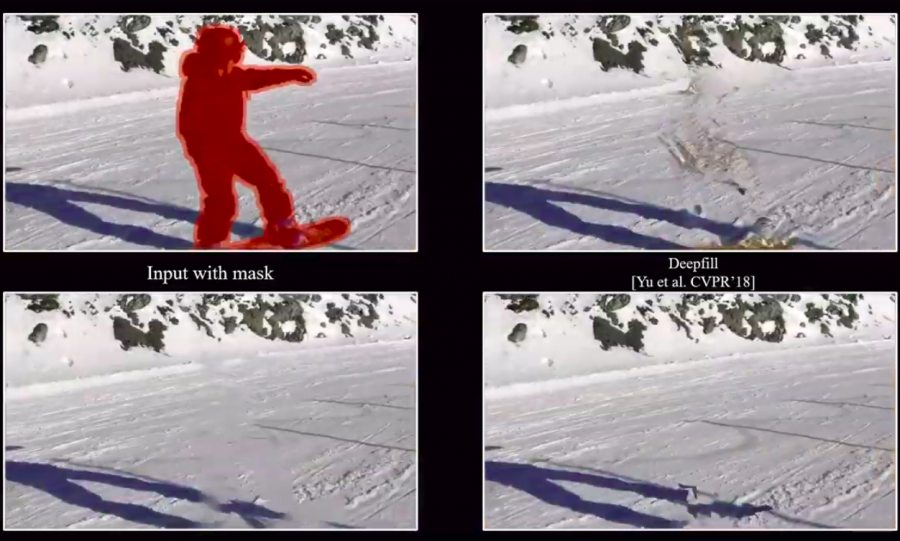
A group of researchers from the Chinese University of Hong Kong and the Nanyang Technological University has proposed a novel flow-guided video inpainting method that achieves state-of-the-art results.
Video inpainting is a challenging problem within Computer Vision which aims at “inpainting” or filling missing parts of a video. Many approaches have been proposed in the past but video inpainting still remains one one very difficult task.
To overcome the problem of preserving the spatio-temporal coherence of the video contents, researchers propose a neural network model based on optical flow. They reformulate the problem of video inpainting from “filling missing pixels at each frame” into a pixel propagation problem. Then, they designed a neural network model that is able to perform video inpainting.
In their method, they compute the optical flow field across video frames using the Deep Flow Completion Network. This flow field is then used to tackle the problem of pixel propagation along with frames in the video.
The proposed method was evaluated on DAVIS and Youtube VOS dataset and researchers reported that they achieve state-of-the-art results in terms of video inpainting quality as well as speed.
More details about the proposed method and the whole project can be found on the project’s website. Researchers released a video showing the performance of the method in tasks such as object removal from video, watermark removal, etc.
They also open-sourced the implementation of the method and it’s available on Github. The paper is available on arxiv.