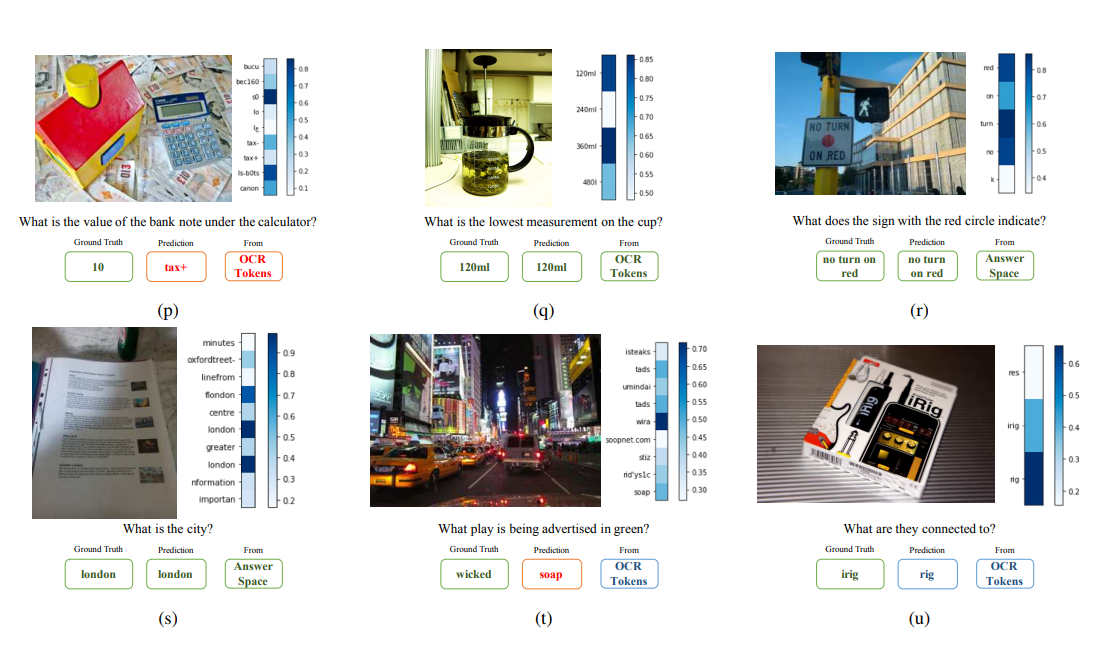
In a paper accepted to CVPR 2019, researchers from Facebook AI Research (FAIR) and Georgia Institute of Technology introduce TextVQA – Visual Question Answering models that can understand text and reason based on both the image and the text.
Researchers argue that existing VQA models are not able to read at all. In fact, all previous efforts in this field have been aimed towards scene understanding and object detection in images to provide reasoning and answers to natural questions.
It turns out that many researchers overlooked the necessity of text understanding, which is present in many of the images and answers to natural questions can, in fact, emerge from just reading that text.
Therefore, in order to tackle the problem, researchers first created a TextVQA dataset which contains images that contain some text and ground truth labels for the text present in those images. The created dataset contains more than 45 000 questions on more than 28 000 images.
Then, they introduced a new model architecture that takes into account text understanding and used the created dataset to train the VQA model to provide answers based on both the visual content and the text.
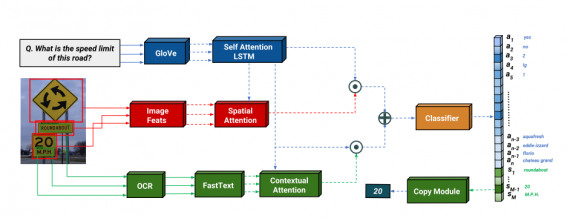
The novel framework was named LoRRA – Look, Read, Reason and Answer. Researchers showed that the proposed model outperforms existing VQA models on the novel TextVQA dataset since the latter are not able to provide answers based on text understanding.
The implementation of the proposed method was open-sourced and it is available on Github, as part of Facebook’s Pythia framework. The pre-print paper was published on arxiv.