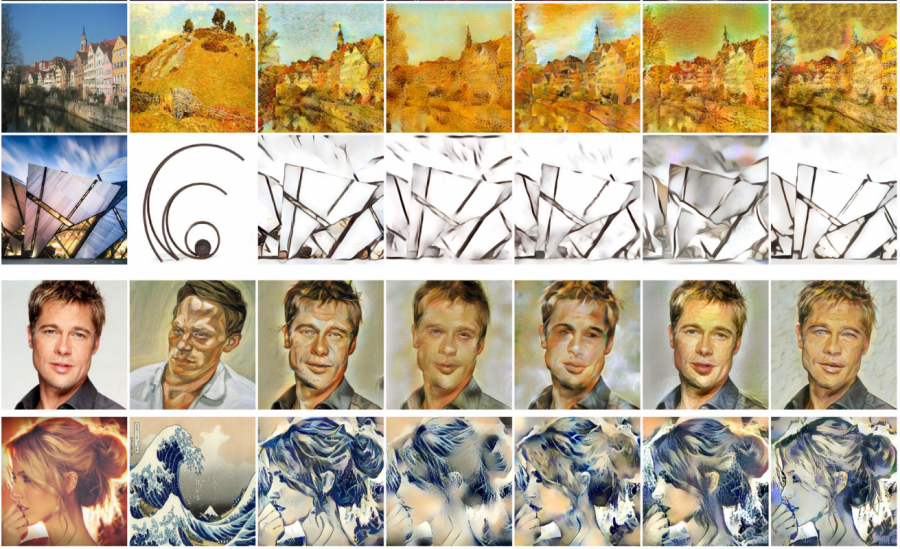
Researchers from the Artificial Intelligence Research Institute in Korea have proposed a novel neural network method for arbitrary style transfer.
Style transfer, as a technique of recomposing images in the style of other images, has become very popular especially with the rise of convolutional neural networks in the past years. Many different methods have been proposed since then and neural networks were able to solve the problem of style transfer with sufficiently good results.
However, many existing approaches and algorithms are not able to balance both the style patterns and the content structure of the image. To overcome this kind of problems, researchers proposed a new neural network model called SANet.
SANet (which stands for style-attentional network), is able to integrate style patterns in the content image in an efficient and flexible manner. The proposed neural network is based on the self-attention mechanism and learns a mapping between content features and style features by modifying the self-attention mechanism.
The proposed style transfer method takes as input an image of a person and a “style” pattern image to be used for the composition. Both the image and the style are encoded using an encoder network into a latent representation which is fed into two separate Style-attentional Networks. The output is then concatenated and passed through a decoder which provides the final output image.
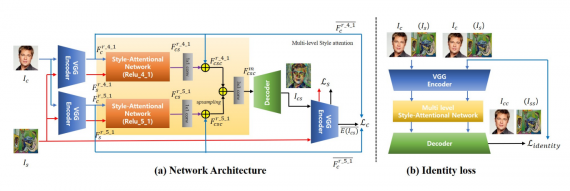
Researchers use identity loss as the loss function which gives the difference between the original image and the generated one. For training and evaluation of the proposed method, they used WikiArt and MS-COCO datasets.
In their paper, researchers report that their method is both effective and efficient. According to them, SANet is able to perform style transfer in a flexible manner using the loss function that combines traditional style reconstruction loss and identity loss.
Researchers released a small online demo where users can upload a photo and see the results of the method. More details about SANet can be read in the pre-print paper which was accepted as a conference paper at CVPR 2019. The article is published and available on arxiv.