Researchers from the Hacettepe University in Ankara and the Middle East Technical University have proposed a new GAN model for image-to-illustration translation which tries to balance between the style and content transfer. They introduce a new generator network architecture that uses concatenative skip connections from the encoder to the decoder to produce a stylized image as an illustration, given a normal input image.
The new model was called GANILLA and was trained with illustrations from children’s books as a novel domain in image-to-image translation. For the purpose of training such a model, researchers first collected a dataset with illustrations containing around 9500 diverse illustrations from 24 artists. Researchers used around 5400 natural images from the CycleGAN dataset provided as the source domain in an unpaired image-to-image translation setting.
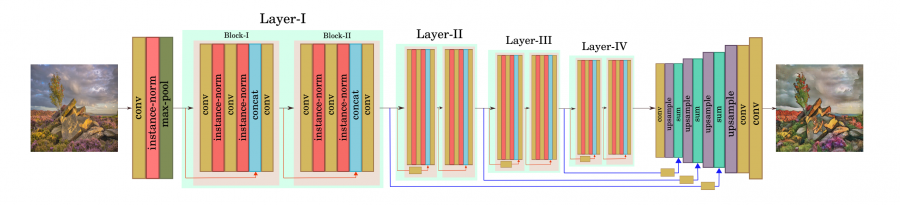

The trained model was compared with state-of-the-art GAN methods that use unpaired data such as CycleGAN, CartoonGAN, and DualGAN. In order to quantitatively evaluate the performance of the proposed model and compare it to existing methods, researchers proposed a new framework to quantitatively evaluate the models in terms of content and style transfer.
The evaluations showed that GANILLA outperforms existing methods on the novel illustrations dataset in terms of overall performance and balance between style and content. Some of the existing methods outperform the new method in the tasks of style or content transfer individually.
The implementation of the method was open-sourced and it is available on Github together with pre-trained GANILLA models. More in detail about the model or the novel evaluation framework can be read in the pre-print paper published on arxiv.