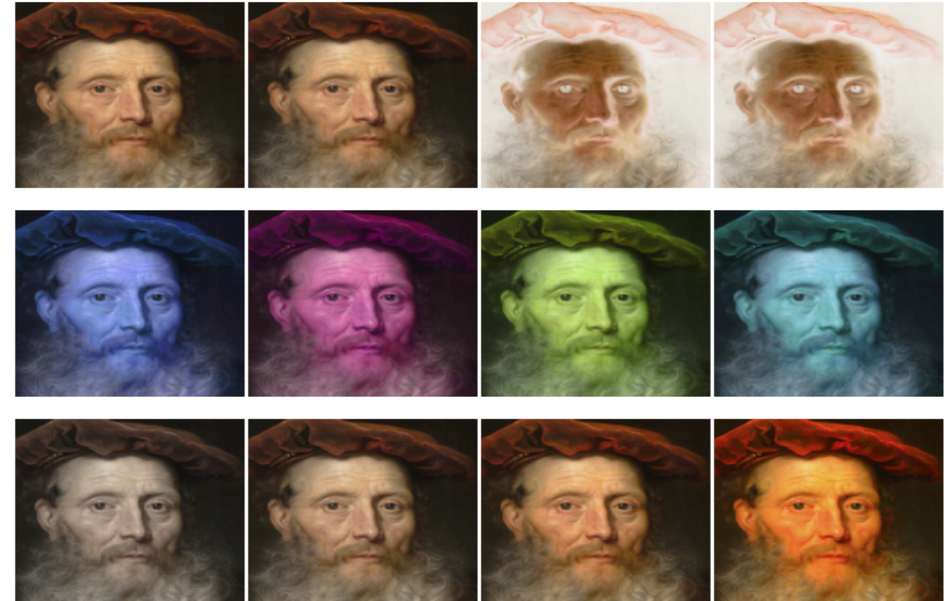
GAN networks achieved great success in the past few years. Among them, StyleGAN2 stood out as one of the most powerful generative models, which was able to model high-resolution images of faces and showed impressive results.
In a novel paper, researchers from NVIDIA propose an augmentation technique that improves the training stability and convergence of StyleGAN2. With this improvement, researchers were able to reach (original) StyleGAN performance with an order of magnitude less amount of images.
Their work builds upon recent research where Zhao et al. proposed a new regularization mechanism – balanced consistency regularization (bCR). Researchers showed that bCR has some drawbacks and indeed still leaks augmentations in the generator, and proposed a similar but alternative version that does not leak. The solution that they suggest is to apply a set of augmentations to all images shown to the discriminator and evaluate the discriminator only when using the augmented (not original) images. They call this approach, a “stochastic discriminator augmentation”. In fact, they went a step further and proposed to dynamically control the augmentation process and strength based on the overfitting degree. In the end, they call this “adaptive discriminator augmentation” or ADA shortly.
Researchers conducted a number of experiments to prove their claims and evaluate the proposed solution. First, they proved that bCR still leaks its data augmentations to the generated images. Second, they tried the ADA approach using several limited (or small-sized) datasets. And last, they also showed that CIFAR-10 is a limited benchmark dataset since they were able to achieve an enormous improvement compared to the current state-of-the-art method in terms of FID score.
The implementation of ADA augmentation was open-sourced and can be found on Github. The paper was published on arxiv.